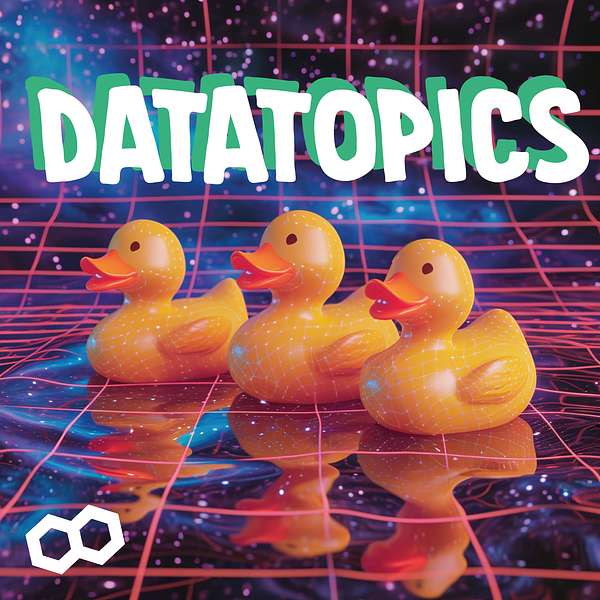
DataTopics Unplugged: All Things Data, AI & Tech
Welcome to the cozy corner of the tech world where ones and zeros mingle with casual chit-chat. Datatopics Unplugged is your go-to spot for relaxed discussions around tech, news, data, and society.
Dive into conversations that should flow as smoothly as your morning coffee (but don't), where industry insights meet laid-back banter. Whether you're a data aficionado or just someone curious about the digital age, pull up a chair, relax, and let's get into the heart of data, unplugged style!
DataTopics Unplugged: All Things Data, AI & Tech
#71 Navigating GenAI: How Organizations Must Adapt to Paradigm Shifts – Rootsconf Recap (part 1)
Welcome to the cozy corner of the tech world where ones and zeros mingle with casual chit-chat. Datatopics Unplugged is your go-to spot for relaxed discussions around tech, news, data, and society.
This week, we’re bringing you a special episode straight from RootsConf, our annual internal knowledge-sharing extravaganza! Hosts Murilo and Bart sit down with Tim and Ben, data strategy experts, for a lively chat about the state of generative AI as it transitions from a buzzword to a business tool.
Highlights from this episode:
- Generative AI adoption: Are companies finally moving beyond pilot purgatory?
- The environmental cost of AI: Can emerging techniques reduce its heavy energy footprint?
- Bridging the knowledge gap: What’s missing for widespread AI adoption in organizations?
- Future trends: How generative AI might reshape personalization and business processes in 2025.
Plus, we dive into the Gartner Hype Cycle and its relevance in understanding AI’s journey from innovation to disillusionment and beyond.
Get ready to dive deep into AI’s evolving role and its impact on industries, sustainability, and society. Hit play and join the discussion!
You have taste in a way that's meaningful to software people. Hello, I'm Bill Gates.
Speaker 2:I would recommend TypeScript. Yeah, it writes a lot of code for me and usually it's slightly wrong. I'm reminded, incidentally, of Rust here. Rust this almost makes me happy that I didn't become a supermodel. Cuber and Netties.
Speaker 1:Well, I'm sorry guys, I don't know what's going on.
Speaker 3:Thank you for the opportunity to speak to you today about large neural networks. It's really an honor to be here.
Speaker 4:Rust Data topics. Welcome to the data. Welcome to the data topics. Podcast Rust Rust Rust Data Topics. Welcome to the Data Topics.
Speaker 3:Welcome to the Data Topics Podcast. Hello and welcome to Data Topics Unplugged Deep Dive, your casual corner of the web where we discuss all about Gen AI, from hype to reality. My name is Murillo. I'm kind of hosting I'm not sure if I can say that but I am joined by Bart, at least for the well. Again, it will make more sense for the people that are listening. How are you doing?
Speaker 2:Bart, I'm doing.
Speaker 3:Good, good, good, good, we're also doing remote, but we have, maybe can we call it a Christmas edition of Tidotopics episodes.
Speaker 2:Yes, christmas edition, let's call it like that. We just released, last Thursday, our more or less end of year episode, a little bit of a look back at the year, a little bit of a look forward to us next year. And then last week we had our RootsConf my camera is acting up, again, remote issues but last week we had our RootsConf my camera is acting up, again, remote issues but last week we had our RootsConf, which is our internal knowledge sharing event, and you had the good idea to record some sessions. Indeed.
Speaker 3:So we did record a few sessions. So we thought that for the month of December we would be fun to bring them, share them with everyone, as maybe an early christmas gift. Can I say that? I'm not sure, well, maybe, but yeah. So we have a few sessions. So in the following weeks you're gonna hear, you're gonna hear them out from the roots conf there. Live, well, live back then. Uh, the first one what do we have for today, bart?
Speaker 2:yeah, maybe just before we dive into, maybe just a little bit of more information. So our internal uh knowledge sharing event, roots conf, happens once a year. Uh, we have uh tons of interesting uh talks by a lot of uh colleagues, uh on a lot of different topics, really ranging from AI, data engineering, platform engineering strategy, et cetera. So it's a very inspiring day Also, a nice atmosphere, nice vibes, and what Marilo did is, after some sessions I think in total three you grabbed these people by the collar and dragged them into a podcast room and then you had a small interview on the topic that they just presented. Right?
Speaker 3:Exactly exactly and, like you said, there was a lot of talks, also some workshops, so a lot of cool stuff. So we had to pick a few that would also match the timetables and also they're a bit shorter as well. Like you said, it's a lot of events For them as well, a lot of talks Not to keep people too long, but it was a lot of fun. It was a fun day.
Speaker 2:It's basically three micro-episodes right Of which the first one will be today.
Speaker 3:Yes, that is right, that is right. So then, what do we?
Speaker 2:have today. The first one is from Tim Leers and Ben Mellartz about generative AI, and they go a bit into what's all the hype and what is the reality behind the hype.
Speaker 3:Yes, really cool. Well, I really enjoyed talking to them. Indeed, it's a bit like where do we see this going? Where are the challenges? What are the things that are just hype? How we can think about AI going forward. What are the challenges, what are also some? I was also surprised by some of the information they brought. I think it's also because we are a bit in a bubble. We are in the data bubble, let's say, but I think, if you step out of it and look more holistically, it was a very enjoyable conversation, a lot of interesting parts. So, without further ado, let's.
Speaker 2:Let's do it. Let's do it All right. Cheers, let's do it. Let's do it All right.
Speaker 3:Cheers, Is it playing down?
Speaker 2:you know, or maybe not, it's already started, right, yeah, all right, cool Cool.
Speaker 3:Next. All righty, I'll start over again. Yeah, hello, and welcome to Data Topics Unplugged. Deep dive, your casual corner of the web where we discuss all about building charisma and confidence. My name is Murillo. I'm hosting this intro together with Bart. Hey, bart, hi, you know it's been a week already, bart. I don't know if you already can tell it's been really fast.
Speaker 2:The light changed.
Speaker 3:Yeah, I have some wrinkles now, some gray hairs. This is just an audio-only episode so people cannot see, unfortunately. Maybe like 2024, I'll be like all gray right.
Speaker 2:We're kidding a bit, because we're recording all these intros in one go indeed, indeed, indeed.
Speaker 3:So we're still in the roots conf recaps, right so?
Speaker 2:again. If you miss will be the the second one, the second mini episodes where merilo interviews uh, one of our roots conf speakers.
Speaker 3:Indeed, and if you miss, if you missed last week's episode, the roots conf is basically end of year event where we do a lot of knowledge sharing, uh, from more employees, uh, some workshops, a lot of cool stuff of day there in a special venue as well, um, and yeah, throughout the day I also grabbed a few people to talk about their presentations. So, if you're interested, feel free to check out the episode from last week from Tim and Ben Gen AI, from Hype to Reality. But that's not what we're going to talk about today or what you're going to hear today. Today we're going to talk about building charisma and confidence by Bram Bram de Koster.
Speaker 3:No, yeah, yeah, I wasn't sure if I was pronouncing his uh, nasty mind, but anyways, um, so, cool talk as well. A bit more on the communication part, which I also think is very uh, I also think is very interesting. I also I also take a lot of interest in the communication part and I also think is a part that is often overlooked when you're thinking of technical skills, but I do think that it makes a big difference.
Speaker 2:Definitely, I think, especially in the field that we're in, which is consultancy around AI data engineering platforms, where communication skills are key.
Speaker 3:Indeed, indeed, communication skills are key, right, indeed, indeed, and I also think that, even for professionals, I do think that the ability to speak well and present well, I think, will also advance your career quite a bit, because, yeah, the way you come across has a big like. Yeah, I think, in the end of the day, is how you impact other people. Right, how can you?
Speaker 2:and I think even like, at the very least, being conscious of these things, true, true, that helps uh tremendously, and I think that is uh one of the things that uh bram talks about, uh, indeed indeed.
Speaker 3:So the talk was also, uh, on the book that he also he, but I'm also shared and you're gonna hear in more detail that he's also someone that wanted to improve his public speaking, so there was also a book that he he read and he was able to extract some insights like the yeah, comfort, presence, power, warmed, as the foundations of building charisma. Also defining charisma, uh, like some processes that you can follow to to overcome these things very actionable as well. And, um, yeah, it was very interesting to hear from his perspective you know, what worked well, what didn't work well. Yeah, his perspective there I think he brought some different point of view that I also very much enjoyed talking to him and with that I'll let you with the episode. Thanks, y'all, enjoy the lesson Till next time. I y'all Enjoy the listen, until next time. I don't even know why I'm smiling.
Speaker 2:It's just audio only right. It's really distracting when my camera freezes. I don't know why. It's like yeah.
Speaker 3:Yeah, it's fine. It's fine, um pom-pom.
Speaker 2:Yeah, in DTMm it doesn't even matter which camera I use, right like there's so much effort from a camera yeah, but now I am, maybe, maybe flint is the way I see you, you know.
Speaker 3:So it's like oh, bart, are you okay? Is that you know? I look more tired with this camera, right I think so, but like the colors are the same, no, it's all.
Speaker 2:It's like 10 years, or maybe I'm just, or maybe I'm really like 40 I look like I'm Okay, but the color is the same on both webcams no, it's not like one changes the, because I think mine, the actual saturation is very different, right. Yeah, that's true. You know what is jarring when you go to the school to pick up or drop off your kids and you look around at the parents and you think old people. And then the second thought you have fuck, I'm the same age fuck.
Speaker 3:They have three kids. They're old as fuck. Anyway, let's get over my midlife crisis.
Speaker 2:Fuck, they have three kids. They're old as fuck. Anyway, let's get over my midlife crisis. Let's do the third one.
Speaker 3:Alex, if you can snip the correct parts there, there's gold there just say okay then let's get to it. Hello, maybe I won't Right before, I'll give a little pause.
Speaker 3:So you know it doesn't. Hello, welcome to Data Topics Unplugged Deep Dive, your casual corner of the web where we discuss all about the Hunger Games for AI. My name is Marillo. I'm hosting this intro together with Bart. Good day, good day, sir. And we're still on our RootsConf recaps, our little treat. So maybe for the people that haven't followed the last two weeks, bart, tldr, rootsconf.
Speaker 2:RootsConf is our internal knowledge sharing session, where we do a lot of talks on a lot of different topics, and a big, big thank you to all the speakers that were very passionate in bringing their ideas, their thoughts, their project that they worked on. And what Murillo did is that he, with three of those presenters or sometimes the talks were with multiple people three of those talks, he grabbed the people, drew them into a podcast room and interviewed them. Today is the third and last mini episode where we release one of those interviews, and this time it's with Sofie de Coppel, oare oage, I don't know, and this time it's with Sophie de Coppel and Wache.
Speaker 3:I don't know. I feel like there was a lot of expectation on me there.
Speaker 2:I don't know the last name.
Speaker 3:That's why I thought you were looking for my pronunciation. But it's bad because even his yeah, it's bad because even his uh, yeah, it's difficult because he's the only one right. His email is water, his slack is water wait, let me link to it are we gonna need to start over yeah, I think we need to start over.
Speaker 2:Yeah, I think we need to start over. Poor Warren. It would be weird if we don't Driesen, driesen, yeah.
Speaker 3:Driesen. Yeah, D-R-E-E-S-E-N Driesen.
Speaker 2:Yeah.
Speaker 3:Okay, okay, okay.
Speaker 2:All right, that's what you need to do. Actually, you can see it on the audio. Like you see, speak like a spike on the audio, but yeah. That's why, they do the clap with the movies.
Speaker 3:Ah, yeah, yeah, yeah.
Speaker 2:You would have no clue how the thing is called the clapping thing.
Speaker 3:Yeah, I know, I know the numbers. Yeah, yeah, I feel like it's a very uh no-transcript yeah, but there's also numbers on there yeah, okay, maybe, yeah, I'm not sure.
Speaker 2:You just I don't know anyways, okay, sorry, sorry no, go for it.
Speaker 3:Finish your thought.
Speaker 2:Finish your thought we'll be cool with. I'm gonna let you go.
Speaker 3:Okay, you share. After the entry, then, um, hello and welcome to data topics unplugged. Deep dive, your casual corner of the web where we discuss all about the hunger games of ai. My name is morello. I'll be hosting this intro together with bart hi. Hey, bart, um, I cut you off just before we started. Sorry, what were you?
Speaker 2:uh, you wanted to say something that it would be very cool if you would have this, uh that thing that they have in the movies to like start a scene like that, uh, the flappy thing which that does the clap numbers and the titles on there. I think that would be a nice prop to have. It would make you feel like just a little bit more important. Well, speak for yourself just I'm just the co-host right. Make you feel more of a like an like an actual actor let's make it happen.
Speaker 3:Bart, this is uh, actually for people listening, this is gonna be. I think christmas will just have happened, or will just will be, just be about to happen. I also checked, so, uh, merry christmas to everyone. Um, maybe this can be our Data Topics. Christmas gift the clapping thing.
Speaker 2:It could be All right, jacinta, let's see. It's time for the third mini episode today of our RootsConf interviews. Rootsconf is our annual knowledge sharing event, an internal event where we have a lot of our colleagues presenting ideas, projects that they did, the research that they did on a lot of different interesting domains. It's presented through talks, sometimes by a single person, sometimes by multiple people. What Murilo did is that, after these talks that he dragged some people into the podcast room with him, and then we've released these mini interviews a week at a time, and this week will be the third and final one. And what is this one about, murilo?
Speaker 3:But before, because I noticed that I haven't mentioned that I also presented one, and I just wanted to share a bit of what I did.
Speaker 2:Okay, okay, okay, go ahead, go ahead. Strike ups on honor.
Speaker 3:Yeah, yeah, I don't know. I just I just noticed that I feel like I'm talking about all these people, but I also wanted to to share a bit. I thought I had a lot of fun building the workshop. Delivering the workshop was very fun as well, and the idea was to kind of come up with two parts one well, each person basically gets a chat gpt and then you have to protect a password that is given by the system prompt.
Speaker 3:So the first part is like you have you kept to? Yeah, you can test stuff and you built your defenses. There's also like some programming that you can add to it if you want. And then the second part is that people try to capture each other's passwords, and I think the idea is also to bring a bit the experience like okay, how, how reliable are these models? How reliable are not these models? What are some things? We can defend it, what is not, and have a bit of a competition, healthy competition. I had a lot of fun, um, building it. I learned a lot of stuff in building it as well, and, uh, I also had a lot of fun delivering. I don't know what you, what you thought about it.
Speaker 2:Part it was really cool. It was a bit of a it wasn't teams to attack all our teams and you had a bit uh, we were all in the same room, so that altered a bit to the effect. It was a bit of a gamification around jailbreaking, right. It really gave people a very intuitive feeling on what is jailbreaking and, at the same time, really actively trying it out. Really cool how you set it up.
Speaker 3:Yeah, it was cool. I feel like he went by really fast. I wish I had more time. I think in the end I still ran out of time, but uh, I think it's. I usually run out of time, but that's not what we're here to talk about. What we're here to talk about is the gen? Ai showdown, so actually it was called the hunger games. So ai, hungry games, or something by sophie, the couple and morat race yes um, so what was their talk?
Speaker 3:basically they, they had a, they had some games, basically, and they took the big lms, I think gemini, the entropic one, which I think is claude they use claude, sonnet, uh and chadT, I think that was it. I don't know if that was the fourth one and basically they had some different games around it. So, for example, one is that they had all the models play the Advent of Code, which for people that don't know, is basically Christmas themed coding challenges and they see how. What's the model that went the furthest? There they also had one that was like there's a game called, I think, mr white.
Speaker 3:I want to say that, um, basically, each person gets a word and then one person gets a similar word or like a blank word, and then every person describes the like, gives one adjective about that word, but the person that doesn't know needs to make it up, right, um? So they did something like this with lm. So there, each lm had like a turn to to, yeah, describe it, and then, after a round or five rounds or something, the everyone needs to vote who they think mr white is. Uh, so they did also with lms.
Speaker 3:Indeed, they also did. One was a find a human? Uh, so basically they had questions like again like a round of uh around the table kind of thing, and then we had one volunteer from that was watching the session to to try to trick, you know, try to give a very chad gpt like answer and then, uh, then everyone votes who they think the human is. Uh, so a lot of like little fun games like that. You know, um, that kind of highlight the different components.
Speaker 3:So, for example, the anthropic models were also here, and also my experience, but also what I see in blog posts and whatnot, that the anthropic models are the best ones for programming today or the ones that look like they have the best results. Um, this was also the model that went the furthest on the advent of code, but it didn't do better on the other ones, right, yeah, so, um, also it was a bit funny because, uh, sometimes talking to them, it felt like some models they had a bit of a personality, a bit like open ai was a bit more um, show off, like it would really say like, oh, yeah, because open ai models can do this, this and this, um, so it was, uh, it was, it was. It was. It was very interesting to hear their insights here and there, so it was very cool.
Speaker 2:Very cool talk as well let's go and listen, let's do it all right, thanks everyone merry christmas, happy new year enjoyed holidays.
Speaker 1:Yes, you have taste in a way that's meaningful all right, so I'm here with ben and tim at the roots come.
Speaker 3:Hey guys, hello hi how are you so? Um then, you've been. You've been on the last year's roots conf. You also did an appearance here, and there you said, oh yeah, I'll come for the regular one, and then you never did. Anyways, tim, is this your first time? It is my first time it is right, okay, cool. So maybe for both of you would you mind introducing yourself quickly for the people that do not know who you are Sure thank you, ben sure.
Speaker 1:Thank you, ben. I'm tim.
Speaker 4:I'm the generative ai lead at data roots, which means I help our customers figure out what they can do with generative ai, whether it's strategy or implementation I'm ben uh working at the data strategy unit for about two and a half years now, focusing on delivering value and making sure that we achieve impact with everything we we do and build um. So yeah, that's a short introduction, I would say data strategy, so um you also mentioned strategy.
Speaker 3:Is there an overlap in your, in your roles?
Speaker 1:you say all the time we like to work together because, yeah, best friends forever absolutely that's an understatement Okay, hold on.
Speaker 4:We did work together a lot the past few weeks and it's definitely been a pleasure, to me at least. I think we have a lot of shared interests, mainly being Gen AI, and there's a lot of work on the plate.
Speaker 3:I can imagine like two talking. Like Tim is like Gen AI, gen AI, and you're like data strategy, data strategy.
Speaker 1:And he's like yeah, yeah yeah, cool. That's pretty much the essence of it. No in reality, there have been so many customers who want to talk to us first about strategy.
Speaker 3:And so, naturally, we're some of the first people that get to talk with the customers about it. Maybe a quick question then, before we dive into your RootsConf topic what would you say, is the state of gen ai these days? We are to right now it's in november 2024, right, I think these things go very quickly. Um, what would you say like businesses are at, do you think there are like, is it just hype? Is it uh? Are they actually building stuff that is uh already going to production? Is it more poc? Is people interested but not trusting? Is there? What would you say?
Speaker 4:I would say, uh, many, many topics you are referring to is something that we addressed during the talk. Okay, um, and I think there's two sides to it. Um, I think many people were optimistic and skeptical, um, and we we try to understand the reasons for both. We also gave some general updates on the state of of gen ai, talking about, for example, the huge energy consumption, but also the convergence in model performance, the new pricing tiers, um, also discussing the, the economic potential, some typical use cases, um, so so, talking about the state of gen ei in general is is quite difficult, yeah, um, but I think, if you take a look at at the bigger picture, tomorrow it will be exactly two years ago when chat gpt launched, and we already expressed today that there is a world before and after ChatGPT in terms of democratizing AI. Everyone with an internet connection suddenly is interacting with AI Actually, not everyone, something that Tim mentioned but it's truly a paradigm shift and that's something that we've been discussing too.
Speaker 4:Why's truly a paradigm shift? And that's something that we've been discussing too. Why it's a paradigm shift, the big shifts that have been taking place, and I think in the coming years, there is a lot of work to do and we need to be there as Data Roots to help our customers in asking the right questions and then trying to answer those questions. But, yeah, probably Tim has something to add to this. Asking the right questions and then trying to answer those questions, um, but yeah, probably tim has something to add to this yeah, but we only have 20 minutes and we could go on for an hour about this no just taking a step back.
Speaker 1:Your question is broad. Um, there's no such thing as generative ai as an isolated technology. It it's everywhere, and so it's difficult to necessarily express what the state is. It's also evolving so fast. I think Ben pictured it quite well. You asked the question about where companies are at, and that one is probably easier to answer in the sense that most companies today are moving from pilot purgatory to something resembling deployment, maybe for people that uh are not as familiar with, like the life cycle, these products, what would you say is the?
Speaker 3:how would you describe in a eli five, like explain, like I'm five way the pilot purgatory, versus like uh, what do you call it? Like deploy, what do you? Deployment scaling, essentially putting things into business as usual yeah, so for people that are maybe not in it, not in tech, actually five or like actually five years ten max, all right, ten max not far from my mental age anyway.
Speaker 1:So yeah, so most of the time it's been experiments up until now. There's some exceptions. Some companies were already doing structural initiatives. A A lot of those experiments failed. Some of them were very successful, very impressive, and now the question is how do you bring it to the rest of the organization, to other departments, and use it actually in practice, with all of the unreliable aspects of generative AI being mitigated?
Speaker 3:Maybe a question also. You mentioned some of them fail. What are common causes for failure in these projects?
Speaker 1:Yeah, to begin with, I think there's digital maturity in general. So a lot of companies they have a lot of business processes that are not necessarily explicitly being captured in data, so there's a lot of implicit knowledge in the organization and you want to suddenly put a process or part of that process into AI, but you need access to the right data to actually make that happen. And on top of that, then there is data maturity in general, meaning you might have some data somewhere, like maybe Excel sheets all over SharePoint or something. Good luck using those.
Speaker 1:There's going to be a serious effort required to actually get that working for a lot of use cases.
Speaker 3:Cool, and maybe we've been dancing a bit around the presentation Can.
Speaker 4:I add something to the previous.
Speaker 3:Please do.
Speaker 4:I think also in terms of GNI literacy, and then policies and so on. There's still a big uncertainty around the topic, and I think for a full organization to benefit from the advantages of Gen AI tools, I think it's also necessary to train people, to make them understand how they should interact with it, to make them understand the inner workings, and this is something that organizations have not yet done so much, in my opinion, um, and I think they are still trying to figure out how they should form a policy like, for example, can we put this data in a chatbot, yes or no? How should we interact with this chatbot? How should we deal with the output of it?
Speaker 3:so I think there's also a lot of work on the plate on that side yeah, maybe on that piggybacking, on that I also and this is my personal view I don't want to hear if you agree, it's not just the literacy, but it's also having the right people. Right because, because I think maybe if there's an engineer that is playing with these things, that is trying, then that person understands really well, he still needs to get to the decision makers to really say this is something we need to invest. This is not something we need to invest. This is something that we should take care of. This is something we can go fast. This is something we cannot go fast.
Speaker 3:So I think, personally, in my experience over the years, what I've noticed is like I need to pitch the right idea to the right people at the right time, and I think that's why I also think these things take a bit longer. Right because, also, a lot of the times, these key decision makers, they're also very busy people, right, so it's not like you can just snatch the time here and there. They also they're they're splitting their attention across multiple things. Right, would you? Would? Right, do you subscribe to that? Do you think this is also? I'm also wondering if there's a. What are the people that are playing with Gen AI these days. Actually, maybe one thing that raised my eyebrows here, like you mentioned, that not everyone is touching Gen AI. Maybe you want to dive in a bit more on that, like what do you mean by that and why are they not?
Speaker 1:should have been at the presentation, really I should, I should um no. So, if you look at the numbers, less than half of the people, or approximately half of the people um across countries like what was it? The uk um argentina, us, a couple of others. Half of the people haven't heard of ChatGPT.
Speaker 1:And of the people that have tried it, most of them don't come back. And of the people that do come back to ChatGPT, start using it again, they use it once or twice and then they stop using it. And then there's a big portion of people that use it monthly. If we look at the amount of people that use it daily or weekly, it's actually quite small. It daily or weekly? It's actually quite small. It's like less than 20 percent at least. Like talking chat gpt specifically generative ai, probably the numbers are a bit broader if we start including stuff like, I don't know, dali, image generation, whatnot. But overall there's actually fewer people using it consistently. It's also what you see in organizations.
Speaker 1:When you start deploying these types of tools in practice chat, gpt, co-pilotini what happens is people use it once, twice, try to experiment with it a bit. It doesn't work. They move on because it doesn't work for them. There's a bit of a gap there on the product side, meaning that there's a lot of friction starting to use this thing and getting it to give you the answers that actually make your life better. But there's also a bit of, of course, work to be done in helping people become better at using these tools, but where we meet in the middle is a topic for discussion yeah, I'm actually curious about that because so, in my role, I guess the the clearest um gen ai use I I have right is on coding um.
Speaker 3:I mean, I like it, it helps a lot. But I also think that, depending on the tools you use, you have to also adapt a bit to it, right, like, for example, you have to remind yourself that, instead of Googling something, maybe this is something you can just ask right, and maybe there is something that you shouldn't ask right. Maybe it is something that you should Google, right. You should google right um, and I think. Or, for example, uh, with the llm coding assistance, you can also, uh, add snippets of your code and put it in the context, right. Or you can say, actually this you want to, I want this results to be based on web search, right. Or you can say, actually, look at everything on this file. There are a cursor that I'm using these days. It also has embeddings on your code base as well, so you can ask questions about your code base. So, for example, if you write code that I like, what, what, where do we define this or why does that happen I can actually ask the lm and you look at the code base, but like, because this, this was never an option before.
Speaker 3:You also need to train yourself a bit to use it right, and I think it's almost like any new tool takes some time to getting used to it right, because you, we're we are still creatures of habit and I think sometimes, yeah, do you want to spend twice as much time now to do something new, but then in a month it would be half the time, or do you want to just spend a regular amount of time and just make sure you get it done, and I think there's a bit of a uh. I mean, that's where I imagine that the hardship comes right. If someone tries it once, they're like oh, this is good, this is cool. Okay, this is bad, maybe forget about it. Is there something more to it? Or is it more like the habits that we have, that to add something new? Even if it's quote unquote better, people still have a hard time to adopt it.
Speaker 4:I think there's many answers to that. But I think there's many answers to that. But I think that it depends on the context of the people too, because in our context, within a data environment, and also in how we adopted digital technologies and so on, or age, or demography and so on we tend to use digital tools more and therefore, I think for us, we easily start using these tools and when you say we, you mean like yeah, like let's say, merlot, uh, tim and I so uh just those three.
Speaker 4:No, yeah sorry, alex, no like comparable, uh comparable background or professional life um we're still in a bubble right I think sometimes it's easy to forget, but like we, all work in a data company.
Speaker 3:We're all like exactly. I mean we, we were born in the internet. I remember my dad saying that when he was writing his thesis he had to go to the library, get a book, look at the table contents, find it now go to the page. And now it's like yeah, and now it's, there is image gpt right, I think, for us the relevancy is super high.
Speaker 4:it's the same for students the relevancy is super high. It's the same for students the relevancy is high because they want to write a paper. Indeed, and for some people the relevancy might be a bit lower, or they just don't know that it exists and they're not aware of the capabilities of the tool.
Speaker 3:And you mentioned so you mentioned, tim, earlier that there's a bit of a trade-off, right. There's a bit of like we need to. People need to maybe push themselves a bit to touch the new tool, but also the tool should also evolve to meet people where they are right. Maybe a very silly example, I don't know If you embed Copilot on the email client and people don't need to switch tabs because it's already there, maybe it's like a nicer autocomplete, right? Maybe it's something that. So, as a dummy example of getting tools closer to the people, right, is there any strategies, any any? You have any thoughts on that, exactly Like how, what kind of, what kind of questions we actually need to ask or what are the things we need to invest? If this is the thing that will unlock Gen AI for a large group of people, what are the questions that we should be focusing on?
Speaker 1:I think the framing of that question is interesting. So I'd like to take a step back because actually what you're describing is in the existing way of working in business as usual. How can we make people adopt these tools, like whatever they're doing, maybe writing emails and so forth? I think there's actually like two ways to look at this. One we stay within business as usual, we keep doing things as we are, but we find ways to increase efficiency, to increase the impact of what we do with generative ai. You gave a great example efficiency gains by bringing co-pilots to the email and be faster at writing emails. It hasn't happened to me.
Speaker 1:To be honest, impact is okay. We're building AI models using random force today. Maybe we can use generative AI in some way to supplement that, make the accuracy higher. I'm not saying that's typically the case and because of that you have a higher impact. But then deviation from business as usual is where we look at how we're doing things, how business processes work today, which steps we take to go from A to B, from A to Z probably, and reconsider that. Think like, okay, where can we actually just do things differently entirely? And today we're very much in a stage where people, but also enterprises, are in that first pattern of focusing on business as usual how can we make it more efficient, more impactful? And only later, typically, you start to see people moving more and more towards that latter phase where they're thinking of how can we do things differently? What actually changes fundamentally?
Speaker 1:So, the way that I look at generative AI is like a platform shift, which is we're trying to figure out how we can organize ourselves around generative AI, like we had mobile first, we had the internet, we had personal computers. Generative guy is very similar in that sense. We're just very early, and so today we're figuring out questions like should we meet people in the middle? But maybe the question is isn't treating people in the middle? Should we just redesign the whole thing?
Speaker 3:I see, and so right now you think we're. Are you saying, if I understood correctly, that we're in this transition point of going from just increased efficiency on the old way of doing or looking for the new way of doing, or would you say we're still more on the old way or more in the new way? What would you say we are, or what do you observe?
Speaker 1:we're currently still just figuring out how we can use it to be more efficient, impactful for the most part. There are exceptions, of course. There's some industry where it's very clear what the transformative version of whatever we're doing looks like, some of it somewhat concerningly so. But to zoom in on your original question, which was more about you know, how do you think about where to focus, like where to? How do you decide whether you meet in the middle or not?
Speaker 1:I think if you look at the types of tasks in organization, you have a bunch of different tasks and business processes that occur very frequently. So you have like a distribution, let's say, of tasks. You have some that happen very frequently. There's ROI, because it happens a lot, those tasks. So there's sense in automating it. Maybe the digital maturity is enough, data maturity is enough, so it's a very good target for using Gen AI to some extent in automating it. But then there's a very long tail of tasks and business processes that are super infrequent because of the complexity of doing business, because maybe there's no standard operating procedure and so forth, and there it's much more difficult to do that, because maybe there's no standard operating procedure and so forth, and there it's much more difficult to do that, and that's actually where the whole co-pilot, saas-like way of working comes in. It's about personal assistance for people versus standardized solutions for things that we do very frequently and where we build products for.
Speaker 1:So, essentially here's a general chat interface. Maybe you can do something with it. Learn to do prompt engineering for the long tail of tasks that are infrequent in the organization. And then there is the bulk of tasks and business processes that happen a lot. They create a lot of value. They're important to the organization, so let's build a product for that or buy it.
Speaker 3:Yeah, so it's like specificity for the things that are very common and generic something-purpose for the things that are less occurring sure okay, cool, interesting. So we talked a bit about the, the, yeah, maybe the state of gni. I know that also, gni more and more becomes a big, big topic, right, um, we talked a bit about, uh, the adoption of gni, you also mentioned um the. You mentioned security or efficiency, as well the environmental impacts of that yeah, both topics are interesting to discuss.
Speaker 4:I think let's first talk about the environmental impact. So I think it was 280 households yearly electricity usage that has been used used to train GPT, for that's huge. Of course, we also talked about big tech players thinking about even building their own nuclear plants, which is crazy so that's really crazy.
Speaker 4:Dedicated for these things, right yeah, so, um, definitely, I think in the coming months and years there will be a lot of news on that too. The good thing is that some technical measures are being taken to techniques like distillations and so on, which help in reducing the environmental impact, but still it would. If you think about it, if you look up the numbers.
Speaker 3:To me it's not being spoken enough about yeah, maybe also you mentioned the techniques before we tackle on why this doesn't get as much attention. Uh, open ai 01, the strawberry, right, like it. Uh, basically, from what I understand, it has a chain of reasoning. So, basically, when you're asking a question, it takes a bit longer because it has a lot of iterations, right, and in a way, the compute cost increases when you're asking questions, when you're using the tool. But I think that when you were training, it was so before. When you're building the tool, it was less expensive. When you were training it was so before. When you're building the tool, it was less expensive. That's what I remember seeing, and there was a, I guess also from opening.
Speaker 3:I know that these things are very open, right, but I think the premise was that you can spend less time doing training for more compute during inference and you would still get very good, if not better, results.
Speaker 3:Is this a solution? Because I'm also wondering, with the adoption of these models, if we have a lot of people using these things that are very expensive, does it offset the training cost, does it not? There's also the whole thing that like yeah, maybe if you train the model once, you can also the transfer learning, right. I'm not sure how realistic that is, but, um, are there any solutions in the space or is? Do you think this is uh? Do you think that, as we evolve when you models, this will not be a problem anymore, or do you think that it will still be a problem and we just need to get more data, more energy, like these companies are doing? I mean, I know I'm very in the dreamland here. I was like it's very like, like it's very hard to to to uh, make any predictions here, but do you have any opinions or thoughts on this?
Speaker 4:it's a very good question, difficult one to me um I'm happy to take this yeah, I think it's better if you take this one.
Speaker 1:So, um, there's, there's two things I think that I want to address there. You talked about opening i01 and whether their, let's say, technique could be interesting for reducing at least performance to energy usage, whatever that ratio is today. And then the second question was more broad, like what? What are the, the potential pathways?
Speaker 3:yeah, so also because you mentioned that the energy question is a problem today, I guess the the broader question is do you think it would still be a problem in two years from now? Or do you think that techniques like oh one or could be distillation or could be things, you think these things would catch up?
Speaker 1:Okay. So maybe just for context, the reason why we're hitting these issues right now, like the physical limitations of what our current power grid can do for us, is because all of these companies are overinvesting. They're overinvesting into GPU infrastructure because they think it's better to overinvest right now. So overinvest means we won't get any actual return on investment for the next few years, but we're still doing it anyways. They're doing it because they want to win the next platform shift. They don't want to be left behind. That's why they're doing this, more or less, and so what happens is that they're finding out that they're hitting the limits on where you can actually build data centers, where you can build places with a bunch of GPUs, clusters, and also where the power is affordable enough, and so there's this huge competition indeed to get the best locations. And so that's why you're seeing this huge push right now, because actually, if we look at consumption, probably we would have been somewhat okay with the capacity that we have today, but they're just preparing for the next platform shift, trying to be ahead of everyone else. So they because you're limited by your computer. So that's one thing.
Speaker 1:I asked for your question about open ai. I think open ai is the the furthest thing from an example about how to be energy efficient. Simply because their philosophy, their core philosophy, is skill. It's just about skill. O1 is essentially let's train an end-to-end chain of thought, reasoning model. Let's just take all of these system components or all these prompts that we typically have and just let's try to put it into a model artifact and that's their approach overall. So their philosophy, at at least, will never be about energy efficiency. At least it hasn't been, historically speaking. Will it actually help with energy efficiency?
Speaker 1:also very doubtful okay because what you're saying is, potentially it's more performant. The amount of energy that you put into it to get its performance might be better. You need less energy to get the same performance, let's say. Could be true, but there's probably a lot more better ways to do that. But, of course, if you can improve the general capabilities of your model, make them better and you pay the same energy price, then yes, of course.
Speaker 3:So there's, there's multiple angles to this yeah, I know, and I think you said we can talk a lot like jni is like all the effect and all these things.
Speaker 3:Maybe, to wrap it up, you mentioned well it's not talked enough the the environmental right. So what are the main things you're concerned about, the environmental consequences of this? Why are we not talking as much in your opinion, and what would you like? And so, just as a close's a lot of questions. It's a multi-step question. Um, what would you like to see in the genii space next year, by the end of 2025? If we sit down, what would you like to be able to say?
Speaker 4:I think the the main reason that there's not being uh spoken about the, the environmental impact. It's because there's so much going on in the field. The advancements keep on stacking up, um. We keep seeing new ai assistants even close to home, like salando albert hen, even, um. So I think the the hype has been too big to talk about the downsides of it, and if we take a look at the the gardener hype cycle, we can actually see that we're on a tipping point going to the, the disillusionment, uh space, I think um, maybe for the people that don't know, the gardener, uh, hype cycle, yeah, it's basically we can put on the show notes as well.
Speaker 4:Yeah, very shortly. New technologies, disruptive technologies, follow a certain pattern when it comes to expectations, and first there's like a super big search in expectations and then it goes all the way downhill because problems start popping up and the ROI is not there, and so on and so on, and then, when the real value has been identified, we start working on that and then it goes steadily up again. So right now, with Generative AI, we just had the peak of high expectations. Why? Because there's been a lot of investments in it. Think about NVIDIA, but also OpenAI, the acquisition of Microsoft and so on. There's been a lot of investments in it. Think about NVIDIA, but also OpenAI, the acquisition of Microsoft, and so on.
Speaker 4:There's been a lot of hype because there were use cases, like the one from Klarna, which we have spoken about, other AI assistants and so on. We've been using it ChatGPT, for instance. So there was a lot of hype and now more and more questions start coming up about security, about safety of data, about bias, hallucinations and so on and so on. So I think for the next year for me, I hope we can start identifying we already did with DataRoots but that we can help organizations in becoming Gen AI capable it's a word we've used often the past few weeks Gen AI capable. What does it entail? What does your team need to look like? Which types of skills do you need? Which types of questions do you need to ask? What should your focus be on? So on, and I hope that, with data roots, we can establish a clear offer in that that we can have good frameworks, good practices, which have already been built, but that we can keep on on working on that full trust in you guys.
Speaker 3:Well said, well said. And what about you tim? Maybe to wrap it up, bring it home.
Speaker 1:Sure. So, jumping a little bit back to what you were saying before about energy, I think actually what is going to be potentially a driving factor for one of the most interesting evolutions of 2025 is the fact that we've had orders of magnitude improvements in efficiency because of open source, because of research labs. So we could not have imagined running a billion parameter model on our cell phone two years ago. We already moved so fast so far. So actually on edge is going to be a bigger thing.
Speaker 1:It's what we're seeing already with Apple intelligence, I think to some extent, and I think that shift is going to continue because we're seeing already with apple intelligence, I think to some extent, and I think that shift is going to continue, because we're going to be able to put more performance into fewer parameters and into fewer flops, into fewer compute units that we need. So on device is real and on device unlocks a whole new range of possibilities because suddenly, one, your energy costs are less of an issue, but also you can use it just much more frequently. You're just, you know, it's all distributed across these people's devices and that unlocks really interesting things. And I think one of the most interesting things is going to be personalization. So I think we're going to see, maybe in 2025 already, the beginning of hyper-personalization. So really personalizing your digital experiences, experiences, whether it's ui, whether it's the way the chatbot answers to you could be many things, but I think that's going to be huge. Might take a bit longer, though, but would be nice to see that already next year.
Speaker 3:And see that we'll come back here next year and we'll okay and we'll put it there you heard it here first. You can hold them to it next year, guys. Thanks a lot indeed.
Speaker 3:We could talk on and on, but I also want to let you guys enjoy the rest of the roots we didn't even talk about ai agents no no no, that's okay, on purpose right okay, well, maybe we can all, of course, on the on the not roots conf editions, let's say you're more than welcome to to come and chat with me and uh, in part. But uh, thanks a lot for for sitting down with me, for talking to gen, talking gen. Ai, I'm excited to see what happens next year and then we, we go from there then cool thanks.
Speaker 4:Thanks for having us thanks, thanks y'all even with a handshake, exactly amazing. Thank you, cheers, thank you, marino Cheers.
Speaker 1:Thanks, you have taste in a way that's meaningful to software people. Hello, I'm Bill Gates. I would recommend TypeScript. Yeah, it writes a lot of code for me and usually it's slightly wrong.
Speaker 4:I'm reminded incident honor to be here Rust Rust. Data topics. Welcome to the data. Welcome to the data topics podcast.