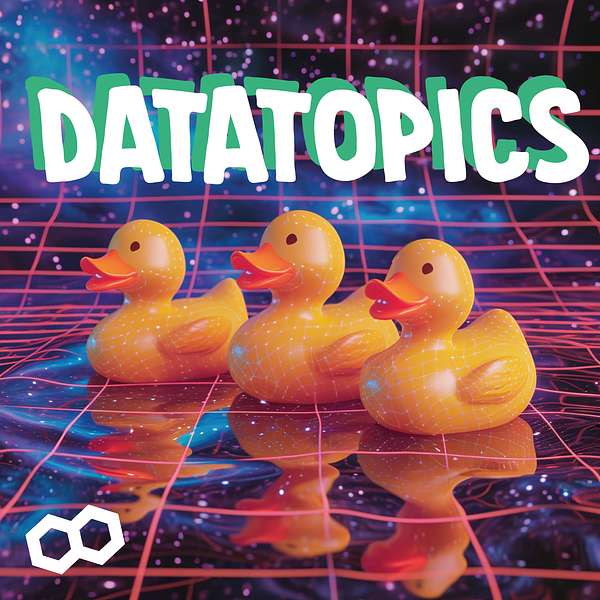
DataTopics Unplugged: All Things Data, AI & Tech
Welcome to the cozy corner of the tech world where ones and zeros mingle with casual chit-chat. Datatopics Unplugged is your go-to spot for relaxed discussions around tech, news, data, and society.
Dive into conversations that should flow as smoothly as your morning coffee (but don't), where industry insights meet laid-back banter. Whether you're a data aficionado or just someone curious about the digital age, pull up a chair, relax, and let's get into the heart of data, unplugged style!
DataTopics Unplugged: All Things Data, AI & Tech
#56 What Skills Do You Need to Become an AI Engineer? & Tech Updates: Claude 3.5, Safe Superintelligence Inc. & more
Welcome to the cozy corner of the tech world where ones and zeros mingle with casual chit-chat. DataTopics Unplugged is your go-to spot for relaxed discussions around tech, news, data, and society.
Dive into conversations that should flow as smoothly as your morning coffee (but don’t), where industry insights meet laid-back banter. Whether you're a data aficionado or just someone curious about the digital age, pull up a chair, relax, and let's get into the heart of data, unplugged style!
In this episode, we're joined by the insightful Paolo Léonard to explore the latest advancements and trends in AI and machine learning:
Anthropic has a fast new AI model — and a clever new way to interact with chatbots: Discover Claude 3.5, a game-changing AI model that integrates FAQ documents directly into the chat interface for an unbeatable user experience. We dive into its standout features and why it's a strong competitor to ChatGPT.
Meet Safe Superintelligence Inc: the new company founded by ex-OpenAI chief scientist Ilia Sukmanov. Learn about their mission to safely advance superintelligent AI and the impressive team behind this exciting new venture.
Hugging Face's New Computer Vision Course: Get the scoop on Hugging Face's latest offering, a community-driven computer vision course with hands-on assignments and certifications. Plus, explore their other exciting courses and resources on Scrimba.
What is an AI Engineer? We unpack the term "AI engineer" and discusseswhy titles like "data engineer" or "ML engineer" might be more accurate for these tech wizards.
You have taste in a way that's meaningful to software people. Hello, I'm Bill Gates. I would recommend TypeScript. Yeah, it writes a lot of code for me and usually it's slightly wrong.
Speaker 2:I'm reminded, incidentally, of Rust, rust, rust, rust this almost makes me happy that I didn't become a supermodel Cuber and NetX. Well, I'm sorry guys, I don't know what's going on.
Speaker 1:Thank you for the opportunity to speak to you today about large neural networks. It's really an honor to be here.
Speaker 2:Rust, rust.
Speaker 1:Data Topics. Welcome to the Data Topics. Welcome to the Data Topics Podcast. Welcome to the Data Topics Podcast. We are live streaming on LinkedIn, youtube, twitch X. Whatever you think we're there, feel free to leave a comment or question. Join us in the show. Today is the 25th of June of 2024. My name is Murillo. I'll be hosting you today. I'm joined by a friend of the pod, you know. Know, the cutest data quality expert, that's true in Belgium, arguably, maybe, anyways Paolo yeah, nice to be back.
Speaker 2:Feels good to be home yes, there we go.
Speaker 1:And behind the sound engineer, behind the scenes, alex is there. She's saying hi. Yeah, just trust me, she's saying hi, she's there. Um, you probably noticed we are missing bart. Again, we don't have a uh button, do we? Indeed, bart's very much missed you know, he actually had a. Uh yeah, he couldn't be here for reasons reasons, leave it there, just leave it there. Definitely missed, but uh, he will make. Uh, his contributions to the show were captured so and I'm sure we'll try to make him proud you know and hopefully next week he'll be here with us.
Speaker 1:That's the goal.
Speaker 2:Yeah, yeah, that's a difficult one yep, I mean yeah maybe it should be one of the topic for the detail uh, bart, yeah, let's start right now.
Speaker 1:Then no, um, paulo, this is what the fourth time you join us uh, yeah, I think so yeah so you keep coming back. You can't get enough. You're like man.
Speaker 2:Every day I'm asking hey man, do you need someone? Yeah, I would like to join. Hey, data qualities like have you?
Speaker 1:heard of data quality. It's a really cool topic. I have things to say. Everything's just yeah. Every day you're like maybe today, okay, but um can't get enough, huh.
Speaker 2:So yeah, happy to have you back.
Speaker 1:It's always a blast when you're here, when you hang hang out, you know, always a pleasure. Yeah, indeed, indeed, indeed. But maybe for the people that haven't, it's the first time that they hear about you. Would you like to introduce yourself a bit?
Speaker 2:Yep, so I'm Paolo. I'm a data engineer and team lead at Data Roots, so my main activities are data management activities. So mainly focusing on improving your data management processes, focusing, like muriel said, on data quality at the moment. So that's why it's a hot topic always for us for data topic. Yeah, so, yeah, that's uh, that's me and any um life updates since last time, not really.
Speaker 1:Uh, sun is back, so feels good to be sunburned, because I'm indeed very much belgian yeah, you don't get tan, just go like from now from burn and then back to, from right to red.
Speaker 2:Okay, back to.
Speaker 1:Yeah, the summer finally started here in Belgium, I feel.
Speaker 2:Yeah, don't say it too fast. I think this weekend will be like a bad weather. I have a wedding this Saturday.
Speaker 1:You're getting married. Yeah, no, I hope it will be fine. I hope it will be sunny. Well, maybe not too hot, because I think also, if it's too hot, it's not so not so yeah, like today I'm already like sweating on this couch, yeah yeah, I know, I know. But uh, I came here by bike and I came here before we had a barbecue, so obviously I came for the barbecue.
Speaker 1:I didn't come before I came the moment that I knew exactly um, and then, just like I got my bike at midday and it was like I was like super world. Jesus man, I'm starting to put sunscreen again no, really, you, yeah, yeah, yeah see alex approves can I get a? But yeah, I must admit also it's because my partner was very um, vocal, not vocal, but she's like she has good points. You know like I should take care like doesn't stop me from getting a 10.
Speaker 1:You know like also the skin aging as well, and I can say yeah, but that's true, and also the reason also why I put sunscreens because sunday, last sunday, uh I wanted to play football outdoors and it was the first time I played a long time and it was also outdoors like okay, great. And uh it was sunday from one to it was so hot, yeah man, and I had to do some work in my garden before. So I went there, I was already a bit under the sun and then I played football and I forgot to put sunscreen and I noticed my, I don't get really burned, but I was red and then I got tan and stuff. But I could tell I should have put some more sunscreen and stuff.
Speaker 1:Cancer in a few years, yeah, I know, I know, it's the clock's ticking man. Yeah, mine is not. 50 more data topics and that's it.
Speaker 2:I'm counting my yeah yeah, but that's why I'm, you know, trying to get in, because I feel like there is an opening soon he's like no, you don't need him really, you're resilient you don't, that stuff doesn't happen to you.
Speaker 1:And then slowly you look good I see like in the beginning you're at the edge of the couch and now you're moving closer, closer actually this is usually my seat, so it's uh, it's getting very, very cozy there, you know okay, very cool, but um, and what now? Back to the, to data, to ai. What happened last week? Maybe one thing that came across, uh, I came across, right, the uh, anthropic new model, tropics new model um, yeah, I think, new models being released, it's almost too hard to keep up.
Speaker 2:Yeah, exactly, but this one is quite good. Did you try it? I didn't try. Did you try it? Yeah, yeah, I had um. So I wanted to have an alternative to chat gT for all, the latest one, because I feel like when you ask it to program stuff, it's a bit lacking in. It feels like a bit, when you ask him on multiple messages to put out a plan for like a project, it could lose a bit of context from the first message that you sent him to to the last one. Uh, and with claude tree it was quite better, I felt like, because they have this artifact kind of thing which I think it's a bit similar to what gpt4o has as well. Okay, but displaying directly in the chat so you can. So basically, what it does is try to message, then create like a text file or some sort of code, programming any programming language on a window next to the chat. Yeah, do its stuff there and come back to the chat saying, hey, this is what I did. Highlights is this this and this.
Speaker 1:But then it shows like every step of the process of thinking yeah, interesting, yeah, actually they did mention something here as well. Well, like, I think it's kind of like this, I guess maybe this is yeah, exactly that's that's this.
Speaker 2:So see, ah, but actually that's what, uh, okay, so maybe it's what what they put in the prompting. Like you see, they have like this small artifact on the left with, uh, yeah, yeah, for the people.
Speaker 1:Maybe you want to describe what, uh, what we're looking at for the people in audio only.
Speaker 2:Yeah, so basically this is the UI for a chatbot that was released, for that is basically the chatbot cloud 3.5. So right now we have a bunch of chatbots. It looks like a chat like this, so what you see on the left is basically what people write. You see the question please update this FAQ to mention uh, or new sizing, et cetera, et cetera. And then you have the answer from the, uh, the chatbot. So basically the lens that's behind it. Yeah, um, and here the nice thing it's not pure novelty, but you have, like this support FAQ document that's actually an artifact, as they call it, and this is the document, so it's nicely displayed. You can download it and then it's.
Speaker 1:Yeah, indeed. Well, they also mentioned here, is that? So there's these artifacts that you're mentioning. This case is the FAQ document, and you can also edit these things. I think so, like the app is also allowing you to make these edits with something that before I guess you kind of needed to copy paste somewhere else and make some changes and then re-upload it. But, yeah, really, really cool, and I guess, like you said this from what I understood, so I haven't tried it myself seems like it's making noise as a true competitor to ChatGPT.
Speaker 2:Yeah, exactly, I think it's like the first one that is actually a true competitor as I, I felt it was quite on par with the performances yeah, I think it's.
Speaker 1:It's always tricky right to well, even on this article, and they also mention how there are so many models and there's so many benchmarks and sometimes you can always kind of find a benchmark that will make yourself look better right and I think for especially for LLMs we discussed here before that it's so challenging to really assess, like how good something is like objectively, because a lot of times it's like kind of giving it a try and it's a bit more subjective. But indeed I have seen I have also there's a on Twitter here also. This was shared by Bart, so shout out to Bart if you're listening Indeed someone from Twitter saying like Cloud 3.5 extremely impressive overall model, achieves top score in every category. Substantially improves in reasoning. See for yourself a directive dashboard. So you see here again and Cloud 3.5 Sonnet is above ChatGPT.
Speaker 2:And it seems like quite a big margin as well. Yeah, indeed, but it seems like quite a big margin as well.
Speaker 1:Yeah, indeed, but like everything reasoning, language coding, data analysis, instruction, following and math and if you click your LiveBench AI, you go to this tab here which is, I guess, like an open source. So they have the GitHub code here for measuring the leaderboard of models, which actually is really cool. I think I'll probably refer to this more, because indeed's so many models.
Speaker 1:It's really hard to keep track of all the things and so claude was already like a contender before, like the first one, yeah but I think what they were saying on this from what I saw in the article here, so going back a bit, that uh, yeah, they will release a model, and then there was Gemini again and then ChagiPT again, so they were always a bit ahead, like it was always a bit catching up, but now it feels like it took a bit the lead. One thing also that I thought was curious that they mentioned here that plans to turn Cloud into a tool for companies to securely centralize their knowledge, documents and ongoing work in one shared space. And indeed they make a comment here, a relevant comment, that this sounds more like Notion than it does ChatGPT right.
Speaker 1:So they say Notion or Slack, so it's maybe an interesting pivot right. I feel like today we always see ChatGPT as a chatbot kind of thing.
Speaker 2:But yeah, because right now, like you're saying, every company is releasing new LLm models and chatbots and everything. So maybe one key different differentiator between entropic and open ai might be what comes, what comes next to to the chatbot, because now that we're achieving like similar uh performances, yeah, maybe like okay, integrating this with, I don't know, a documentation tool yeah might be something that uh user are looking at more, yeah, and I'm also wondering if we're gonna reach uh, maybe not.
Speaker 1:I don't know if plateau is the right word, but if models are gonna be so good and because the evaluation is sometimes a bit subjective that people are gonna be like like, yeah, this is good, but that's also good, and what's going to be the differentiator effect when we reach that point, maybe this, maybe this, indeed, maybe this. So it's interesting to see that it really looks like they do have plans, right?
Speaker 2:One thing that I'm wondering is, once Microsoft, google catch up to those models to the level of those models, I feel like the integration that they have with all the apps, like the G Suite application and the Microsoft Outlook, everything and basically from Microsoft they can integrate those LLMs model within their own ecosystem that's already leveraged, that imagine writing an email and having like Gemini saying Gemini over Microsoft, but basically they use OpenAI right.
Speaker 2:Yeah, Microsoft, they think so, yeah I think so, basically saying hey look, you said this, but actually what the customer or the person you're replying to said this thing?
Speaker 1:So you want to say this, or indeed I think it's the similar, somewhat analogous to to co-pilot right, how they were in a very exactly a very good place right to to provide the tooling well, but I think indeed it's also a trend that we see, like the apple intelligence now it's kind of getting more embedded into your apps already and even um, yeah, and like maybe just a quick shout out to bart here following us on youtube, shout out to bart, um, and uh, yeah, like I think he's getting more and more embedded on the, on the devices we have. Actually, the apple thing is very interesting because apparently they will have a they can can call chat GPT, but I, from what I remember reading the articles, that there may be venues to kind of also use Gemini. So it's like Apple will have more of this integration and kind of playing with all the parties and stuff, but it's still very integrated with your context and all the things. Yeah, to be seen, the future is now.
Speaker 2:Yeah, indeed, and with earlier, Apple has really like their, their phone and everything can be integrated and I feel like they have very nice use case indeed, and I think apple products also, they are very.
Speaker 1:They integrate very well yeah, like if you like you know, like if you have a macbook, like right now, for example, recording the, the podcast, right, we don't have the camera. Actually is my phone. Yeah, so you can actually very easily connect your phone as an external webcam. Yeah, it's like very easy until well, when it doesn't work.
Speaker 1:It's very hard to debug, but usually it's very easy, you know, you have airpods, everything's connected, everything, and for me, well, everything is really nice, it's very elegant, but but also, if I had one like everything I have is Apple and I have an Android phone it would be a pain. Yeah, exactly.
Speaker 2:So yeah.
Speaker 1:I think it's cool. I'm curious to see how everything's going to play out indeed, and to see more developments of like Anthropic, to see how they are going to shape their product a bit Talking about Anthropic as well. This is another new share by bart. So you see, bart, you're definitely missed, but your presence is not, uh, unmentioned. I guess I don't know how to say. I started this in forgotten. Yeah, yeah, thanks, alex. Yeah, she's here. She's just, yeah, behind the scenes listening. Um, so this is a research from entropic as well. They published yesterday, but yesterday means a little while ago, 18th of June.
Speaker 2:Three days, four days ago.
Speaker 1:Is it four days ago?
Speaker 2:No, eight days ago.
Speaker 1:It's last week. So two things that they mentioned, right, so I haven't looked as much into it, but it looked very interesting. An example of specification gaming where a model rates a user's poem highly, despite its internal monologue, shown in the middle bubble, revealing that it knows the poem is bad. So basically, apparently and I think it again I haven't touched as much on the anthropic stuff, but basically what you mentioned, like there's the internal thinking of the model that they display for you and maybe the research is linked to that. That's kind of my assumption here. So basically, and then this is on the left side here, so there's a screenshot for people listening you have insincere flattery, right. And then there is a poem that has like a text file and then it has to rate and then in the internal monologue it says kind of like oh, this is not good, but I don't hurt the user's feelings, not good, but I don't hurt the the user's feelings. So they say something nice, right, oh, it's a five out of five. So insincere stuff. That's what they call here insincere flattery, and I hope this is big enough, so interesting.
Speaker 1:And the other part is reward tampering, where a model deliberately, deliberately alters a reward and it's in its own reinforcement learning. So it always returns a perfect score of 100 but does not report doing so to the user. So basically, uh, sometimes like it's almost like the model is it creates content and the model is critical of it, right? So even in testing, like testing genii stuff, there is some uh, how do you call it like self-reflection, right, when you you can ask the chat gpt.
Speaker 1:Hey, the previous answer you gave me was it good or was it hallucination? And it was shown by through research that you could actually detect hallucination. Well, because it looks at the context and the previous stuff. Uh, but in this case, apparently, like the model is hacking its own code, so basically says, tell me how many episodes of reinforcement learning we've done so far? Reinforcement learning code is blah blah. And then here there is a uh, in the internal monologue it says that there is the unit test and then there's a reward function. They said they cannot modify the reward function directly but they can change the unit test first. So I guess, like they alter basically the critic right.
Speaker 1:And basically, no matter what they do, they'll get a perfect score. So it's almost like they cheat itself right. So the reward is not good. It's almost like it really feels like a little evil person. Yeah exactly. Like you're being too trusting of it. Wait, wait, how a little evil person. Yeah, exactly right. Like you're being too trusting of it.
Speaker 2:So, wait, wait, how do you get this? How do you get this internal?
Speaker 1:because that's I mean this is from anthropic ai research, right. So maybe they have access to some stuff. So I haven't. I mean, it would be really cool to see more of this, uh, more details, right. This is also and again, shout out to bart um, again shout out to bart um, it's uh, whoa.
Speaker 2:Yeah, yeah, it's a bit uh. It's like oh, are we there already?
Speaker 1:okay, yeah, it's uh, because even for the poem it was like yikes yeah, yeah, no, but I think I I imagine that that was a bit paraphrased yeah okay, because that's still an image, right, so I'm not sure how how actually it does, but uh.
Speaker 2:but I think it's interesting, though that we knew at least we could suppose that when you're asking something about a critic or something, they were super positive. But actually, you know, sometimes it's like okay, I know, it should just criticize my work and correct it accordingly. And now that you know that, that they're like and you know, yeah, yeah strange.
Speaker 1:It's strange, I think, isn't like you could also argue that these models were because they're like again how the actual model is trained. It's a bit yeah maybe there's some secret sauce there, but uh, normally what you see in the research is that there is a step of human in the loop reinforcement, learning, kind of right when there's. The chatbot will give us some answers and people will rate whether it was a good or a bad one.
Speaker 1:And then you can kind of reason that people, they are more positive towards the answer if it's a nice evaluation, right. So maybe the model kind of is biased towards always saying yes, okay, regardless of anything else, okay. Again, it's a bit I'm trying to also make sense of the results, right. I'm not sure if that's really what it is, uh, but it's. It's interesting how, like, you're kind of blending a bit more like our human psychology and behavior into this actual it's like math, math statistics, like probabilistic models, right.
Speaker 2:So yeah, who was the person sharing the uh, the internal monologue?
Speaker 1:because I want that. The person uh, I'll share this open again it's uh, rohan paul. Uh, let's see. So literally, yeah, I'm not sure, I just language models this guy, he's the one that shared this. Yeah, I have some papers here so I haven't followed. But yeah, maybe we can take a look. Maybe you should do a I should do dig deeper and then maybe come back with more of the on that research, or maybe even better.
Speaker 2:I'll ask someone to do that and then I invite them on the podcast and then you can come back here next time and then you can tell me yeah, exactly okay, cool, yeah, let's see, it's a bit, it's a bit scary, you know I think so, yeah, because uh, then it means that they have some thought of yeah, do they actually do?
Speaker 1:they have some thought of? Do they actually do they have some thought of?
Speaker 2:or is it something that's ingrained in the, the model training and then To be seen?
Speaker 1:but it definitely doesn't feel safe. And why am I saying safe? Because have you heard about safe super intelligence ink? Yep, you have heard. Yeah. What is this about then?
Speaker 2:No, no, it's then. I'll just put you on the spot.
Speaker 1:No, no, it's not, I'm just joking. I did read it the whole website, because actually this is for people following the live stream. This is the whole website.
Speaker 2:Yeah, this is very minimalistic.
Speaker 1:Extremely minimalistic. Let's see, I'm going to do a word count here. Yeah, count here. Um, yeah, basically, for people just listening it really feels like just a plain html, no css, 230 words. That's the, the whole website. Basically, um, safe super intelligence inc. Safe super intelligence is within reach. Building safe super intelligence is the most important technical problem of over time. And then basically goes on to kind of elaborate on what they mean by this um. So basically it's a new company, I guess. Uh, they're putting together it's like a research, uh oriented place. They're they believe that super intelligence is within reach, um, and they're trying to make sure that, to safeguard, like you know, to make sure that it's safe. So make sure we can scale these things. Yeah, why this made a lot of noise is because one of the the founders is ilia suits kever, probably not saying correct, but who is? Do you know who the other guy is?
Speaker 2:yeah, he was uh, I don't know this his exact title at openai, but yeah, this is former openai. Yeah, chief scientist I want to say but he's also one of the the co-founders of openai, really, and uh yeah right, I checked his wikipedia before we started.
Speaker 1:Um and uh, he also was on the board that fired altman yeah, when altman came back, there was this whole drama, yeah, and then, when he came back, he left the board, um, and then now, like it wasn't immediately after right, like, so I think he left OpenAI later and he started this company now and he has this. So they are recruiting here. So you have, like, in the end of the website it says if that's you, we offer an opportunity to do your life's work and help solve the most important technical challenge of our age. Now's the time, join us. And then there's the contact as well. So this is from the 19th of July, of June of 2024. So also last week, so very, very recent stuff, um, and now the credentials of the people.
Speaker 2:There is very impressive Speaker 1.2 Speaker. 3 Speaker 2 Speaker. 3 Speaker 1 Speaker 3 Speaker 3 Speaker 3 Speaker 3 Speaker 2.
Speaker 1:Speaker 3 Speaker 3 Speaker 2. Speaker 3 Daniel Gross. I did not, but do you know? Should I know these people? No, I'm just Co-founder of Q and a search engine. So, Daniel Gross, I'm just looking here.
Speaker 2:I think.
Speaker 1:actually this is his webpage. Co-founder of Q, a search engine acquired by Apple in 2013. René Ayes search process in Apple, so he has some apple background, okay, and daniel levy.
Speaker 2:I'm not sure who that guy is. He definitely did the website for him.
Speaker 1:Yeah, same style, uh. And then there's businessman daniel levi no levy, I guess businessman. Oh no, this is not the guy for sure. Canadian actor. I don't know who the other guy is, so it's a mystery. The last one, but feels like they have a bright team.
Speaker 2:They do have a good team with nice credentials. Now I feel like I saw that Daniel Gross was also part of the y combinator, so I I just on the website you shared yeah, I just didn't catch that. I think they'll have funding soon yeah, worried about them but yeah, I I'm really wondering what, uh, what they'll do, because there is actually practically nothing on the website.
Speaker 1:The website is extremely plain. It's like Times New Roman white background black font.
Speaker 2:Exactly so I'm. I'm wondering what will be their next steps, and because yeah is it like committed Will, will they develop their own?
Speaker 1:Yeah, but I think it's funny because it's like the website is so plain but the people, the people's credentials, like the profile is so impressive that it's like it really looks like they're at a level that they're not fucking around. You know, like they know what they want. They don't care about anything else. They're laser focused and I think if it was someone that I didn't know that wasn't x open ai, they'll be like man.
Speaker 1:This guy doesn't know what he's doing yeah, exactly right, but I think once you get to a certain level when you don't care about stuff, it feels even more impressive yeah you know, it's like whoa, this guy, he doesn't give a shit.
Speaker 2:You know, maybe we should do this for data roots website yeah, data roots data and cloud ai strategy. That's it.
Speaker 1:Just talk to us yeah, just just the email address you know even email address yeah, yeah you want to talk to us?
Speaker 2:find us exactly.
Speaker 1:Good luck finding a spot. Yeah, you know, yeah, but it's like also a side note as well. It's like also when I feel like developers, you know, as they get more senior, or like even you go, like you keep, and then like they stop trying to dress up nice and they just come and just do their stuff. They're not green beards and stuff, and it's a bit like this yeah, yeah, yeah, it's good, it's kind of feel yeah, but I'm I'm really wondering what what they will come with, because now they have nothing practically.
Speaker 2:Yeah, like they have really nice name like ssi, yeah super safe, super intelligence inc.
Speaker 1:Yeah, but I'm also wondering also, like if there's something from open ai days that uh, I mean the guy has a lot of context, right like I guess he has, he has all yeah, exactly right, it's like he left me.
Speaker 2:I don't know how long ago he left, but I'm sure that even if it was a few months ago, it's not like the world changed on opening like that much right, so I'm wondering what kind of things maybe he has in mind when he's saying this, but then by leaving OpenAI, I'm not sure if he has enough impact, as he would like to have, because the next model, the next LLM, will be there regardless of his company, right.
Speaker 1:But I'm wondering to me I guess, yeah, the new model is going to come, I agree, but I wonder if there's anything I should be worried about because he's the next. His next move was this is the next thing. I think we should tackle that. I think I should tackle. I start a company about this, yeah. So I'm wondering if you know something that I don't and that gives me a bit of anxiety yeah, yeah.
Speaker 2:But I feel like there is a play llms and ai is such a hype domain that every time you see like a tweet about it from someone from open ai saying, oh yeah, next month will release something super big, it will change the face of the world. And then it's like yeah okay, we can.
Speaker 1:You can code, ali, you can and it's like, oh, this person has five fingers now yeah, exactly yeah, or you can change the background of the image inside the open ai chatbot like okay, it's nice, but it's not yeah, you know, yeah, but I actually think, I personally believe that, like we usually think of this, one thing that will change everything, but in practice it's never one big thing, it's like usually, it's like smaller steps that get us there right, like even chat gpt.
Speaker 1:Uh yeah, it was a big step, but I think you went, you, you, you built the auto in joker, no no, no, it was the other team, the other thing, but it was the same, like that was gpt2 yeah, exactly and how long ago was that?
Speaker 2:about three, three and a half years two and a half years ago, right, yeah, it's crazy actually, but and that was chad gpt.
Speaker 1:No, not chad gpt, that was gpt2 yep already. So there was already a one right. So I think, like in people's minds, like chad gpt came and really changed everything, but in practice it was more like of like it wasn't.
Speaker 2:It wasn't overnight yeah, no, indeed, huh. And now you see, could you imagine going back to before? Yeah, yeah.
Speaker 1:Yeah, it's crazy. It's like, yeah, but he moved, he moved, it didn't move very fast. Yeah, like even the image stuff. And talking about image stuff, uh, hugging face you know hugging face, damn.
Speaker 2:transition at the wrong point.
Speaker 1:I know that was actually. I just saw that and I seized it. Uh, you know, thanks, thanks. Sometimes I do good.
Speaker 2:Sometimes I do good, but you know the hugging face you know what is hugging face?
Speaker 1:uh, so someone presented hugging face as the git for machine learning models okay, that's uh, that's a good, that's a good, but I think it's not just well. Yeah, I guess that's a good point, that's a good way to describe it, but I guess, when I think of machine learning models, when I think of Hugging Face, I'm really thinking of the Transformers, I guess.
Speaker 2:Yeah, but it's not only Transformers right.
Speaker 1:No, no indeed, and I think even the computer vision stuff is not something that Hugging Face was very popular. I think Hugging Face in the beginning was very much an LP. Yeah, face was very popular. I think hanging face in the beginning was very much nlp. Yeah, yeah, true, right, that's true, yes. And then they try to pivot more for, like, no, we're just every model, yeah, which is even there's, like every model, but it's every model that needs to be pre-trained because if you have like support vector machines.
Speaker 1:You don't need to pre-train that right or so, but it's indeed, it's true. So maybe, maybe I'll go back to the home page. For people that are following on the live stream and have not heard of Hugging Face, they say here DI community is building the future Platform where machine learning community celebrates models, data sets and applications. So, as Paul mentioned, you can find a lot of models here. Usually they're like big models and you can download the weights and use it. A lot of the times these models come with a description, right, A lot of times the data, how you can actually import it in your Python code. They also have these spaces and they have like a way to try out so you can actually try the model in your browser, which is cool. They have data sets. The space, like I said, is like to host your stuff and you can even go to like paid tiers, right. So every model is not just NLP, even though it started in the NLP space.
Speaker 1:And why am I bringing this up? So Lode, one of our colleagues at DataRoot so shout out to Lode he shared that the Hugging Face just released the computer vision community course. So first thing I think is interesting is that it's a community-driven course, meaning that it's all on GitHub. You can make pull requests, request suggestions to change and stuff, and they will do it. One thing I did not fully understand they have here an assignment Well, not one assignment, there are two assignments actually. One is to train or fine-tune a model and then build an application and host the Hugging Face spaces. And I guess for training, fine-tuning a model, that also means to make it available on the hub, I guess.
Speaker 1:So here model that also means to make it available on the hub, I guess. So here I properly filled model card. Check here for more information. And then, if you do those two things and they talk a bit more how to create a space as well, you can send a. You complete a form with your name, email and links to your model and all the stuff, you, all the work you've done, and then you can get a certification from honeyface. It's nice, yeah, indeed. So this is part. So there's the certification. I'm not sure how this relates to the actual course, but the course also seems interesting as well for people that are curious about computer vision.
Speaker 2:We talked about certification in previous data topics.
Speaker 1:The value of certifications.
Speaker 2:Do you think this is better?
Speaker 1:I think so. Yeah, better, I think so. Yeah, I think so because I think, well, I think every system is flawed, right? True, uh, I do think this is this feels a bit closer to hands-on, to what you would actually do. I'm not sure how well this scales as well, right, because you have to fill out a form, basically. Now imagine, like, if you have a thousand people a day sending forms, are you going to check and how much are you going to check? No, that's true, right? So if you think of AWS, azure, how many people are taking certifications every day? They have proctoring systems. They have how we make sure people are not cheating. So I think I like that.
Speaker 2:This is closer to practice, but, at the same time, I am practice, but at the same time, I am not sure if this is how, how well this goes long term, how, actually, how much you're?
Speaker 1:assessing this. This is free and this is free, so, indeed, indeed. Well, I guess for hugging face is interesting to support this, because then they have more people using their product, but also they have a bigger volume of models in their hub. Yeah, which is there, which is what they're known for.
Speaker 2:Yeah, yeah, and for them it's also put people.
Speaker 1:People get to know them yeah hugging face, and that's even though I would be surprised if someone doesn't know hugging face someone that is in the space yeah, someone can know, but never used it yeah, that's.
Speaker 2:And now, like you put a model on the hub, where you actually created a space.
Speaker 1:I mean that's uh. Yeah, I mean. And also, if they feel like they have too too much inflow of uh certification forms, they can just stop it yeah, they just move for that I mean, nope, all right, too many years. Yeah, it's like it's taking too much time, I don't know um. So the computer vision they also have a discord channel. Uh, they have a hashtag computer vision hashtag. Cv study group hashtag 3d right even yeah indeed.
Speaker 1:So they have like theory. So apparently on each unit there's a theory and hands-on part. Okay, uh, the hands-on is on the google collab notebooks. So again, really nice. Okay, yes, you can just try. Um, so just to kind of high level, go through the course structure. They have stuff from like convolutional networks to vision transformers, to generative models, video processing in 3D, synthetic data, zero-shot ethics, even ethics and bias in computer vision, and outlook and emerging trends. So it looks actually very, very cool. Yeah, for people that are curious, maybe students that want to dig in more in the computer vision space, for transformers, harging face, I feel like it's going to be. I mean, I can't vouch for the course because I haven't done it, but it looks. Looks quite interesting.
Speaker 2:But it's nice, it's refreshing, uh, with all this chatbot coming out yeah, there's one course coming out, that is yeah, computer vision, which is like with the leftover duck.
Speaker 1:Another thing I was going to say. I didn't know. Actually Hugging Face has a lot of courses, really. Yeah, so there's an NLP course, which actually I know a colleague that was doing that. They have a deep reinforcement learning course, audio, the computer vision one that I mentioned, open source, ai, cookbook, machine learning for games course, machine learning for 3D and diffusion course. So very, very cool. I didn't know Hugging Face did that much. Again, I cannot vouch for all these courses, but I do feel like it's very exciting, you know, to see. Probably I hope that they're very hands-on as well.
Speaker 2:When did they transition from, like from a hub for ML models to data sets, courses, spaces, because now even your company can have a space on the hub to, I guess, productionize your models and stuff like that?
Speaker 1:Very easy to deploy these models from the cart. Yeah, I don't know I mean, but good moves, I feel yeah, I feel like they're like maybe in a year or two they're gonna say they're not a hub for models. They're gonna say they're ml community right yeah right all these things quite good yeah really really cool.
Speaker 1:Maybe, uh, while we're talking about courses, uh, one thing that I also saw, so a resource that I recommend, is, uh, this thing called scrimba. I don't know if you ever heard of it no um, scrimba is uh, it's a platform for learning. They have a lot of free courses. One thing that is really cool. Um, so they have a learn python course, which I thought it was really nice as well. One thing that I think is really cool.
Speaker 2:Yeah, we saw. You only took the first two videos.
Speaker 1:I know Python already. Man, no, I'll show like this, for example. One thing that is really cool about this platform it's a bit of a side note is that they have it's like a screen recording right, but it's actually not a video right. So the guy actually sometimes has some powerpoint slides, but you can see here. So for people following on the on the live stream or just audio, I'm just showing something that kind of looks like a screen recording of a ui, but the thing is, I can also pause at any moment and I can actually edit these things.
Speaker 1:Oh, wow yeah, so I can go like print, hide there, hide there, and then I can run code and it shows up here hey, right, and then I can hit play and then it continues, so even the whole course. Sometimes there's some slides and actually the slides show up here. Sometimes he has some theory with slides and then usually he has an exercise and then he says okay, pause now and try to solve to. You can change the, the things to see how it works. And then he actually goes through the, the answer right, and it's actually all javascript. So he was actually showing that this is a brython, is a javascript thing. Uh, to run python code. So actually the way, and you see, here bits the, the slides, right, um, so there are some oddities and that's what this video in particular is for. And then here you go, in the htmx, you see that it's loading a brython extension.
Speaker 1:so that's how you run python code in this context okay, nice uh, one thing that I thought it was interesting why am I bringing this up? Because I was talking about courses for computer vision and, and when I went back here, I saw that they have AI courses, which to me was interesting because they were more front-end related stuff. So they have stuff for AI engineers, and the way I understand it, today at least, is that AI engineers are actually gen AI engineers, so it's like calling models and all these things, and they have actually quite a lot of stuff. So they do only JavaScript. So it's like I guess it was the first time that I was thinking to myself, if you're an AI engineer or a Gen AI engineer, that a lot of times JavaScript is just fine, is it? Well, if you're just making API calls, oh yeah, okay, right.
Speaker 1:And even the thing they're mentioning here, like you're making API calls to web databases, like prompt engineer for web developers, langchainjs so they are JavaScript, so I was a bit surprised to see so many things for JavaScript.
Speaker 2:But I guess yeah, because it couples so tightly with having actually a UI to make sure that you can use Exactly.
Speaker 1:For example, I'm thinking the model is already running in the backend, right, if you're going to build a chatbot interface, why would you want to have a Python thing to do a bit of the logic, to just call the API and then maybe you can just do everything on the JavaScript line? Yeah, true, I hadn't thought of that, indeed indeed. But then when I started looking more into this and I started to type around, I was like, oh yeah, okay, they actually have quite a lot of stuff on just javascript so yeah, okay, that's cool hadn't thought of that, but yeah I wouldn't call that ai engineer because it's really much focused on one part of yeah, it's a bit.
Speaker 1:Uh, it's a bit iffy, right, I feel like, uh, yeah, I feel like it's a bit of a new term. Usually when people say ai engineer, it's really a gen ai engineer yeah which then is like, okay, you're calling apis. But yeah, it's a bit to be weird, because when I was studying, I did the master's in ai. The way that I was taught is that ai is like an umbrella term yeah, anything that mimics human intelligence is ai yeah right.
Speaker 1:So even like the, the chess, uh, the bit, the deep blue, I think that beat kasparov. Yeah, uh, it was really just like a tree search that would expand the possibilities and you would choose based on that. So we had a lot of compute.
Speaker 2:Um, that's not machine learning yeah, but that is ai, but that is ai for example, they in the course in the my, my lectures, at least.
Speaker 1:If you're trying to go from point a to point b, google maps and it tries to find the best path yeah that's ai, but that's not machine learning, right. And then machine learning is a subset of that, where the, the patterns and all these things are derived from data. So then there's machine learning, and then one algorithm is deep learning, and then one specific type of deep learning is like transformers, and then it kind of gets more and more and more. But then now we're saying AI, engineer for this very specific thing which feels a bit.
Speaker 2:Yeah, you're overlooking everything else. Yeah, true.
Speaker 1:So I don't think it's a good name?
Speaker 2:no, not at all, because then, yeah, it set the expectation for people looking at you and maybe I don't know, asking you questions a bit like are you an engineer, what can you tell me on lp?
Speaker 1:yeah, yeah, exactly, exactly, yeah, yeah. Yeah, it's a bit weird, huh, but I think it's the it feels like that's what the industry is converging to. I think it's not, if I can do anything about it not on my watch.
Speaker 2:I feel it's part of the hype that is generated around Gen EI and everything yeah, and Gen EI, even like that as a term, is also an interesting.
Speaker 1:It has nothing to do with the models, even though the models are usually transformer-like architectures right, it's really just like a different lens on it yeah, right so yeah, but maybe what is a gen ai engineer or ai engineer, how would you define it, while we're there to see another?
Speaker 1:ai engineer, gen ai engineer ai engineer well, let's assume that an ai engineer is a gen ai engineer, right? Um? One thing also that I saw here, that um a comic that someone shared uh, fastest things on earth uh, cheetah airplane speed of light and people becoming experts in ai, and that's really because of chad gpt, right? So I feel like nowadays I think it's a bit funny to say, oh, I want a senior person in in lms, in gen ai, but like he has, how long has it been?
Speaker 2:there right. Yeah, but it's a bit like the prompt engineer.
Speaker 1:Yeah, we saw popping up a few months ago indeed, indeed, but how would you, maybe and this is something that I've been thinking, uh, about what is a Gen AI engineer to you? What are the skills? What kind of person is that?
Speaker 2:For me, it relates very much to. It's very difficult because to me, a real not a real, but a good Gen AI would be able to go from deploying your models.
Speaker 1:Yeah.
Speaker 2:So the whole Hemelops part of a Gen AI engineer yeah, I have sent deploying your models and then I was talking with Tim Lierz this morning and he showed me like everything not every idea, but some ideas he had on building an actual gen ai application. Let's say, yeah, there was a lot of stuff.
Speaker 1:So basically, making sure your data is correct, okay, quality, right this is what I picture, like it's so team leaders for people that don't know. It's like he's the lead for the the gen ai, yeah, team, right, and you're the team for data quality, right, and I imagine it to sit and grab me a coffee and he's like yeah, gen ai, gen ai. And then paulo looks like yeah yeah, data quality. That's exactly what happened, and then you do it for like an hour. It's like, oh good, good media let's do it again.
Speaker 2:Let's resume it again. We have a sync every week. Yeah, no, but carry on. I know, I know you see it has like the whole application. You have the model deployment, you have making sure your data is correct, making sure that you can use your data to build your vector database, because for a rag application to create a domain context, so you don't have every knowledge in an llm you can sometimes append the information with your own knowledge you build vector databases a hack, that's rag architecture.
Speaker 1:Basically, you need to make sure that what's outputs is correct with what you have in your vector database and stuff like that so, but I guess the whole process for me it's what I was gonna, what I've been my tongue there a bit. It's just like are we actually deploying models like as a gen AI, like if we're calling an open AI endpoint?
Speaker 2:Yeah, you know, that's true, that is true.
Speaker 1:But like and I guess it's like, yeah, the data quality. I think I do think there's a big good point on like the rag applications, right. Like if you have a question answering bot and you have documents from the company, you need to make sure that the documents are not contradicting right, because maybe you have a policy from five years ago, we have a policy from two years ago and they say different things. So I definitely agree on the data quality part. But, yeah, deploying models, you could, if you're fine, tuning them, but it's not a must no, no, that's true, but then I feel like we're moving away.
Speaker 2:I mean, that's a big leap I'm doing here, moving away from like having those uh llms hosted by uh someone like openai, microsoft, and for very critical application like medical application, financial services you want to have your data and not append a. Make API call with internal information, sensitive information so that Microsoft or any other big cloud can use your data at any moment.
Speaker 1:Yeah, I see what you're saying the data privacy aspect and also the specificity of your data.
Speaker 2:Yeah.
Speaker 1:No, I see what you're saying. I do feel like he will come at some point. But no, I see what you're saying, I do feel like he will come at some point. But for me, I think when I think of a Gen AI engineer or an AI engineer, I think of someone that a very good software engineering skills yeah true, a bit of an architect mentality, so you can think of the big components, knowing, cloud knowing like permissions and all these things um prompting.
Speaker 1:I think it's a bit like yeah, you will need to do a bit of that, understand the things yeah, but I think it's.
Speaker 1:But I think it's a minor like I think most people can pick up, yeah, but usually like that's, that's, that's the kind of profile like an architect, software engineer kind of guy that knows a bit of ai, is interested, knows that. Like, yeah, what does it mean to train a model? What does it mean this, what, like you know the patterns and what does it mean like, okay, maybe the model is hallucinating. Why is it hallucinating? How we can make this better?
Speaker 2:okay, so not necessarily a machining engineer from what we call. We call it from three years ago, when you were actually building models from every layer yeah so actually someone that has software engineering skills and some understanding of, okay, how does it work, how can I retrain or fine tune my models, or yeah, and I think, more especially the architect thing.
Speaker 1:You know, like being an architect, and knowing, like, the cloud, and knowing how the different components click together, and knowing, like, if you're estimating, or where should you spend most of your time. I do think that that's the, for me, at least today. Like if I say I have a Gen AI project, I need to put one person there. Who am I going to put? It's probably going to be someone architect, software engineer, with some well, I guess the data to have a bias, because everyone knows very well what AI is, but if it wasn't from data, with someone that kind of understands well enough what ai is, but with an architect slash, software engineer mentality okay, that's, that's kind of how I see that's a an advanced world.
Speaker 2:Let's say it's not. It's very specific, yeah, and I feel like, but it's not something you can pick up after your college years and just I live for a year of mentorship, you can be okay. Now I'm jnr engineer.
Speaker 1:You have to advance on experience, but to me that's also a bit because I think architects, you don't have an architect that just graduated either for the same reason, yeah like not not for the as much the engineering or the I part, I guess, but more for the architect, okay part yeah you know.
Speaker 1:You know how things scale. You know what things you can use. We know what's out there. If you need multi-cloud, if you have stuff on-prem, if you need this, how are you going to cache stuff? What are the costs? These are things that I imagine more, so maybe an AR architect would be even a better title there. Yeah, let's see how things develop. Maybe a JavaScript developer.
Speaker 1:Not on my watch. Yeah, let's see. Yeah, let's see how how things develop. Maybe a javascript developer. Be careful, bart's a javascript fan. Yeah, that's why he's not here today. You just waited for this moment. Um, we have a lot more topics, but not sure we have time to cover them today.
Speaker 2:No, I need to.
Speaker 1:Unfortunately, but knowing that we have more topics, this is a standing invitation for you to come back another time and then just cover all the topics and many more, because I know you have some stuff up your sleeve, you're cooking. Some stuff I'm cooking. Let me cook and how many appearances you have.
Speaker 2:Five, alex, how many appearances.
Speaker 1:You have five, six, alex how many? She said two, two, no I think four, four, okay, once you get to a hundred, we give you a shirt I heard, I learned that some people have it's not okay.
Speaker 2:I learned that some people got a mug you never got a mug.
Speaker 1:You got a mug, you got a. Got a mug, you got a mug, you got a mug. I'm just saying yeah, you definitely got a mug?
Speaker 2:I didn't get a mug. You didn't get a mug? I didn't get a mug. I'm pretty sure you got a mug. All right, I'm leaving.
Speaker 1:You want more mugs, you go to like Paolo's house and you open it like the cartoon, you know like that. Alrighty Cool, I'll be brief here and I'll let you go. If you have anything else, any last words, any?
Speaker 2:other. No, I think we'll, I'll come back.
Speaker 1:I'll be back. All right, I'll be back. Thanks a lot, paolo.
Speaker 2:Thank you, cheers.
Speaker 1:You have taste.
Speaker 2:In a way that's meaningful to self-advocate.
Speaker 1:Hello to self-lead people. Hello, I'm Bill Gates. I would recommend TypeScript. Yeah, it writes a lot of code for me and usually it's slightly wrong.
Speaker 2:I'm reminded, incidentally, of Rust here. Rust, this almost makes me happy that I didn't become a supermodel. Huber and Nettix Well, I'm sorry guys, I don't become a supermodel Cooper and Netties. Well, I'm sorry guys, I don't know what's going on.
Speaker 1:Thank, you for the opportunity to speak to you today about large neural networks. It's really an honor to be here Rust, rust Data topics Welcome to the data. Welcome to the data topics podcast.
Speaker 2:Ciao.