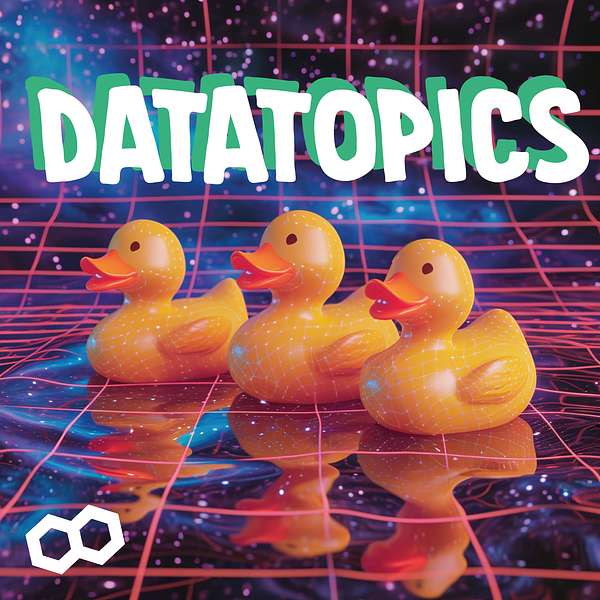
DataTopics Unplugged: All Things Data, AI & Tech
Welcome to the cozy corner of the tech world where ones and zeros mingle with casual chit-chat. Datatopics Unplugged is your go-to spot for relaxed discussions around tech, news, data, and society.
Dive into conversations that should flow as smoothly as your morning coffee (but don't), where industry insights meet laid-back banter. Whether you're a data aficionado or just someone curious about the digital age, pull up a chair, relax, and let's get into the heart of data, unplugged style!
DataTopics Unplugged: All Things Data, AI & Tech
#70 What's Next for AI? A Recap of 2024 and Predictions for 2025
Welcome to the cozy corner of the tech world where ones and zeros mingle with casual chit-chat. Datatopics Unplugged is your go-to spot for relaxed discussions around tech, news, data, and society.
This week, Yannick joins the conversation for a lively year-end retrospective on the state of AI, data, and technology in 2024. Whether you're knee-deep in neural networks or just data-curious, this episode offers plenty to ponder.
Grab your coffee, sit back, and explore:
- AI’s meteoric rise in 2024: How GenAI went from hype to tangible business tools and what’s ahead for 2025.
- Strategic AI adoption: Challenges and best practices for embedding AI into workflows and decision-making processes.
- Real-time data: From dynamic pricing to e-commerce triggers, we explore gaps and future trends in event-driven infrastructure.
- The ethics and compliance puzzle: A dive into the EU AI Act, data privacy, and the evolving landscape of ethical AI usage.
- Developer tools and trends: Productivity boosters like Copilot and the rise of tools like PDM and Ubi in the Python ecosystem.
With reflections on everything from Lakehouse data platforms to open-source debates, this episode is the perfect blend of geeky insights and forward-looking predictions.
Pull up a chair, relax, and let’s dive into the world of data, unplugged style!
You have taste in a way that's meaningful to software people.
Speaker 2:Hello, I'm Bill Gates. I would recommend TypeScript. Yeah, it writes a lot of code for me and usually it's slightly wrong. I'm reminded, incidentally, of Rust here Rust, rust.
Speaker 3:This almost makes me happy that I didn't become a supermodel.
Speaker 2:Cooper and Ness. Well, I'm sorry guys, I don't know what's going on.
Speaker 3:Thank you for the opportunity to speak to you today about large neural networks. It's really an honor to be here Rust Rust Data Topics.
Speaker 1:Welcome to the Data Topics. Welcome to the Data Topics podcast.
Speaker 3:Hello and welcome to Data Topics Unplugged, your casual corner of the web where we discuss what's new in data every week from 2024 to 2025. Anything goes. See what I did there. I just made it up. Now Today is the 10th. Oh no, it's 10 am. It's the December 2nd of 2024. My name is Murillo. I'll be hosting you today. I'm joined by the one and only Bart Hi, and we have a new special, super special, super, duper, duper special guest Yannick.
Speaker 1:Hey, yannick, how are you, hi guys, thanks for having me over yeah, I'm doing great.
Speaker 3:Thanks. Start sweating already, or not yet slightly, but I'll it's okay, I'll try to keep it for myself. He just confided in us that this is the very first time he's on a podcast exactly, so we're taking his yes handle with care yeah, were you waiting your first time time to be with someone, some special people we feel honored?
Speaker 1:yeah, I was like okay no thanks, thanks, guys, for having me over no, happy to have you here.
Speaker 3:Uh, for the people that don't know you yet, would you like to give a quick intro?
Speaker 1:definitely, definitely. Uh, so my name is, as you can see, I joined the data roots a half a year ago. I have a bit of a general background. The majority of my career is bridging business with IT and data, so a bit, let's say, more on the business IT or business technical spectrum. Originally from Antwerp you will not notice by my accent and I think, yeah, definitely happy to be here today and happy to contribute, and I think my role at Data Roots is a bit on the strategic side and a bit on the business side, so a bit on the client-focused spectrum.
Speaker 3:Very cool and, as I can imagine, as part of your role, you have to be in tune with what's happening in the AI and data landscape, right? You also have a bit of a high level view of how the market is shifting and where things are going, and that's why we thought it would be a great idea and also, since we are nearing the end of the year, to have a little bit of a look back and a look forward. Can we play the harp, you know, like a look back? She's thinking so, look back, it's January 2024, right? So months ago. Now, going through the year, we have prepared a few topics here In the AI adoption, for example, and strategic impact. Is there anything that stood out for both of you, anything that, uh? Yeah, if you were to look back on 2024, what would you say? How would you summarize 2024 in the ai adoption and strategic impact domain or area?
Speaker 1:yeah, I think, first of all, what you mentioned, it like the speed of change has been crazy. It's like just trying to catch up following everything. I think there was a lot of content about waking up another monday, like all of a sudden you have new models, new players, new actors yeah, maybe very like indeed it moves so fast.
Speaker 3:One little side fun fact I saw that I think yesterday it was a change pt turned two years old two years only only two years and it's crazy. I mean, yeah, for people that have listened to us like every week we talk about jni and every so it's like it's it's really insane. Huh, it's insane, sorry, but let me talk no, no, but I think it's.
Speaker 1:It's the key takeaway the rapid change of change. And if I bridge it towards our data partners and the companies where we co-create ideas, I think 2024 was definitely about creating a valuable roadmap, about creating what is actually tangible, what is actually creating value and what is sufficiently mature to apply inside a company context. I think that that's one side of the of the key takeaway that 2024 was not about, oh, we need to do something with that eye, but making it more specific and making more tangible, like what are we actually going to do? Yeah, I think the year before, with the genii, everyone was like blown away, like what's happening, and I also think that everybody wanted to do something, but I'm not gonna say that a lot of people were clueless.
Speaker 1:But yeah definitely saw that transition, I think in 2024 yeah and part.
Speaker 3:You have a face like you have something very uh, bring it home no, it's been an interesting era.
Speaker 2:Like uh, like you were saying it's, she said gpd is only for two years old.
Speaker 3:A lot of different uh evolutions um, and now I think, if you look back, maybe a year and a half, chad gpt, I think, was the the main one, and I think this year, like now I would say, we have way more players right, like I think anthropic has a, I think even for coding, for example. What I see is like anthropic is normally better than open ai's models. Um, gemini also is investing, like with the google right, facebook, with the llama models as well. So I think, yeah, I also feel like there's a bit more diversity now as well on true competitors. You know, like some people, I think, yeah, like I said, entropic today is maybe better in some domains than open ai's models, but I don't know if last year I would have said that yeah, I'm not sure if I 100 agree yeah, there are a lot of different players, but we had that also a year ago and I think.
Speaker 2:But it's been a while that I saw the, the estimated numbers. That a bit a while is, uh, two months or something, where OpenAI was by far the biggest in terms of usage like 80% of the market and then a lot of the rest of the 20 goes to Entropic and the rest are very, very small players in terms of actual usage.
Speaker 3:That is known publicly but would you say that Entropic, like December last year, would you? Would you? Would you would you have foreseen this? Because I also, I agree, I also think a lot of people are using open ai and even clients, like, even if someone is on aws and they want to tap into the gen ai, and I guess when we say ai adoption right now, we're talking a lot about gen ai, right? So just to separate the terms a bit um, a lot of people, even if you are on AWS, people still create an account on Azure just to use the Azure OpenAI. So I still see that, indeed, but when you think of the quality of the models and all these things, I do see a lot of noise about Entropix models, for example, something that maybe it's just my perception.
Speaker 2:But they're definitely challenging and I agree with. But that's the thing and I also wonder if it's just what I'm trying to say is that I don't think there is a lot of different serious competitors.
Speaker 3:I think you have open ai and you have entropic, and then you have a lot of small ones yeah, for a market that is still very young, it's actually curious to see that you already have like very dominant players yeah, but I think, indeed, but I think it's I well, my hypothesis is indeed like OpenAI is still dominating, but I think it's more because they were the first one to really make a lot of noise and everyone really discovered Gen AI through OpenAI's models and I feel like there are some.
Speaker 3:Well, we saw some articles on Technoshare and our internal sharing of news and topics and all these things that people are already hypothesizing that they reached another limit on the Gen AI models, right? So if you reach a limit, maybe just a matter of time until the other people really catch up, right. So I'm not sure I'm just hypothesizing here, but compared to last year, I do see, like, in terms of the quality of the models, I do see there, like, in terms of the quality of the models, I do see there is a bigger distribution, not in terms of adoption, I agree.
Speaker 3:This entropic is much closer to, but we're just saying just entropic.
Speaker 2:Well, I think you have Gemini as well. I don't there is, especially in Europe. You hear about mistral, but I have to feeling less and less yeah, that I also. Last year I feel like mistral was more I think the only challengers, if you do if we don't talk about open ai and uh and entropic are probably google and facebook these days, which is llama and gemini right for people and maybe also from a sourcing budget capabilities point of view.
Speaker 1:I think it's going to be hard to let out of the blue if we cut out all the Japanese or Chinese alternatives. I think we're more or less done in terms of options in the market at the moment.
Speaker 2:Yeah, that's true. That's true, indeed, and there are some like this. You have Alibaba that is publishing an open source model. There are some non-Western model alternatives, but I've never really looked into them in depth.
Speaker 1:And maybe looking back to like 2024 as well, what I more or less noticed was like 2023 was like the year that everybody on the business side was like banning all the options. It was like calculating tools in the late 80s. Like everybody said at school you have to work without them. Like 2024 was like starting to embrace it and like 2025 will be like embedding it.
Speaker 2:Yeah, that's good to know. I think that's like a fair evolution.
Speaker 1:Like 2024, everybody's like okay, we cannot keep ignoring it. 2025 is like okay, it's still a standalone option. Maybe it's better that we implement it in our operating model.
Speaker 3:Yeah, I agree. I agree. I think you see a lot of that with new technologies in general. I do think with Gen AI in particular, it was faster because I think there was a lot of attention to it, but I think indeed, this year a I noticed more projects. A lot of the project was still like pocs, right so basically people like people that are interested but are not fully trusting yet. Right, so they're still doing. There's some experimentations and I think now we already see more people that actually want to integrate the business's processes. They understand a bit more the risks to understand more of it. Like you're not gonna I mean some, some people, right, but uh, they're not gonna replace humans, right, but it's gonna be something really next to people that still someone's gonna validate. Like, if an ai model is gonna write your email, you're still gonna read it and check to see if it's what you want to say, etc. Etc.
Speaker 1:Right, so I do would expect that next year we'll see more of the projects that maybe won't be large, large scale, but I do expect that we'll have more the genii things in production like things are actually embedded in the processes, yeah, and then like next phase could be like productionizing it, we one thing, but it would still be internal focused and then maybe 2026, we even see more maturity on external focused models in production. Because, do think, companies and the partnerships we currently have, the projects we're running, even if it goes all the way to production, where we we definitely have a role to play, then we still feel that there's, let's say, a bit of a a bit of a fear of actually getting external focused ai project on the roadmap where there's really a client exposure, where there's really an unsupervised interaction with the customer. I do think there's that's still the next gap we need to close from a maturity point of view agree to me.
Speaker 2:Uh, it comes to genii, like we've seen, genii as a service really taking up, like you have like just an api endpoint that you need to call to get something. Uh, I think that you had that in 2023 as well, but it has become a bit the de facto standard with pros and cons. Like makes that it's very easy to integrate into your product in your workflow. Downside is, like you also need to use it because it's become so big. Like, running these models is not that easy. You can do it, but it requires a lot of custom engineering. But because, like you have now Gen AI as a service, it becomes super easy to integrate into your workflow. That also combined with uh multimodal, which I think now has become the default, but it's still very recently.
Speaker 1:2024 multimodal is also one of the things I noted down. It's like yeah it went so fast that we almost forgot that it didn't initially start exactly.
Speaker 2:Yeah, that's true yeah, and for for the people that, uh, that don't know, like this, this was, I think gpt 4.0 was the first that supported it, and it was. It was released only in may of this year and it basically means, like I have a screenshot, uh, paste it into chat, gpt, uh, make a summary of that screenshot, do something with it, generate another image with it, uh, play it out in audio, like these type of things. Like you really, uh, mix and match with this type of approach, and it makes it very easy to integrate these type of things into your processes. And I think there is also, like, when it comes to adoption.
Speaker 2:Today is still a bit of a challenge. I think what we because there is also a lot of hype on this and what you see is that a lot of companies like they see this as super strategic, super big projects, very risk averse, and then typically, this ends up like let's build a chatbot, but let's be very certain that it doesn't say the wrong things, let's make sure that we have a knowledge base with a rack based architecture that always gives back the right information, and these become very big projects and in the end, hopefully, you have a useful chatbot, but there's so much more you can do, like, like we're, we're integrating it in today in, uh, in processes where you say, okay, I have this text, uh, I have all these. For example, for us internally, there's all these business opportunities.
Speaker 2:Summarize I'm given overview, something very simple yeah, including scoring, including exactly, yeah enforcing it and so forth, and this is like this is like literally built with like a few hours. Right, this is well before that would have been so complex to do. And all these small time automations, where you have like small time automations become simple because, like in lm, is very easy to go from noisy data to something structured and I think that is where you can have like a lot of small automations result in big wins.
Speaker 1:Yeah, that is I think a lot of traditional ocr projects, named entity projects, where, like typically, companies would like have a workforce uh like really manually processing fax confirmations old school banking activity uh, transaction and trade confirmations where that the go-to market of a back old school, traditional AI project would be costly, would be very time consuming and would be very narrow. You could train one template and then, if you would like, to duplicate it for another stakeholders it's very, very big and now you can like more or less. Yeah, I think not entirely erase, but the setup is completely different and also that part of the landscape completely changed. I don't know if everybody's like fully aware of that impact as well.
Speaker 2:Yeah, and I think there is. I think there's a risk in going too big today, because from the moment it's a big project you end up in often a multi-man-year project. Maybe it doesn't take multiple years but multiple man-years, and the reality is that the technology also so quickly evolves, so it's super hard, even if you use the knowledge that is out there today. If you have a project that runs six months, your approach is maybe going to be outdated because a lot of standards are not there yet and I think, especially when it comes to uh to rack, like basically injecting a knowledge base into into your uh, into your lm yeah, what you say there bars is actually quite funny.
Speaker 1:It reminds me of the all the shell and wrapper companies like all the companies, yeah, that that were built on the v1 endpoint of of the models. Also, in 2024 was also the year where half of them just got completely, let's say, killed by the rapid evolution of those providers.
Speaker 3:There was a lot of funding and a lot of startups actually just building a shell on top of those models and I think 2024 was also definitely a year where a lot of them actually got a very brutal wake-up call but I also think that, uh, I don't know if we talked about it throughout the year, but a lot of these, yeah, there are a lot of startups or little apps that were like, indeed, a layer, thin layer on top of these models that you could kind of see that it was a matter of time until opening. I would actually implement these things right, like, if it's a thin layer, they could also make it part of, like I don't know, the creating queries from text. Right, that was actually even on 2Dools and this was before the GenAI boom. Right, there was also some on a startup that we talked to. But, yeah, like things like that that I also, as I saw I was saw like this is really cool.
Speaker 3:But if one person from open, I says, oh, this is really cool, we should include this on our thing, that's kind of it's right, yeah, incoming codex yeah indeed so it's like it's kind of, yeah, you could, you could, in a way, you could foresee the beta, but uh, yeah, but I still think it's in a way it's also important as people kind of go out and try to publish these things so we can also get more ideas and maybe do this and kind of move as a community right. One thing I wanted to also touch you mentioned like multimodal, and I do agree that this was a big yeah, now it's so common, right. Like you kind of expect your models to be able to deal with images and all these things Maybe not video as much. I'm spectrum models to be able to deal with images and all these things. Maybe not video as much. I'm not sure about video capabilities, just less, yeah, less, but I see that, but I still I'm not sure like it is available.
Speaker 3:But I still think that it's a bit more immature in the sense that I don't see a lot of pocs or projects or something that really leverage the multimodality yet so or even just images in general, I think image generation today I still it still feels to me like it's a bit more like for fun generation maybe I agree yeah well, but editing indeed so like actually that's true, adobe adobe has image extension. Yeah, it's like that, I know there was a video from adobe that they were like they could show, like you could select a character and then you can use ai to generate different poses and you can rotate the 2D character to different poses.
Speaker 2:That was super cool.
Speaker 3:Even like the guy was doing a live demo and the crowd goes whoa, you know, it's like you know you're doing a good job. When the crowd like a promo of a product and people are like whoa, you know.
Speaker 2:But also like if you if you extend it like something like canva, which is used a lot in marketing.
Speaker 2:Like you have a lot of genii generated templates and stuff like that sure um, and maybe so to me like, like, multimodal, in combination with as a service, so you have an endpoint, makes it super accessible and actually, because you see a lot of these more convenience tools, pick it up like it's super easy to integrate into Zapier, super easy to integrate into, for example, home automation. I have a good use case, not this weekend, the weekend before. So I have a small home assistance setup which is a smart home software and it integrates with my smart doorbell and like in I think 10 minutes time, I set up an integration that if someone rings my doorbell and like in I think 10 minutes time, I set up an integration that if someone rings my doorbell it will uh via audio. Let me know if it's uh, if it's uh the postman and if, if so, if it's a package, if it's bay post or a personnel, because it's on the van it actually reads from the van.
Speaker 2:So basically what happens is it takes a picture, sends it to open ai with a specific prompt, gets back to result and place it on the, on the stereo, and that's like in 10-15 minutes setup like that is unimaginable two years ago. No, I, I definitely agree and I think that's a very good use case and these are super small automations which do add some value. Right, and it's not going to be life-changing, but I think the the small things that you can do automop, especially in a business context where there are a lot of manual processes, where there is a lot of noise, this can really help.
Speaker 3:But then I think I agree and I think I also agree that it's a good use case.
Speaker 3:I'm wondering if you think of these things because you're in the know, like you know how these things go and you think of critically and you're a very creative person. But I'm also wondering if someone that is like a business person that wants to implement these things, if they're going to think of that, if they're going to know that it's reliable enough, if they're going to you know. So I think it's like I see there's a lot of tinkering. I think maybe next year you'll see more applications and maybe the POCs, because even today, to be honest, I don't see still a lot of like the POC stuff yet, right, but but I do agree that the capabilities are there. But I feel like I guess what I'm trying to say is that I think there is an opportunity to leverage these things more and for us to think a bit more, like the use case you just presented, like what are good use cases for using the multi-modality, and now we're just talking about text. Actually there's also audio generation, right, so there's a bit of all the the components there.
Speaker 2:So, oh, yeah, but I think one of the challenges today and we often um it under hallucination, but I think it's more performance is what keeps businesses from really widespread adoption. Look at our internal process. We sometimes get a validation from customers on hours we performed and based on that, we make an invoice. There's an email and you need to make an invoice. That theoretically would be like even with Zapier, would be super easy to automate, but we're a bit hesitant because one of the hours are not correctly interpreted. Yeah, like you, still have this hesitation, but two things on that One.
Speaker 3:I think, yeah, maybe people and I think we already do, but like people in general need to reframe it a bit, like it's not going to do the job for you but it's gonna. Instead of you having to type everything, you're just gonna read it, right, yeah, and the text, you can assume it's right and if it's not right, okay, it's gonna be. There's no profanity whatever. But, like, you just need to check the numbers. This is the most important thing, so you can still one person can do five, five people's time, whatever, like it's a 5x invoicer. You know um, which is already a very big win.
Speaker 3:The other thing I also think is like, if I see a chatbot today, I would a bit expect that it uses chat gpt. So I think people, people are getting more educated as well on these models, right. So I think people are not like just thinking that it's a everything there is right. So even if you see a chatbot in a service or something, people will question it a bit more. I feel so like, and I think it's the same thing. Yeah, people are using chatgpt. They see the hallucinations.
Speaker 3:Now, if there's a chatbot, they kind of expect some gen ai models and I think the hallucinations. They're not as intolerable in a way, because you can't understand how you see this in other contexts. You know you're a bit more educated on these things. For example, if I go to a website and I search for something like a just searching thing, I would expect the sources there Right, like if I'm really just Google information like perplexity, ai or findcom Right, because I understand that. Like if I'm searching for information that is very important, I want to know that this came from sources and I want to be able to verify the sources if it just gives me something like this, I'm going to be a bit skeptical, right?
Speaker 3:or if I'm not skeptical because I don't care as much about the, the correctness of the information, yeah, right, um, and I would expect that, yeah, people are a bit more tolerant to some of these things because they understand a bit better I think when it comes to chatbots specifically, we've had a big wave of chatbots before, jenny, I yeah which I think nobody was bad experience nobody was really
Speaker 2:happy with it I think the only upside that, jenny, I will give is that you can interpret noisy data, be it to the request of someone that asks a question, and that you can take actions on that, like, like. If you just do interaction, like just reply, you will very quickly get the same fatigue, like, maybe it is going to be a bit more creative in answering, but it's going to come down to the same thing. You're going to ask can I talk to a real person? I think what you can do now with Gen AI, when we talk about chatbots, is that you interpret our. This customer wants to, uh, get a copy of his invoice and automatically have, like this forwarded to an automated agent that gives back this. Like you can, it's more easy to interpret and to automate these type of actions yeah, I agree, I agree, maybe I think that is the big uplift of gen ai in chatbots.
Speaker 2:Not necessarily that the frequently asked questions is going to be better.
Speaker 3:Yes, I agree, but I also.
Speaker 1:I guess my thing is more like, for example, apple intelligence is a good example, right, something that big company, they took their time and now they came to market and we can even talk a bit about the privacy concerns and all these things and the fact that we all buy a new iPhone and still are unable to use the capabilities.
Speaker 3:I didn't buy a new iPhone yet, but indeed, but I think it's like something that made noise and I think they had an interesting approach of if you're going to use, you can ask Siri something. But Siri will say, oh, let me ask ChagPT, right, so they explicitly voice it. And I think if there's a hallucination, yeah, it voice it. And I think if there's a hallucination, yeah, it's chagpt. You can expect that there may be some hallucination there.
Speaker 3:I mean, the models are getting better and better, right, so I think hallucination is less of a problem these days. But I think it goes to a bit to kind of people like when I give you information, you know how reliable it is, you know the risk that you give in there. Because now I'm saying, yeah, it is chagpt. And I think in terms of, like, educating the users of these bots or these assistants, there is also a difference there, right, I feel like the hallucination, like when two years ago, one year and a half ago, when ChagPT was really booming, the hallucination thing was really screaming on your face, like a lot of people were like even getting a bit disillusioned because of this hallucination, things right. But because of this hallucination, things right.
Speaker 1:And I think today, if you, see something is going to be less of a scandal. I think it's tightly linked to everything regarding the hype cycle, like also true traditional vibe. I think like there's a bit of a disillusion, there's a bit of people that say like yeah, is it actually going to bring business value beyond just playing around, beyond the just experimenting? And maybe, referring back to the original question, I'm gonna let you ask, like I think also us being very much into the data topic, very much into the scene data topics oh yeah, I accidentally mentioned that um creates like definitely a bias. If you ask me, like we're on a day-to-day basis trying to follow up, trying to catch up, we name drop casually multimodal LLM rack structure and then you have companies I'm not going to say trapped in business as usual, but having like a multi-year IT roadmap budget planning exercise a year in advance.
Speaker 1:There's a traditional saying, saying soy shit of yesterday that they're they're very busy with all their operations, yeah, and then they have a lot of governance, love structure and so so I get why people in 2024 are looking at valuable use cases because they have to defend roi, they have to defend the business case and they have to battle against it and data projects that are already on the roadmap for multiple years. So I think that's why adoption, that's why we think that it's strange to maybe see the first POCs now, but actually, from a company's perspective, they're growing, they're changing and they're actually adopting. They're just adopting, maybe at a more general pace.
Speaker 3:There's a lag right. Yeah, at a general pace, that's a good point.
Speaker 1:Which is fair and which is positive, but it could make us a bit biased in saying, like I'm not seeing all that much production-ready cases actually currently in the market.
Speaker 3:Yeah.
Speaker 1:If we just, let's say, cut out the major players out there, or like the really tech-first companies, say, cut out the the major players out there, or like the really tech first companies, if we look more like, let's say, at the incumbents in the market. I do think I'm referring back to your question that that the lag is quite obvious and I also, let's say, a week ago, read some metrics about the amount of people that, like, for example, in belgium and in europe, actually used uh gen ei on a day-to-day basis, on a weekly basis, that the uplift is still really, really big. Yeah, so we're. We're just let's say we are ahead because we're in it on a day-to-day basis but, like general companies in their operating model, on a day-to-day basis, we're still far from maximal usage and impact no, and I I think you make a very good point.
Speaker 3:I think, when you look from the other perspective, it makes a lot more sense. Maybe a question is there a way to, to? Is there a better way? Well, I'm just not sure there is a better way, or it if it is better. But suppose that we want to shorten the lag. Is there something that businesses could do in terms of strategy, in terms of something like this yes, this is exactly what we do.
Speaker 1:So, actually we do so. I think we also 23 and 24. Let's definitely the beginning of 2023 and 24 was, I think. If you could summarize it in in one word, it would be FOMO. Like everybody was like, oh. The boardroom was like, oh no, we have no, I ai on the roadmap.
Speaker 1:It's like yeah, and so it was, let's say it was. It's a bit. The purpose was maybe to put ai on the rope up and not to create value, so it was more from a formal perspective. Yeah, and I do also think that 2024 I think that's how we opened today's podcast is like the pivot into tangible use cases with tangible value and a proven outcome. Yeah, and that's where we, as data resources, play a like, a very active role and we have the experience. Data roots was in ai before the hype was in ai before gen ai.
Speaker 1:Yeah, uh, and that gives us, like this, this, this, indeed this leapfrogging effect that we can we can have, when we, when we partner up with yeah, and actually there we see that, speaking about tangible use cases, then we go beyond, we go beyond the FOMO and it. Just if it mentions AI, it's good. Yeah, I think 2024 was a good year and like that's not gonna make it work.
Speaker 3:Yeah, no, I agree, I think. Maybe just to reemphasize a point for the people that are listening, they may be not as in the know as we are, but uh, yeah, indeed, there's ai and there's gen ai.
Speaker 3:we are using a bit of terms interchangeably here, but if you think of ai like neural networks is like the 1900s. There was already some stuff image recognition, machine learning, data science, machine learning all these things are still happening. I think what's the really on the spotlight these days is the gen ai, which is what we're talking about here. So just to for people listening like we're talking mainly about gen ai here, if not exclusively.
Speaker 1:I'm not um but it's good thing we could bridge to ai, because then we reach to ml, ops and, like, that's true, still a lot of companies prototyping in ai. But also there the challenges to put it in production exactly, monitor the drift of the model, model monitor, like, how do we cope with data?
Speaker 3:so also that's, I think, something that in 2024 also really kept maturing indeed, I agree, I think, indeed, like, uh, I think for ml ops is a bit of a more mature topic. I think in terms of like, yeah, basically ml ops for people that are not as familiar with the term, the way I see very simplistically is like, once you're putting these things in production, what are the other things that go around the models? Right, like you said, monitoring is the data drifting? Do we need to retrain the models? Even just deploying models? How to make it simple and easy and scalable, et cetera, et cetera. And now thinking again of 2025, if Gen AI, we expect there to be more production use cases, then there's also going to be maybe more the gen ai ops, right, so, or the llm ops, right, the stuff that goes around these models that are not necessarily the prompting, they're not necessarily experimenting these things.
Speaker 3:It is a bit of a different beast because you're not training these models, but all these things like how, how do you track your um, how many tokens you're using? How do you track how many tokens you're using? How do you track how many? Because you can put like guardrails right To make sure that the models are not using profanity, for example, simple example how many times does this hit right? How many times are you getting caught by the guardrails? So, like all these things, how do you monitor these models? When it's time to upgrade, when it's time to do this if you want to change a prompt and you're classifying like the named entity recognition right, is this better, is it worse, etc. Etc. So all these things is maybe something that we can also expect more in the next year yeah, you can go on it's.
Speaker 1:It's also about context, it's about reinforcing uh, general models and like the like, the big models, reinforcing them for your local context. Uh, I think, yeah, everything, gen ai, ops. I do think it's it's a good terminology yeah, just put like ops, sounds cool no, but it's true. It's actually if you want to go from labo, slash, poc prototyping to industrializing, actually that being part of your operating model. That also means that business will require uptime, that they expect it to maybe less hallucinate or be more predictable, that yeah indeed, and that you need to document it and that you need to like who's the owner, who's a we're really it.
Speaker 1:Then you come back in like more it driven transformations. Yeah, if you really want to make it company first, true.
Speaker 2:I think when it comes to LLM, ops and still LLM is still such a young field I think we will see advances in components. I don't think we see a consolidation yet.
Speaker 3:No, that I agree.
Speaker 2:LLM Ops is still very broad. It's prompt optimizations, testing, it's automated deployment, it's monitoring, it's active guardrails and we will see, I think, a lot of improvements in each of these specific fields. But I don't think the ML flow of LLMs will be there next year, that I agree, or DVC, that I agree. But I do think that but I agree with that there will be a lot of advances. Advancement in that because it's it's an answer to this is something risky because it's a bit probabilistic.
Speaker 3:We're not 100 sure and good lmo practices give a partial answer to that indeed, I think maybe, like best practices, they may not be there because indeed it's early and I feel, like the frameworks and technologies, there's not a technologies, there's not a clear winner, there's not a clear standard. I think, even in terms of the like you mentioned, apis for the models, I'm not sure how if all the models kind of follow the same API or like, for example, tool calling, if they all have there. So it is a bit immature in the sense. But I think the questions that they're going to be tackled and I think, because there is an attention to that, I would imagine that the frameworks they're going to become more mature on that space fairly quickly.
Speaker 2:And I think the nice thing with that is if you have a good LMOP setup, then it also becomes relatively easy to just switch it out for another model as a service and see what the performance is Indeed.
Speaker 3:But I think and I, and that's what I was thinking as well you kind of need a common standard between these models, right, like if you are using open ai today, open now you have model validation, which maybe you don't need for, like, yeah, you can do it yourself in a way, but like tool calling all these things, you kind of need to have a some, some standards, right, and I think not all the models are there yet, um, but so we're tackling a bit like, yeah, what are the challenges, right, we're talking about next year? What are some other challenges that you can also see, that you can expect? Well, challenge that maybe you had. But, more importantly, what are the challenges that you can foresee for next year? We mentioned llm ops. Is there something else that you can think of? And I'm hinting a bit when it comes to ai adoption.
Speaker 2:So I think what we saw uh, rack retrieval, augmented generation, um which basically means when I ask something to my llm, it's going to try to fetch relevant data, relevant information from my knowledge base and give an answer based on that. I think today that is still very, very ad hoc. Like you try to build something that is suited for the LLM, that is suited for the legacy system that you might have, the unstructured data that you might have, and I think within now and definitely five years, and I think already next year, we will see RAG as a service.
Speaker 3:And I actually have a maybe. I don't know how hot the steak is, but I actually think that RAC systems are going to be replaced by agentic workflows kind of thing, and maybe I don't think he will go away.
Speaker 1:But before I'll just explain what I mean for people that are not as familiar with the terms, that could be the elephant in the room next year.
Speaker 2:But let's maybe just before we go into agentic, because another, it's another evolution that we're seeing, yes, so I think what we, what we've seen this year, is that the big providers already have this to some extent. If reg as a service, um, azure has it open, ai has it uh, I don't know if entropic has it. To be honest, um, if you use their platform directly, uh, so you already see this, but when it's more than a frequently asked questions use case, you quickly like run into its limitations, um, at the same time, we're seeing, uh, the big open source database like postgres, having extensions where you can do like really embedding a factor search fully in database, like it's really becoming like there's a lot of attention on this, so I think the performance of this rack as a service will really go up in the next year yeah, maybe if uh, but like when you say, like vector search, that's a component of rag yeah, but even like, like, so how that needs to require us to delve into a little bit what it is yeah
Speaker 3:but, uh, so maybe can you explain, maybe what like, what are the like?
Speaker 2:very eli five so let's say that you have uh and uh. Give me an example of a knowledge base ice cream shop.
Speaker 2:Yeah, you're working at an ice cream shop and there are instructions, there's a big manual on how to make different uh type of scoop sizes and flavors and how you can combine them. Let's say that this is a 500-page document. It's really an artisanal shop. It takes a lot of time to get people to read these 500 pages right. In other words, it takes a lot of time to train people when they're new. What you could do is that you take this knowledge base, these 500 pages. You then embed, embed them into vectors. You split this up into relevant things.
Speaker 2:It's already the difficult thing, like how you need to chunk these 500 pages into something smaller. It's already a big question like how and today a lot of manual work. If you find, how how big do these chunks need to be? These chunks, you then turn into vectors uh, into a vector supporting database, and that allows you to very easily search on this. And the searching is these chunks. You then turn into vectors into a vector-supporting database and that allows you to very easily search on this, and the searching is something else. The searching is, let's say, I have a chatbot within my ice cream shop and whenever something new comes, you say use this chatbot when you don't know something. And so this person let's call this person Murillo doesn't know how to make a vanilla ice cream with a medium-sized scoop, so he types it in how do I make a vanilla ice cream with a medium-sized scoop?
Speaker 2:What then happens is that this question, in other words a query from Murillo, is then turned also into a vector. So it's called an embedding, so you need an embedding model there. So you have a vector, so it's called an embedding, so you need an embedding model there. Um, you, so you have a vector, and then the database basically compares like marilo's question what chunk is it closest to? And then it takes that chunk, turns, it takes the text of that chunk.
Speaker 2:Hopefully that is the description of how do you make a vanilla ice cream. Uh, injects it into your lm and basically uses a prompt like uh, we have an employee asking instructions about making ice creams. This is the relevant knowledge that you need to use for this answer, and this is his question. Muriel's question how do I make vanilla ice cream with a medium-sized scoop? And that full prompt then gets run through an LLM. Hopefully you get the answer on how to make a vanilla ice cream with a medium-sized scoop. So that's a big process and on each of those points today there is a lot of optimization, manual optimization to do and I think that full solution we will get out of the box as a service in the coming years and that full solution is RAG. That full solution is RAG.
Speaker 3:And that full solution you can already, if you test a bit of boundaries, do, for example, in postgres today yeah, indeed indeed, and the vector search is a part of that, and the generation part is part of that indeed.
Speaker 1:I think the contextualization is like so powerful yeah yeah, that's like the big difference also in maturity of the output, immaturity of the the use cases where you can potentially in the future embed it in but there are a lot of like.
Speaker 2:The challenge today is like we were taking a very simple example like but if you want to do this for someone that does customer support, and then you say, okay, all the knowledge that we have on customer support injected into a database and just automate it, it's not going to work, because probably there is this document on how to answer this question from two years ago and there's the same document from a year ago and there's not really consistent and you're sometimes returning like there's a lot of things that happen.
Speaker 3:I'm also wondering if there's a like for users, if you want to have a history of the user and you actually have personalized and you cannot have like all the users together, right, because I'm going to say, oh bart, how much one, why, why can't I make this payment now? And it's like, oh yannick, actually two days ago. You know, it's like doesn't, doesn't make any. There's also privacy, boundaries and all these things that we can hard code right.
Speaker 1:Imagine indeed that you can add the specific, constant context of that client, yeah, and the the context of the situation. So the domain context of the question boom.
Speaker 3:Yeah, indeed, it gets very powerful and maybe, uh, and it's okay for, like, do you want to talk a bit more about this?
Speaker 3:or let's go on so therefore, yeah, for the agentic things. Um, to be honest, like I, I did try to like what is this agentic model beyond the hype? Yeah, yeah. So it's like and I heard that, basically, if you're into working with LLMs or building these things, you probably heard agentic agents, agentic workflows, whatever. From what I gathered from research and all these things, and also what I see and what I try to do, people still use the term very loosely. It's the new hype, right?
Speaker 2:this is going to be the hype of 2025.
Speaker 3:Yeah, when I'm saying agentic workflows or agent or agents or whatever, I'm thinking of really models that have the tool calling capability, okay, and then you can, as you interact with the tool. So, for example, to call capability, you can really think of it as a python function that the model can actually call right, so it can be Google search, right, it can be a calculator. If I say, can you convert? Or maybe a good example is like can you convert? I'm from Brazil, so we have reais and actually the dollar is really expensive right now, so probably euros as well, and I want to say, ah, can you convert this to this? Right, the model can actually understand the question. And maybe I have a hero to real converter and you can get the latest information there, right, so it's not something that the model would know by itself. It can actually have a very deterministic block to it and then from that it can give my answer right, so that's a bit of tool calling thing, and usually when you're actually working with these models, there is a step that basically the model calls the output. It gets back the. It calls the sorry the function. It gets the output. It gets back the. It calls the sorry the function, it gets the output and then it continues processing these things. So that's kind of what I'm thinking of agentic. It's in a way you have a model that calls something external, it gets the response from the external thing and then it keeps going and then you can choose to make another call or not.
Speaker 3:In the context of RAG, one thing that I've come across is that a tool you can have is to actually do the vector search. So, for example, if I say what is the age of the two DataRoot founders combined, maybe the first query will be who are the DataRoot founders? And then you come up with part and the others, and maybe the second question would be what are their ages? Right, so? But then you kind of have a bit of a. You don't need to do everything in one go. Right, the model can actually have this step-by-step thing because, first, because if you just throw it out there, maybe the chunks that you will get will not be enough, because you also need to see how many chunks you need to pass in the model and all these things, right? Or the example that I saw was what's the temperature? No, what was it? Is it hotter? Or maybe I'm adapting the query before who's older, bart or Jonas, right, they maybe need to get the age of Bart, the age of Jonas, and then you compare the two and say, okay, this and this, maybe you can do it in parallel, maybe you have to do sequentially, right, but the agents, they should be able to kind of figure these things out.
Speaker 3:I think, like, in a way, it's more abstracted than RAG, right, it's a bit more fluid, right. The vector search is just a function that the model can call and you can still have this dependent information and following queries that you can have. And that's why I think that maybe the next year the agentic search will be more common or more used, or there will be benefits to using that compared to just Rack, even though I agree that Rack is simpler and there are some already off the shelf kind of things, so it's easy to plug and play. But I do expect that as we go along more, these Rack systems will be replaced by more of these agent search models. I think they're really something different.
Speaker 2:Yeah, I also want to add to that but Bart, go ahead.
Speaker 1:I think they're really something different. I also want to add to that but, bart, go ahead.
Speaker 2:I think Rack is in your definition of an agent.
Speaker 3:Rack is just a tool where you can say you have, knowledge to the vector search is a tool, but that's what I mean like that's why I try to make the distinction before. The vector search is a tool, rack is the whole system. So if you take that component and you put it as a tool for the LLM.
Speaker 2:I don't really agree there. So I think the fact of the matter is it can be a tool, yes, but an API that you can query, in other words, your rack system can be a tool as well. True, like I think.
Speaker 3:It's true, it's true.
Speaker 2:And in that sense it will maybe improve the outcome.
Speaker 2:And that's a bit like the example that you're giving is like build a search strategy to come to the age of these two people or to come to the maximum age, whatever the exact question was, but like, and you have access to these and these tools to do this, do this within maximum 10 steps and build out a path towards that and to also make sure that it's verified. And then maybe you're going to use that rack steps and build a, build out a pot towards that and to also make sure that's verified. And then maybe you're going to use that rack or maybe you're going to use a payroll api or like right, like you're going to give a bit more freedom to the, to the quote-unquote agent, to do that. But to me it's a bit like the, a little bit the evolution that we're also seeing with something for the people that use chattie pt daily. That's what we're seeing with um, what's the name again of the? The thing that keeps uh, that goes a bit further than just giving an immediate answer that quote unquote things.
Speaker 3:Ah the reflect. No, it's the reflection of the o1 model or whatever the o1 model. Thank you, yeah, where no one? You also have this a bit like you.
Speaker 2:You get a first answer and it's the reflection with the o1 model or whatever the o1 model. Thank you, yeah, where no one? You also have this a bit like you you get a first answer and it's right kind of a reasoning it's.
Speaker 1:Yeah, well, quote unquote reasoning yeah, and it basically asks a bit like uh, like uh is this the correct model?
Speaker 2:can other other parts that you can explore and it tries to, yeah, in its own, go to go further right?
Speaker 3:and I think if, when you add this type of reasoning to a large tool chain, like if you have a multiple chains like, it becomes very, hopefully, very powerful yeah, indeed, because maybe also to add a bit on that like the, the reasoning is also like there are empirical studies that show that when the model reasons on the previous output, it actually can detect hallucination and all these things better, right?
Speaker 3:So there's some research on that as well, and that's why these reasoning steps quote unquote reasoning usually leads to better quality output, right, which is also something, by the way, that I think if you're doing agentic search, it could be better, right? Because if I ask, I mean again, and I agree that a rag can be a component instead of just a vector search. Because if you ask, just, I mean again, and I agree that a reg can be a component instead of just a vector search. But if you have an agent, that kind of like, you have a query and then the chunks are not the correct chunks, right? Maybe you ask for vanilla ice cream and for some reason, the chunk is about strawberry ice cream. Maybe the model would be able to say, okay, this is not correct, maybe I need to do something else okay, this is not correct, maybe I need to do something else, right?
Speaker 3:so you can kind of exactly, but yeah, sorry, and you were going to say something.
Speaker 1:So, yeah, yes, um, maybe the agents hot topic indeed, there's a lot of fuss and definitely 2025 will hopefully define a bit the boundaries, because now agents, everybody has his own definition. I have the feeling if you'd ask me but not necessarily under the umbrella of agents, but how the future would look, then I would go beyond query and retrieval to actually emphasize more on the tooling capability, as you mentioned. So actually book it for me, execute it for me, execute that update statement, do something where you really have like a wingman, but like an active co-pilot, not a co-pilot to query as your external hard disk or additional, let's say, computing power, but actually also somebody that can go the next mile and actually update your calendar. Yeah, send a request to a hotel or something. So the tooling box, yeah, on top of that agent capability. For me it's like a dream come true, because then you literally have that full force virtual assistant.
Speaker 3:Yeah, that's for me the argentico, the agent definition, but of course it's but looking ahead and forward yeah, I think that may not be in 2025 yet, because, also, like, if you have something that you make a payment and then the model makes a mistake oh yeah, I bought a car, yeah, I bought a car for you, like, you know, it's like whoa, but I think and then it brings to another like bit tangential topic to this that I also, I read about it and I hear about it and I see courses about it I don't see as much applications it's with like the human in the loop kind of thing. Right, maybe you just ask ChatG, like, so basically you need to encode it in your model the a moment to say, if this happens, stop and ask the user. So basically it's not running by itself, but it still speeds it up, right, like instead of saying, ah, book a meeting with bart, and then just say, okay, check, check, check, check. Well, is this, is this a good time?
Speaker 1:yeah, and it just summarizes.
Speaker 3:And the final goal yeah, and I think that's also where the gen ai today I I see people thinking a bit more of Gen AI models as like a productivity booster instead of a human replacement right, which I also think. In this context, having something like a human in the loop that stops and says, is this okay? Is also indeed of a productivity prover instead of really replacing At least my guess 2025, right, and maybe the next year we can sit down and see if this is the case or if next 2026, we're going to go further and these things are reliable enough, but that's a bit my guess and again, the human in the loop may be another topic that comes up once we're looking into these things, but in terms of use cases, capabilities, value creation, that would already be so, so powerful even with the human in the loop.
Speaker 3:No, I agree. So, so powerful, even with the human in the loop. No, I agree, and I think even like. And then if you go back last year not even 2024, in the beginning of the Gen AI for coding and all these things, I remember a lot of people saying like, yeah, these Gen AI models, they're 80% correct. They're never correct, but they're 80% correct. And I changed 20% and that's still a big productivity game and I think that's still a bit the same thing that carries through. Maybe they're never going to be 100% correct, but that doesn't matter, because if they're 80% correct, it's already a win.
Speaker 2:I agree. I agree with you, I think, when it comes to GenDeck something that I hear a lot about as well, but I also think there's a lot of hype too much hype is done on uh, reactive uh versus proactive can you elaborate on that?
Speaker 2:so where we say, today, when we use uh and I'm not, I don't, by the way, I don't really agree with the definition but today when we use gen ai, it's often uh reactive, in a sense that an email comes in, do something about it, do something with it. Like I have a manual trigger right and I think what is is hyped up a lot about is that this will be more autonomous, that this is something that keeps running and does something uh proactively, um, but let's be honest, like every, every process that runs needs triggers to do something. So to me it's a very, very vague definition.
Speaker 1:You're referring to behavioral changes, then maybe Eventually in the future Would that be like a potential implicit trigger?
Speaker 2:No, I think like something proactive, I think what people don't really agree with the definition again but something, let's say, I make a gen ai uh driven bot that continuously monitors all social channels on to see if there's any negative remarks about my brand or about my company, and you give it the tools uh, instagram, uh x, uh, blue sky, uh, whatever and it automatically keeps, keeps, uh, scraping this view and you give it some quote-unquote intelligence to do that right and which is in the other in order was just smart prompting, yeah, and also tools to flag something, to, to to take an action if they and that's, I think, today is being positioned more as a as an autonomous agent, yeah, but it's very vague, like what?
Speaker 2:what is the definition between something, something autonomous and non-autonomous? Right, because there is no. Let no, let's be honest, there is no general AI.
Speaker 3:Yeah, yeah, yeah. And I think when you say proactive, reactive is very anthropomorphized, is that correct, alex?
Speaker 2:Yeah, yeah, exactly.
Speaker 3:But I think when you think of software, you don't have reactive and proactive, you usually have event-driven or schedule-based.
Speaker 2:But I think for people that are further off, removed from more software engineering, yeah, to them this feels very autonomous, right indeed, and I also think there's a lot of hype about agents, because it's really again, it's anthropomized, right like anthropomorphize is that right, alex, is that right?
Speaker 3:okay, she doesn't know. Anyways, let's, we're to roll with it. But when you think of agents, you almost feel like an FBI agent, like someone that has sunglasses, the earpiece and is just doing stuff for you, and I think that's also why it gets a lot of the hype. But that's also why people use it very loosely, true, because it's just like oh yeah, it's like a person that has a mission and they're going to do it. Yeah, you know, and it's yeah. So I do agree. I think we also need better definitions for these things, and that's why, even when I was talking about agentic workflows, I tried to make you very clear what I mean here. Right, but yeah, I don't know if this well, maybe it will come. This is proactive.
Speaker 3:Yeah, like the idea of people dreaming, you know, yeah, yeah like the idea of people dreaming you know, yeah, yeah, but it's true. But I feel like, if it does, I think it would be more well, I think it would be more. I don't know if it'll be next year. I don't think it's something for 2025, to say the least.
Speaker 1:Yeah, some of the things that people are envisioning could actually be, indeed part, can actually be indeed.
Speaker 3:Part of it will be code, part of it will be models, part of it will be still a dream, if you ask me yeah exactly but it's good to keep the hype alive definitely well, I think I mean I'm not saying like, maybe to be very clear as well hype is not all bad, I think, just to get people talking about it, just to get people thinking about it, just to give ideas and like ask questions.
Speaker 2:I think it also moves, it gives you also uh moves investments yeah it creates, uh, for better or for worse, formal indeed, um, but I think that's that's always the question. Like, from the moment that you use it, like I think there is good arguments to say, like, make sure that you really understand it in order to also estimate, like, what is the values will bring.
Speaker 2:Otherwise, it's very, very easy to do things that will cost a lot and not result in anything but that's I think that's also true in general, right it's true in general, but I think for ai specifically, because there's so much hype for every subtopic in in gen ai, there's hype and a new thing is agentic, yeah, um well, at the same time, it's moving very quickly. Like you have this combination that makes that it's hard yeah, but that, but they.
Speaker 3:the reason why I also say it's generic is because I also see that in just machine learning, in ai we used to see that a lot and I think the difference between ai is that in a way it's easy to get from zero to one. It's faster because the models are there. But like I've seen projects that were two years in the making for machine learning and after three months people were asking for some feedback and then they kind of inflated a bit the results to make it look better and then like it kind of snowballed, and two years later it's like and then I've even seen something that in the process of these two years, uh, the legislation changed which made the, the use case a bit irrelevant, and that's. And I'm thinking I'm saying a lot with them, but my mlops hat on. Because then the, the counter proposal is like okay, instead of making this big thing for two years and deploying, deploy after three months, and then you, but then you need to make it easier to deploy another one.
Speaker 3:But I and I mean I fully agree with you, but I wonder if for Gen AI, because the models are trained, because it feels like it's easier to get from zero to one or to scope it down, I think in not every use case. If it's, I don't want to see less of a problem, but it's not as severe as for the traditional ai or traditional machine learning stuff I think the big difference between genii and traditional ai is that is this the speed of development?
Speaker 2:yeah, that's true, like the pre-trained context yeah, like, if you look at back, uh, eight years all kegel competitions were won with XGBoost, true, and every month there was new things, but six years later there was still XGBoost that won everything on Kaggle.
Speaker 3:Yeah.
Speaker 2:And that we don't really see Like the stability, like what is the best thing, and is it still going to be the best thing next year?
Speaker 3:Like it's really hard to predict in the end I also think that even the tooling right, the frameworks, the standards, it also is evolving quickly, right. So no, but that that I that I agree. Maybe, uh, I think we talked about a lot of stuff. We talked a bit about the guardrails, that llm ops and all these things. Maybe on the data privacy also, and maybe we can. Well, maybe let's talk about the data privacy first and then I can segue to the next topic we haven't talked about data privacy yet True, I think if I look back at 2024, we have the EU Act.
Speaker 1:We have a lot of evolution on this framework about models. So I think a lot of research was done on the side of data rules as well to, let's say, not only comply but also see what's coming in our direction. And I do think, yeah, being both on the research as on the development side of AI things, I think, yeah, it was important to stay on top of things. So we created a few, let's say, easy assessments to see the impact of changes and pre-assess a future project as well. So I think that's very valuable, both from a toolkit side as from a knowledge partner position, and I do think it opens a lot of, let's say, opportunities for companies to better understand the impact of their project and to better assess the go-to-market strategy definitely yeah, I do see, indeed, that the, the legislation, I feel like legislation is a bit of a quote-unquote slow process and I think when you contrast that with gen ai, the difference is even bigger because gen I is moving so fast.
Speaker 3:Um, but I do see, indeed, like the EUAI Act has Maybe it's a feeling that I have, but this year I felt like it became more concrete the things that I need to be worried about or the things that I should flag, or the things that I should raise I see you nodding, yannick, yeah, but I agree, I think it came into force this year, um, which also, like, is a big evolution because before there was not, let's say, a very um mandatory framework.
Speaker 1:Although it's, yeah, let's say, there are different opinions and there are people definitely out there that say that it could be a potential blocking for innovation. There are people out there that clearly emphasize on the must, like we are in need of a framework because this could potentially tread some of our basic values and I think, all in all, I think it's a challenge for companies definitely smaller companies, I think to stay on top of that regulation and so forth smaller companies, I think to stay on top of that regulation and so forth. So I think that's also where we could play a role as as being a trust and advisor and helping companies to to scope what is, let's say, in line with with the projected boundaries yeah, definitely think, and I think this also falls in the umbrella of like, even like, testing all these things like what are the things that we can move fast?
Speaker 3:what are the things that we need to slow down? Uh, what are the things that, yeah, it's okay, maybe to take quote unquote shortcuts, right, like, what is the things that we can move fast? What are the things that we need to slow down? What are the things that, yeah, it's okay maybe to take quote unquote shortcuts, right, like, what is the impact of this? It's something that we also focused on, maybe also. So now, segueing to the, you already mentioned the EUAI Act, but also ethics and compliance and, I think, gen AI. Now, again, going back to the Gen AI stuff, ethics has always been a bit, in my eyes, more prevalent there, because you're generating stuff and like the race to stay, ai models and all these things, right, or?
Speaker 1:there were a few podcasts about author rights, you know True, uh, author rights, you know true. Strikes in hollywood, uh. Some music artists, uh, that got a bit offended. Some newspapers that were sick and tired of all the crawling of their, their neighbors.
Speaker 3:A lot of monetization as well, I think there were lots of trials as well right for these cases right, which, uh, I don't know if there was any resolution on those, do you know? But I do think that they also, yeah, they will set a precedent as well for future. For future, things like scholar johansson's use of for the for the thing, right. So I think there was also a lot of yeah, like the ethics and all like, I mean even the bias and stuff, right, you mentioned, like your, is it a postman or something? Right? And maybe if the guy's brazilian, would you just immediately assume, you know, like there's also a bit it's always a bit of um, I I have, I I'm not.
Speaker 3:I do think that we need to do better in terms of, uh, the models and the safeguards and all these things, but I also think it's very difficult because, in a way, the models are always like, it's always bias right, like the predictions you're making away, it's bias right. But the thing is like we have to basically choose. What are the things that we say it's okay? One of the things we say it's not okay, right, if you look at that, was years ago, like I think it was. What was it For managers? They would have an AI screening CVs before AI, yeah, and I think they were passing more males for manager roles, right, and if there's a clear explanation because historically there's more. But is this something that we want? Do we want that bias to be in the model? Right. So in a way, it's like you have to kind of slice and dice what is okay, because in the end models they just kind of track correlation, not causality, right, and we need to basically slice and dice what are the things that we actually is okay for the model to have that bias and what is not? So I also think it's.
Speaker 3:And again, gen AI, the hype, the fact that we are generating stuff, makes it like, if you ask, give me a picture of a Brazilian, maybe there'll be a lot of things that for you it's okay, maybe for me I'm going to think it's racist or not right, like and what? Even have you ever watched the show Community? It's like the community college. Yeah, they have like. Basically it's like it's the same creators of Rick and Morty. I think it's funny.
Speaker 3:But the guy they have a mascot and then they try to make it the least offensive mascot and the name of the mascot for the community college is human beings and it's just like a weird like. It's like a guy in a white suit with like a, like a blurred mask or something. You know, because they're just trying to do the most general thing. You know, because they're trying to be politically correct. And I'm only like, if you say, give me a, an image of a brazilian, where do we draw that line as well? Yeah, because there need to be some traits that say, oh yeah, that guy is brazilian. But at the same time, where do we draw the line to say, okay, this is not okay and this is okay?
Speaker 1:I think companies do have like an obligation to do a lot of de-risking. I think that's clear. So de-risking means framework, iterate and definitely learn. I think it's a very learning, very steep learning process. The most important thing there is that everything's evolving so fast. So there are definitely improvements on that. A lot of uh, let's say, committees, initiatives and reviewing and evaluation uh, initiative as well on on that line. So the only challenge, I think at the same time is that it's moving so quickly and there's always this balance between trying something out and the fear of is it already mature enough for us to try and play with it? So I think that that balance, definitely for companies is is a bit of the the pivot, but I do think everything's heading in the right direction.
Speaker 1:Um, and then, yeah, you can debate on it. Uh, and I think companies are at the moment, very aware of the, the potential reputational impact of trying models using client data and so forth. I'm not saying we're there yet, but the awareness of companies has never been that prominent or on the radar than it's ever been before. I think we had GDPR in 2018, new law on privacy in Belgium moving from there. Moving from there, I think, compared to awareness about data encryption, about privacy, has never been that much on the radar than it has ever been before. I'm not saying that we're there yet, but also, on bias of models and so forth, awareness has never been this big, if you ask me no, but that I know.
Speaker 1:I agree that doesn't mean we're there.
Speaker 2:So yeah, but I, I agree yeah, I fully agree with what you're saying and I think the the concern that you're raising, mailer, is also like. If we look at 2024, then um, like, uh, biased models. I think a lot of this is is to some extent covered in the ai act.
Speaker 2:Like, if you use this like the the risk classification, um, but there are also other, especially in the finance sector, a lot of other regulations and compliance guidelines that that's basically unfortunate. To not be biased on certain decisions that you, I think, need to implement at a local level to make sure that you have the right guardrails in place. I think the the, the only reason why we're discussing like, for example, a racist model, is because there are so few players in this space, in this space true, because if there were a lot of players and you just use the, the player, that is right for your use case right and I think but that is that is definitely a a challenge that there are only today, like I think, if it's 10 is a lot to that have the resources available to build a model from scratch.
Speaker 2:I think that is that is definitely a challenge and that that is why you need, we need these guidelines yeah and I think, when it comes to and I fully agree with with janika, say, with gdpr, we have the, the ai act in europe, we also have the dma since march or April or something this year the Digital Market Act, which also puts a lot of focus on data privacy or handling data correctly, and I think for the end consumer, that is very well, very good. You do get I think you touched upon it a little bit like what is the effect on, on, uh, innovation? Um, I don't think. I don't think it improves some innovation, but I also think maybe that's maybe more of a hot topic that, uh, the chip has sailed for Europe Like we don't have any big players whatsoever.
Speaker 2:You can maybe say mistral, but there are no big cloud providers in europe.
Speaker 2:There's, there's a, there's a lot of other things that make it difficult to build something like that in europe. Yeah, and again I agree, like it's good for the end consumer short term, but like something like take, for example, open ai. What they did from the beginning is just say a big fuck you to everybody and just took everybody's data. Yeah, it would never have flown in Europe, like it would not have been possible to do that. And there is the argument like because of that, you can innovate. Um, whether that's like, it's very much like it has two sides, right.
Speaker 3:Yeah, yeah, yeah, yeah. No, that's true, maybe it's one funny like not funny, funny at all, but tangential to this One thing that I forgot the article that I was reading. But they also referred how most of the AI, gen, ai systems, they're women voices, and for me it was like oh, that's true, right, you don't hear a lot of like chat, gpt and all these things. Most of the time it's women, yeah, like. And for me it was a bit of a light bulb moment, right, like how it kind of plays a bit like yeah, like the helper and all these things, how women are portrayed and all these things. So just a quick side note, you also mentioned guidelines as well, and I think one other thing that happened was the guidelines for what is open source AI, right, that we covered like second half of the year, for sure.
Speaker 2:Which I also thought A definition of what is open AI.
Speaker 3:Exactly, open source AI, because open AI yeah, you're sorry. So yeah, because indeed there's a lot of models that they were like they call themselves open source, but like now, like, yeah, usually have open source software. But like they call themselves open source but like now, like, yeah, usually have open source software, but like, what is open source AI? Because do you have access to the data? Do you not have access to the data? Do you have access to the weights? Can you use it for anything? Can you not use it for anything? Meta has like an in-between, like they let you use for most use cases, but not all of them, and before they. So we saw more consolidation, a standard in the I don't know if it's legal, but like a standard on the, on the artifacts, right, like a more cold definitions of what does it mean to? And this is from the open source initiative, right? So it's a very, uh, yeah, known organ for this um now.
Speaker 1:All of that is just to prove that the market is still very maturing like exactly, and there's a lot of attention to it, right?
Speaker 3:so, again, if you think that chad gpt is two years old, so this whole thing started two years ago, to see that we are here, it also shows that we're moving very fast, in my opinion. Um, and segue into the next uh topic. Uh, the innovations in data tools and infrastructure. Right, talking about open source, you don't see something?
Speaker 2:maybe just one step back when we talk about compliance, but we could, because we were mostly discussing 2024, right yeah, um 2025.
Speaker 2:so we 2024 brought, especially with the ai act, a bit of a view on roughly what are you supposed to do when it comes to transparency, these type of things when it comes to making an assessment of what type of risk do we fall under, and I think we roughly have a gut feeling idea on what to do when, but we don't really see it translated into frameworks that create a certain transparency right, and I hope for 2025 that we that this becomes a bit more concrete yeah like that.
Speaker 2:You know, okay, if I implemented it like this, if I used these, for example, llmops practices, then I'm, then, I'm okay. Yeah, I hope that we get a bit this.
Speaker 1:I don't know, sorry, maybe to to emphasize on that part. So, um, we did some research.
Speaker 1:So with the data strategy unit mainly focusing on, on, like, the three key trends. So there's an iso 42001, there's a part of a NIST framework, uh, so NIST, uh, national institute, so very well known also for cyber security and others, so that one that really focuses on AI, and then, of course, the EU Act, where we already touched upon earlier. So those three, we worked on a framework. So, actually, if you have those three in mind for, of course, eu based companies, we have like a checklist and we have like research done on that because, yeah, indeed, all of those things are actually there today, it's actually happening. So it looks like some theory for the future, but it's actually today it's actually happening. So it's, it looks like some theory for the future, but it's actually. It's also a checklist. So, exactly, I think in line, but that does not mean fully in line. What you were saying. That is already market standard, that people are aware of it, that people are applying it on a day-to-day basis or using it as an assessment before they actually start approaching, for example.
Speaker 3:I do believe what you say, that that is something we're definitely going to see next year I think we're going to see, we're going to start seeing this next year, but in terms of the frameworks and making sure like we are like the best practices and all these things, I I think we're not going to be in a mature place by the end of next year yet. I don't know. I feel like it would take a bit longer for people to really see what the frameworks are and make sure that everything's covered, to test it, to make sure that they are like once people that have implemented this, because it also, I guess, to gather the feedback to see if everything is compliant.
Speaker 1:It may take some time Retrofitting Exactly so there will also be some iteration.
Speaker 3:So I think we'll see some of it next year, or I hope we'll see some of it next year, but I think it would take maybe to end of 2026 for us to really say, yes, we this like for me to come to, to call a client and say this is the way to go and if you do this, you're you can sleep well at night. All right, so, um, so now. So, going to the next uh subject, and we did talk I mean we did talk about open source ai. So I also wanted to say and when I was looking back on the episodes, the open source stuff with the open tofu namely also happened to say, and when I was looking back on the episodes, the open source stuff with the open tofu namely also happened this year.
Speaker 3:I think it was 2024. Oh yeah, it was still this year. I think it was still this year. It feels like a long time ago. Yeah, it feels like a long, long time ago. Right, and maybe for the people that don't remember, the open tofu, basically, um hashicorp, which is a company, they had the terraform uh, which is an, which was an open source uh technology, and again, open source meaning that you can contribute, you can do this and that, and they decided to switch the license to something saying you can only use it if you're not directly competing with us, right, and they made a lot of noise. Uh, some people forked the latest truly open source, quote-unquote version of it and created OpenTOFU and the idea they're going to diverge. There was also the Elasticsearch thing that oh sorry, my bad, so Bart was fact-checking me, but we did talk about it this year for sure. We did definitely talk about it, yeah yeah, yeah, elasticsearch was this year.
Speaker 2:There was a lot of evolutions on this topic no-transcript.
Speaker 3:But the other thing is the Elasticsearch. This was definitely this year. You can check it Then I'm sure I'll put money on it. The Elasticsearch, basically, so they were open source. They removed the open source license because they had issues with, I think, namely aws, and this is longer ago. Right, this is way longer ago, but then this year they actually went back to open source very recently, I think very recently. Yeah, yeah indeed. So there was also some movements on the, the open source things. But now going back to so innovations and data tools and infrastructure, so what is the? Maybe moving a bit away from the AI slash Gen AI more in the data tools, data engineering tools, is there something that I know it's? I've heard feedback from people that listen to the podcast that we talk a lot about AI. I think it's also hard not to talk about AI.
Speaker 3:It's hard to talk about AI, that's true, but if we had to look, is there something that really caught your attention on the more data engineering tools or just software engineering, maybe even more in general?
Speaker 2:um, I think, as a more, let's say, big time trends, I think we see in 2024 was about the year where, uh, lake houses became like a unified default for as a workplace where you store your analytical data can you define lake house?
Speaker 3:for people that heard this for the first time now, if they think it's like a lake, a house by the lake, yeah, if we look back google images yeah, as hbt, if we, if we and this this timeline is a bit vague on me yeah, so bear with me.
Speaker 2:If we look back 10 to 15 years, everything was in warehouses, ideally on-prem, ideally on-prem, which, especially for analytical workloads, began to run into its limits. A lot of adoption of data lakes, initially via, via, via cladera or these type of hadoop distributions. Later we saw this movement to parquet on on things like s3 and stuff like this and engines to query that, and the trend towards lake. Lake house is basically a combination of those that you have a data lake, where you can, for example, land data like data that you get from different sources, or where you can store unstructured data and from there take that data and put it into a more structured environment, in other words, your warehouse. That's where the two like a lake house, a combination of a data lake and a warehouse, and what we see is that almost all big players in this space think Azure, think Snowflake, think AWS. They offer this, now this quote-unquote, the modern data platform, on a lake house infrastructure, and they all implement a little bit differently, but I think what? What we see in 2024 is really there like this.
Speaker 1:This has become the default to think about a modern data structure, it's reliable, it's tested, all these things exactly is it tightly related more maybe from a business point of view to the vast increase of the use of semi-structured and unstructured data in a company context as well? Do you think it's linked to that maturity?
Speaker 2:Definitely linked, in the sense that it gives you a lot more flexibility on unstructured data.
Speaker 2:That definitely data. That that definitely. Um, I think, uh, what it comes down to is also like, like the, the big move towards elt versus etl, where in a traditional setting, we were extracting data, transforming and then loading it into a storage. Uh, where now we extract the source data, the raw data, loaded into our storage, so we have it also uh for for later ad hoc analysis and then transform it to do something with it. Um, and the challenge of, of uh, of that latter approach of loading raw data, is that you often don't really know the structure yet, or structure might change through time.
Speaker 2:So landing that in a unstructured storage like uh, like, uh, like a data lake is much easier yeah, otherwise you'd break exactly exactly yeah, and what we see now in parallel is that you also have a lot of, especially with the llm movement, is that you have stuff like pdfs, knowledge bases.
Speaker 3:You have stuff like pictures that you want to store object exactly yeah, also on the llm you also see more that, uh, like snowflake and all these things. They are trying to bring more these, the ai to be built in on the infrastructure, the computer and all these things as well.
Speaker 2:Like you mentioned postgres before as well, so yeah, but that to me is really like uh, I think the lake house is here to stay for the coming years yeah, indeed, I think yeah no, I agree, I agree, I agree um, one thing that for me and this is very uh, it's very overfitted on my uh news gathering. I'm curious what's gonna come now uv I feel like for me this also I think you need to explain a bit what uv is.
Speaker 3:Yeah, yeah maybe also, uh, for part of the audience sitting in in this couch so this segment, this area, um no, so this is for python developers, so usually there's ways to like, manage your dependencies, to package, to basically distribute these things, and Python is.
Speaker 3:One of the main downsides of Python is that, first, it lacks a bit of standards and second is that the tooling is very fragmented. There's a lot of different tools and the latest one is UV, which is from a company called Astral, the same people that create another tool called Ruff for people that are just a linting tool, but to me, really, this time it feels different. It feels like the community is really rallying behind UV. It's a tool built in Rust, rust Anyways. So yeah, I'm actually very optimistic that this will be the way, like the mainstream way, in 2025. I don't know if there's going to be as big of a change because, like tools like pdm came and came as well, or hatch and all these things, and I did hear some noise, but nothing compared to the noise that I hear around uv maria, maybe for myself being more like a bridging person, uh, between between technical and business value.
Speaker 1:Um is going all the way from standards to cicd to so and and maybe second question sorry, combining two. Yes, why do you think this is actually going to change? And the other initiatives, world?
Speaker 3:so it's not necessarily to answer your question. It's more on the Python development. You can use these things on CICD, but it's not like a CICD tool. Okay, right, so it's something that you can use when you're calling the continuous integration or deployment stuff, but it's really more focused on the Python level. Why I think this is different. One I think the space is more mature. Two there is a company that at least exists now, so there's people that invest a lot of time on this, so they actually move very fast. Third thing is the user experience is the nicest one that there is for now. It also learns from other experiences. Again, it is more mature, but they learn also from experiences from other tools and from other programming languages as well. But I think the main thing is that I do see a big community uptake and a lot of people that are very passionately advocating for this tool, which, yeah, like if you include having community around using the tool, it makes a huge difference right like even if you have the best tool in the world but no one uses.
Speaker 3:It's a bit like if a trial for particles, like if a tree falls but no one hears does it. You know it's a. It's a bit like that. So I think all those things combined the fact that they're always trying to implement the standards quickly, that we have more standards, and all these things I, I, and also the, the things that are here I do have a feeling this is the. This is gonna stay for a while. So it's a very, very niche thing, but something that to be also stood out this this year as well I was wondering you about the infrastructure part.
Speaker 1:Is real time still something we need to talk about or nothing really changed about real time in 2024?
Speaker 3:well for me. I may be a bit biased as well, because we're talking about gen ai, and most of the times when you're talking well, gen ai, uh, well, real time, I'm thinking of real time as an event driven right so you do something and then right away I give you something back, right?
Speaker 3:So real-time, is it a second, a millisecond? Maybe it depends a bit, right? I'm calling everything real-time. When you talk about Gen AI, most of the stuff you do is well, most of the applications like chatbot and all these things like parts, example, on someone ringing the doorbell, that's real-time, yeah indeed Companies also, I think more thinking in event-driven, in real-time orchestration, I think definitely the large companies we interact with.
Speaker 1:They still have a lot of batch infrastructure, still have a lot of operating systems that are anywhere close to near-time. So that's why I was like maybe it's 2024 and 2025, still about real-time and the challenges around that.
Speaker 3:I think in the. I'm curious to hear what Bart thinks as well. If you think of now, I'm talking about AI. This is where I'm more ingrained in, let's say, traditional machine learning or AI or data science, however you want to call it. I still think it's a bit more batch based. It's like more of the ELT or ETL processes, at least as I see it, the Gen AI. I think it's way more on the elt or etl processes, at least as I see it, the gen ai. I think it's way more on the real time side. People are you know. I think it's also more intrinsic on the experience you have with chad gpt. Right, you do something, you wait and you get something back um challenges.
Speaker 3:Yeah, I think things can all be like well one thing I see here on the notes and we also talked to the ceo of red panda right, which he also brought this up how you can have basically streaming data. So I think things can all be like well, one thing I see here on the notes we also talked to the CEO of Red Panda right, which he also brought this up how you can have basically streaming data, so basically data that kind of continuously come in and in that process you can also embed some AI in there. I, yeah, I think, and again, maybe just the streaming thing. I remember from the conversation that it's a bit of a in-between the two the real-time and the batch thing. Right, I think real-time for Gen AI is there. It's going to stay.
Speaker 3:In terms of challenges, there could be new challenges that we create quote-unquote, like if you want to host your own models, if you want to have I don't know. Another thing that we saw quote-unquote, like if you want to host your own models, if you want to have I don't know. Another thing that we saw is that you can have an lm router quote-unquote. So basically, depending on the question, you can say this is something to use, a very expensive big model that you need to use open ai. Maybe this is something you can host yourself. Um, there is like olama, which is something that basically for you to host your own models. Maybe you can put on top of Kubernetes. There's some stuff you can do.
Speaker 3:Today, we found a way, in my opinion. Right, there will be. I do think that's something that is going to come more as well in 2025, right, because people are going to be worrying more about it, I think also as people use it more. Okay, this is there to stay. Is there any way we can make it more? Uh, okay, this is there to stay. Is there any way we can make it more efficient? Could cost, etc. Etc. This may be also another question there. What? What do you think, bart?
Speaker 2:I'm curious also to hear your thoughts on this um, I put this down for my uh hopefuls in 2035. Okay, real time, um, real time spans a lot. So I think what we're talking about is is real time spans a lot. So I think what we're talking about is is is real time quote, unquote data analytics? I mean, what we're not discussing here is is like, like transactional systems, right, like everybody agrees there, like you need to be close to real time.
Speaker 1:It's more on the consumption side.
Speaker 2:Yeah, it's more on the on the consumption side. So I think the challenge there that we have today is that, where you have this, uh, in a more, let's say, batch process, where you have, where we have today, elt, what we're, what we're just discussing we have a lot of more or less tools that have become standards. If you take dbt for transformation, these type of things, because what you typically need to do when you ingest data let's assume it's real time it's going to be multiple data sources and you need to do some transformations on them. You need to bring them together to get insights, take an action, trigger your agentic AI, whatever you want to do. And I think the challenge there is that it still requires a lot of engineering effort unless and that's not the case today that it still requires a lot of engineering effort Unless and that's not the case today unless you have set up everything from scratch, really in an event-driven manner.
Speaker 3:Yeah, but that I agree. I think also relates to Yannick's point a while ago that these are not all start-from-scratch projects. Right, there's a lot of stuff that already the reality is that that 95 is not start from scratch?
Speaker 2:right exactly so and I think, like real time becomes easy when you have a full platform setup, event driven um, which is often not the case, aka greenfield exactly, and and even if it's fully event driven and let's let's assume they use something like kafka or something like, it's still a lot of like.
Speaker 2:There's not like let's use this tool and we can join these different streams, et cetera, et cetera, these different queues it still requires a lot of engineering know-how, like this standard in a non-event-driven system when it comes to data processing at least.
Speaker 2:Like there's dbt, but maybe there are different sources, and like there's lakehouse, but maybe there are different tools underlying that. Like it's, it's relatively easy for someone to switch environments and still be productive, just learning to understand different sources and destinations. I think don't think you have that yet with real-time. So you have some evolutions where, like Materialize, which is a data platform that allows you to do these type of things, where you can basically build more like SQL-like views and tables, but they're up-to-date with real-time data and you can actually use them with dbt, but there are very few standards. There are very few standards like it's um, and I hope in 2025 we will see more like that. You can do it a little bit with dbt, with incremental views, but it's not really real time. You can do a little bit with micro batching, but it's it's a bit. It's a bit misusing it to get something done so you mean like for?
Speaker 3:just just to make sure I understand. When you're saying like real time, you mean more, like Yannick went on a website today, like now, and I have a dashboard and I can see Yannick's. How do you say like a history, not a flow, like the?
Speaker 1:website flow. How do you call it? The traffic behavior? Yeah, like the website flow. How do you call it? Uh, the traffic?
Speaker 3:behavior. Yeah, like the traffic behavior, I can see that within a second or something in my dashboard.
Speaker 1:That's, that's what that's maybe as a use case was more thinking about the dynamic pricing, uh, for ev charging. Okay, because if you take into balancing grit cost of distribution, all of that should be close to real time, which is not the case today, but I think it it could be very powerful use case I see, okay, okay, so basically, like, based on the input we have, now you have to compute something.
Speaker 3:You already make changes on the actual pricing of the infrastructure, but that's like, just so I understand better. Like, so there is still an etl process which today is very much batch, but you want to make that from the new input to the actual new output to make it very, very it's maybe a bit.
Speaker 2:Let me try to sorry, make it a simpler use case yes right.
Speaker 2:So, uh, I have an event. Uh, a visitor is on my e-commerce website. Okay, I get this trigger, real-time trigger, this trigger, I'm going to link this person to his history historical card. And I know, ah, this person was looking at these and these data. It's going to be Black Friday, so I'm going to send him an email. Uh, if you finish this car now, you get 50 reduction. But what that means in a very minimal setup is that you, let's say, you use shopify, you pull that into your data warehouse. You have a rich view on this customer. Like, who is this customer? Where does where does he live? Is it maybe a vip customer? Uh, can we uh take a loss on this customer because we want to want to keep him? Um, what was his card history? Like, you have all this information about the customer. Plus, you have this real-time trigger.
Speaker 2:Like, ah, this customer is here now there's an intent yeah, intent to buy and you want to bring this together like this, this, this information about a profile and what is happening now, and then do something with it, which maybe sometimes is called reverse etl. You have, we have, that we are ingesting this data, transforming it into some, into some conclusion, and that you want to do something with then. Next, and in a very minimal setup like your, your, uh, your shopify stuff, like every hour lands incrementally in your, in your data warehouse, so you have more or less an up-to-date profile. But from the moment that you say, okay, I have this real-time trigger, I need to do something with it now, then suddenly you can't use the t and dbt anymore to transform because because data, data wise, you could perfectly, could right, like you can perfectly come to a sql-based transformation, but dbt is not real-time. So you need to start adding custom stuff there.
Speaker 2:Yeah, I see what you're saying, which should not be needed because in the end it's just a data transformation, I see, but because it's not really there now or you need to maybe a bit misuse how you're doing it. You're going to build like a custom Python script to cover these things and before you doing it, you're going to build like a custom Python script to cover these things and, before you know it, because you don't want to respond to this trigger, but like 50 of these triggers, you're going to have either 50 different Python scripts and not really knowing what's happening, or you're going to say, okay, no, this is really not keeping, this is not. We're going to go all the way, we're going to go Kafka and you need to fully redesign the system.
Speaker 2:And like there is no good way, no good, easy way to integrate these type of things now.
Speaker 1:But we just discussed it. But agents will solve that next year, yes, but I really liked the example because it's very tangible and it's exactly the gap between end user expectations and potential future behavior and like, still the way to. There is way more steps than an end user expectations and potential future behavior and like, still the way to. There is way more steps than an end user envisions at the exactly, exactly, yeah I like the idea.
Speaker 3:Yeah, indeed, I think no, but I agree, I think today I'm thinking again like of uh, recommendations or something like if you want to trigger the email, if you ask me today, I'll probably think of like feature stores and like a rest in point, that like calls, just that to get the context, and then you have machine learning, whatever to generate the email. Um, but I guess what you're saying is like we don't like it's very, it's very crafted.
Speaker 3:You still need to craft a lot of things, put a lot of things together and when you craft very case by case very case by case and when you see that doesn't scale very well as well, right yeah, that's a, that's a a good point I do think it's a.
Speaker 1:It's a fair tool and infra challenge for next year and any maturity still uh yeah yeah, that is data space still to uh, I agree.
Speaker 3:But I and I also agree that, yeah, saying I'm gonna start from kafka from scratch now, it's not the solution. Like I don't think it's now it's not the solution because I don't think it's gonna it's not gonna solve the problems that we have today, because most people are not gonna say, okay, let's restart from scratch and just do this whole thing. And I think, if you start something new.
Speaker 2:Uh, I'll take a solution application platform, whatever. I think from an engineering point of view, there are very strong arguments to make why you should do this, event driven, I think, from uh, longevity, finding skills, finding people being able to grow this.
Speaker 3:I think you should doubt yourself but that's also what I was gonna, that that was gonna. I was gonna challenge as well, like, if you have just the standard data engineering team, yeah, it's uh. You know like how many of them would say, oh, yeah, no, we're all comfortable, we can do this, I can give you this in three months and I have zero uncertainty because I've done this multiple times and this and this, and I can see there's a lot of examples online, there's a lot of documentation, so I can do this. I just don't think that's. But again, I think you perspective right. I do think dbt is something that most engineers will know. Spark most data engineers will know.
Speaker 2:Um, but yeah, like the, the streaming stuff, not sure how, how there are we already and I think, when we talk about spark and dbt, what it comes down to today is doing something with data, which translates to sql yeah, today I think, and I think there, we, we, I hope and that's what materialize is trying to do, for example like that, we see that also becoming more of a standard in the real-time world, but we're not there yet.
Speaker 3:Yes, yes, we're not there. So maybe on the last data innovation and leadership in AI, what should technical data professionals so I'm thinking data engineers and GNI engineers and machine learning engineers and people someone from DataWits, for example what should they focus on to stay relevant in this future, this 2025 future? So I already mentioned that you would like to see some more real-time easiness.
Speaker 2:Do you think that's what people should focus on? I maybe have a uh, a less hopeful one. Okay, there's a counter question no when it comes to like what should you, as a person in the space, be doing, and I think especially people that are today coding?
Speaker 1:in the technical space in the technical space.
Speaker 2:I think what they, what all these ai tools do today already and I very much do this myself as well um, and I see, like, like leverage smart tools to more quickly write code, and I think, if I compare myself with now versus two years ago, this is a very significant difference on how quickly I can get a prototype out, with the emphasis on prototype. But I think what this will mean is that there, the market will have a higher expectations in terms of the efficiency of the average software engineer and I think if you do not keep up like this is a serious risk of getting burnout because the expectations will increase. That's what that's. That's that's honestly my, my concern for the future for developers, for developers developers, developers, if you don't keep up, you will.
Speaker 2:You will still get more questions because expectations will change, and then that's not a healthy combination.
Speaker 1:I think I read, uh, I read an article so sorry, but I read an article in the context that for junior developers is considered as as negative because they don't fully understand every advice that is given by a co-pilot. But for me, during senior like the efficiency, effectivity, consistency and everything you see a gigantic uplift. So I do think that's maybe the small, let's say, counter argument or small like nuance I wanted to bring to the answer. Like co-piloting, uh, for somebody who has no, let's say, solid background or very little experience, could be also a risk of not fully understanding and not fully, let's say, solid background or very little experience could be also a risk of not fully understanding and not fully, let's say, going through the gold curve.
Speaker 3:Yeah, yeah I think also. I mean, I fully agree I was gonna say.
Speaker 3:I agree with also your true saying that the expectations that people need to be productive are going to increase. Um, but I also think indeed, like coding with ai, assistance is also there are some different skills, right, like to review the code, understand the code, see if this is really how you want to do it, because I think if it's, it's easier to churn out code. But even for us, I think most of the times you need to understand what's there and I think that part to make it easy to understand needs to be more there. I see you're talking about the challenge for juniors now, juniors especially because I think juniors they're less concerned about how to read the code. Like, yeah, it's easy to. If you have to change a function, it's easier maybe to just create a new one and keep the old one, right.
Speaker 2:I agree with that and I think the challenge of Gen AI is that. So before you could bring the argument yeah, they're going to copy paste something from Stack Overflow.
Speaker 1:Which of course?
Speaker 2:happened, which of course happened, but it was much more harder to like one-on-one copy paste it and it would work. Like you still have to tweak it right.
Speaker 3:To understand it, to understand it, to make it work, and with Gen AI to make it work.
Speaker 2:And with Gen AI it's easier to make something that just works without truly understanding.
Speaker 1:Yeah, and now the downside to add to that. But it will run in the given context, but to what extent is it actually stable, maintainable Is it? And that's, I think, the challenge. Now you can run it and it will work, but is it like solid? But I think that same junior if this is the level.
Speaker 2:If you're concerned about whether or not he, what he builds, is good, then, like without gen ai, you should also be concerned about it. I think what it comes down to again is a bit like the devil of best practices is having defaults on on how do you write code, how do you test code bit of test driven design maybe, and it's maybe we need to have more hygiene on that for starters. Going forward, yeah but it's not like but it's not like the solution on that is not.
Speaker 3:It's not a very difficult one, it's just good devops practices yeah, yeah, but I also, I also think that, uh, now we're talking a lot about software engineering yeah and I think at least for like data scientists, they ever they're yeah, they have a. How do you call it Misfame? It's not a misfame, but infamous for not following as much the soft engineering practices. Single soldier armies.
Speaker 3:Yeah, something like that. So I think it's also, yeah, devops. The answer is not new, it's there, we should all know it. But I also think there's a lot of people that are well, two things First, most of the stuff we do is in Python, which is a very forgiving language, right, If we're using something that is strongly typed, it is compiled. There are already more guarantees. But Python, if you just do it runs, it's fine. So I think it increases even more the importance of testing, the importance of understanding, covering test cases, even if you want to add static typing to already exclude a whole bunch of tests that you would need to do, right.
Speaker 3:But then there's also, yeah, data scientists. They used to be well, even in engineering. You know, like with the notebook stuff, how a lot of people say it's an anti-pattern. I'm not gonna. I don't want to necessarily discuss the use of notebooks, but, uh, I have an impression and I don't know if it's been changing but the traditional data scientist, it wasn't someone that is super comfortable with all the DevOps stuff to implement all the things.
Speaker 3:I also think there's a lot of, again, pocs, a lot of experimentation, and sometimes adding these guardrails also feels like it slows you down, even though I also have a theory that it will also speed up on the long run because to modify and to fix bugs it's going to be much faster because it's more organized. But there's also a bit of resistance. In that sense, I feel like I have to. Really, I don't know even stuff like MyPy, right, mypy is a static type checker and it was by default on the project. But then I just saw that people are just putting any everywhere. So basically just ignore all the types everywhere, right, um, and then you make progress, but then if you need to change something or something breaks, it's it's much harder to to understand. Like me, when I go, when I look in that code, I try to understand what it does.
Speaker 1:It's much, much, much harder that brings us back to bart's remark about the hygiene.
Speaker 2:Indeed, but, I think it's also like, and I think, if you bring it back to more like leadership, more business level, I think there's companies need to understand like it has an impact on how fast it can go to market with new features, but that it also has an impact on the workforce, that they need to support it, that they need to guide this, but also that they need to enable this and allow for experimentation.
Speaker 2:There's a bit two sides of the same coin. What I think also it goes a bit hand in hand when it comes to leadership. It's also more focused on and I think we've been discussing this for the last years, but I think it's becoming even increasingly important with now, with LLMs it's like a focus on data as a valuable asset. So data is what will enable Murillo's agentic agents and I think, when it comes to data and to make the link there from DevOps, companies also need to look at their data more in a DevOps kind of way. I think the new hype term for 2025 will be DataOps, but it's not a new term. Huh, it's not a new term's not a new term, but there is. It is something where we don't have any clear standards either, like we don't have clear standards on.
Speaker 2:I want to, indeed yeah, it's something like lmops. I think it's very. You can draw parallels like there are subdomains where we are making evolution, but there is no clear, like this is how you do it, um, but I think this has become more and more important. To so show. Like there is no clear, like this is how you do it, um, but I think this has become more and more important. To so show like there is a clear data lineage. There is, there are some checks on the ingestion. You have monitoring of what you have actually there. You have a clear observability on your data. You know in which products, which which derivatives of your data are being used.
Speaker 2:And I think, from the moment you really want to go to the next level with these, these uh, ai driven solutions. You need to have that in place. That is uh, and I think that is an understanding that is not really there yet because it's really behind the scenes. Like you don't see. Like if you're not, if you're not working as a data engineer, you don't see the challenges. That's a bit to the, the. I think that is a. It's very hard to make the argument like there will be an ROI because you do this.
Speaker 3:Yeah, yeah, yeah, yeah, it's more of a like.
Speaker 2:this is a prerequisite that you need to have good enough in order to create value, and that makes it hard in these discussions and I think also on boardroom level.
Speaker 1:I think that the gap is as big as, as bart just mentioned, it is like there are more and more ambitions to do bold, innovative things, and also from a budget perspective, I think it's equally important to take a step backwards, like so in french we say so take a step back, to then take at least two leapfrogs or two steps forward.
Speaker 3:So the data catalog, the lineage, better understanding who's using which data, who is accountable for which domain, all of those things to then, when that's all done, jump into more, let's say, more tangible or more exotic data use cases. I agree, and I also think that, if you take the, the least optimistic or the worst case scenario is that you just kind of build.
Speaker 1:You don't have very solid foundations, you just build on top of that, at one point something cool on a very shaky foundation yeah, and then at one point just everything, the house of cards kind of like all fall down right.
Speaker 3:We heard also from an internal Slack discussion that the use of Gen AI. It was a free university project, so I'm assuming they're more inexperienced developers that they just kind of had to stop because they just kind of it was very easy to add stuff, but again the foundations weren't there, the designs weren't there, the maintainability wasn't there, and then at one point it's just we just cannot continue with this. This is this is no one knows what is done, no one wants to touch anything either, because there are no tests, right. So if you change a, then maybe b breaks and you don't see it and people just kind of say, okay, let's just start from scratch. And I think that's the the worst case scenario.
Speaker 3:That's what I think too that's maybe one of the more skeptical key takeaways for next year, but I do think it's a valuable one I do think, yeah, I think the the skills for software, I think maybe to frame it a bit differently, like the skills of software engineers are constantly changing. I think the change now with gen ai is maybe a bit more clear. But I think the ability to understand code, the ability to write a good code, the ability to um, yeah, to test, to make sure you are promoting these things, um becomes more, more important, right? So that's what I I would also advise data or software engineers, but data professionals as well. And the other thing I would also say is to again, like part said, like, do try to use these tools. Uh, if you're not using, you are falling behind. Um, understanding the basics as well, uh, it's something that the default software engineer even, I think they they are already familiar. They should be familiar with the basics, because otherwise you are falling behind.
Speaker 1:In my humble opinion, and the same goes for, let's say, more hybrid roles, more business roles. Uh, the same goals like, if you're, if you're not testing it today, someone else will. So you're competing with somebody who's trying it out, who's exploring those things. So I think, uh key takeaway is it's never too late. Today is the next best day to start just for those who are maybe not, uh, experimenting with the co-pilot look-alike projects, yeah, and I think companies need to realize this shift needs the correct guidance.
Speaker 2:I think, because we're very hands-on involved in these things, we try to underestimate it, but I think when you look just even historically, people going from typing text and WordPerfect and MS-DOS moving to Office on Windows was a big change and required training et cetera, et cetera. And I think even like people writing code now in VS Code and going the next step with Copilot and stuff like that, doing that efficiently is a big change we should not like for some people. It's not For some people. In their evenings they're very passionate, they'll try everything out. But I think we need to realize that that is not the default. It's not going to go by its own Like you need to invest in it. You need to invest in training of people.
Speaker 3:Yeah, and one talk that we did actually, we had a conversation with a team of years as well a few days ago, still going to release this.
Speaker 3:We also touched a bit upon that, like how there maybe needs to be an effort for the tools to meet the users where they are more um in this sense, for example, uh, embed the very simple, silly example, but like, embed the limb model in the email client instead of having people switch, to make it easier for people to adopt. So it's like a smooth transition, but also there needs to be an effort from the users to really try. I think you need to go a bit outside your, your worn, well-worn and tested way of doing things to redefine this better way, right, and I think Gen AI a lot of times is for this. Another example is like I started using Cursor, which is like a AI-focused VS Code forks on IDE, but there are some changes in the way that you interact with your code. There are some different ways on like where to search stuff, where to do A and B and C, which doesn't come as naturally in the beginning.
Speaker 1:But once you get used to it it's like, yeah, way, way more productive here. Yeah, if you can identify some ambassadors inside your company, you can be very vocal indeed, evangelize values and about really setting up ad hoc sessions at first, to to do it slow and to just like emphasize on the benefits. Uh, I think that's that's how we.
Speaker 3:We also approach it in strategic exercises at the moment you know, really cool and I think, uh, with that we can wrap it up, unless there's something else you want to say, any inspiring last words, yannick, but? But yannick, but part was gonna say something, interrupt him, go for it. I saw you like you're eager to go for it.
Speaker 1:I think we could continue for at least another half an hour.
Speaker 2:You continue to 25. We touched upon a lot of topics.
Speaker 1:I think it was very, very nice Also to see, let's say, the bridge between the impact for companies, the impact of technology on uh data, uh as a whole, uh, looking back at a lot of podcast work that was done, uh, zooming in and zooming out at the same time. So I really liked it and think it's very valuable um to uh, to continue uh and see what, what 2025 will bring indeed, I think there's a lot of exciting stuff to look forward to.
Speaker 3:For sure, cool alright. Thanks, y'all. Thanks, yannick, ciao, ciao, ciao. You have taste in a way that's meaningful to software people hello, I'm Bill Gates.
Speaker 2:I would, I would recommend TypeScript. Yeah, it writes a lot of code for me and usually it's slightly wrong. I'm reminded, incidentally, of Rust here Rust, rust.
Speaker 3:This almost makes me happy that I didn't become a supermodel.
Speaker 2:Cooper and Netties Boy. I'm sorry guys, I don't know what's going on.
Speaker 3:Thank you for the opportunity to speak to you today about large neural networks. It's really an honor to be here.
Speaker 1:Rust, rust, rust. Data topics. Welcome to the data. Welcome to the data topics podcast.
Speaker 2:Are you happy, Marilu? Happy that you did not become a supermodel.
Speaker 3:Now it's Jenny I oh, yeah, yeah, happy that I know I chose a. I wanted to help people, you know, so I was like no.