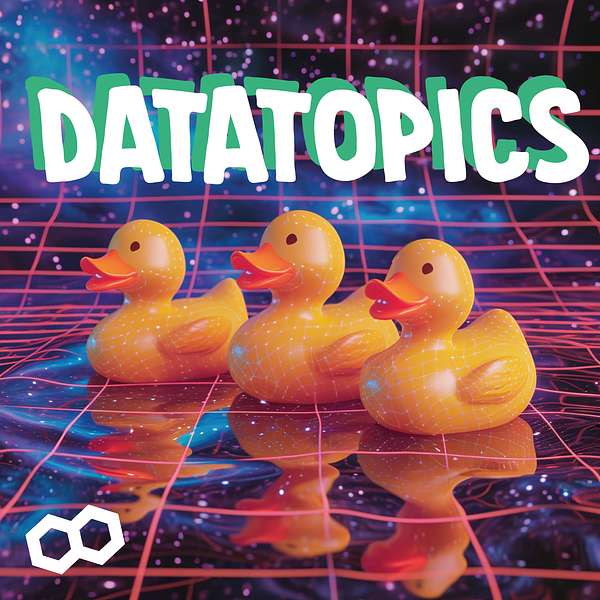
DataTopics Unplugged: All Things Data, AI & Tech
Welcome to the cozy corner of the tech world where ones and zeros mingle with casual chit-chat. Datatopics Unplugged is your go-to spot for relaxed discussions around tech, news, data, and society.
Dive into conversations that should flow as smoothly as your morning coffee (but don't), where industry insights meet laid-back banter. Whether you're a data aficionado or just someone curious about the digital age, pull up a chair, relax, and let's get into the heart of data, unplugged style!
DataTopics Unplugged: All Things Data, AI & Tech
#49 How Will the EU AI Act Affect the Future of AI?
Welcome to the cozy corner of the tech world where ones and zeros mingle with casual chit-chat. Datatopics Unplugged is your go-to spot for relaxed discussions around tech, news, data, and society.
Dive into conversations that should flow as smoothly as your morning coffee (but don't), where industry insights meet laid-back banter. Whether you're a data aficionado or just someone curious about the digital age, pull up a chair, relax, and let's get into the heart of data, unplugged style!
In this episode, we're joined by special guest Maryam Ilyas as we delve into a variety of topics that shape our digital world:
- Women’s Healthcare Insights: Exploring the Oura ring's commitment during Women's Health Awareness Month and its role in addressing the underrepresentation of female health conditions in research.
- A Deep Dive into the EU AI Act: Examining the AI Act’s implications, including its classification of AI systems (prohibited, high-risk, limited-risk, and minimal-risk), ethical concerns, regulatory challenges & the act's impact on AI usage, particularly regarding mass surveillance at the Paris Olympics.
- The Evolution of Music and AI: Reviewing the AI-generated music video for "The Hardest Part" by Washed Out, directed by Paul Trillo, showcasing AI’s growing role in the arts.
- Hot Takes on Data Tools: Is combining SQL, PySpark (and Python) in Databricks the most powerful tool in the data space? Let's dissect the possibilities and limitations.
Don't forget to check us out on Youtube too, where you can find a lot more content beyond the podcast!
you have taste in a way that's meaningful to software people I'm bill gates, I would. I would recommend.
Speaker 3:Uh, yeah, it writes oh, that's a difficult a lot of code for me usually it's slightly wrong.
Speaker 2:Maybe paulo's reminded it's a rust here, congressman. Uh, iphone is made by a different company and so you know you will not learn Rust while skydiving.
Speaker 3:Well, I'm sorry guys, I don't know what's going on.
Speaker 1:Thank you for the opportunity to speak to you today about large neural networks. It's really an honor to be here.
Speaker 2:Rust.
Speaker 1:Data Topics Welcome to the Data Topics.
Speaker 2:Welcome to the Data Topics podcast.
Speaker 3:Welcome to the Data Topics Podcast.
Speaker 1:Hello and welcome to the Data Topics Unplugged, your casual corner of the web where we discuss what's new in data every week, from the AI Act to rings, anything goes. We are live on LinkedIn. We are live on X Twitch. Feel free to check us out there. If you're following us in the live stream, feel free to drop a comment. I will try to keep an eye out. I think last week last week we missed Alex actually, so there was also a comment on the chat that I saw later. So my apologies, sam, but if you try, if you leave a comment, we'll try to address it in the stream. Um, come hang out with us virtually. Um. Today is the 7th of may of 2024. My name is marillo. I'm going to be hosting you today. I'm joined by the one and only bart hi.
Speaker 1:Alex is back behind the scenes hello and we have a very special guest today. We have Mariam actually return guest. Yeah, I think the she's the third return guest. No, I'm not not, yeah, taking a score yeah, I was surprised, the first one, the second, I was like okay, maybe, maybe it's not that bad yeah, maybe it's not that bad. Yeah, maybe it's not that bad. You know it's fine.
Speaker 2:Okay, a friend of the show.
Speaker 1:Yeah, a friend of the show, indeed, but, mariam, for people that haven't followed us the first time, uh, would you like to say a few words about yourself?
Speaker 3:Um, I. I work at the data strategy team, then in data roots. I've been working here for a year now. I work as an analytics engineer, but I do have broad interests, so from there the topic today that we will discuss. What can I say more? I live in Hasselt. That's a good detail. Hasselt is a very cozy city.
Speaker 1:Nice, cool, that's it. Yeah, if you mentioned analytics engineering, I think last time we discussed a lot about analytics engineering, so an invite for people to follow up there as well. And, mariam, did you know that May is let me check my notes so I don't mess it up is actually, I did mess it up already May is the Women's Health Month. Did you know that?
Speaker 3:actually no.
Speaker 1:Actually no, yes but it is so and the reason why I know about it is because I, I have an aura ring. Bart likes to call it a friendship ring because you know, besties, we both have one. Yes, yes, for the people, yeah, well, let's just listen, we both have our rings. So our ring, what is it?
Speaker 2:our ring like very tldr Bart uh, smart ring, that, uh, that uh shows you basically how good you slept.
Speaker 1:Does a lot more things yeah, it's like a health tracker thing, right, so it does like kind of what the watches do, but it's a ring and there are pros and cons. I like kind of what the watches do, but it's a ring and there are pros and cons. I think a big competitor is the garmin watch, right, like has a good battery life, etc, etc. Uh, but yeah, it's a ring and uh, they also part of the. The services they have is like they have a blog that they show some updates also how you can also use your uh ring with like how you can optimize it. I guess, right, like how you can do experiments or what does this thing mean?
Speaker 1:that means, and this month actually- it's a bit similar to uh like whoop straps or uh functionality in an apple watch indeed indeed, indeed, indeed, and this month actually, I wasn't aware either, but they mentioned that it's the women's health month or women's health month awareness and uh, actually ordering. Did you know, bart, that I think it was uh built by women here, I didn't know, built for women, by women. I think actually, looking back, it makes sense. If you think it's a ring as a wearable device, I can see how that's like a more catered towards women kind of thing. Bart's second guessing every decision of his life.
Speaker 1:But, yeah, so there are a lot of like scientists here and think part of the reason why they wanted to to come up with this is for spreading awareness, right? So I think, historically, women have been left out of clinical trials, of studies and all these things, because the woman's body is very complex, with the hormonal cycles and all these things, um, and our ring. They actually addressed this head on. So they do mention this year and this part, part of the the may awareness month and all these things, uh. So some some facts here. Um, female health conditions outside on college receive less than two percent of the current health pipeline, despite women making up half the world's population. They also talk about some eye-opening consequences of these things, that women are twice as likely as men to experience adverse events from drugs, and I believe so. I have I'm not an expert on this, but I believe that it's because women, again, are left out of these clinical trials, because there's a lot of more factors that vary, right, but that percentage is great.
Speaker 2:So female health conditions, female health conditions outside of oncology receive less than two percent of the current healthcare pipeline. That means, like uh, attention to research for female health conditions yeah, outside oncology right, but of course a lot of health conditions are not general, specific right.
Speaker 1:So yeah, but I, that's the thing I feel like again, not an expert but probably for me it's more right yeah, I think.
Speaker 1:I think it's like male physiology is more not stable, but it's like it. It's more predictable, I guess, like we have like a 24 hour cycle kind of thing, and women with the hormonal fluctuations and stuff, it makes it more difficult to study in a large scale. So people like scientists I also think that may be a bias towards men, like doctors as well, right that they tend to focus on that. So they even mentioned here like they did a poll and 77 of women reported that they felt they were the only advocates for their well-being or I don't know. Sometimes like things are misdiagnosed, right. So aura actually brings a lot of stuff. They have like specific for women that have the period prediction on the aura ring. They have cycle insights, pregnancy insights, which I thought was pretty cool and I think, since this is a may, is the women's health month. Uh, just wanted to do a small shout out here. Also, we have a illustrious presence of mariam on the panel, so I thought it would be, appropriate here, but enough with that.
Speaker 1:Any comments, thoughts, reactions.
Speaker 2:Surprises anything maybe is do they give any insights on, uh, like women related? So they have a lot of data right like do they, do they share anything that comes from their aura ring?
Speaker 1:I have seen. Well, I think they, they do. Um, they also partnered with other things as well. I have received also, like there was a blog post that they talked about the whole population, so not just women in general. Uh, because you can create tags right on our ring. So usually there's some pre-made tags, but there are also some, uh, other tags that you can actually edit yourself right. So, for example, if you go for a run, you can actually say, oh, today I went for a run and that's why my heart rate is higher, or whatever. But you can also do things like I can create a custom tag right, so I want to play cricket and maybe cricket is not on the other ring. And then they, they launched how, actually the most common tag, custom tag, is actually weed or cannabis, and they were talking, they did a little article like how this can affect your sleep and all these things. So they do collect this anonymized data.
Speaker 2:I'm not sure about women specific, but I I can imagine that they, they do and we're a data podcast like do you do anything with the data that comes from your smart ring?
Speaker 1:no, not yet. I do think. Uh, well, the so more context on the ring itself. They do have like raw metrics right, like hardware, variability beats per minute and all these things, but they also have more interpreted metrics right, like based on this, you are very good sleep or not so good sleep, right? Um, so I, I, I look at those kind of semi-frequently, you know, to see patterns as well. Am I sleeping well, is it going good or bad? But I don't do anything custom myself because I know you have used it for personalized training sessions yeah, you get a readiness score.
Speaker 2:It's basically something that's a proxy to your recovery and I use it in my uh building a training schedule.
Speaker 3:Yeah, for my workouts yeah, but that's the thing with the data, because I wanted to try something similar, but you have to manually put in the data. I couldn't find a way at least not for the samsung watch I had at that time that I could generally just extract my data out of it. I would have to really ask for permissions or submit a form to get my own data. That's also something.
Speaker 1:Even for your data, yeah, just for my personal data.
Speaker 3:I would have liked to get all the metrics and then I would have a database and then I can do my analysis on it. But yeah, I couldn't get it. It was not very easy.
Speaker 2:Yeah, it's very close.
Speaker 3:So for example OR integrates very well with.
Speaker 2:Apple Health To some extent. But indeed it's very close to ecosystem.
Speaker 1:Yeah, the Apple Health is really nice. I think the only thing is like if I go work out because you know, so if I'm going to go do weightlifting, then I don't use the ring because you can actually damage the ring, right?
Speaker 2:yeah, then a whoop is better. Yeah, but like I use that because I also have a apple watch and it's a bit uh, oh. Yeah, you also have an apple, I also have an apple watch starting to become very gimmicky, I know, I know.
Speaker 3:I'm curious how it works. So your sleep would be tracked two times. Does it still come one time or is it more accurate because it's two times?
Speaker 1:no, I I mean so the the things that get shared from apple health is like if I do a workout, I can actually tag this exercise on the apple health on the apple watch.
Speaker 2:Then it goes to apple health and then it shows up in aura and is your data consistent, like the sleep on your apple watch versus the ori?
Speaker 1:usually I mean, I have, I haven't really checked that much the apple health, um, but like usually, it's like sometimes you get notifications from apple health, hey, you met your sleep goal, right, like how, and then I don't know, I never said oh, I'm sleeping.
Speaker 1:Now, you know, like usually the text from the the no, but if you would compare the data from the apple, uh, the health watch yeah, it's coming from there and the ordering I don't check that like that much in detail, like what's the percentage of deep sleep or everything, but like I do get notifications from health like oh, you slept more than eight hours, great job, and the o-ring says the same thing. So I don't have any reasons to believe it's not the same. But I haven't done a thorough analysis on it and maybe I should. But the fact is, like apple health, uh, apple watch doesn't. The battery doesn't last that well. So a lot of the times I leave it charging overnight and it just uses the ring right um a lot of sites on the weekends I don't want notifications, so I don't even use the watch right, I just have the ring. So I'm a bit selective with this. So that's also why it's a bit hard to really really measure and the data is still not collected at one place.
Speaker 3:You have or and the app for the data coming from our ring yeah, I think.
Speaker 1:I think that the data from the ordering may go to Apple Health as well. I think they share that with the.
Speaker 2:If you enable it, that's good.
Speaker 1:So then it still goes to one place, but yeah, it's very private data, right.
Speaker 3:Yeah, you can't access your own. You can see it through the app. That's all.
Speaker 1:And now, in you as well, we're strengthening the regulations around data and AI. You see what I did there pretty, pretty smooth um shouldn't mention your segues. If you want to do it, I know, I know but it's just like, I feel like it's smooth, but it's not really smooth. Um, but yeah, what's what's about to happen, maybe, uh, what is? What am I hinting towards here, mariam?
Speaker 3:Yeah, what I actually a bit gave away in my introduction, so that we are here about the EUAI Act, which is actually in a lot of news, a lot of people getting a bit maybe anxious, but some maybe getting excited I don't know about the things that are going to come within the next couple of months.
Speaker 3:So, to generally explain, maybe, what is the EU AI Act and why is it important. So the EU AI Act is actually, yeah, the act on AI, to make sure it's on the continental level, so for the EU only, and these are the world's first AI rules to be put into place. And the big thing about this one is that almost 90% of the AI systems that are created would fall under like I can say 99, actually but a lot of the AI systems that are created would fall under like I can say 99 actually but like a lot of the AI system that are currently being generated or created or tested or at least employed into the market are going to fall under it. So it's very important for the companies to say, okay, it's going to come and we should be aware of it, but also see what the impact it's going to be on their AI tools that they've already created and put into production. So yeah, impact it's going to be on their ai tools.
Speaker 2:That they've already created and put into production. So, yeah, and what I understood is that you have this a bit of a risk-based analysis, like yeah some of those, like the 99 that will now fall under this regulation, like some of them will be will be classified as high risk and that entails like a huge amount of compliance regulatory stuff that you need to do right and that's yeah, that's a, that's something that has also a huge amount of compliance regulatory stuff that you need to do right.
Speaker 3:Yeah, that's something that has also made a lot of vagueness, brought vagueness into these rulings. It's based on a high-risk and based risk approach and there are four categories. The first one, I would say, is a prohibited approach. That is also the most regulated one, actually the one that is banned. So if the AI rules are going to go into action mid-June, next month, so mid-next month is the deadline to sign, because at the moment they are translating all the regulations into the different languages of your EU. So once that is done, it's going to come into action, it's going to be published, action it's going to be published and three months after that the prohibited AI systems that fall under those categories would be banned. Actually, it's quite close. So three or two, six months, I think. What kind of AI?
Speaker 2:solutions are we talking about?
Speaker 3:They try to put it into some categories, but still it's a bit concerned to subjectiveness how you interpretate it. For instance, these are also the ones that they believe that they are a danger to the fundamental rights and that's why they said, okay, we are just going to ban it, to be sure.
Speaker 1:Could you give an example maybe, like what would be something that would fall in the prohibited?
Speaker 3:risk. I will give the categories they mentioned and then try to give the examples. So, for instance, the first category that falls on under is like the behavioral manipulation. So if they, if there's an ai system that tries to exploit, um, some vulnerabilities of people that could be based on age, on physical or mental disabilities. So meaning, like, say so based on age, for instance, like a voice activated toy, that can lead to that, if it's activated by voice, that it can lead to dangerous behavior for the baby or for a child using it. So, really, the exploitation of the vulnerabilities based on age or on mental or physical disabilities, that will be banned.
Speaker 3:Okay, yeah, that's one of the categories. The others are also for the real-time remote biometric categorization, for instance, meaning that if you are trying to mass surveillance that's the article that they also put in there, a large number of groups without their consent that it could potentially fall under this category and that's also something that they would want to employ at the Paris Olympics, yeah, so I put it here for people following us on the live stream.
Speaker 1:I also put the article here. Title reads the 2024 Paris Olympics AI mass surveillance under the upcoming EU AI Act.
Speaker 2:So this is one of the categories mass surveillance under the prohibitive use yeah, so and yeah, mass surveillance without consent. So, and I guess that is where the vagueness comes from, right, like if you, if you point a camera at the street and you have, like a classification is this a person, is this an animal like, is this already under the I act and is this prohibitive use?
Speaker 3:yeah, because it's remote, um, because that's like there are some words that you really have to pay attention to. So, for instance, they say biometric, um, yeah, identification is okay, but if it's real time and remote, then it's not okay. So there are really like some things that you specifically have to focus on, and if you don't, you could be like oh, mine is okay, but actually it's not. So there are like some things that are, but they're also very specific.
Speaker 1:So, yeah, it's hard to know, like okay, but this is a small thing, it's unharmable we would say but then this is not going to take place, right, because the Olympics is going to be after the UAC.
Speaker 3:Well, it's under discussions, it's not. I think I'm not sure if there's already some decision made on it, but this is a point of topic, a topic on discussion, saying okay, is it okay or not? But these are the things that we would say ah, baby, it's good because it's for security and safety and like that's something I like, but it harms the fundamental rights of privacy.
Speaker 1:So the EU-AI Act is defined, right, we have the categories. So the discussion is whether this should fall under prohibited risk, which means it's not allowed, or if it should not fall.
Speaker 3:So the discussion is more like it's not to shape the EU-AI Act, it's more to kind of this case yeah, under which category does it fall? Yeah, okay, and I think it's devised, not 100. Clear is also the different parties that are involved. Um, the parties creating the eu ai act are most mostly legal, legal people working on it and people working on the on the tools and stuff are more technical side. So people look on the same stuff but from a different angle, and that also cause a lot of discussions.
Speaker 3:So for others they would be like yeah, but this is not 100% what you are trying to say. But the legal would be like yeah, but this is fundamental right, and you didn't think about that. And I think it's going to be some topic like when it comes into an action, it's going to be like okay, is it an ai system? That's already the first thing, because there are a lot of exceptions as well, because if it's used by the law enforcement might be okay, but if it's used by a private sector, it won't be okay. So stuff like that, that's still.
Speaker 2:That's still there, it's defined, but still every use case has to be checked out for that and we're probably gonna have to have legal cases going to court to actually establish some precedent on what are the boundaries on. Uh, yeah, how do you classify this?
Speaker 3:yeah, I would say that these rules that they try to imply are a first good first general ground rules and to be able to specify okay, does this fall under this or not? Yeah, a lot of low suits. Another important one that also falls under prohibited risk is the emotion recognition on the work floor, but also on the universities or schools that feels very specific. Yeah, it is.
Speaker 1:But what is it? So? You mentioned emotional recognition, emotional recognition but what is a?
Speaker 3:so you mentioned emotional recognition. Emotional recognition like, like. Sometimes I don't know if you've seen like. Sometimes I see videos of um like a camera, looking at your employees and seeing how you're happy or not, yeah oh, really yeah that's shouldn't be allowed if you're not happy enough, you get fired.
Speaker 2:That's how it works.
Speaker 1:That's what they're trying to protect here if you're too happy, we're paying you too much yeah, you lower the optimization, yeah, yeah.
Speaker 2:So that's very specific that they added something like this. So, emotion detecting in the work environment or schools.
Speaker 3:Yeah, but except if it's like really the main exception, like if it's something that is really causing harm and you detect it and it's used for better good, then it's okay. But if it's used for commercial purposes, like improving your salary or giving feedback on ah, I see on the cameras that your happiness level is 50% up of the time that you're here then it's not okay what if it's used for like psychological safety? Give an example.
Speaker 1:I don't know hypothetically, you were clearly thinking of something no, I don't know, uh, if someone feels discriminated at work or something okay I mean, this is an like emotion that you would look sad maybe not sad. I mean maybe sad. It's a very blank, blanket right, but maybe um tense or fearful or something, I don't know because I think that's I know. I know when you when you go to the concreteness and the necrete, that kind of falls apart like cameras everywhere, and then say yes, for your own good. Yeah, that's the thing.
Speaker 3:No, I think in that case, but I also because there's a lot of cases I'll be like, yeah, but that's good. But I think the idea of AI Act is also to give more transparency, more disclose that these decisions are happening and they're not 100% happening by the AI system. If AI systems say, ah, you're frustrated, and your manager comes to you, ah, I saw that you're frustrated by the results of the cameras that we implemented, I think that's not okay.
Speaker 1:Maybe. So this is AI Act, so there is a system that detects that. We also have the GDPR. That's just something more mature. If we don't have an AI system system but we just have. Like bart's is just when bart's in his office alone, he just has multiple screens with cameras everywhere and he just kind of watches people. It's like, oh, he looks a bit I don't.
Speaker 2:That is a hypothetical situation.
Speaker 3:You're making a statement we wouldn't turn there on, yeah yeah, yeah, like on the other side right. All the cameras?
Speaker 1:No, yeah, it's very hypothetical. It's a bit absurd even. But if someone is just watching the employees, is this like oh, and now it's okay Because there's no AI? Does it fall under GDPR?
Speaker 3:I think it would fall under GDPR.
Speaker 2:The GDPR stuff Because it's still and also on Ethical A lot of local law around workplaces.
Speaker 1:But then, if this already falls under the GDPR, do you still need something like this on the AI Act? Like, if I say I cannot record employees, even if it's on the workforce without their consent, do I need something to say, oh, oh, yeah, and you can now use that the gdpr is more around like like, if we take the quote-unquote, high risk in gdpr is pi data, personal identifiable information, um, and it's not necessarily on the use of this.
Speaker 2:Is it high risk of it or not? Like it's more like a type of data that is classified, as you need to be very cautious on this and like the ai act, in my understanding, is more like what do you do with this? Like, what is the what?
Speaker 3:is your, I see but I think that's a good and fair question. Um, yeah, how you gather your data? Because the training data, for instance um, that is also something that is now more like okay, if you are training your data within a web, scraping of the, the facial that you find on the internet, that's also like something that you cannot do. It's also prohibited now to use that as your training data, where you just scrapped all the faces of the internet and you created a facial recognition database in europe.
Speaker 1:That's not allowed no, no, in the eu ai, I guess yeah I don't know about, because I also see like all this gen ai stuff right, and I'm wondering like they get very good results right. Like if you say you never used anyone's picture ever to get that, I'll be very like surprised there's a whole other discussion but also based rules on rules like.
Speaker 3:The second category is then the high-risk category.
Speaker 3:That category, for instance, is saying okay, if you put something, if you, if your ai system is high risk, you can use it, but it's still you need to generate, put it into the eu database of the ai systems. You need to put more like assessments and other stuff, because it's more related to safety. And one of the things that they said is, for instance, there's already a European product list that has very specific rules on those products, for instance, toys, medical devices and stuff like that. So now in that category they said, okay, everything that falls under that product list and if they use an ai system within those products, it would be a high risk. Then you need, again, very specific reporting. Uh, yeah, there's like an assessment that needs to be done by a third party and stuff like that. So they really incorporate the existing rules with the rules of ai, saying, okay, if you use ai in the toys or in medical devices or in even lifts, that needs to be registered and accounted for very specific rules. So they try to merge different laws with the AI.
Speaker 2:And I think from the point of view as an AI practitioner, I think this is the difficult category because it requires a lot of compliance, regulation, it requires a lot of admin and we have the. I think the discussion is there ongoing like whether or not LLMs by default from a certain size are classified whatever you do with it, but by default because of their nature, are not a high risk category and lms are becoming like a big part of a lot of ai solutions, so uplifting a lot of these solutions by default to the high risk.
Speaker 3:I think that is a bit the the discussion that have been going on in the last month, right and, if I understand it correctly, lms um, I don't know if I can say, but it's more also general purpose ai accounted as a general purpose ai and that's actually they put in a third category. Uh, so not the high risk, but like limited risk categories, and then it would be so it wouldn't include, like the, the general purpose ai with llm, so it has like different functionalities.
Speaker 1:You can't specifically say it's for emotional recognition they call it foundational models, and yeah, yeah, that's true yeah that's also the name that people in the industry use, right and if this is by default in another category.
Speaker 2:It's in another one, like a bit lower and I think in the original draft it was it was by default high risk. It now gets lowered, but I think from a certain size, I think they even define some parameters, something then it becomes high risk, something like that. Yeah, I think there's a cut off so in general, the law is now.
Speaker 3:So the general purpose ai with the high impact foundational models um, they fall under the limited risk, okay. And then the here it has really like okay. So they have enhanced transfer transparency obligation. So they need to provide a technical documentation. They want to.
Speaker 3:They have to provide the training data okay, and also that's a lot yeah and also have to say okay, like, take into account the copyrights and the watermark. So they really have to say okay, everything that you are, uh, that is generated is by ai. They have to really watermark that, that this is AI. You cannot just say okay, just like chatbots, for instance, or deepfakes, you have to market with AI generated.
Speaker 2:Okay, and do you know where the responsibility for this compliance is? Like, let's say, I built a chatbot on top of OpenAI's GPT-4. Like, do I have to provide this extra transparency or is it the provider of? Or can I say, yeah, I'm using this supplier, which is OpenAI. It's up to them.
Speaker 3:Yeah, but that's also something that has been into discussion since a while now. The parties that the UAIX specified are the providers and the deployers parties that you aix specified are the providers and the deployers. The providers would be the one that created and initially distributed the ai system, like the developers of the ai model would be then the providers of that ai model and then you have the deployers deployers with the people who use that model and deploy their application on it okay.
Speaker 2:So in, in my thing, I would be the deployer if I create a chatbot. Okay, even though I'm the one having the relationship with the end customer.
Speaker 3:That actually yeah, yeah okay but I think that's also it. So they would be like, okay, um, if you have, if you um yeah, I don't know about the if you have like something you based on something of the chat gpt, but you put it into your application, I think both of the parties have to put to enforce or to respect the regulations, but at some different level. So if you put your application but you added or made the change to the model into some aspect, you add other training data to make it more specified to your use case.
Speaker 2:I need to be able to show that data Only what I added. Yeah, only what you added.
Speaker 3:The one that created the initial model would have to also follow the regulations, but specific for his or her use case.
Speaker 1:I have a question. You mentioned like anything that is generated like is produced by these models. So you mentioned like deep fakes, but I imagine if I tell the judge pt to write me a blog post, that also needs to be explicit. What about the things that are like almost co-creation? Right, like I have? Judge pt writes a draft, but I'll change this, and there are tools as well. You can see how much of it is the original judge pt answer. How much is it that you edited? Um same thing for images? I guess like what, if I have this, but then I added the image quite a bit Is there an in-between kind of that? You mentioned that there is an influence of the model.
Speaker 3:But I think that's also. I don't think they put a percentage on. If it's 50%, then you would have to watermark it and otherwise not. But I think the general rule is that it's well-known, it's more transparent and that's the general rule of the AI, that it's just transparent. So if your article is 80% generated by a judge, apt, and you read it once and you agree with it, put it in there. So I think the transparency, that's the road to follow in this kind of cases.
Speaker 1:And I have another question or clarification, because what I understood in the beginning is that the AI act is more on how these models are used, but then we also had a discussion that LLMs, or foundational models, by definition they already fall in the category, regardless of how they're used. Is this? Did I understand it well? Because to me those two things don't fully match. Do you see what I'm saying?
Speaker 2:well, I think that was the original draft, but I think um. Let me just explain that this is now in its own category yeah its own category with the limited risk.
Speaker 3:Yeah, okay okay, cool. And then there's another category no yes, well, um, it's a done, it's a minimal risk, and everything that you do internally within your company, so you create a spam. Um, it's a done, it's a minimal risk, and everything that you do internally within your company, so you create a spam folder. That's a bit smart. That would be limited risk there no, so it's like last category, doesn't that?
Speaker 3:doesn't have like hard uh obligations on it it's like the rest the rest yes not rust yeah but there are like a lot of things that are actually good, because now there's a definition of eu. It's not clear on that percent, but at least there's a definition of the ai act in a like written down. There are rules, um, that can follow. So it gives a bit of clarity. But it's also like containing a lot of nervous anxiety by people also because the cost of the compliance rules and the documentation that you would have to do is huge.
Speaker 2:And you need to get audited right. You need to have a third-party auditor to.
Speaker 3:Well, yes, to make sure that you're compliant, I think, Because I don't think these rules are complicated, so it's not that if someone did a study then they know it all. Yeah, random ones.
Speaker 2:Interesting to see Indeed Because of the examples you gave, like also with spam, like almost everything will be under there. It's indeed like you said, like the 99%.
Speaker 3:Yeah.
Speaker 2:And even in the limited risk, like if I make a spam filter that detects, Meriloifies merilo spam like very discriminatory but as long as low risk, as long as it's just let's wrap this up if you don't put it company wide.
Speaker 3:I think it should be okay, but I think a lot of people are also going to be surprised by how much of the AI systems are used in their day-to-day life.
Speaker 2:That is true.
Speaker 3:Because now you need a consent saying is it okay if I keep using that Normally, if these rules are put into action, you're going to get a pop-up in two years. Maybe Is it okay if we use this information for that and if you click on okay, then they're.
Speaker 2:They are probably compliant plus, from the moment you actually use it, you have like a watermark somewhere that's yeah transparently shows that this is yeah something indeed like in terms of like, understanding, like this decision is actually based on some ai logic, like it's today.
Speaker 1:We don't know right well a lot of the things this reminds me a bit and I don't know if I'm. I don't know that, I don't remember the details, but like something that for images like on instagram whatever everything that was edited there was a filter that people were obliged to include it there, and I think it had something to do with like unrealistic beauty standards and all these things and also how it affects the younger people. Like just make a disclaimer like yeah, this was this image was altered digitally, not sure actually how, how enforced it is.
Speaker 2:I hope that it will be interesting to see, like in a few years from now, how it's applied.
Speaker 3:But I think because we can say, because we are in data and we are really in the field, for us it's like but you know, this is AI-generated, you can read it. You can read this as chat GPT. But I think a lot of people who maybe don't use chat GPT even they would be like okay, for them it would be a big, big, big surprise.
Speaker 2:And.
Speaker 3:I think it's also for those people to protect them.
Speaker 2:I think the biggest negative reactions on the AI Act is that it might prohibit innovation, even though I think there is a clause in there that says, like some r d initiatives are excluded from this, yeah, but that will still because it needs a lot of of administration, other things, stuff like this like we'll slow stuff down will make us less competitive on a global level um, yeah, on that as well, I'm wondering if it's not my idea because it's written here, but uh, it also discourages big companies to operate in the EU.
Speaker 2:Yeah, yeah, good question. I think the EU has its volume with it. Its volume and prosperity. That's why large companies still operate here under the GDPR, under the what's the new one? The DMA and DSA. It's almost never seen anyone retreating. What you do see is that it takes sometimes a bit longer for them to open their services in the EU especially when it comes to GNI and stuff.
Speaker 2:But at the same time, I very much hope that this will also create a bit of a framework to understand about the potential risks In the Netherlands a bit of a framework to understand about the potential risks.
Speaker 2:I think in the Netherlands, a lot of people will know the child benefits scandal a few years ago the Toeslagenaffaire in Dutch where there was an automated system that I think calculated no, no, it's a long time ago, but it calculated more or less like how many benefits should you get, how many child benefits should you get on a monthly level, and it was incorrect.
Speaker 2:The system it wasn't AI, part of it were AI driven and it's meant that when it came out like it was corrected and a lot of people had to pay repay, like up to tens of thousands of euros to the government and like it really led to bankruptcies and stuff like that a big, big impact. The government resigned, um, but this is this is an example of an, of an ai-based system that had huge negative impacts because and and hopefully, with something like the ai actually would have had this step up front where you know, okay, I'm going to create this, this, this will be classified as high risk something like this um, you need to create transparency. How are you going to do this? You get audited on this and hopefully would have avoided that, and that's a bit the question right, like are we going to avoid these type of cases which have?
Speaker 3:for instance this case would have fallen there, I think social scoring, which they even put in prohibited ones so they're not allowed to use it, that's, that's next level, then yeah, because that's also the case. They didn't use any human intervention or supervision and stuff like that. That's the thing that I think I actually really want to focus on that every decision that is taken that there's still some human impact in it, and that's their idea.
Speaker 2:Okay, so that also would mean that that's things like creditworthiness scores and stuff like that. Like you like, if you, if I calculate a creditworthiness score, you're gonna go for a loan.
Speaker 3:Like if it's 100 based on ai and then no one from the human I think, but it's. That's also the. The categories are not exclusive, okay. So I think it's like either on unprohibited one, because social scoring based on your personal characteristics and assigning them a score based on that would like, for instance, the social or the economic that would harm the social and economic opportunities of that person, would fall under prohibited.
Speaker 3:But I think you can a bit of deviated from by saying okay, every decision that is taken by ai is still, uh, overseen by a human but I hope they do it and don't just say it, but that's the idea behind it. That's like all the decisions, but that's something like what you just mentioned. In netherlands, that doesn't happen ever again because, if someone would have taken into account the decision that were made and why they were made.
Speaker 1:Yeah, yeah, yeah, which I guess is like always human in the loop approach, right, joseph, is like my perspective also, like thinking of ai, use cases and the trajectory of a company you usually you, you would see use cases, that ai is more an advising role yeah but the ambition was always to have a fully automated ai automated decision yeah, but I think with this it's almost like unattainable right, because a lot of it well for these type of decisions, right like where there is a big impact on someone's life.
Speaker 3:I think that also helps on social level, because that also gives a bit of job security for people, because it'd be like, yeah, but now everything is automated, but yeah, still someone has to with the specific skills and expertise and the people having so much experience but okay, they are not in IT, but still they have experience of the scope of the thing that they're working in. That's still useful and that's also positive in what I see. Yeah, indeed, Job security is also something that's still useful, and that's also positive in what I see.
Speaker 1:Yeah, indeed, Indeed indeed indeed.
Speaker 2:Yeah, job security is also something that's in general like maybe not the AI act, but AI in general often brings up.
Speaker 3:Yeah, but I think AI does help it in one sense saying okay, decision-making, still a human needs to be involved.
Speaker 2:I'm a bit hesitant on that, to be honest.
Speaker 3:Yeah.
Speaker 2:Personally, like we don't have any coal mines anymore in belgium. Right, it's not that we keep to kept them open just for the job security and we moved on. But I think the major difference here with ai is that it's going very, very, very, very fast, much, much faster than people can adapt to. I think that is. That is the what is specific to the situation where we would grace the challenge in brazil.
Speaker 1:I was watching like on the news and they said actually ai created jobs because they were asking people to label stuff sure, okay and I think it's like, yeah, okay, this is not the job you want to do, but I think it's also yeah, but if you're in a position where you don't have a job like I was in cabo vision on holidays and I was we were walking on the roads and the guy was like, very like, it's like islands, right, so a lot of mountains and stuff, and then we're walking on the roads oh yeah, my grandfather built this. And I was like, oh, that's horrible. He's like no, no, everyone had a job back then, you know. So it's like the bits.
Speaker 3:You have to see that, okay, these 10% would be sold with it, but the rest?
Speaker 1:Yeah, I guess. For me, the main takeaway is like AI will make changes, for sure, jobs will not be the same, for sure, but that doesn't mean that there will be less jobs, right?
Speaker 2:I think there will be new jobs, but, like I said earlier, the landscape moves very quickly nowadays, sure.
Speaker 1:I think it's just an accelerator, right, because if you look at over time job, jobs naturally change, right, like they're not going to be static forever. You never like kind of have one profession that you did the same thing today, same thing as you did 50 years ago, right, it's just that. I think now the pace of change, we expect it to be much different because of ai.
Speaker 2:Yeah a podcast will just be bots chattering amongst each other exactly virtual avatars with your virtual, your favorite, uh, personality.
Speaker 1:You know, that was the whole like abraham lincoln kind of thing. You know, maybe you want to hear him I'll use morgan freeman's voice for my oh yeah, that's a good one okay, cheesy much.
Speaker 3:Your voice is great so the people that their new part is the ceo?
Speaker 2:it just got awkward yeah, maybe.
Speaker 1:Uh, we are in the data space. What does this mean for us?
Speaker 3:I think a lot um, it's a lot um in the of. I think, not just in data, but also the AI, like, because the data scientists, also the data that we work on that is used in the training and all the documentation. It needs to be a lot. You need to know what you're creating. Does it fall under the AI, yes or no? Does it to know what you're creating? Does it fall under the ai act, yes or no? Does it? Um? Okay, so does it fall under the ai actor or not? So I think a lot, of, a lot of things are going to happen. Like, even for data, if it's used under um, if it's used under a training data set, then it needs to know where it came from. Was the consent there?
Speaker 1:um, yeah, yeah, it's kind of like, in a way, it's kind of what, uh, gdpr did, in a way, right like there's a, now there's another thing that we know we have to comply, so we need to keep an eye out for it, um, but I'm also like uh, I'm also wondering if, um, now there's like all these categories, and how can we prepare for these things?
Speaker 3:Because I feel like there is.
Speaker 1:We do have a quote-unquote legacy, we do have a whole bunch of use cases, we do have a whole bunch of these things that we need to transition into this framework. So how can we prepare better for this?
Speaker 3:I think at our data unit, at Data Strategy, we are already working on creating an introductory presentation for not just for data routes, but also externally for the people who work on an AI system to start creating an introduction. So I think a lot of people they have heard about AI, they have heard about that, it's risk-based approach and stuff, but still a lot of things that are not known because, uh, I think when I mentioned the categories, there are still so many rules that I just didn't like you can't go through them, um, by yourself.
Speaker 3:So getting a general introduction of it is already something, um, that you're working on and I think it's a good start. But besides from that, um, I think, if you like, if your department, uh you know that it's okay, we are creating a lot of AI systems, then it's also good to have kind of a checklist. That's also something we are working on, on creating a checklist to say, okay, answer those questions. Does it exploit vulnerability based on age? Also give examples, because vulnerability Make it tangible. Yeah, because vulnerability based on age. What does it?
Speaker 3:say so we try to make it more to create questions but also provide examples, provide use cases, also some made up or given examples within the EU AI itself. So these are two things that we try to work on. So create an introduction, but also try to create a checklist. That would be helpful for our AI unit then. So if they are working on an AI system that they can say, okay, I'm going to use this kind of data, I'm going to use this is the functionality that I'm going to incorporate, then they already have a general idea of, okay, it might be high risk. So I need to be sure what I do is, since the beginning, very documented, very well thought out, that I'm not just creating stuff and then in the end be like, oh, maybe I should have thought about the other stuff so that's what we try to create a data to really have a like a guideline on uh, to quickly see like what, what category are we talking about here?
Speaker 3:yeah, like you said, we are making it more belgium approach. So making, making, putting it maybe into Mobi already in a higher level, just to make sure that okay, that you're 100% sure that you are compliant. So maybe a more risk-averse. How do you say?
Speaker 2:it Okay, okay.
Speaker 3:Yeah, risk-averse approach Okay okay. So maybe making sure, okay, if it's already, if it's in high risk, if it falls in minimal risk or limited risk, that we may say, okay, maybe you should check these things to be 100 sure. Okay, so that, like you know, we don't want to have the case where you're like, ah, it's not high risk, so I can do la, la la yeah, that's what you mean with a bit more bells.
Speaker 2:Okay, I was thinking are the waffles in there somewhere?
Speaker 3:okay, cool, more risk covers Cool.
Speaker 2:In the meantime, we'll let Marilo go for a bio break. He's jiggling around in his chair. Thanks for the announcement and he's going to share something on the screen for the people that don't see this. It's the first commissioned music video based on Sora OpenAI's video generating model. It's by an artist called Paul Trillo, which I don't know. Does anyone know it?
Speaker 3:No no.
Speaker 2:No, no, we don't have any sound with it, but we'll add it to the show notes. It's called here, but it's a little. Added to the show notes. It's called here, but it's. It's an impressive video. So this is fully generated by, so and it's uh, the. It feels a bit um dreamy, I'd like to say. But if you would have shown me this, even two years ago, I would have said someone actually made this and added some weird effects to it yeah, I agree.
Speaker 3:But yeah, I agree, or they, because you, still there are mirrors and the people running beside it, and then you sometimes you see the reflection in the mirror, but the people is, the person is not there. Yeah, so then I would think, okay, they really. Uh, the technician was very good.
Speaker 2:That's what I would think and what you do have here and I think you still see that a lot also in non-sora ai generated videos is like this very linear effect like let's, let's zoom in on the center of the screen and then see what, what, what is the next scene that gets generated. You see it very much here as well. I think in general, it's still hard to uh to prompt, like certain camera angles and stuff like that, and really have that natural, natural flow what I really like is like the body and the faces of the people yeah you don't see that that's generated.
Speaker 2:That is very good like and that used to be very, yeah, very present, like you, uh, yeah, I have the feeling, like it's only like a year ago, that, uh, you would really have to squint your eyes to see, oh yeah, this is a person that is running, that is like, even for people now make mistakes in photoshop, that you think that doesn't look like a person with hair.
Speaker 3:It's like is it a normal person?
Speaker 2:existing person exactly in the meantime, I'll go, I'll try to even they put babies in it, so they try to make it also.
Speaker 3:It's cool. Yeah, because I would think. Yeah, you need a lot of data to generate such kind of to train such models, like the baby photos. I would think that they're not that much in there. Yeah, normally it shouldn't. There is a rumor, but there's no official information. That would think that they're not that much in there. Yeah, normally it shouldn't.
Speaker 2:There is a rumor, but there's no official information that it's uh, that they used a lot of youtube data youtube data.
Speaker 3:Yeah, yeah, the?
Speaker 2:there's their. Uh, yeah, I forgot the name, their cto, I think they're. They kind of messed up an interview on it. But did you use YouTube? Yeah, I'm not sure.
Speaker 3:Yeah, but YouTube for video. That's like a huge, huge data source to train your models, and I'm just waiting for the time when YouTube comes out with something Themselves. Because, I think YouTube has a very good scope for that.
Speaker 1:That's true. They have a lot of data.
Speaker 3:Like. Every video that exists is probably on youtube, not just to share with everyone, but like also, you can easily share it. They have the right database for it.
Speaker 2:I think they do it in a very I haven't really seen the result of it yet, but they're doing it for music generation as well, like you can find some snippets, but the way that they do it is that they collaborate with known artists and use their data, so it's also very, very transparent and that's why I think it's easier for them. If they do it that way also for video generation, it's easier for them to get a bit of support from the community, from the creative community, on this.
Speaker 3:But I really like the idea that you because at some point there were discussions about ah, the song is created by the voice of, but that person didn't know, didn't got any copyright and stuff, and that's just not fair. Yeah, so it's nice if, like it's like in collaboration with someone, so that they can make sure that the person get the things that or get something out of it at least.
Speaker 2:Cool. Okay, let's do. We have some other points.
Speaker 1:Yes, we do Well so many. Usually we have to cut them short.
Speaker 2:Yeah, I think that's the case today as well.
Speaker 1:Indeed, but maybe it's time for a hot take.
Speaker 2:Yes, let's do a hot take.
Speaker 3:Oh, hot, hot, hot, hot, hot, hot, hot, hot, hot hot.
Speaker 1:So today we have two. Well, we can choose one of the two. I'll save one, the other one Maybe I'll go one that is more timely. Internally, that was More timely.
Speaker 2:Yeah, there was a discussion. I'm wondering what you're going to do now. There was a discussion. Maybe you need to take the video off the screen now.
Speaker 1:Yes, yes, my bad, now we can see us. Yay. Internally, this poll was launched just now. Actually, I won't share the results yet with you, but the statement is combining SQL, so it's very technical. So get ready. Sql in PySpark and Python in Databricks is the most powerful tool in the data space. It's a big statement, huh.
Speaker 2:It's a big statement, yeah.
Speaker 1:Maybe can we dissect that a bit for people that are not super familiar. So SQL is like a database relational thing. It's not super new but it's very popular very well. It performs very well, very distributed. Pyspark is the Python flavor of Spark, which again is like a distributed computing. Spark is actually Scala. So PySpark is the Python flavor of Spark, which again is like a distributed computing. Spark is actually in Scala. So PySpark is the Python version of that. Distributed, because if you have a lot of data you need to tell computer A to do the first 10 rows, computer B to compute the second 10, et cetera, et cetera. You need to have some coordination. That's what PySpark will do. Databricks is one of the cloud providers as well, but they also have a lot of open source stuff.
Speaker 2:Scala is originated from Databricks, correct? No, spark comes from Databricks.
Speaker 1:Spark comes from Databricks, yeah, yeah, yeah, they also do a lot of stuff in ML flows also from Databricks, and the statement here is that SQL and PySpark, so Python in Databricks, is the most powerful tool in the data space. Maybe, mariam, do you agree with that.
Speaker 3:So you can use normally separately. So you have notebooks in Databricks, I guess. You can use SQL and you can use PySpark already. So I think that's SQL, a lot of data. The novice people even know SQL, so that's already good. Pyspark is also like okay, if you start, pyspark has a lot of SQL in it as well, so that really also helps. So yeah, but it's done, databricks later. I think SQL and PySpark are already very good.
Speaker 1:So you would take the Databricks part out. Well, to me it's a bit like are already very good. Okay, so you would take the Databricks part out Well.
Speaker 2:To me it's a bit Like I think I always have difficulty with the most Like the most is always debatable, right, like what is the most. But if you take the most out like combining SQL and PySpark in Databricks is a powerful tool in the data space it's also a bit of a very obvious statement, right. Yeah, a powerful tool in the data space, it's also a bit of a very obvious statement, right. Like SQL is a de facto language of anyone in data. Spark in general is probably the most well-known large data transformation-ish tool and Databricks is probably the biggest cloud provider for that. So it's a bit of an obvious statement, yeah, it's a bit of an obvious statement, I think the only thing you can debate is is it the most learned?
Speaker 2:There are also good tools that have similar feature sets.
Speaker 1:And this is also brought up. For example, you also have SQL plus native Python data frame transformations, right? So Snowflake has the Snow SQL and Snowpark. So you have again SQL and Python Fabric. Have TSQL, spark SQL, pyspark, starbust. Have Trino SQL, pystarburst, duckdb have the DuckDB SQL, but Arrow Polar, same thing, gcp data, bigquery, dataproc right, like I think this is a very identifiable pattern the SQL and the Python stuff, right. I feel like. I feel like it's today. It's what you would expect Of a data platform.
Speaker 3:Yeah, but I think the difference Between all of those and with the Databricks Is PySpark.
Speaker 1:Yeah, that's true.
Speaker 3:Arrow, py, starburst, snowpark, sql. Okay, there are some differences Like some differences like some writing, but normally generally don't shoot me if I'm wrong in that but like sql, can you can say okay, you can read sql, even though if it's like t sql, if it's three, you can read it's very interpretable, even though it's a different dialect.
Speaker 2:Yeah, it's very interpretable, that's true but by spark versus I.
Speaker 3:I don't know, arrow data broke.
Speaker 1:I've not ever used it, but I think I can read by spark yeah, yeah, yeah data yeah yeah, I think spark is just the language to, to to uh interact with your processing engine yeah, yeah, yeah, yeah.
Speaker 2:So, but I think maybe that processing engine, the api, that is very specific to that processing.
Speaker 1:Well, sql is very generic but I do think we kind of agree that today, if you have like a transformation pipeline and this is my opinion, so curious what you think go for a sequel, see if it meets your needs, something most people can pick up. It's usually like easier there's nest, like I think it's harder. I'm not saying it's impossible, but it's harder to to write dirty sql code than write dirty Spark or Python code.
Speaker 2:Maybe there's another hot take Wait tell me again what is the dirty thing.
Speaker 1:So like clean code dirty code, right yeah. It's harder, not impossible, but it's harder to write dirty SQL code than dirty Python code. I don't agree, you don't agree.
Speaker 3:Yeah, me neither, because I think SQL is very you can pick it up very easily. Okay, and that makes it that people pick it up very easily without having to think about the best practices.
Speaker 2:It's like way too easy to make like a query of five pages, yeah. And then this person like knows all the business logic around it but like let anyone else read this and like they will think, whoa, what the fuck happened here? Yeah.
Speaker 1:I don't know, but I do feel like SQL is less flexible.
Speaker 2:I don't know, but I do feel like sql is less flexible. I don't think like you can make this, I think it's.
Speaker 3:You can write everything in a horrible way. Yeah, you can write very stupid queries, they still work. They're still like five seconds or something and you're like ah, this is a perfect query because it's so long, but it works well, but still, it could be a shitty query.
Speaker 1:Yeah, I'm not sure. I feel like if I had to review code or for, like you or someone that just started working your second day on the job and you have to write something python or something sql, or rather you're doing sql, I think your likelihood of doing something that I cannot read and understand later is smaller than python debatable debatable but I would say like if you've, because you bring up sql and python and python to interact with apis.
Speaker 2:I would even say go as far today, because I think it's a logical statement to say you use it sql and python if you come from data engineering in the last five years. I think today what you might want to explore is maybe I can do everything I want to do just in sql yeah, but that's sql has come along like no. The underlying database to sql have come a long way but why would you say s and not Python?
Speaker 1:only Pure Python, and that's the thing For me. I say SQL because?
Speaker 2:Because SQL will be there anyway on your storage level and Python just interacts with that.
Speaker 3:And SQL is more. How do you say more yeah, more attractable or more agreeable if I just say more People know more SQL than they would know Python? And also, if someone wants to learn coding, I think it's easier for them to start learning SQL. So it's more yeah.
Speaker 1:So it's more like because Python is a generic language.
Speaker 2:SQL is very specific to.
Speaker 1:So that's why it's SQL, but it had nothing to do with what's the likely outcome of that for someone with the same level of experience. Let's say Because I do feel I I know you disagree with me, but I think we agree that sql first and then python for the things that sql cannot do, because sql is specific, it's more rigid, and then you can go on data related yes, but I do maybe agree when that is maybe.
Speaker 2:Uh, it takes a bigger learning curve to write nice python, because python is a general purpose language. You can do much more than there's much. It takes a bigger learning curve to write nice Python, because Python is a general purpose language. You can do much more than there's much more ways to F up.
Speaker 1:But that's what I think. And that's why I think about dirty code Like you can mess it up, or you can have something that has a huge like has a nasty bug, that is very like, you know, cryptic.
Speaker 2:It's a general purpose language.
Speaker 1:Indeed, but that's what I meant. It's a general purpose language Indeed, but that's what I meant. It's like dirty code is you can have dirtier code, you can have dirty code with both, but you can have something unmaintainable more easily with Python than SQL. In general, if I have to close my eyes, I have this I have SQL scripts and I have Python scripts.
Speaker 3:I would expect that I'll have a harder time maintaining python than sql don't necessarily agree, but yeah, well, if it's focused on data aspects, then also because you can use multiple stuff and you can start writing your code in py spark and then you load it to a data frame and then you are doing changes on the data frame and then you are again writing it into. I think like the functionalities is also bigger than I do understand somewhere at this point, because the different functionalities that you could do and different libraries you could use.
Speaker 2:There are more ways to mess up.
Speaker 3:Yeah, more ways to mess up. Exactly that's the best.
Speaker 1:Okay, this was a more interesting discussion than I had anticipated. But, yeah, if anyone wants to voice their opinions as well, if anyone wants to voice their opinions as well, if anyone wants to tell me in the chat that I'm correct, feel free to do so now.
Speaker 3:That's very bold.
Speaker 1:Yeah Right, flood of comments Wow.
Speaker 2:So many people.
Speaker 1:Do we have the cricket sound or no? We should have for these occasions Okay, but cool, interesting discussion. By the way, the within Data Roots only three voters, but everyone is neutral. So no one agrees, no one disagrees. It's a bit of a lame outcome for this hot take, but anyways, it is what it is. But thanks a lot everyone. Thanks, mariam, thanks for joining.
Speaker 2:Thanks a lot for joining.
Speaker 1:Curious to. Maybe you can do a follow-up later on the AI Act, like what it actually came out. I feel like now there's expectations, right, I think, for us data practitioners.
Speaker 2:It would maybe be good to maybe at some point go a bit more in detail on the framework you're creating to assess AI use cases.
Speaker 3:We are still working on it Very cool be very cool, but thanks a lot.
Speaker 1:Thanks for shedding light on this. Thanks, bart, welcome. Thanks, alex, thank you.
Speaker 2:Thanks everyone on that note you have taste hello I'm bill gates, I would. I would recommend TypeScript.
Speaker 1:Yeah, it writes a lot of code for me.
Speaker 2:And usually it's likely wrong.
Speaker 1:When are we going to film you frolicking around in the meadow?
Speaker 2:Congressman, iphone is made by a different company and so you know you will not learn Rust while skydiving.
Speaker 3:Well, I'm sorry guys, I don't know what's going on.
Speaker 1:Thank you for the opportunity to speak to you today about large neural networks.
Speaker 2:It's really an honor to be here Rust, rust Data topic Welcome to the data Welcome to the data topic Rust Rust.