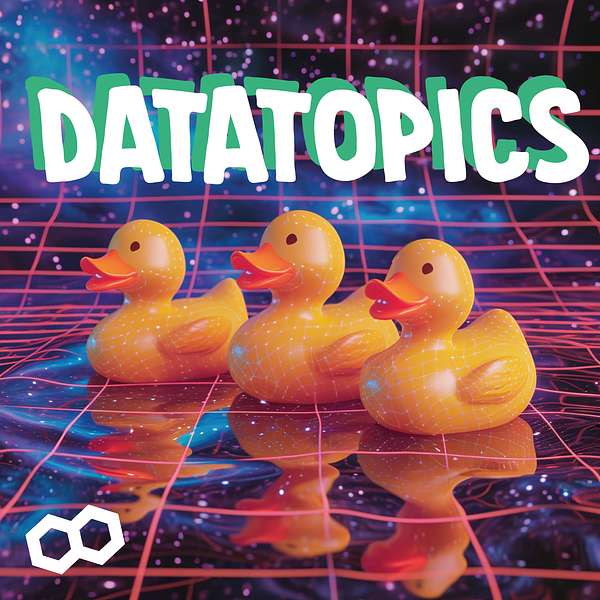
DataTopics Unplugged: All Things Data, AI & Tech
Welcome to the cozy corner of the tech world where ones and zeros mingle with casual chit-chat. Datatopics Unplugged is your go-to spot for relaxed discussions around tech, news, data, and society.
Dive into conversations that should flow as smoothly as your morning coffee (but don't), where industry insights meet laid-back banter. Whether you're a data aficionado or just someone curious about the digital age, pull up a chair, relax, and let's get into the heart of data, unplugged style!
DataTopics Unplugged: All Things Data, AI & Tech
#68 GenAI meets Minecraft, OpenAI’s O1 Leak, Strava’s AI Moves, HTMX vs. React & Octoverse Trends
Welcome to the cozy corner of the tech world where ones and zeros mingle with casual chit-chat. Datatopics Unplugged is your go-to spot for relaxed discussions around tech, news, data, and society.
Dive into conversations that should flow as smoothly as your morning coffee (but don’t), where industry insights meet laid-back banter. Whether you’re a data aficionado or just someone curious about the digital age, pull up a chair, relax, and let’s get into the heart of data, unplugged style!
In this episode, we are joined by special guest Nico for a lively and wide-ranging tech chat. Grab your headphones and prepare for:
- Strava’s ‘Athlete Intelligence’ feature: A humorous dive into how workout apps are getting smarter—and a little sassier.
- Frontend frameworks: HTMX is a tough choice: A candid discussion on using React versus emerging alternatives like HTMX and when to keep things lightweight.
- Octoverse 2024 trends and language wars: Python takes the lead over JavaScript as the top GitHub language, and we dissect why Go, TypeScript, and Rust are getting love too.
- GenAI meets Minecraft: Imagine procedurally generated worlds and dreamlike coherence breaks—Minecraft-style. How GenAI could redefine gameplay narratives and NPC behavior.
- OpenAI’s O1 model leak: Insights on the recent leak, what’s new, and its implications for the future of AI.
- Tiger Beetle’s transactional databases and testing tales: Nico walks us through Tiger Style, deterministic simulation testing, and why it’s a game changer for distributed databases.
- Automated testing for LLMOps: A quick overview of automated testing for large language models and its role in modern AI workflows.
- DeepLearning.ai’s short courses: Quick, impactful learning to level up your AI skills.
you have taste in a way that's meaningful to software people hello, I'm bill gates.
Speaker 2:I would. I would recommend uh typescript. Yeah, it writes a lot of code for me and usually it's like you're missing out.
Speaker 3:You can just put it just for the song, just for the song every night you just like rust.
Speaker 2:This almost makes me happy that I didn't become a supermodel.
Speaker 3:Uber and Nest Boy. I'm sorry guys, I don't know what's going on.
Speaker 2:Thanks for the opportunity to speak to you today. I don't think it's good catching.
Speaker 1:This is Data Topics. Welcome to the Data Topics podcast.
Speaker 2:Hello and welcome to Data Topics unplugged, your casual corner of the web where we discuss what's new in data every week, from Minecraft to the Octoverse, everything goes. Check us out on LinkedIn, youtube. Feel free to leave a comment or question or send us via email. We'll try to get back to you. Today is the 8th of november of 2024. My name is morello, I'll be hosting you today and I'm joined by my sidekick, podcast sidekick, but the life mentor, I'm not sure. Let's just try to spin it back.
Speaker 2:Bart's just made it awkward yeah, I have run, uh, and we have a very special guest today.
Speaker 1:Nico.
Speaker 2:Yeah, for sure, hold on. I think I'm going to put the applause.
Speaker 1:Thank, you Glad to be here.
Speaker 2:Glad to have you here, nico. Nico is one of the tech leads for the data and cloud business unit, data Roots. He's well, why don't you introduce yourself? I don't want to. Yeah, well, as Murdo said, technical lead for data and cloud roots. Um, he's uh. Well, why don't you introduce?
Speaker 1:yourself. I don't wanna. Yeah, well, yeah, as more low sets, uh, technically for data and cloud, been at data roots for almost five years. In general, it will be five years, um, and I've mostly been working on data platforms.
Speaker 2:Uh, from the more from the more the cloud side.
Speaker 1:Yeah, and when you're not designing data platforms, you're cycling right here I'm cycling, yes, and next to that I also found another love, but in the same uh, in the same vein as, uh, my, uh, my work, uh, more like software engineering and like recreating tools that already exist okay, cool in Rust, of course no not in Rust. Wow, hold on, let me just right now I'm I'm working with Go cool.
Speaker 2:Go, but you already knew Go when you joined DataWits. No, I remember there was a no, but you already knew Go when you joined DataWits. No, no but you worked on Deploy, which was in Go. No. I did the Python part oh ok, I thought you did the Go part cool, so yeah, and the reason why I brought it up is also, nico, he's. You cycle from Belgium to Austria that's one of my feats it's what it does on fridays.
Speaker 3:Yeah, yeah, it was three days.
Speaker 1:It was three days, but still.
Speaker 2:I remember like. So the backstory is that we do a yearly ski trip at data roots and then nico's like oh no, I cannot make. Like, actually, like you're very direct, right for people that don't know, nico, and he, I think you just put it on slack. You're just like I can't make it. I got covid and everyone's like, oh man, oh, I'm on Slack. He was just like I can't make it. I got COVID and everyone was like oh man, oh, I'm so sorry, but it was really like five words, you know. And then, like, we went with the van and then the next morning I just saw Nico sitting there and I was like what the fuck?
Speaker 3:I was like, did you? And he cycled all the way there.
Speaker 2:Yeah, and I, I was like, did you just say you had COVID? How many kilometers?
Speaker 3:was it 1200 or something?
Speaker 1:no, no, it was 900 kilometers, almost 900 kilometers still yeah in three days.
Speaker 2:So yeah, that's alone and it was also not. It was in the. It was like winter.
Speaker 1:No, not winter, but it was going towards well, at end of March, I think, somewhere there, and I had really I was really lucky because it was three days no rain- yeah, that's good.
Speaker 2:I imagine that if it was raining like ice and stuff, it's probably not. It's also dangerous. No, but did you have to cycle like on the highway as well?
Speaker 1:No, not on the highway.
Speaker 2:There was like also back roads and stuff.
Speaker 1:Yeah, well, it's a bit difficult of creating a route for 900 kilometers.
Speaker 2:You don't put on Google Maps and just go.
Speaker 1:Well, yeah, something like that. But then I looked a bit like, if there are rivers or something, I'd rather go along a river than along streets, because I know a river, even though it's like 5 or 10 kilometers longer, it's flat and no cars.
Speaker 2:True yeah.
Speaker 1:I think there were like two or three roads where I was a bit afraid, because sometimes it's a road where you can go 90 kilometers an hour yeah, you got a cycling lane, so that's a bit uh yeah, I can imagine, I can imagine, but uh, well, yeah, very cool, very cool uh.
Speaker 2:But what do we have here today? Maybe I think the most, uh, well, okay, bart maybe it's a good segue to strava strava. What about strava bart?
Speaker 3:strava. It's actually just something fun that I saw popping up today. Uh, strava is a platform for people that don't know stuff, as a platform for at least, like they record their their uh, their workouts there, basically, and it's also a bit of a social platform where you can like, you have followers, you can follow people, stuff like that and Strava now has athlete intelligence in beta.
Speaker 1:I've been laughing at that for a long time.
Speaker 3:Athlete intelligence, athlete intelligence. I actually didn't know. I just saw someone from our team, thibaut, posting a screenshot that he got after he went running.
Speaker 2:Really, what does he do? I'm trying to find it here.
Speaker 3:It was a very motivating message that he got from an athlete.
Speaker 2:Ah, but that's the athlete intelligence. It's like go get it tiger.
Speaker 3:No, it says he went on a running trip right as he went running and he got a message from Strava Athlete Intelligence on his mobile. Great job on another activity. This activity was recorded in trail run mode, but based on your workout analysis, we suggest recording future activities in hike mode. Selecting the correct sport will provide you with the most comprehensive and accurate data.
Speaker 2:So basically it's like you were walking yeah.
Speaker 3:Let's all be honest, she was just walking, okay, so this is what AI Strava brings you.
Speaker 1:I also got one A bit of the same, like a bit of context. When I go cycle I always go by a river, so it's flat and so I don't know, sometimes I go over a bridge or something, so it adds a bit of elevation height. So now it gives me like the comment like oh, you did 30 meters of altitude, that's higher than your average.
Speaker 2:Good job. Meters of uh altitude. Uh, that's, that's higher than your average wow, yeah, yeah, yeah.
Speaker 1:It's like wow, it's so impressive. I would never expect you to do this. I don't know like it literally doesn't add anything to my experience but I think also, these things is like I don't know.
Speaker 2:I mean, I think the classifying the activity it's not something very new, right, I think? Uh, apple watch has been doing this for a long time. A lot of other devices have been doing this for a long time, right?
Speaker 3:uh, that's true um being burned by the app yeah, that's true.
Speaker 2:Now they have their lamb too.
Speaker 1:You know, it's like maybe later you can like have a slider like how sarcastic yeah, yeah, exactly how sassy. Yeah, a bit like uh uh the, the robots from uh interstellar. You can say it uh yeah the level of sarcasm and all these things yeah yeah, yeah, that's true.
Speaker 2:I actually think could be also like a nice social experiment. You know, like how do you motivate yourself if you're saying some guys like, nah, you can't do it, just go home. Uh, cool, cool, cool, um. Well, maybe also while we have nico here I know you are, um, we have the roots come coming up. You're gonna do to do an HTMX workshop.
Speaker 1:Yes.
Speaker 2:I'm just reading the show notes, but apparently someone thinks that HTMX is a tough choice.
Speaker 3:I didn't know that you were going to start out with this.
Speaker 2:Well, I wasn't going to, but I was looking for this Strava thing and I saw this there and I saw Nico and I was like, well, if he's here, I think it's also good to let's clear the house. You know we were gonna have this clash at the beginning of the part so well, I think let's set the tone here and then, if you don't have time for anything else, that's okay.
Speaker 3:Yeah, maybe maybe nico you can. You can give like uh, for people that don't know, like a very short, like what is htmx? When would you use it?
Speaker 1:um, yeah, if you don't want to get into, uh, front-end frameworks like React or something or Angular, you want to stay on the server side with very minimal HTML text. You can basically have a plus or minus good experience in terms of not really your activity but navigating the web and doing post requests and stuff like that.
Speaker 3:Um, so, yeah, that's very, very, very, uh, a basic explanation and um I have and I think, like on paper, like if you try it out to get started, so like it works very intuitively it's with. It brings you much closer to just writing html and css instead of like a huge framework that you need to get to get to know. Like react of you or and it works a bit like like it and you annotate your html elements to give it extra functionality. Like instead of um, like you can have a button, actually send a certain request on Submeta, like these type of things. And over the last two years I think it really hyped like a few years ago, right.
Speaker 1:I think, yeah, a year, year and a half ago, yeah, by some YouTubers.
Speaker 3:Wow, is it.
Speaker 1:I think so. Are you going to name names? That's where I started from, so.
Speaker 2:Put them on the pod. Which YouTubers it?
Speaker 1:yeah, yeah, I think so, yeah, well, yeah, that's where I saw it from, so put him on the pod. Who which?
Speaker 3:youtubers uh, the primogen and teo and uh, I've been trying. Okay, always start out with it when I have a new small project, and I always move away from it I can understand that, yes wait, wait, why wait?
Speaker 2:I think that's also interesting comment. Why, why do you understand? Why does why does it come so naturally to you?
Speaker 1:Because I've worked on the other side as well. I've worked with React, I've worked with other frameworks and, yeah, you get familiar with them and I think Bart is also familiar with them and then it's basically a shift in the model that you have to think about, and there are some caveats that there are that you have to think about, and there are some caveats that there are that you have to know. Sometimes you think it's going to work, but then yeah, you try 15 different things, it doesn't work.
Speaker 1:And then it's just because the JavaScript of your library, that's your component library, hasn't annotated some elements. I see, so I mean there are some elements, so I mean there are some pitfalls, but I mean, yeah, it's something different.
Speaker 2:But then it's more because people are. It's a new way of thinking, mental model, kind of so people that are not familiar if they're already doing other frameworks. The hardship of transitioning. It's easy, it's very attractive to just go back and just get it done.
Speaker 3:I think, HTMX, you get started very quickly, but from the moment something becomes bigger and composability becomes a thing and maintainability becomes a thing all of these things there is a framework for it in React, for example. There is a good mature routing framework, these type of things. They've been tackled. At a certain stage you need to reimplement it for the feeling in HTMX. Is it because? Or you need to start forming your own opinion zone as well? In React, there is an opinion on how to do it.
Speaker 2:But then do you think it's with time HTMX will get there, or do you think it's more like the way the HTMX is set up?
Speaker 3:it's not easy to build these, I think, for people like me. There are a lot of people that's using hd mix in production, so I'm just talking out of my own experience here, but not now, recently, and it's maybe, uh, the the most recent reason why I switched I think is an interesting one in this context is actually genii based. So we were playing with v0. V from vercel, the genii component generator. V0 from Vercel, the Gen AI component generator, which can generate HTMX. Actually, is it?
Speaker 1:Yeah, I tried it out, is it?
Speaker 3:new, I don't know. Okay, tried it a week ago, we talked about it a little bit on the pod but by default it generates a React component, right, yeah, so I generate a React component. It looks very nice, it uses a chat CDN for for components, for styling and stuff like that. Everything out of the box looks very nice out of the box. And then I asked do this for hdmx, for me, you know? And then it does generate it and then you need to say, okay, but what with what kind of template like? What kind of css? Like? You get very custom stuff.
Speaker 3:So either I was going to go with this HTML and then do very custom CSS stuff, build my own components, go for it with something like Pico CSS, like a small CSS library, or do Tailwind. But then I can't use Tailwind directly because I can't use chat CDN. So I need to re-implement the chat CDN components to have it look the same way and like there's just the easy, because I mean this is not a project I'm going to use for the coming years, so it's just a small hobby project. So I was going to go okay, just the lowest path of friction was to not use hdmx but just use, use.
Speaker 3:Use a react framework like v I'm using v now, which v with v0 and I just copy paste the component and it's ready to go. And it was really just like and this was the first time that that was the reason not to go for hdmi, because it was just the lowest part of friction. And I thought it was an interesting one, because if you don't have this generated for you with either v0 or chat cdn, actually the lowest part of friction to get started is hd mix. Yeah, because setting up a react framework is a whole lot of things.
Speaker 1:Well, yeah, you can just do uh. You can go uh mpx, start project or create React app, and then you have all the boilerplate as well.
Speaker 3:Yeah, but that is fully agreed. But like, for me that feels weird, like the boilerplate, like the create React app or feed, like it pulls in like 250 megabytes of dependencies and like it doesn't feel like a lightweight start, right, no, no, no.
Speaker 2:It's not a boilerplate it's like your whole project already.
Speaker 3:It's not a body plate.
Speaker 2:It's like your whole project already. It's just like you're just doing it.
Speaker 3:So, yeah, it's just an interesting experience.
Speaker 2:But then I guess, like if you were to use HTMX today, it would be if it's like a very small thing that maybe doesn't like. It's very custom and very small.
Speaker 3:Like you're not building like a whole website, it's just never use React. If you only have written backend code, html will feel much more natural, I think, much more easy to get started.
Speaker 2:But then at the same time, from what I understood, what you're saying is that there are less components that are out of the box that you can just use either.
Speaker 3:Yeah, it will be more basic HTML than you will need to build something like Tailwind or something like that, but like using react if you've never touched react.
Speaker 2:It's also quite a steep learning curve, true, true, but I do feel like that's where people are. I mean, I'm not as in-depth as either of you, but I do feel like most of the stuff is like react is the most popular. There are some other ones, but I feel like, uh, I feel like react is the most popular one. It takes us away. I feel like React is the most popular one. It is as well. Okay, now that that's out of the way. Are you still friends, by the way?
Speaker 1:Yeah, but if it works, it works, I mean.
Speaker 2:Okay, it's fine. After we can stop recording real quick and you can just tell the truth, it's okay.
Speaker 1:Why I use HTML Mixer? Because I just wanted to use something new, something else.
Speaker 3:Yeah, but I think, like the promise of it is super cool because I think everybody that builds that builds something either whatever, reactview, whatever. Like you have the feeling this is way too complex, like all the dependencies, users, the webpack, all the, all the translations from the from TSX to JSX we've built on top of, on top of, on top of, on top of, and we've built.
Speaker 1:How many times has the package manager already changed?
Speaker 3:Exactly exactly.
Speaker 1:I think every time there's a new one.
Speaker 2:Well, I think, even like Deno is like they play a lot with this, right, like even the marketing thing. They want to uncomplicate JavaScript and they make the joke about all the frameworks and all these things. I think it's duplicate javascript and they make the joke about all the frameworks and all these things. I think maybe a little side note, because you mentioned building things on top of things um, for the python user group, right, so we have the, the website, and I I wrote that in the belgian python user group, the belton belgian python user group.
Speaker 2:I can actually just put it quickly here, so there's like a little website that we, we put together, right, and this is actually built in Python, but it's actually a Python framework. That is Python, and then it gets transpiled to Nextjs. Yeah, it's like. It's like layers on top of layers on top of layers but it gets central transpired to javascript, basically, yeah, exactly right. So uh, actually, I just want to see if I can find it here. I think the name is called again.
Speaker 2:It was called pine code. Yeah, yeah, it's renamed because of the. Yeah, even the name of the repo is pineco website, but they renamed it to uh reflex yeah, yeah reflex.
Speaker 2:but uh, yeah, I just thought it was funny, like it's just, yeah, they, it's probably like a javascript developer that was like, let's just add another layer for python people, yes, and then they, uh, they put this together. Um, so, just a small, small side note. But since we're talking about different languages, I also thought maybe we can segue into the Octoverse. Ai leads Python to top language as the number of global developers surges. So basically, octoverse, as I understand, is like a report from GitHub that they see what's the most used programming languages and all these things, and JavaScript actually has been the first one for a long time. But this year, I think, was the first year that Python was ahead. I don't know, like this, it was a graph, just like this. What is it? Oh, I had it, but I think they also, I think it was actually for new repos actually. So they were saying that probably still, the usage is still bigger for, uh, javascript, but the amount of new repos that are in python is actually ahead would there be more javascript or more php?
Speaker 3:in uh, existing lines of code. You mean? I don't know.
Speaker 2:yeah, I don't know what it tracks, but I think it's like probably the same stats that you get on your repo, right? Yeah, top programming languages on GitHub Okay, interesting. So you see here, up until last year, javascript was there, but now Python went ahead, ranked by count of distinct users contributing to projects of each language.
Speaker 3:Okay, interesting. Yeah, so this is really the amount of users per year.
Speaker 2:Indeed, but, mickey, I thought, what is it? I thought I saw Most popular programming language is Python Beats out JavaScript as most popular language. Iac continues to grow with HCL, the HashiCorp and Shell. Typescript continues to grow strong as triple. I don't know, I don't remember where I saw it and what do we see here?
Speaker 3:So the top language this year is python, uh, overtaking your javascript, below javascript, typescript. I think people would argue that they fall on the same bucket, so together they would overclass python. That is true. That is true. The java c, sharp c plus plus php, and there is a php one.
Speaker 2:Yeah, php has been dropping since 2014.
Speaker 3:It was the third and now it's the seventh I think it's only uh at this size, still because of wordpress of clear.
Speaker 2:Yeah, I'm, I'll get the research and now that's level.
Speaker 1:Level uh has yeah, yeah, that's got some money, yeah, but laravel.
Speaker 3:I have the feeling I hear again more and more of laravel.
Speaker 1:Yeah, indeed that's a good point. So let's see next year if it maybe jumps up.
Speaker 2:Indeed, and then there's Shell, and then C and Go, and then Go, yeah, and then Go joined the top 10 in 2022, and now it's kept its steady position there.
Speaker 3:Is it because of Nico? I think so, I think so.
Speaker 2:You pushed it into the top 10. It's just that one extra. Uh, yeah, it was cool.
Speaker 3:I mean, yeah, any surprises here for you huh and maybe, but it's maybe the more the bubble that we're in I would expect to rest there somewhere. Yeah, probably the bubble that we're in.
Speaker 1:It's a bit sad but distinct users I mean distinct users.
Speaker 3:True, yeah, distinct active users. Yeah, but not just people that talk about it.
Speaker 2:Yeah, it's like the ones that use rust.
Speaker 1:They are fully on rust and not, not, not all the rewrites in.
Speaker 2:Uh yeah, all the rewrites uh, let's see what else state of gen ai. Number of public gener generative ai projects on github, and then now we're close to 150k and then?
Speaker 3:but what is that right?
Speaker 2:it's just a clone of another issue with 98 year over year growth from 2023, 2024. Yeah, so since last year it doubled. Basically that's what they're saying, right well, yiam, state of open source let's see one billion contributions, public and open source projects. Fifteen percent uh what's? What's why? Why spike in javascript package? You're over here, I guess. Yeah, jupiter notebook uses in search of mid ai python growth.
Speaker 3:Yeah no big surprises. It's crazy that javascript because they have another, another bucket for typescript. I would expect TypeScript to be a very strong grower.
Speaker 2:Yeah, that's true. Yeah, that's true. I think for most purposes I would bucket, like you said. I would probably put them together, but they didn't, so probably.
Speaker 1:Don't say that out loud, cut that out.
Speaker 3:These are the top 10 fastest growing languages.
Speaker 2:Yes, yes, we also have this here in 2024.
Speaker 2:So then, they have the yeah, they're taking up at the percentage growth contributors across all contributions on github. So the first one is python. So there are two. For people listening it's like a horizontal bar plot and then for each language there are two uh two bars basically, and the one in the smaller one is 2023 and the bigger one is 2024. So python is the fastest growing language in 2024 as well. Typescript is the second one right, and then right below the top five languages most commonly used in repos created within the last 12 months on github. Ah, this is actually javascript, so actually there are more, there are newer, so there are more contributions for python, but in terms of new projects, there are more javascript new projects than python projects. Does that surprise you?
Speaker 3:yes I think maybe a factor in that is also that, like, if you uh just the project that I was talking about, that like the chat, is actually uh, it's a go project, but there's a small front end in javascript so you have this effect that where there is a small front end component, you also have javascript on. Maybe that inflates the numbers a bit, so like, even if it's just a little bit of JavaScript.
Speaker 2:Yeah, because everything that has a UI is probably going to be some JavaScript, right, probably? Yeah, true, but I will remind you like, yeah, I was a bit surprised when I saw this because I thought this is the to be way more Python. But yeah.
Speaker 2:That could be it. They also mentioned here Rust continues. Oh where is this? Is Rust here? No, is Rust not even here? Rust continues to gain popularity for its safety, performance and productivity. Blah, blah, blah, blah, blah. Yeah. They also make the note here that Rust is the most admired language amongst developers.
Speaker 1:Maybe also why it's JavaScript, is because people are more. If you start programming, you'd rather create something that's visually um, yeah, visual, but basically yeah, it's true, rather than python, which is more like data oriented, or I oriented it yeah, I think, I agree, I think yeah that's a good point, like if you would.
Speaker 3:If you will search learning to program one-on-one, you probably get end of in these type of uh, let's, let's build a minimal interface like these type of true I also wonder if you have like.
Speaker 2:If you're building a toy project, you're probably building it to show people, and if you're going to show people, you want something a bit nicer than just a terminal, just a terminal, yeah, right, so so it's like maybe maybe it also attracts people to add a leave-in if you just a little bit of JavaScript right to display this stuff. Like Cheek has a lot of JavaScript now no.
Speaker 3:Cheek has some JavaScript. I think we use Alpinejs there For.
Speaker 2:For the front-end Maybe what is Cheek?
Speaker 1:Why Alpinejs and not just Vanillajs?
Speaker 3:Cheek is a hobby job scheduler. A very small job scheduler that you run on a single node environment when you can just say basically cron with a frontend. That's maybe how you should see it.
Speaker 2:You also described this to me one time. Con is too simple, airflow is too an overkill. Chick is tries to be a bit in between for small scale projects.
Speaker 3:Yeah, yeah and it's written in go and now has javascript and css and the question like why, why, why uh alpine js and not vanilla javascript? I? Mean today you can do a lot in Vanilla JavaScript. I think Alpinejs allows you to do a bit more closer to how you do reactive stuff in.
Speaker 1:React.
Speaker 3:Well, I've looked at it, but I'm honestly not super opinionated.
Speaker 1:Yeah, I've looked at it, but I don't find it that appealing to write JavaScript in your HTML tags. Yeah, Because I look at it briefly, but I'd rather split it out.
Speaker 3:But you can, and then you just refer to the function in the tag. Okay, all right, but this, to me, is also a way to play around with these libraries.
Speaker 2:Last thing that I want to bring up from the Octoverse that they're saying Dockerfile. They also noticed that there is almost exponential growth of Dockerfiles in GitHub projects and they're saying here what they're concluding from this is that the increase of HCL. So the HashiCorp what's that? Hashicorp? Something language, or is it HashiCorp language? What's that HCL stands for? No one knows configuration, something language, or is it hashi corp language?
Speaker 1:what's that hcl stands for?
Speaker 2:no one knows um configuration language hashi configuration language.
Speaker 2:Yeah, so basically this is for people that are sounds like it could be correct. Yeah, yeah, sounds. Yeah, if it's hallucination, it's okay, it sounds good enough. But uh, for people that don't know what it is, is basically what you use in terraform to define your infrastructure, right? So they're saying that, in the increase of popularity in HCL and Go, as well, as Dockerfile suggests that people are working more and more on cloud native applications. So I don't know, not sure if I'm surprised, not sure if it's true, but it's one of the takeaways that they put there.
Speaker 1:It's also more than uh just uh terraformer uh, yeah, yeah, yeah, yeah, they have packer.
Speaker 2:That's also in it package also uh probably probably uh their um console.
Speaker 1:It's also in hcl, so basically it's higher level um configuration language basically okay, so, but everything is related to infrastructure. Yeah, yeah. Infrastructure configuration.
Speaker 2:Then I think the conclusion is still valid, right, yeah?
Speaker 1:I just wanted to clarify.
Speaker 2:No, no, but that's good. I appreciate the clarification. Yeah, any big surprises here. Anything that you're looking at this, you're like whoa, where did this come from? No, no, no, of course. There's a lot of Gen AI there. I'll even have a topic on the Gen AI stuff, and one thing that was brought up was Minecraft, gen AI, minecraft. Have you heard about this part?
Speaker 3:No, I'm not sure. I've heard about the gen ai generated worlds.
Speaker 2:Yes, what is the what is this? Uh, what is this thing, uh, nico?
Speaker 1:well, basically, uh, oasis, that's basically a couple of weeks ago you also discussed uh doom, yeah basically, it's the same but for minecraft, yeah, okay cool and uh, this one differs a bit because you can play it in the browser, so you get a five minute session or a six minute session, I don't know I'm gonna try this while you're talking.
Speaker 2:I'm gonna try this for people following it's actually pretty trippy.
Speaker 1:It's like a bit like you're in a dream and and what gets generated like everything everything, everything. It's like it's the same as the Doom 1. So everything gets generated. So it's just a GeniI model.
Speaker 3:But the rendering? There is a rendering engine, but it's just no, no, no. The picture the picture.
Speaker 1:The images that you see are Just like the Doom 1.
Speaker 3:Okay.
Speaker 1:And so you'll see if we get it running the coherence, the time coherence. So from one frame to another, right it works. But, for example, if you're in a desert, you look at the ground and you look back up, you're teleported to somewhere else.
Speaker 3:Ah, yeah, okay Interesting interesting. Because it basically just tries to infer what is the next best frame, right, yeah, indeed.
Speaker 1:So it's really trippy. It's like you're in a dream. So, for example, you can stand still and break a block or place a block. That works, but once you, for example, go to grass, it keeps on generating grass and higher grass, and higher grass, and you would never come out of grass unless you look at the ground, look back up, and you're oh my god yeah, wow, that's interesting, or you can look at, like look at stone, and then you turn around and you're in a're in a dungeon.
Speaker 3:But that means that there is not much link to what happened in the past, because if you look at a blank screen. Basically, you look at the ground. It doesn't know that it was 10 frames ago.
Speaker 1:It was standing between the no, indeed, but it would make sense, right? Because when you're looking at stone, it's highly probable, if you only look at stone, it's highly probable that if you turn because when you're looking at stone, it's highly probable, if you only look at stone, it's highly probable that if you turn around, you're in a cave yeah, true, fully agree, but like, it's not highly probable if you have the context of the past, with no but that's why I said the the time the cohesion.
Speaker 2:Is not that, not that the temporal context it's cool or like one thing we're playing with. It is like you can kind of yeah, keep walking down to a cave or something, and then you like walk down for I don't know 10 minutes, and then you turn around and then it's like a beach and it's like I was just going out for what?
Speaker 1:But it's really like. It's kind of like a dream it tries to make sense.
Speaker 3:Wow, that's really cool, and we were looking at the images because you're in the queue. Yeah, i're in the queue is? Yeah, I'm in the queue much if we have time, but we're looking at some videos and it is surprisingly fluid, right?
Speaker 1:yeah, it's not right, it's like not sure, but still yeah, but it's, you have to know. It's impressive that this is rendered in a browser so um, I imagine if you run this locally on your laptop or something.
Speaker 3:But this looks impressive. What we're looking at now, yeah indeed yeah, but this is the gameplay, it must cost a huge amount of resources to run this.
Speaker 1:I'm not an expert, but probably.
Speaker 2:You can actually see the model weights in the code. So it's actually open source as well, that's really cool. You can also see what kind of model they're using and all these things, and it's nice, like you cannot like. Indeed, you can actually play, you can break blocks, you can do this. It's not just walking around as well, it's like, uh, an environment there I'm really wondering.
Speaker 3:I mean to me it's the same thing when we were looking at doom, like what does this mean for the future of games?
Speaker 1:yeah, yeah, because minecraft is already like uh how do you say it? Like, uh, progressively generated. Yeah, yeah, actually it's kind of static, because once you have the seat you can generate everything. But like, will this add an extra layer of?
Speaker 3:yeah. Generation explorability maybe? Yeah, indeed, like this is a no man's sky to the extreme.
Speaker 1:Yeah, indeed, because maybe it can invent new mobs or something based on the situation.
Speaker 2:Yeah, it's really Cool, it's really trippy and I mean, yeah, the performance part, I think, is also very interesting. Yeah, maybe you mentioned something that I hadn't planned. I had put, I think, a while ago. I don't think we talked about it, bart. Uh, by the way, the queue I was waiting, but I think it's gonna take a while still, so we'll just have to put the link on the show notes and people can try for themselves. Um, did we talk about this before? No, um, what is this? So basically, it's an mit technology review article that they talk exactly about this before. No, what is this? So basically, it's an MIT technology review article that they talk exactly about this how Gen AI could reinvent what it means to play. So they talk about, like, I think, what's the red something? The redemption? I forgot the name.
Speaker 1:Red Dead Redemption.
Speaker 2:Red Dead Redemption. So the guy he's basically saying like, yeah, now I'm playing these games and sometimes you see these non-playable characters and you see him walk around and it's fun to kind of follow and see what it does. But at some point you kind of get repetitive right and then he starts to to kind of do a deep dive on what could gen ai do, uh for, for for gaming in general right.
Speaker 1:Um well, it's a bit like we live. It's repetitive you go to work, you come back home.
Speaker 2:You go to work, you go and then one day you die, okay.
Speaker 2:So I mean, all right, thanks everyone, I'll see you next week no, but um, because what they're also saying is that, uh, they could maybe have these non-playable characters, they could add some jni on top of it, so they add some uh, unpredictability of things, but then they're also I mean, they kind of I think there are some companies that that do this and I think the interview.
Speaker 2:So I read this article a while ago, so I don't remember everything that they go into but, um, they also question if this is a good idea, because there was also games. I think that I forgot the name of the game, but there was like something about space exploration and then it was programmatically generated. So you can this, you can explore indefinitely as many worlds as you can, because they're like generated in the game. But then they also said that this was a bit of a letdown, because it felt a bit there was no like storyline. If they said like I don't know, I remember that they mentioned that the outcome in the end for people that were playing it wasn't really as it didn't add much to the game. It was more of a disappointment than something. But it.
Speaker 3:It's also like Darius saying, but like we use Gen EI to get to a Gen EI generated storyline, which is maybe more complex than just having an adding a bit of let's say, quote unquote intelligence to an NPC.
Speaker 2:Yeah, that's true. Well, I do think there is a, indeed it's a spectrum, right, like you can try to say jenny is going to do my whole game for me. Or you can just say jenny is going to have a personality for this, this non-playable character or for that thing or for this thing.
Speaker 3:Right, let's say, in the world of warcraft you have an npc and you uh add some sassiness to his uh character. Yeah, like that generates like a way to have this a bit more organic feeling to interactions. Yeah, without starting from scratch, right? Without, it's a little still clear. I think you can do stuff with it.
Speaker 2:I was also thinking that, depending on what it is, this could also be interesting. Like hallucinations is not as much of a problem, maybe.
Speaker 3:Well, if it interrupts the gameplay, it does Like. If it interrupts the storyline, it does. That's a bit the. So I'm just thinking like you want to have a captivating experience.
Speaker 2:This player depends a bit, of course, a bit on the game. But yeah, that's true. But do you think, yeah, because I was just wondering, like for the npcs, right, uh, the red, whatever, redemption, right, if you go and start talking to the guy, then you start having this very unpredictable conversation. Is there something there that could happen in terms of the conversation there? Like, do you think there's an issue of hallucination or do you think that game makers, they can just kind of use jenny I more freely in this context?
Speaker 1:I think maybe what, not what could be nice is that it remembers what you, you did and but certain games already do that like Like, if you, for example, steal somewhere in a bank or something, then it remembers, but maybe a bit more extreme, that it can also more naturally react to that maybe yeah, I mean it could be, I think, also the memory thing. Yeah, yeah, yeah.
Speaker 2:I think the memory thing is also be something a bit trickier right? Because I think if you play for a long time and you have to remember what was generated and what was the context and like, yeah, but what if you have?
Speaker 1:like you can easily look, uh, each action in the game, like, okay, he stole there or whatever, and you can pass that into the, let's say, prompt of the of the guy and you say, okay, uh, he yesterday stole a bank and he's a bank teller.
Speaker 3:He maybe reacts differently ah, yeah somebody, your uh, your friend that also helped you uh rob the bank yeah, that's true, yeah, that's true, that's true, that's true, that's true, that's cool yeah, look, there's some, there's a, there's some, there's a playing room there and I think, like if you ignore the, the computer that's necessary for it, that then you can do a lot already to do with a lambsa, yeah, yeah, true, like with very limited effort you can can do a lot already to do with LLM. Yeah, yeah, true, like with very limited effort you can already do a lot of these things.
Speaker 2:Oof Say Nico this is your moment. No, no, no.
Speaker 1:This is your moment. Yeah, Sometimes I think we, yeah, Grab too too much or too too quickly to another LLM to solve a task.
Speaker 3:Well, I think, think that, but I like.
Speaker 1:That's why I'm saying ignore the compute, but like you, can use lms for a lot of things without, without a lot of effort, right, yeah, but I can, for example, give you but you can make probably something better and more performant yeah, but for example, at the client, somewhere, somewhere, uh, my namey names, no, no, no.
Speaker 1:so, uh, we have to basically classify, uh, yeah, a transcript, and we do it in the three national language, in three languages, and so dutch, uh, french and english, and uh, we basically give a transcript as the lmM, which, which, um, which language is it, yeah, like, whereas you can just have like a couple of keywords that you search and you have it Like it's literally probably 10,000 times faster and more efficient to do it that way, but because it's so easy to just uh send it to LLM and we get something back that people just do that yeah, I agree.
Speaker 3:I agree with what you're saying it's difficult because, like we just say, the approach you take, keyword search or whatever, like it's way more computer efficient yeah, more explainable as well, at the other end, like you have this black box API which you can send an instruction and it's probably going to give the right answer, but you don't need to think about it too much. Just in natural language, say give me back the language.
Speaker 3:It's this it's this balance, like there's also something to say, like there's some efficiency in developing in such a way with something that probably just gives back the right answer yeah, developer, yeah, developer, yeah. But I fully agree that it's much more performing and probably cleaner solution to do.
Speaker 1:Sometimes you're maybe a bit more critical about these things than just ask it yeah, because then I think we're going in the wrong direction. I think there's a lot of good applications to it, but also, I mean Because MPCs have a bit of a parallel example to the MPC thing.
Speaker 3:It's a while ago now, I think a year ago, I tried with uh uh, tpt four uh back then to like you have a player uh on a 2d uh uh area and a player, let's say sort of a Pac-Man, that needs to fetch apples, and if you find the apple, you eat the apple, you get points. Typically you do this with a. So I implemented with a more traditional reinforcement learning model. Deep q uh reinforcement learning takes thousands of iterations until you have something that is performant. I did. I took the same, exactly the same thing. I replaced back into an lm which is horribly inefficient computer-wise. I fully agree with that. But you just like, for every choice that the, that the, the player needs to take, just send the environment to the lm and you ask the lm what should be the next best uh action to take based on that these. This is what I want to achieve and it was, from the get-go, at least as good as the 1000 iterations.
Speaker 1:Yeah, I can imagine.
Speaker 3:And that is a bit like what you're saying Having a trained good model for that specific for that is probably better long-term Because you can let it evolve. Once it's trained, you can basically just… Exactly exactly, yeah, but the LLM is super easy to get going and I think that is the challenge like that's because that, because of that it's, people don't even think about it anymore, like maybe there's an efficient way to do it, lms.
Speaker 2:Lms is looking a lot like a hammer these days. Right, it's like.
Speaker 1:So we just use it everywhere yeah, maybe that will become a new branch, like you have finops but a fin gen or something like optimizing this in a couple of years.
Speaker 2:Yeah, yeah, I fully agree yeah, but I yeah, I mean I know what you're saying. Yeah, I get the feeling as well, because even for more traditional nlp tasks right, stuff that like sentiment analysis or nr um I mean there were models that did good enough, like it wasn't like this is because I still think that reinforcement learning what you're or NER I mean there were models that did good enough, like it wasn't like this is because I still think that reinforcement learning what you're saying I can still understand that it's probably a difficult machine learning task, right, because even reinforcement learning you have to do a lot of iterations. Sometimes if you don't constrain things right, maybe it just explodes the grains. But even for the simple things that there were good models, there was like a well-worn path. You started to realize that I think we did this for NER, so name entity recognition that someone spent some time, like a week, trying to prototype something and someone was just like oh, let's just ask Chagipi, let's just see how it goes.
Speaker 2:And it was just like much better, like much, much, much better, which also is like makes you, makes you. I mean I completely get it and I think for the very simple use cases I would stand with you Like I wouldn't ask, kind of them, just to classify between three languages Part JSON, part JSON yeah.
Speaker 2:But in the end it's kind of like, it's kind of like the hope, like I don't remember who I think maybe it was you there was like someone was going dict equals to eval, json string, you know, and json string, you know, and it's like, yeah, it works right, but that doesn't mean that it's the best way to go about it right. So I get you, I get your point, I get your point, but uh, I think it would take some time before we we bounce back a bit from it, right, because actually I heard it on another podcast like nothing, no, yeah, nothing is as long term as a quick fix. That worked right, and I think that's true. It's like people are like, oh, let's just see if chadji pt can work, you know, and then it works, nothing is as long term as a quick fix that worked right and I think that's true.
Speaker 2:It's like people are like, oh, let's just see if GFD can work, you know. And then it works and it's like, okay, why would I give you more time and money to spend on something else that just works? So I think that's also those two things combined that we see this inflation, llm stuff.
Speaker 1:In the end, we're just giving our money to Nvidia.
Speaker 2:Yeah.
Speaker 1:All these cloud companies anyway, yeah, that's true, they'll be happy yeah.
Speaker 2:Someone is happy. You mentioned BART GPT-4. Gpt-4. That's when you did the Pac-Man stuff. Yeah, do you think it would have been better with O1?
Speaker 1:What's the O1 one is that with the reasoning?
Speaker 2:yes, with reasoning it would probably have been better, but way slower yeah maybe, uh, maybe, just it's a reasoning, and I I hate how much we anthropomorphize. I'm pronouncing right, like ai, because I was even talking to. I'm talking to two other like some people play futsal with and the guy he did a degree in math, like mathematics, so like he's well equipped to understand the, the mechanisms, right, maybe not programming, but like he could. And then like they're talking, yeah, but this thing is not thinking. It's like no, no, but now the one model, this is thinking. It even says like, thinking, thinking, thinking, thinking.
Speaker 2:And I was like, ah, man, but like you know, it's like when you say reasoning and then people see it on the UI, it says thinking, you know, and like it's not even that they are not capable of understanding, but it's just there on your face, right, like if you're not critically stopping saying like is it says thinking, it's just outputting something and using that as input for the next, but it's always just predicting the next word and it's just a mathematical thing and this and that like I think also people don't want to open that box, right, it's easy to just think it's a little person in there in the computer that is thinking and you just need some time, right but uh, like the mechanical turk yeah exactly, but uh, but yeah but so what's the difference between the the normal gpt4 and one?
Speaker 1:is it just reprompting itself, or is there some added layer to it?
Speaker 2:so, it's well, I'll, I'll spit something out and then, bart, you can correct me. Yeah, so, uh, I think in essence. So it's like the actual the in-between things. It's just like they have some instructions to break the problem down and just say, okay, describe what you see, describe this, describe the text, and that becomes the input for the next time. So, basically, the JGPT just reprompts itself a few times evaluating itself in a way yeah it's like two models working together.
Speaker 2:We don't know actually right because it's open ai and they don't. They're not open, right? Uh, despite the name. So we don't know, but they. We also think that they actually mentioned on the blog post as well that during the training phase they also embedded this, this like in per step kind of evaluation, right. So if the first step in the reason is wrong, they also have some like, uh, some training, back propagation, whatever right to to correct that. So it's not just, it's not just like the plain chat gpt that just changed the ui a bit and they said this is a new product, there was a bit more work into it, but I think in essence it was, uh, it's the, the different way of computing things. No, you can uh what how how was I?
Speaker 3:no, no, I agree. It's also. I understand, like I think it's mainly reprompting. Like you ask a question and instead of spurting out immediately an answer, there's like this step, like are you sure about this? And then there's another, another answer, and then did you check out this way or that way, or is this, is this phrase in the right context, based on the person that is asking the question? So you have a number of these steps in between that quote unquote, enrich the answer before giving it back, and then, indeed, that GPT-4.0 was fine-tuned with, indeed, data that does this.
Speaker 2:Yeah, and I remember I think you told me the first time that there was this thing called like self-reflection, that basically if a GPT model says something and you ask like, did you hallucinate on the previous answer, you could actually tell if you had hallucinated, Like, and I think yeah, then if you empirically you validate this, assuming that that's true, something like this chain of reasoning makes a lot of sense why the output would be much better right.
Speaker 2:Because if you can always evaluate the previous step, they just say, okay, just say five things before you give me the answer and that's it. So I mean, yeah, it's more powerful, they say, but the people's experience, from what I gathered, is also it takes longer, right? So you wouldn't use it as a chat completion on vs code?
Speaker 1:yeah, it's a bit like the entropic thing, like the the web ui, the one that controls your pc ah yeah, yeah, the computer right, yeah, topic, yeah, that they had it's nice, yeah, but it takes a long time each step.
Speaker 2:Yeah, I can imagine it takes a picture.
Speaker 1:Yeah, then it thinks about the picture, the next action, and then, okay, it gives a button yeah, and then it gives another image yeah, yeah.
Speaker 2:And then sometimes it just goes off like if it takes one wrong path and you're yeah, I yeah and I heard.
Speaker 1:It's also very expensive because you're always a lot of prompts and a lot of tokens indeed, but yeah, you know, like two years ago, we were practically nowhere with this yeah, that's true, and so I can't yeah I can't really imagine how it will be in two years from now, right?
Speaker 2:yeah, to be seen these things are moving so fast. But, uh, the reason I also wanted to bring up, because you tried, oh, one preview mini or no, mini or no both. Apparently that was the oh one. The actual oh one leaked. I'm not a preview, you mean not the preview.
Speaker 3:Okay, this is on the showdowns and I was thinking, but oh, one is already there. But that is, that is released already preview is released.
Speaker 2:But this is not the actual uh model was leaked, so that's what I saw. So this is from november 4th and uh, basically, the way that he leaked is that they, if they clicked, let's see if they can they show it here. I think. Let me show you somewhere. Um, basically, if you clicked on the, see more maybe on the on the tweet.
Speaker 2:No, this is just a tweet, but where is it? I read it, I saw it here. Basically, if you changing the parameter in the URL URL, so basically whenever you click to preview on the URL, it was like chatgpt forward slash, blah, blah, preview. And if you just remove the preview part, so you just put like chatptcom forward, slash question mark model 01, okay, it worked, people could just go. So one person, like some people, put it on uh, on x, right, and a lot of people went there to check. You never know, right, if it's actually a one, because open ai didn't say that this is a one, but they did compare the performance and they did conclude that it is probably a version of a one. I mean, maybe it's going to improve as well right.
Speaker 3:What is the big difference?
Speaker 2:just that it can reason over images yeah, so that's the thing I don't know. Well, I was looking as well. I saw some videos, every example that I saw that they're trying to show how powerful it is. They were doing reasoning on images, so, and maybe they're awful this leak, is you mean?
Speaker 3:yeah from this leakage, because what I understand and I haven't tested this very recently, but when the o1 preview was released, it didn't support tools, and tools was interpretation of images, but also like executing python code for calculation or stuff like this. It didn't support us. I think it still doesn't. Yeah, maybe this, the release, will actually support the tools again maybe maybe indeed so maybe also to put this on the screen.
Speaker 2:Um, this is a video for someone that is trying something apparently between the two, I guess the oh one and uh, this is the four oh, but um, yeah, I the the examples that I saw, like they show the image on the preview one or the mini. Let me see describe this image. Maybe a better example, since if I'm talking about this is this one here. Okay, but so it supports images, basically Supports images and apparently has very good performance, right and?
Speaker 3:it interprets them better as GPT-4. That's what I get from what you're showing on the screen.
Speaker 2:Yeah, and actually the 40 and the 01 preview and the 01 mini. So I thought the one youtube video. The guy was like there's a picture of a construction workers on a on a like old school, right on a beam and then it says how many people are there and then they're basically all getting the the answer wrong, except for this 01. But the one didn't even just give how many people there were on the beam. They also said this is probably a new picture from this and this it's probably this location. This is black and white. The construction workers are in this. So it seemed very impressive, right, but I still I think there are use cases for 01, but in general, like 01 mini preview, whatever, but I haven't, I'm not sure exactly when you would like you have to use it, like because you do have a cost on time, right, you have to wait. So everything that you need, a fast response, like code completion or anything you probably don't want.
Speaker 3:So, if I just talk about my own usage, is that I tend to enable it when I need to generate text Like text with a certain instruction set, like for this type of, with this tone of voice, with that uh for that uh type of like is it for a post, is it for an article, is it for a? And and like rework, like some, some comments, and when I have like a very specific set of instructions to rework some text to something else, I have the feeling it's hard to make an objective that the 01 preview works better than GPT-4.
Speaker 2:So but yeah, I see what you're saying, but it's basically when you're going to make a post and you can afford the wait, basically.
Speaker 3:I can afford. Yeah, just I think most people that just use the UI can afford to wait.
Speaker 2:Yeah, that's true. Yeah, yeah, that's true. Yeah, yeah, that's true, I agree with you. I guess for me it's just like I never had any. Yeah, but I guess what you're saying it's very, a bit of subjective, right? I don't think of a clear example like oh and this, I definitely need the chain of reasoning for the stuff that I do, at least, even if it's the ui, I probably well, I'm not creating as much content, let's say. But even if I do create UI, I probably well, I'm not creating as much content, let's say, but even if I do create content, I'm probably going to read and edit, right.
Speaker 3:Are you telling me I'm lazy? I mean.
Speaker 2:I'm not saying nothing, but you know. No, but I'm thinking it's just like I'd rather get a fast answer and then I'll read and edit, because I think you need to read and edit anyways. I don't think like 50 milliseconds and 100 milliseconds are going to change your, but is it? Is it?
Speaker 3:50 milliseconds, 100 milliseconds versus a second. But is it? Is it a second?
Speaker 2:is it two seconds, something like that, because what I saw, seconds it's not minutes, but it's like 30 seconds no, no, no okay, that's a big prompt then, because I saw the guy with the image, he.
Speaker 2:He said it took 18 seconds for the old one, I, but maybe I'm right. Yeah, yeah, I mean I know what you're saying, yeah, but uh, okay, yeah, but indeed, if it's, if it's like one, two seconds, it's fine, because I also feel like I have a very short attention span. So I feel like if something's more than 30 seconds, then I'm very tempted to just check on something else real quick, but then it's like I'm switching context and then like it's five minutes later when I'm back.
Speaker 3:Um, I have the feeling that this year that is not really the problem of the it's the problem between the keyboard and the chair.
Speaker 2:Yeah, yeah, for sure, but uh, yeah, maybe I'm a bit uh, a bit picky with these things, but yeah. So I don't know when it's going to be released the 01, but apparently some people tried it, they verified that the image at least the image understanding is very, very good. But are you excited for this part or no?
Speaker 3:I don't see any.
Speaker 2:I don't do a lot of image interpretations, but like just 01 in general, something like what you use for 01 today, a better 01.
Speaker 3:I don't know it doesn't like to. To me it hasn't been as drastic as previous.
Speaker 2:Yeah, like 01 was like to me, very like it's a small change versus 4.0 yeah, I would be looking forward to GPT-5, though I think I'm expecting a big change there. Right like they've been very careful as well not to call anything 5, because even the 4-0. Let's see. So I'll be more excited when I think we hear about GPT-5. But that's just me. What else do we have here? Tiger Beetle, tiger Style, tiger Style dst yes docs tiger stylemd. What is this about, nico?
Speaker 1:all right, so it can be a bit of a long story, but so I um, I really like testing. Maybe not not everybody, but I really like testing my code and failing as fast as possible like unit testing.
Speaker 1:You're talking about that yeah, you're testing integration testing and doing testing. Okay, anything, anything, um, I like to do it. I like to do it on dev environments and stuff like that. I like to do it as complete as possible, so the full chain, basically, I always try to test and so I always try to improve my testing and the way I write code, and I stumbled upon this. It's basically do you know, tiger Beetle?
Speaker 3:No.
Speaker 1:Okay, so Tiger Beetle is a database for the trans I'm maybe skipping over some details because it's very in-depth, but it's a database for transactional workloads, so basically banks. And so I also linked a YouTube video about the creator or one of the creators. Uh, I think his name is johan joram, uh, I can't remember out of my head. So basically, uh, he does a full talk about the design philosophy, yes, um, and so at the design philosophy that he has invented this tiger style and it's basically based on some design principles of NASA. Okay, and next to that, he also talks about how he tests this. And he tests this with DST, which means for deterministic simulation, testing. And I'll get a bit.
Speaker 1:I'll briefly summarize the talk. So he starts about, like, okay, these transactional databases that banks used, they're all built around Postgres or MySQL or databases you get. So you have Postgres and you build basically a transactional layer around it. Okay, and one of the examples that he shows why it is very inefficient, because for one transaction you need 10 escalator queries. Okay, and then he wants to basically improve the performance on this, because one of the statistics that he gets is that in India, one of the banks did 12 billion transactions in a month. One of the banks did 12 billion transactions in a month, and so the amount of transactions is always going up Because, for example, now electricity, you also maybe need transacting on electricity and you maybe want to sell your electricity or buy electricity. It's going up anyway.
Speaker 3:So you want to basically make so not only monetary transactions, but going to be energy yeah, energy transactions.
Speaker 1:So then he's building basically a new database, and he found out and Tiger Beetle is that transactional database?
Speaker 1:Yes, indeed. So basically he says, like you only need two methods, basically you need debit and credit, okay, and he optimizes everything behind the scenes. Okay, he optimizes everything behind the scenes. And then one of the design principles that they created to do this is TigerStyle. So why? Because all this financial, this layer with Postgres, has been battle tested for 30 years. So how can you battle test something that has been in the making for two or three years now, I think? For this amount of time and giving the confidence that you have all the bugs and whatever, Because this is mission critical, right?
Speaker 3:Yes, indeed, this can't go wrong.
Speaker 1:So then they designed this, and one of the things is fail fast as well. So what it's called is, like people program program in the positive space, I think they call it. What does the programming to do? But not in the negative space? Where can it fail? So?
Speaker 1:basically what they do is they would assert everywhere. So you assert your input of your function and you assert your output, and basically what happens then is that where you expect something to fail, it fails at that point Because, for example, you might parse some JSON into an object and use that object somewhere. 10 calls deeper and you try to access the fields, but it doesn't exist. Or it's null. Then it fails very far from your Searching.
Speaker 3:Yeah, or it's null, then it fails very far from your, it's so true, yeah, yeah, yeah.
Speaker 1:And there's all other design principles, for example static allocation of all the memory, and it's very, very detailed and basically combined with that, combined with the deterministic simulation testing which basically there, he basically mocks everything or simulates everything. He simulates disk failures, network failures, everything, everything they basically mock. They can also increase speed and time, they can fast forward time. So basically what they can do is run a simulation and every two days they test 10 years of simulated data. Oh, wow, and because it's deterministic, so they generate a seed.
Speaker 2:Yeah.
Speaker 1:And when there's a failure, total Magic captures it, posts an issue on their GitHub with that seed and they can just replay it, oh, and they can see where it went wrong. And next to that they also, with the simulation, they built a game on top of it. So they basically compiled Tiger Beetle to Wasm, put some game on top of it and you really see, like, because it's distributed, you see like six or seven nodes that play together. And the first level is very easy, everything goes well. Then the second level, they inject network. Everything goes well. Then the second level, they inject network failures or latencies or memory corruption and you really see, like gamified, the it's just basically this test simulation, well, this test on simulated data, but then visualized.
Speaker 1:Yes, but yeah, this is just for presenting, basically. Okay, I see it's a fun way, but yeah, just for presenting basically Okay, I see. It's a fun way. They use Zish so it easily compiles to Wasm. They built a small UI framework on top of it and you literally see all the nodes and then everything fails and you really see the consensus algorithm running like who is going to be the new leader, and stuff like that, and you can also add failures in the middle. It's really fine.
Speaker 3:Is Tiger Beetle also implemented in Zig?
Speaker 1:Yeah, so there they have the choice between. They say it's rust between Zig, but in Zig you really have control over all the memory allocations. That's something that you really wanted, because one of the design principles is that you can statically allocate all the memory up front and you don't have any free or malloc or whatever in in your code.
Speaker 3:Um yeah, and his testing styles and really like make sure to cover everything that could go wrong instead of focusing on what am I trying to do and does it go correctly? Is that a correct?
Speaker 1:well, it's a bit of both, but basically what he said he says like I want to. I want to set trip wires in the code. So that when I test it with 10 years, it, when I test it with 10 years, it fails, and I just fix it.
Speaker 3:Yeah.
Speaker 1:And also it says, like one of his colleagues accidentally sets tripwires, so accidentally sets failures, so that they can yeah, they keep themselves on top of the game.
Speaker 3:Yeah, that's interesting Because when I I'm just talking for myself, when I build tests, I typically do this to say, okay, I wanted to do this, so that's a server that can do this. It's typically how I do it. And then I got to 70% coverage and then I see which lines do I not have covered yet and I built coverage for the next time. But it's not with the idea of what could go wrong. And let's simulate what could go wrong.
Speaker 1:Yeah, but here you assert inside the compiled code. You don't assert in your testing code, which is pretty normal, but here you reassert in your test.
Speaker 2:Yeah, but, like for me, I just don't write bugs and then you don't need to test anything like I just I just think it's just easier.
Speaker 1:So yeah, there's a talk of that also on the primogen stream no one reacts, hold on hold on hold on hold on do we have a? It's a classic joke that you already hit like 20 times there's a. I don't need y'all okay, if you just look up Tiger Beetle, tiger Style Program, there's like a couple of talks very interesting if you're interested in the topic.
Speaker 3:I'll link it in the show notes. It's an interesting.
Speaker 2:This is cool. Never heard of this. Yeah, me neither, but it looks really big, huh.
Speaker 1:That's also why, for example, I really like to try out new languages, not because it's fun, but it teaches you different design philosophies.
Speaker 2:Yeah, I was thinking in the htmx, when you're discussing htmx, how like sometimes when you go to a new way of thinking, it's uh, there's like growing pains, I guess. But I also think that too, when there's like to to kind of see from things from a different perspective, it also adds you as a like a problem solver, really. You know, like, even if you know you're programming this in this language and there's not. That also, I think it maybe adds a vocabulary for you to try to solve things this way, you know.
Speaker 1:So it's really like about getting good ideas from different places yeah, so for me, for especially to go, you have the concurrency model. Uh, I played around with that and then, okay, on the job, I mainly use python, but I basically re-implemented a bit the concurrency model and all the weight groups and the channel stuff also in Python, because it's just easy. The concept makes sense, the concept indeed.
Speaker 2:No, this is really cool. This is really cool. Yeah, no, it's very interesting as well. I like the whole experimentation stuff and like trying things out and gamifying these things. I think it'll be really cool. Maybe a question In machine learning, ai, all these things.
Speaker 2:I don't see as many people writing tests. I'm not sure if it's just like a bubble that I'm in, but a lot of the times when I'm working on projects, I'm the first person to say, oh yeah, we should write tests for these things. I wish to do this and I also understand, like why, yeah, we're writing something. We're trying to assert that something is going well, not something that something's going wrong, right. But I also like tests because, depending on the test, if it's well designed and all these things, it's it also makes it easy to understand what the function is doing. If this is what I have as an input and this is what I have as an output, it's very clear to see. Okay, this function is doing this.
Speaker 2:So I feel like there are many benefits, but I still feel like people don't, at least in data science projects, people don't invest the time and I think my theory is that because a lot of times, these things people are working by themselves, because it's like a POC or something, so you're a one man team. So, and maybe because people think that by not writing tests you're going to move faster, which may be true in the beginning, but I also think, as soon as you get to a point that you need to refactor something, you need to change something, then I think not having tests doesn't give you the confidence to make changes and make sure that everything still works. Is it just my experience or do you think it's about people not testing as much for machine learning projects?
Speaker 3:I think that is true, you think it's it's true.
Speaker 2:okay, I think it's a trend they were seeing, because sometimes I look at it, I'm like writing tests and I feel like sometimes it's from moment it's more traditional software engineering project with no tests, everybody will immediately question why there are no tests.
Speaker 3:Yeah, but if it's a machine learning project with no tests, you need to have someone opinionated about tests to say, but why don't we write a test? Yeah, I think for me. I think it's a bit of a difference. I think the typical machine learning engineer has a very experimental mindset yeah, and like, let's try something and it's not really thinking about how do we build tests.
Speaker 2:But for data engineering stuff? Do you see that as well, or do you already see?
Speaker 3:because data engineering is more software engineering oriented, I guess I think for data engineering is much more to them to default yeah, but do you see?
Speaker 2:so I guess if I go to a, if I walk into a data engineering team, they're building a project. I look at the repo. They're probably gonna have tests.
Speaker 1:I hope so yeah, but maybe it's a bit, a bit more difficult to write this right, because normally a test yeah, you write it's yeah at a high level. Let's say that if you change something in the code it should not break your test, right because otherwise what's the? What are you testing?
Speaker 1:you have to test behavior whatever yeah, uh, but yeah, if you, for example, add a column to your database, you have to change your test. If you change database, you have to change your test. If you change a filter, you have to change your test. So basically, you're basically always chasing what you basically already expect.
Speaker 2:But can you write a test that just checks, like not like just checks at this column? I don't know Like you write a function instead of just passing all the data, you just specify the data that you need to compute the new column and then, if in the actual data set there's another column, you just Isn't there a way to work around these things? Yeah, the data changes, I agree, but isn't there a way to work around where your functions only care about the stuff that you need to compute.
Speaker 3:I think what the point Nico makes is a very fair one, because you're in the experimentation phase. You're always chasing what you're doing with the tests. But I think what and it depends again, what type of model are you building? But let's maybe take the example of LLMs. Okay, I think there are very strong arguments to make that you should test the outcome. I think the difficult thing of that is that it's typically not a deterministic outcome that you have in a software engineering project, but it's a probabilistic outcome. So if I ask an LLM in a rack-based system like, how old is Marilo, how old are you, marilo? 29. 29. Then I'm… 12. Like in a deterministic system, like, the outcome should probably be the numbers two and nine, 29,. Right, yeah, yeah, yeah, yeah, I see what you're saying. Like, with an LM like the, the, the challenge of that is that, like, the answer can be in natural language, written out, 29. It also can be Merilo this year to turn 29, or in numbers 29.
Speaker 3:Merilo was born in and they're all okay right they're all okay based on the prompt, of course, but like that, that approach requires that you test this in a probabilistic manner, which is possible, but, like the, there are very little standards for that today, so you need to be very opinionated on how you do that well, you ask an LLM.
Speaker 1:Is this correct? We expect 29. Is the output of this LLM correct, that is?
Speaker 3:a way to do it, but, like there, you need to have someone that is opinionated on how do you do testing in a probabilistic manner, to set something like that up, yeah, um.
Speaker 2:Yeah, I agree. I think the thing for me is also that this is just a part of your solution, right, and yeah, for that part is a bit harder. But I think for data science, like feature engineering, there's a lot of your solution, right, and yeah, for that part is a bit hard. But I think for data science, like feature engineering, there's a lot of stuff that is deterministic that you could just write some tests, but I think you kind of-.
Speaker 3:But I agree there are also like I agree that it needs to happen. But I also understand, like Nico's point, like you're building features, okay, tomorrow you're still going to say, yeah, this, I'm going to change the definition, or tomorrow I'm going to, and you're always like changing the what is the last state of the experiment with?
Speaker 1:you're chasing it with your, your test suite and you, in the end, you already chased it and you already validated that you have an extra column with the correct, so why would you actually add a test? That's probably nine times out of 10. The next thing you're going to change a feature or whatever will break yeah.
Speaker 3:And break, yeah, and you always have to change your code and your test. And that makes that like test driven design, where you say I want to test first and then I'm going to write the functionality a lot harder, like you can still do, say, okay, this model, this feature, set as far as final, let's build a test suite now. Then you're a bit out of that experimentation phase.
Speaker 2:But yeah, no, but I never. Well, I I like to, I try to test stuff. I'm not going to say that I do it every project, because sometimes, indeed, if it's something very experimental that you don't know exactly, so sometimes you didn't write books.
Speaker 2:No, I didn't, it's just for demonstration, it's for documentation part. You know, um, but uh, I forgot what I was gonna say now, uh, yeah, I think it's like as soon as you know what you want to do, then I think it's the point to start writing tests, right, I think in the data science project, in my opinionated view, is also the moment you want to move away from notebooks, right, like you're ready to do exploration. I think notebooks are also nice to share to people Like, oh, look, I plotted this, I get this example. It's almost like you read us a report and as soon as you know what you want to do, move a bit away, start writing tests, start doing these things. But I still feel like sometimes when I get in the room and I was like, yeah, I'm going to write some tests because of A, b and C, I feel that some people they're not sure what I mean or how to write tests or how does it all work, but no one wants to say anything because they feel like they should have known by now and it's always a bit of that weird, you know, like tension I feel.
Speaker 2:Um, in the end, I just write tests for my code and the other code is not tested. Yeah, that's, that's kind of what happens. But then when I touch on someone else's code, then I also feel like, ah, if I need to refactor something, I'm not confident that I can just change these things. Um, maybe a small plug to this I learned recently and maybe I should have known before, but I learned recently that you can actually mark tests in PyTest. Because one thing I was also doing is this transforming data, and I was actually reading data from Azure. But I was like, yeah, I don't think it makes sense to write tests that read data from Azure, because if you're on NCI, blah, blah, blah. So but then apparently it's very easy to just mark tests in pytest and just say this is slow test, and then you can also change the configuration to say, whenever I run pytest, don't run the slow tests and then you can just do that, so something that is native in a pytest, or is it, like a native, an add-on?
Speaker 2:native. It's just extensions at pytest dotparameterizedmark parentheses the tag you want to put, and then you do pytest-m, whatever the tag you want it.
Speaker 3:So that basically means that you can give a certain test a tag and you can say run this one or not, exactly. Okay, to be a bit more selective. Instead of saying these test files, you can really say these individual tests, exactly. So in the in the same file.
Speaker 2:You can have three that have that require, uh, azure credentials. You have three that are very slow, that you don't want to run on stone pre-push on pre-commit or whatever um the language classifiers yes, exactly, but yeah, but it's something that I learned.
Speaker 2:I was like, yeah, this is pretty handy as well, so now I can have everything there and testing, so I like that. And the other thing I wanted to mention as well you were talking about, uh, testing for for llms and um it was. It was brought up to me by different people actually that deepai, they have a lot of these short courses now, so deeplearningai the creator is the same guy from Coursera, so it's basically like free courses online. They actually have quite a lot of stuff.
Speaker 3:So it's really cool. Oh, you didn't know that it was from Coursera.
Speaker 2:The same creator of Coursera. Okay, yeah, yeah. So this is the landing page. So they have quite a lot of courses. You explore courses and they have now a short course which is like from the tagline was a bit cliche. I didn't even check what's the tagline.
Speaker 3:AI is the new electricity. You are the spark. You read this and you think I'm going to use this.
Speaker 2:You're like I'm the spark. I felt they were talking to me personally. They were like I'm the spark, but they have short courses, which is basically like an hour and a half right? Yeah, I've heard good things about this.
Speaker 2:Yeah, so actually this one was also an interesting one. I may try, but I also think for me, because I have a very short attention span, I feel like it's good to have something short as well that I can accomplish, but what I wanted to share. So we discussed a bit about testing, about LLMs, and I had some thoughts about it, I think similar conclusions to what you did, but then I saw this short course as well, which is actually 52 minutes.
Speaker 3:This is a course that's called Automated Testing for LLM.
Speaker 2:Ops From CircleCI, right. So CircleCI is like a CI provider, ci-cd provider, right? So I did a few I think I did half just to kind of see if there are different ideas, right. That I maybe haven't thought of, but in the end it's basically what you said. They call it rule-based rules, which is like regex. Or you can say if you say what's the age of Murillo in the prompt, maybe the answer you expect to have the word age, right? So if it doesn't have the word age, that's already a failure of the test. And these examples are still deterministic. Deterministic, yeah. So it can be regex, can be find a word, can be length, can be whatever, but basically all things that are deterministic, that's what they call rule-based you were going to say something.
Speaker 1:Yeah, I know, but isn't it easy just to output the response of the LM in a structured way, I know in Chachapiti or with the so there is some.
Speaker 2:there is also that, like OpenAI, they also you can validate the response If you say I give you, like the NER example, right, I give you this text, tell me who is the company name, the country and the person. So, for these three, or maybe the age of the person as well, right? So for these different properties, you already know what to expect. You know the age needs to be a natural number, so not negative, an integer right and then you can actually validate.
Speaker 2:So even open ai in the client, in the response, they actually parse it as a pydentic model, which I was also thinking when you said, like if you have a structure, that something's a no and you find out later. The validation thing is also nice because you find the error as soon as possible, right, so that's something that openAI does have. But this is even more generic, right, like what if it's just text that you actually want, like it's a chatbot, so you don't have a semi-structure format to validate from right. And then they talk about rule-based, which is like Regex, it's very deterministic things.
Speaker 3:And then they also talk about model-graded, which I haven't got to which I haven't got to, but I'm assuming he's just like asking another llm about the output of the other. Well, this is what you often do in probabilistic manner, exactly. Yeah, with the example of how old is marillo, if you say, if you have like a, a correct answer, I think a correct answer that I want to see is merino's 27 years old, 29 years old, wow your.
Speaker 2:Your attention span is really short it was just now, but okay, it's fine you're getting there.
Speaker 3:Let's say I have, I have a correct answer, that my optimum answer. Yeah. And then I ask, like nico is saying, then I ask an alum the follow-up question. This is my correct answer, given the previous answer that the alum gave, score it from zero to ten. And there you can say say something like this test passes if it has a score of more than eight.
Speaker 1:Yeah, true, typically that works quite okay but then it hallucinates and gives you 11.
Speaker 2:Yeah, but that's the thing, but. But I guess the thing is like if you do this a hundred times, like, what are the likelihoods like? That's why it's a bit probabilistic.
Speaker 1:Yeah, there is a chance, but it's very unlikely you're, you're scoring a problem, unproblematic thing or no, it's not a unknown thing with another thing that's unknown the output yeah yeah, yeah, it's a bit like.
Speaker 2:Yeah, I know, I know it also gives me a bit of a. It's easy for me.
Speaker 1:For me, a test needs to be reproducible, like if I run it a million times. I need to be a million times the same.
Speaker 2:Well, there's like with the deterministic testing, yeah that's what you need to do with the deterministic test okay, that's what you okay yeah, but it's like, yeah, I feel like a lot of the times for even this chat, gpt, you can change some parameters to try to get something a bit more deterministic right, like the temperature and all these things. Maybe you don't want that, maybe because maybe you do want a bit more stochastic, but uh, and I agree, I think it's never like the probabilistic stuff. The model graded stuff is never going to be a hundred percent. You're never going to be a hundred percent sure but I'm not 100 percent.
Speaker 1:Uh with lms and how it works in the end. Can you like if I ask the same question a hundred times to the same lm, the the same version? Should it give me a hundred times the same response?
Speaker 2:You can set parameters for that, okay, but like on OpenAI, as I understand well correct me if I'm wrong here, bart but like there's like temperature parameter that basically says how deterministic you want it to be.
Speaker 1:But can you make it super determin, super optimistic, that every time you I believe you can.
Speaker 2:I can believe you can.
Speaker 1:If you set the temperature to zero, every input will be mapped to one output so basically then, it probably takes the highest token or something, the most probable token, every time and then never chooses a random token.
Speaker 2:I believe that is. I believe that is.
Speaker 3:If it's still there. But there used to be a seat setting as well, an open AI at least, but there was talk that it was going to be deprecated.
Speaker 2:Ah, that's true, yeah, you probably also need a seat.
Speaker 3:This is possible in some situations.
Speaker 2:The other thing that they didn't mention on the course and something that I have thought, but I'm not sure if there's something missing. What about just looking at the embeddings? So if you just say like you have an expected answer, you can transform that into a vector and then you have the actual answer that you get and you just compare the vectors.
Speaker 2:If the vectors are too different, then maybe you need to flag it right, there's an alternative yeah because then it's a bit it's like it's a bit of a mix between the two, because you're still using the embedding, so you still have a semantic understanding of what the the sentence is, but that will be always. That is deterministic, right? Well, they're missing the sense of yeah. Actually, if you for two outputs, the, the vectors are always going to be the same yeah, I think it depends really depends on your test suite, like what is what?
Speaker 3:what type of data are you expecting?
Speaker 2:I don't know if that makes sense, yeah but for example, like because even on the course they also talk a bit about, like, the different types of errors, because the problem with embedding is also like you have your source of truth and in order to use it, sort of so, you need to convert it to embedding yeah but when converted to embedding it's basically a compressing step, so you lose data.
Speaker 3:So probably a year from now you want to go to a newer embedding model and you have no clue whatsoever what the impact on your test suite is but like, if you go for a new embedding model, because in the end you're gonna, you're gonna, we're working on the strings level, right.
Speaker 2:So the string, the two strings, they're gonna be the same, the embeddings will be different, maybe they're gonna have different sizes and everything, but the, the semantic meeting, should be the same, because the sentences are the same right, but what's the difference than just using an LM to do it?
Speaker 1:Doesn't the LM behind the scenes do kind of the same it?
Speaker 2:will do some of it, but I feel like, for example, if you say what's. Nico's age. And then the LM says oh, the sky is very blue outside, then I would imagine that the vectors are very different, and that's a very silly example, right? I don't know what's the actual difference between the vectors, but, like every time you're putting it through a model, there's an opportunity for hallucination. For the evaluation step, right, and if you're just comparing vectors, you don't have that.
Speaker 3:Yeah, I think it's like take the example, it's a good discussion. But I think it's like take the example, it's a good discussion, but I think it's difficult to really make an objective what is better or not? Yeah, like, because, for example, I can imagine that if you convert this to an embedding, like, the perfect answer is merido is 29 years old. The answer 27, in texas, okay, the answer 27, 29, sorry, 29, you already forgot again. But the answer merilo is a brazilian guy and he's 29 years old. It's also okay, but all those are very different things. So, if you compare them, euclidean distance, but that's probably what you're gonna do more or less, like between the vectors cosine distance, cosine, yeah, um, like, it's very hard to put a threshold on that, right, like to say this is still okay but also the 27 part, like isn't 27.
Speaker 1:Shouldn't that be very close to 29?
Speaker 2:yeah, that is true, that is true, but I think maybe that's a bad example, you're saying I was very wrong, huh, but I mean that's what?
Speaker 3:like from the moment that you do it like that, then you need to be very opinionated on what is that number where you say that this distance, yeah, but to be honest, but it's like if it feels better, but if you have a traditional machine learning model and you want to do statistical testing, so you'd have a gold standard data set and you say you pass it through ci.
Speaker 2:And it says if the, if the model is the recall is above 80, that's okay, okay, but to say 80% is also arbitrary, right? You have to set thresholds at some point and, yeah, there needs to be some tuning.
Speaker 3:but yeah, but traditionally when you're talking about a classification model, it's easier to interpret what it means.
Speaker 2:Yeah, but the argument of you have to set an arbitrary threshold is the same. It's also there Like I agree, you need to set a threshold, right.
Speaker 3:But that's for me. I guess Investigating what the threshold should be like in this example, marilo, and like all the different answers that can be correct, is very hard and very labor intensive to do that.
Speaker 2:I don't know how labor intensive would be. I think it would be interesting to try it out. But I'm also thinking for things a bit more complex. Like you do have a reg system, right. But I'm also thinking for things a bit more complex, like you do have a RAG system. So you do know, like the classical, is HR what to do with my holidays in Belgium? And there is an answer that we know what it is. But maybe because in the RAG, maybe the model will hallucinate or something, and if it hallucinates, I would expect to say something fairly off, I don't know. Also, these models change, so it's also hard to fully predict. But it's something that I haven't seen anywhere. But I and I because I haven't seen I feel like I'm missing something. But I'm not sure if it's like the idea in itself wouldn't work or if it would be just the same as doing a model. I still see value in it, but I haven't seen anyone propose this yet we actually tried it on a project.
Speaker 3:You tried it, yeah, yeah, oh really, like literally what you're saying, oh really. And then we moved to the lm evaluation. Okay, but like what?
Speaker 1:because you're actually like compressed, because, like, if you calculate the distance, do you get one number or is it multiple numbers?
Speaker 2:one number.
Speaker 1:If you do cosine similarity, it's just one number yeah, because like um, like the 27 and maybe something that's yeah, 27 can be very close to 29, but also Morelos and Brazilian guy that can also be as far as Exactly no. No, I agree, 27, right.
Speaker 2:For me. I'm thinking more the errors of hallucination. Right, it just says something completely off right. The classical, I don't know like you would hear, like I don't know you ask, gemini just says something completely off right. The classical, I don't know like you would hear, like I don't know you guys, 27 is definitely over?
Speaker 2:no, but for example, gemini was the question like on the, when google was trying to use aia, like, oh, my pizza cheese is sliding off, what to do? And then the answer was oh yeah, you should put uh glue on it, like those kind of things that are absurd. I would imagine that a vector search would would would catch right things that are absurd.
Speaker 3:I would imagine that a vector search would would catch right, but but I think a vector like comparison can catch. It is just like how much label you want to put in, because it will be much easier, like, if you have this answer put water on your pizza glue, put glue on your pizza and your reference is a pizza should have tomato and cheese and you're asking a lamb to compare whether or not the glue.
Speaker 3:Yeah, that's true, it will say no, it's not a correct answer. Yeah, that's true. I guess for me it's just because I do the factors. I need to start inspecting from what moment on what distance.
Speaker 2:Like, yeah, yeah no, I understand, For that very specific answer.
Speaker 3:I need to start optimizing.
Speaker 2:I guess for me I went there because also the reaction that nico had, you know it's like okay, now you have something that you're trying to test and you're trying to test the black box with another black box and it's black box all the way and I I I also agree with you that, like, if you put a model and you do this 100 times, what? Like? How many times have you actually experienced this?
Speaker 3:I would say, for most situations where that are simple enough, I would just force a structured output. Yeah, where you say, I want to have a json with with an h and a numeric value for that, and you just actually uh, read that json and see, do an actual deterministic test on it. Yeah, yeah, like, make that the standard and only for those cases using that lamp for evaluation no, but definitely agree.
Speaker 2:I also see from my no, but definitely agree. I also see from my perspective that most of the more mature Gen AI use cases are doing something like this they get the output and even if they do a layer of validation, even if you still want it as a natural language output, you still validate it and say, okay, based on this and only this, write an answer to the user right. So they always have this a bit guardrails every step.
Speaker 1:Yeah, but sometimes maybe you want to parse it to a json, but sometimes you also want to give the full sentence back. Maybe you can get that full sentence sent it to another llm saying parse out the relevant information into a json and then assert that json. Yeah, to be a bit more I know there is a like mocking or something or whatever, like I don't know how I would call it like.
Speaker 2:But I was talking with um button. He said that the there's a framework that we I think we talked about it called instructors instructor. That even does that. I think like, if you say, like you have a pidentic model, that this property is just a string, it can even go as far as like what should be in that string and it will do something like where it allows you to have like this, this class definition, and say the answer needs to be fit into this class, Like extract, all the things.
Speaker 3:But what you could do with in such an approach where you say one of my, one of the, the, the values in the class is my full answer and one of the other ones another key in a class is the is just the h number. Like you can have multiple things, so you can potentially also combine these things yeah, that's true.
Speaker 2:Yeah, interesting interesting food for thought yeah, yeah, yeah, there's a lot of, there's a lot of stuff, a lot of stuff there. But that's why, also, I thought that, going back to the courses, there's a lot of content, a lot of new things that people are discovering, and I feel like we try to stay on top of it. I think the things I'm saying make sense, right, but then I think sometimes it's good to to have this, uh, this quick check, um, anything else you want to cover. I feel like we already been talking for quite a while. Is there anything, uh, anything else that we want to bring up before we call it a pod?
Speaker 3:Maybe one thing when do you turn 30? Next year? What is the month? Let's not make it too specific.
Speaker 1:In five minutes, it will be 28.
Speaker 2:Yeah, it's like when are you turning 20 again September? There's still some time. We have some wiggle room. Yeah, I still have a few more good months left.
Speaker 3:Are you anxious about the big 30?
Speaker 2:I do think that it is a new chapter, I guess, right, like A new chapter of wisdom Well, I don't know wisdom, but something. But well, I'm married now as well, right? Also, I applied for permanent residency in Belgium and I think, like all these things are coming as I'm turning 30-ish, you know. So I feel like there's a lot of You're settling. Yeah, I mean I am settled. I would say I've been settling for some years now. But no, I just feel like it's like every day things like you said. It's kind of the same in a way, but then sometimes you take a few steps back and you're like oh, this is a this is a big.
Speaker 2:This is a big moment, you know, like it's kind of like iPhone releases, you know every release is like a bit the same, but then you look like five releases ago. Oh, actually it's changed kind of a lot, you know. But I think it gives me an opportunity to take a few steps back and be like oh, wow, okay, I feel like it is changing right. But no anxiety, necessarily. I already found some white hairs on my beard and stuff that was rougher, I feel. So, yeah, how old are you, nico?
Speaker 1:28 28.
Speaker 2:How old is nico? Bart? 26, all righty, cool. But uh, nico, thanks a lot for joining us. My pleasure, very cool discussions, glad to have you here, because I know sometimes, uh, it's like, yeah, I was into the pod and yeah, this llm's like I get what you guys are saying, but I'm just not, I'm not, just not buying it.
Speaker 1:So I'm glad you're here. Some stuff. I am for a little bit, no, but it's good I, I.
Speaker 3:It's good to have some healthy criticism exactly, I like that I like that, because of the like, I think we need more people that are a bit critical in this whole big hype group exactly and I like that you like.
Speaker 2:You just don't create like you bring good arguments for it, you know, and you're not just trying to go with the flow, you know. So I appreciate it. I think we had some nice discussions. I also like the topics you brought, so thanks a lot. I hope you had as much fun as I did at least I don't know about Bart, but I did. I did a lot. Thanks, bart, it was fun. Okay, I enjoyed it. Alrighty. Thanks for being here. Thanks everyone. Thanks for listening. Ciao, all right, see you all next time.
Speaker 2:In a way that's meaningful to somebody. Next weekend is a long weekend. No, Hello. This weekend I'm Bill Gates.
Speaker 1:You didn't know.
Speaker 2:Yeah, I went back on a cycle. Yeah, it writes Of course, running A lot of code, I won't do that I'm reminded, incidentally of Rust Rust.
Speaker 3:Yeah, actually we don't have a lot.
Speaker 2:This almost makes me happy that I didn't become a supermodel. Yeah, just super. Unless we can only camping, you're almost dirty I'm sorry, what's going on? Thank you for the opportunity to speak to you today. Are you ready for a met? It's really an honor to be here. Yeah, yeah, yeah, the coach. Yeah, yeah, yeah. Welcome to the Data Topics.
Speaker 3:Welcome to the.
Speaker 1:Data Topics Ciao Ciao.