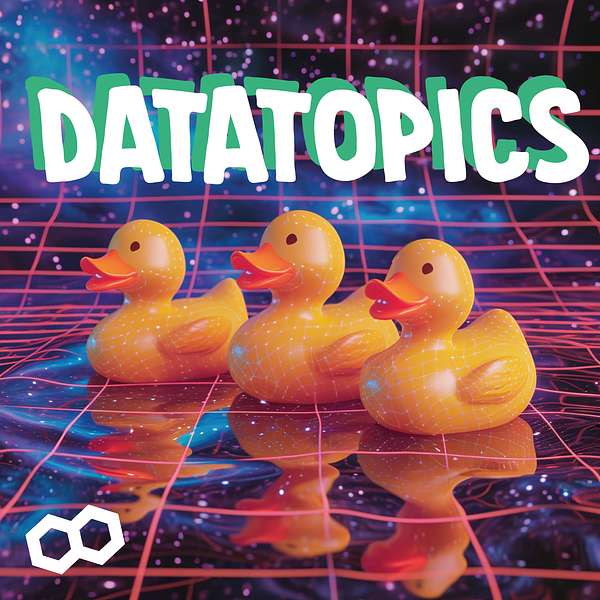
DataTopics Unplugged: All Things Data, AI & Tech
Welcome to the cozy corner of the tech world where ones and zeros mingle with casual chit-chat. Datatopics Unplugged is your go-to spot for relaxed discussions around tech, news, data, and society.
Dive into conversations that should flow as smoothly as your morning coffee (but don't), where industry insights meet laid-back banter. Whether you're a data aficionado or just someone curious about the digital age, pull up a chair, relax, and let's get into the heart of data, unplugged style!
DataTopics Unplugged: All Things Data, AI & Tech
#46 Debunking Devon, Exploring RAG Frameworks, and Tech for a Better World
Welcome to the cozy corner of the tech world where ones and zeros mingle with casual chit-chat. Datatopics Unplugged is your go-to spot for relaxed discussions around tech, news, data, and society.
Dive into conversations that should flow as smoothly as your
morning coffee (but don't), where industry insights meet laid-back banter.
Whether you're a data aficionado or just someone curious about the digital age,
pull up a chair, relax, and let's get into the heart of data, unplugged style!
In this episode, titled, "#46 Debunking Devon, Exploring RAG Frameworks, and Tech for a Better World", our special guest Martin Van Mollekot adds a rich layer of insight to our tech stew, covering everything from 3D-printed humanoids to the harmonious blend of AI and music, all while exploring how tech is cultivating a better world.
- 3D Printing: Martin discusses building a humanoid using resources from Thingiverse.
- AI Generated Music: Exploring Udio, an AI that not only composes music but adds vocals to match your taste.
- Devin Debunked: Unpacking the claims of the "First AI Software Engineer" and why it's not quite time to worry about AI taking coding jobs.
- GPT-4 Over Humans? A critical look at whether AI could replace junior analysts in the current tech landscape.
- The Data Science Dilemma: Is Data Science Dead? Discussing the evolution and future relevance of data science, with Zapier highlighted for its accessible toolset.
- RAG Frameworks Galore:. Discover the evolving buffet of RAG frameworks, making data handling smoother – and whether they're up to the hype: Ragflow, Pine Cone, Verba, and R2R.
- Tech for a Better World: Martin shares his personal story of how computer vision technology can aid farmers in managing their livestock.
- Hip-Hop and Generative AI: How generative AI is stirring up the music industry & tips from Bart on reproducing hit tracks.
- The Low-Code Revolution: Martin shares his insights on the rise of low-code/no-code platforms in data management.
You have taste in a way that's meaningful to software people.
Speaker 2:Hello, I'm Bill Gates.
Speaker 1:I would recommend TypeScript. Yeah, it writes a lot of code for me and usually it's slightly wrong. I'm reminded, incidentally, of Rust here, rust.
Speaker 2:Rust, congressman. Iphone is made by a different company and so you know you will not learn rust while you're talking.
Speaker 1:Well, I'm sorry guys, I don't know what's going on.
Speaker 3:Thank you for the opportunity to speak to you today about large neural networks. It's really an honor to be here.
Speaker 1:Rust Rust Data topics. Welcome to the data. Welcome to the data topics podcast.
Speaker 4:Welcome to the Data Topics Podcast, April of 2024. My name is Murillo. I'll be hosting you today and I'm joined and he's back. Can we get like a quick applause for his return? The one, the only the one, the first, the last, making the one and only Bart.
Speaker 2:Smits, you make me blush, murillo. Yeah, happy to be back, hi everyone.
Speaker 4:Yes, we missed you. We missed you. Missed you bart. And last but definitely not least, we have martin. So quick intro about martin he's 0.908 sam tall. Uh, which makes him taller than me, which I don't know how I feel about that. I always thought that you know, but anyways, I won't linger on that too much. Uh, which makes 1.8815 e to the minus 16 light years, 45 trillion GFIs old. That's 1.3922 e to the 52 playing time. He's the data strategist. But when he's not strategizing data, what is he doing? He's farming. He's a part-time farmer.
Speaker 2:Cool.
Speaker 4:In fact and I think we need to get ready the applause again Recently he just got a bull named after you. Is that correct, correct?
Speaker 3:And he arrived yesterday.
Speaker 4:Have you ever had any animal named after you? Bart?
Speaker 2:My grandfather's parquet was called Bartje Bartje, yeah, because of you, or it was just a coincidence and you'reier Barthier.
Speaker 4:Yeah, because of you, or it was just a coincidence and you're just trying to claim it. I think it was a coincidence, Okay, Okay, awkward. No, I think the thing I'm I was most flattered was when someone they I drew something on a piece of paper and I'm not an artist like Alex with the painting. I drew something a piece of paper, but he liked it so much that he tattooed it on his body.
Speaker 4:Yeah, it's true this is next level yeah, it's not like having something named after you, but you know, it's still uh, and it's maybe even more you think yeah, but the bully's there for only three years, and in two years it would be like this guy will be in the retirement home and uh yeah, yeah, I mean your stamp, the murillo stamp.
Speaker 4:Exactly what was it that you drew on a napkin? I guess I'll let your imagination, uh, wander there. Wow, um, as the aforementioned before, he's a data strategist. I'm just saying this again because on the intro sheet there's twice there as well. So I think you really wanted people to know that you're a data strategist. Mechanical engineer yeah, I'm actually a mechanical engineer too, by education. I never worked as a mechanical engineer. Exactly the same.
Speaker 3:There we go. Specialized in fluid dynamics, never worked in fluid dynamics in years.
Speaker 4:Did you like fluid dynamics Kind of that's a polite no.
Speaker 3:Yeah, you're a runner, I try to be okay, I feel like you.
Speaker 4:You're very bold when you're typing, but then, as now I'm bringing it up.
Speaker 3:It's like yeah, yeah, I run more and more, but I'm still quite far. When you look at other people at data woods, yeah, yeah, bart actually is a big time runner.
Speaker 4:Well, I know you're gonna say I used to be normal back in the days. Back in the days before my uh running retirement yeah, but you still run though, so like still retired, like retired athletes I run without any objectives, okay? Oh really, then this is the best way to look at it but didn't you talk on the podcast that you had like a goopy thing going, not goopy um chp t coach thing going?
Speaker 2:it's past. It's past. It's past. You retired, after then retired again.
Speaker 4:Okay, I sometimes come out of retirement okay, just to yeah, I'm still here, guys, just kind of like an artist like the singer.
Speaker 2:That reese is yet another album yeah, this is really the last one.
Speaker 4:This is the last one, it's like at some point still finds fun in that, then are you saying you're like the michael jordan of running, like you'll be tired and come back?
Speaker 2:is that maybe overstating this a bit too much?
Speaker 4:okay, uh, what is the longest you've run?
Speaker 3:uh, it's a marathon. Okay, that's, that's, that's, but I'm yet to run an official marathon, because it was during covid, so nothing was organized and I had to do it so we have to take your word for us yeah, you can take my strava for okay, okay okay, the european championship marathons is coming to uh next year and you can join this uh as well, everybody, can join us. Is it still time for me to I?
Speaker 2:think it's now like you need to register. Now. I think registration is like the pre-registration closed, but I think the registration is open now I look at it once in a lifetime opportunity and it's in one year exactly, I think. I think it's march of uh 2025 march starts and not 100% sure the direction. I think it starts in leuven and of embraces okay, sounds nice you're also a 3d printer.
Speaker 3:That's weird yeah, it's a weird statement identifies a 3d printer I'm a 3d printer user okay, yeah okay you print 3d stuff exactly, yeah, since a long time yeah, for the people following us.
Speaker 4:Ah, we are also on live stream, of course. We're back on the live stream a new time. Tuesdays, uh, afternoon, end of day. Um, we are on youtube linkedin x, twitch, all the, all, the, all the works and for some reason, bart's mac is like really acting up with the with the automated reaction.
Speaker 2:So when you make gestures like this, I'm doing like the rock horns, like you get these automated reactions yes so that also sometimes shows a thumbs down, for some reason yeah, I'm not sure there should be like maybe we should turn them off. Right, thumbs up. Can you turn it off?
Speaker 4:I can turn it off. Okay. We have thumbs up, thumbs down, hearts and the rock. Yeah, there's also heart, but for people following the live stream, if you have a macbook for free to try as well, I'll turn it off. It should just work 3D printer.
Speaker 2:Do you do this a lot?
Speaker 3:I used to.
Speaker 2:Now what did you?
Speaker 3:3D print I have it for now nearly 10 years, so I 3D printed a lot of things, yeah, and especially during my mechanical engineering courses, I had to print a lot of parts for different projects and it was. It was really nice to to use, but mostly for prototyping, when you had to imagine new machines, new systems. It's really good to prototype even small mechanism with small gears and etc. It works quite, quite well for that.
Speaker 2:What's the most memorable thing you made with it?
Speaker 3:I think it's a project. I plan to build a full humanoid robot with it and the plans are….
Speaker 2:A full what sorry? A full Humanoid, Humanoid, oh wow.
Speaker 3:I did the arm only, but it worked.
Speaker 1:Okay.
Speaker 3:The full hand, the full arm and you could really control each finger individually. And it was quite scary and quite nice. But to have a limb just there on your desk that's moving and the hand is moving, is a strange feeling.
Speaker 2:And did you design it yourself or did you use? Something like what's it called a big open source library.
Speaker 3:Yeah, I used Thingiverse.
Speaker 2:Thingiverse, yeah, yeah, exactly.
Speaker 3:It was on Thingiverse and it was called InMove. You can still find it. But I had to custom some pieces because my 3D printer was a bit sketchy sometimes, so I had to adapt some pieces.
Speaker 2:And what did you do with this humanoid hat on an arm?
Speaker 3:It was just a proof of concept and it's super fun to do, okay okay.
Speaker 4:Never heard of Thingiverse. It looks really cool. There's quite a lot of stuff there.
Speaker 2:Yeah, it's very big.
Speaker 3:It's really specific for 3D printers like myself, Like me.
Speaker 4:That's really cool. That's really cool, maybe for the people. I know people are dying to hear more about Martino, so I'll also put him Martino the bull. There we go. That's today, actually Today, quite recent, yeah, oh, wow, I can see the resemblance. I see why they named him after you, mm-hmm.
Speaker 3:Yeah, I see that he's quite young, only 18 months, so still a bit small, but oh, wow, already really good looking yeah handsome little fella I'm proud of him.
Speaker 4:Oh okay, cool, cool, cool and uh, how's your, how's been your weekend? I see you have a. What have you been up to this week? There's something to play. I think, bart, you need to play, otherwise you won't. Yeah, your audio is connected. No, like there's a notion do you want to try?
Speaker 2:oh wow, sorry um not sure that is actually gonna work I need to share. You need to share your screen and then play it oh yeah, I can share my screen and then unmute, unmute, that okay yeah, there we go.
Speaker 4:Apologize for that. Just make this a bit and let's see, yeah, I don't think we hear anything, but I think it's our setup. I think the people from the live and let's see, I don't think we hear anything, but I think it's our setup. I think the people from the live stream may be here, but what is this? What are we supposed to hear? Let's imagine that.
Speaker 3:So the idea is it's a new AI. It's kind of similar to Suno. If you know it, it's similar to Suno but it's better. It's a new version. You can just ask for any song and it will make you the song in a few few minutes, few seconds, and it's super impressive. I don't actually know how they do it but with vocals, right with vocals, with everything you have.
Speaker 3:The you have, yeah, it's. You can really understand what they are saying and the music is really good and I think in less than one year you could really generate some playlists that you would truly enjoy and don't listen to regular music too much anymore.
Speaker 2:And I think they also very recently last week maybe they announced that they have new funding. Very recently last week maybe they announced that they have new funding. Something like this is really I'm guesstimating now a little bit. I read this somewhere in the feed I think they raised something like 10 million, which is small in all these news that we get these days.
Speaker 3:but still big for something very niche, right For this one or the other one. Three years ago, Udio yeah.
Speaker 3:And yeah, they say Udio and Suno lead the battle of the AI music generators, but with this one. Because on Suno they clearly state that they only use a publicly available music and if you try to put lyrics of an already known music, they they will just refuse to produce it. But on this one, on youtube, on the the main page, you can find, like we prof, we prof versions of really well-known music. So that's a bit that's different and I'm not sure if it will last for long that they are able to take those well-known music and just change them a bit yeah, let's see let's keep the gen ai music topic because we will come back to it today, right, bart?
Speaker 4:we will yeah uh, how has your weekend been, bart? How's the past two weeks? Actually? I feel like you're mr worldwide. You know mr 305, is it 305?
Speaker 2:you know people I know, but I don't know um my weekend was good, but I've indeed been away for uh, two weeks, I heard it was not I went away for one week to portugal to family very nice, nice weather uh to the algarve region uh, very beautiful region, very beautiful coastline uh. And then last week I was like I was, I was uh here, but I was in our uh netherlands office.
Speaker 4:Okay, that's why actually I think, yeah, I heard all sorts of things. I don't know parts is in the netherlands, parts don't buy to work like he's a business trip and it's like portugal was like part open an office in portugal. Um, glad to have you back, thank you, glad to have you back and how was your week?
Speaker 2:you've been off also, yes, part of last week right, I have been off.
Speaker 4:On thursday, friday I went to romania um I will be getting married later in the year, uh congrats, congrats.
Speaker 2:We already knew, yeah, yeah did the world already not sure, not, uh, keeping a secret, let's say.
Speaker 4:But uh, I don't know, I'm not actively advertising either, right? But so my partner wanted to go to Romania to look at a wedding dress. There there's a designer that she really likes, so I also went for a suit. So we did that in one day, and then we also just did some tourism. My family was there, my brother and his partner were there as well, so it was quite nice. It was quite nice. It was really warm in Bucharest. Actually, it was like 20, 25 plus, I think. Okay, yeah, maybe I'm making it up, but I think it was really warm. It was really warm, even for them.
Speaker 2:they're like whoa, it's really warm, and did you find what you were shopping for?
Speaker 4:Yeah, so it's a custom-made suit. Yeah, it was the first time I did anything like that, but I thought it was like a custom made. It was like you kind of have like a template and they kind of just tweak it. But no, it's like full from scratch, right. So I don't know how it's going to look like yet.
Speaker 2:They walk in with a cheap like is this the wool you want?
Speaker 4:Yeah, Like that's raw material, exactly. It's like that one just wait a few months when it's big enough and then no, so they came with a. Yeah, I chose the color and everything, but I feel like I'm very, if you know, do you know the color insights? I know, bart, you know this. You know the color insights, the personalities you have, like people that are blue, red, green, I feel like you know. So people that don't know, so I'll explain to you. But for everyone that doesn't know, so basically you can think of like different personalities, and usually the blue is the person that is very, uh, needs to think things through, very analytical. The red is someone that is very decisive, very assertive, um. The green is someone that is like a caretaker, let's say. And the yellow one is, uh, like someone very energetic, that wants to be involved, wants to be part of the group, etc. Etc.
Speaker 4:Um, so this is just a very brief summary and I'm very blue in a lot of ways, like I really need to sit down, think it through, but then we have like a one hour meeting right with a guy, so it's like do you want this, this color or this color? And it's like they're similar, but I'm like, oh, I don't know. Ah, maybe can I still change it afterwards. Can I answer to you later? It's like no, because it's going to change the price. You need to pay half, and so, uh, I really had to push myself to really have a decision on the spot, but I think it was all okay. I think it was all okay. I see what you have there in your laptop back there.
Speaker 2:I don't think we're gonna have time to cover this one okay, okay, interesting, I'm gonna interesting, uh interested to see what it looks like me too me too.
Speaker 4:Ah, me in a suit. Maybe we'll come next next, next week, I'll come on a suit for you, bart. Okay, okay, deal, deal, deal what you two know what. Okay, all right, um, enough with the chit chat. What do we have for this week? What do we have, maybe? Uh, we can start with. You mentioned jenny. I we mentioned devin in the past. You were here, right, bart, uh no, you're talking about devon.
Speaker 2:Oh, you weren't? No, uh, I don't think so, at least do you know what devon is?
Speaker 4:martin? I think so. Devon is, uh, basically what was the company name? I forgot it's cognizant labs or something. They basically, um, claimed that they had the first ai developer. Well, so it was basically an ai, yeah, cognition. So basically they, uh it was. It's a, it's an ai that can actually go to stack overflow, that can actually do a lot of all sorts of stuff, and the idea, the promise, is that, um, this ai bot can actually make you money, like with upwork. So they actually had a video on upwork, so we didn't dive in as much detail. Why am I bringing this up? Apparently Devin has been debunked, so I'll share this, even though I won't play the video. I'll share this tab.
Speaker 4:This guy from Internet of Bugs, he did basically a thorough review on the demo, right, and throughout the video he actually makes a lot of very good points, right? So first of all, he says that the, the devon, is very impressive as an engineering bot, let's say, um, but they're still overselling it, right? So that's the claim of the problem they also mentioned. So this is the, the demo. They go to upwork, which for people that don't know what upwork is, is like freelance software gigs, let's say and then they pick this one and this is what Devin supposedly finished the job and would be able to get paid for it, right. So full software engineer, let's say.
Speaker 4:And then he kind of just kind of dissect this a bit, right? So, for example, he points out that this is not a random thing, this is the action cherry picked, this example. The other thing is that the requirements here are very vague, right, and actually what Devin delivers is not exactly what is asked here, right? So, for example, ec2 instance on AWS Again, first thing that, according to him, you should ask is what kind of instance do you want? What's your budget? Do you want to prioritize speed or do you want to prioritize that? Devin doesn't ask any of these questions. This is also something we talked about before. There was an article that coding was never the hard part of AI or of software engineering, that the hard part was actually, indeed, translating what the person wants to what we need to do.
Speaker 3:Right.
Speaker 4:So strategy, exactly, yeah, exactly to do. Right, so strategy, exactly, yeah, exactly. Um, so a lot of these things like, okay, what kind of interface? Uh, what kind of instance? Do you have any budget? What? What exactly data do you expect? Right, and these follow-up questions are things that ai never asks today. Right, like, if you ask something to gpt and it doesn't know what it is, it would just assume stuff, right, um, the other thing, too is like well, this is an rfp, so there's usually a bit of interaction.
Speaker 4:He also talks about, uh, he did the same work that devin did. He made some assumptions here. He actually points out that he he took 30 minutes, like 36 minutes. He did in life coding as well. Devin took maybe six hours and he actually saw this by looking at the timestamps of the demo. Right, yeah, here, right, so again, maybe the app Devin is an AI, maybe it was idle for some of the time.
Speaker 4:And I think the main thing that struck me as well is that in the all Devin working a lot of the times, there's a big checklist of things that it did, but turns out that the list was actually fixing bugs that it introduced. So actually, the the repo was actually super simple to use. That was one or two bugs, I think, um. But then devon is like oh, we did this, we did this, we created this file, we did this, we did this, and it turns out that, like a lot of the stuff that he spent just fixing, it was just recreating stuff that was already in the repo, so he wasn't really understanding what was there as much and just like recreating something, and according to him it's like spaghetti code.
Speaker 4:It was not good quality code. He refers it as code that a C developer would write for Python, so something way more complex than it needs to be, um, and he just kind of fixes that right and then in the end they're going to go. Good job, devin. You know you did so much. That's great, but in the end you have something that you didn't need to be as complex, something that is going to be really hard to maintain. So he maintains that we should be a bit more skeptical about these things, and even for myself, right, because I think we are.
Speaker 2:I think the whole community ran with this, huh.
Speaker 4:Yeah, community ran with this. Yeah, exactly, and that's the thing. So he said the people that built devon, which it is impressive, right, like if you asked him and he says this two months ago what would an ai software developer look like? This is further than what he would have thought, but it's not as close to. Oh, no, developers is going to take all the ai developers are going to take all the upworks work, right, it's not like that. It's not like ai is taking developers jobs or anything like that. We're still.
Speaker 4:There's still ways to go there not yet not yet exactly this was not true that's what exactly for whispering, scammed for devin?
Speaker 4:yeah, yeah, exactly, exactly. Um. But it also made me think right because but it also made me think right Because a lot of people ran with it, like you said, and a lot of people were retweeting, posting articles and all these things without doing thorough research. And even for us, right, we're in a podcast and I think it maybe made me think that we should be more skeptical and really look deeper at all the things that we bring here. I think we usually try to be very careful with how in depth I know about this topic that I'm talking about, so sometimes I do say, yeah, I haven't really looked into it that much, but this is my impression.
Speaker 2:Okay.
Speaker 4:But he really urged people to don't just go and post things because you saw them like really go and look. The information is available.
Speaker 2:How do you feel about that? I feel like you have a. It takes so much time. It takes so much time, exactly. Yeah, that's also my first uh reflection. I mean, indeed I think it's like also it's a fair question, like yeah, but like all the topics that we typically cover, like, if you want to do an extensive review of the sources, and I mean you should, yeah, like, should you, yes, fully agree.
Speaker 3:But it takes like five minutes to say something stupid and two hours to debunk that thing yeah, I wouldn't say the default is just talking stupid.
Speaker 4:But I also think yeah, indeed right, I think sometimes but martin is right I think sometimes it's also yeah, I don't know, I feel like the internet is weird, right, because I do feel like there are people that are going to put a lot of time into really giving you the transparent and as much and biased as possible. So I guess it's also like a skill to know what things you should be more skeptical and what things you should check more, and what things maybe, like there's clearly a financial incentive here, right, so maybe people should be more skeptical on these things. Yeah, I think, um, we're commenting before we went live that this guy one thing it was funny that the youtuber he also. He says that he's not anti-gen ai, he's not anti-ai, he's just anti-hype. He doesn't like hype, so he says he's the wrong time to be alive must be so frustrated for him to just go through the day he looks like he looks like he looks frustrated too.
Speaker 4:So that was, uh, that was quite interesting, funny. But yeah, devin is debunked. We can keep our jobs for now, bart for now for now, but how long do you think it would take before we actually get concerned?
Speaker 2:um well, we've had this discussion before. Huh yeah, but I think our job content or job focus will change yeah, yeah, yeah, long term, still still.
Speaker 1:Yeah, I agree, quite a way off.
Speaker 2:For now, the's a tool, a productivity tool what do you think?
Speaker 3:yeah, and you still need someone to blame if, if there is a mistake. So you want to keep someone, just in case you know what did you do now? Yeah, it's hard to blame gpt or anything, but it's easy to blame mojito for everything.
Speaker 4:So that is true from my personal experience. It's very easy to blame me for whatever happens there. But another claim here the chachapiti is apparently better than you already. I didn't say this. He wrote this. Huh, really, yeah, yeah, you can see the edits.
Speaker 3:You didn't write this. I received it from kevin ah, received it from Kevin.
Speaker 4:What is this about?
Speaker 3:So, yeah, it's a paper that states that apparently ChalgePT can do my basic like junior data analyst job better than myself.
Speaker 2:So like Devon V2. That's a big statement, huh.
Speaker 3:Yeah, which is basically a lot of things that happen between the business part and the the tech part. Um, I hope it's not that true, otherwise but yeah, so, but exactly what? Like is it just any task or I didn't go through so much, but I think it's.
Speaker 2:I think Do you even just like, like, if we talk chat, gpt, like chatopenaicom, like the interface?
Speaker 3:Yeah.
Speaker 2:The technical side of being a data analyst. It's quite good, like with Python interpreter. If you upload a data analyst, it's quite good, like with python interpreter. If you upload a data center, you ask a question, you ask it to generate a plot, it's quite good at that.
Speaker 4:I mean, you still need to come with relevant questions, but yeah, but I still think that the one thing that jni definitely doesn't do is ask follow-up questions.
Speaker 2:If there's anything, no, it's always to me the questions are important thing here. Yeah not how you generate the plot, exactly how do you generate the plot or something that can help with?
Speaker 4:but I think it's asking the right questions is the only thing that is important here I completely agree and I think also translating right, like sometimes, uh, when you talk to someone, they ask you something, and then after I was like oh, actually what you talk to someone, they ask you something, and then after I was like oh, actually what you want to know is this it's not that yeah.
Speaker 2:And I think arguably, I think about it also good if we talk about questions Like you ask a question, it gives you some answers, but you can also ask it like are there any topics I haven't touched upon yet? Like to seize a bit as a brainstorm partner?
Speaker 4:Yeah.
Speaker 2:I've done that a few times as well.
Speaker 4:I think that is also valuable yeah, yeah, I think uh yeah, I think that's something good for jenny, like almost like brainstorming indeed. Yeah, right, the different use cases I feel like there are two different modes. One is like well, three different modes that I use, jenny. I one is like to really just give me the end product. One is really when I don't know what the end product is. I just brainstorm like let's try to, you know, go crazy, or. And then the other one is like when I'm looking for something and I just don't want to read three different articles and just kind of, you know, I kind of know what I want. So it's like if I'm looking something on stack overflow, right, I ask for certain keywords. I kind of know what I expect, but I want it to be closer to my context. So those, I think, are the three main ways that I use AI today Well, coding and all these things as well.
Speaker 3:It's still a lot of unit blocks and you are the one that's really creating the mesh between all those blocks and linking all those unit tasks together.
Speaker 2:I have an idea. Uh-oh, all those unique tasks together. I have an idea oh so the statement chat gpt is better at your data analysis. What if we build a chatbot with your user token? We let chat gpt answer any questions, you get on slack and you just let it run for a week and then either after the week people are who the fuck is this guy? What the fuck is this? Or they will be like martin is is the shit he's like.
Speaker 4:We need 100 martins he always replies within like two seconds. Yeah, this is great so productive I think it would be the first one, though, so, so I think you're safe.
Speaker 2:You could be our.
Speaker 4:Devin, that's true, but Devin for data analyst.
Speaker 2:Would be a good experience, maybe.
Speaker 4:Or maybe.
Speaker 2:Or.
Speaker 4:We have like a live session.
Speaker 2:Yeah.
Speaker 4:Where we have Magda versus Chagipiti.
Speaker 2:That is a bit. You need to be ready for that. Ooh, that is, that's a bit. Uh, you need to be ready for that. Yeah, I mean, it's not me, so that sounds risky, then he's really on the spot, huh.
Speaker 4:Yeah, exactly that's what. I'm here for and then yeah, but I think the thing is like there was actually a TED talk from. There was actually a TED Talk from Kasparov I think he's the Russian chess player.
Speaker 2:Yeah, yeah.
Speaker 4:Like from years ago, and I actually watched it, maybe like last year or something. I don't know if I talked about it before here on the podcast, but anyways, it's super spot on though, because he's talking about how he was the chess champion and then Deep Blue came. And then they like actually he's a funny guy too. He says, yeah, no one remembers like we played twice. No one remembers that I beat deep blue the first time actually, but like when deep blue beat me, that's when the whole world was like, oh, ai is taking over, which we actually had a alpha go moment as well, not so long ago.
Speaker 4:Um, but then he goes on to say like okay, we have compute, we have like computers and we have this um, people still play chess, that's the first thing. And then they started doing these competitions that are humans and computers, right. And he said that the people that would win these competitions are not the best chess players and are not the best computers, the most powerful computers. It was like one regular guy quote, unquote regular with three computers, quote, unquote regular computers. And then he was kind of saying like shifting more towards it's not us versus ai. First he says again, this is from years ago. Ai is not the future is the present. We already have ai everywhere and basically he's saying that it's a tool right where we need to learn how to use this tool, right?
Speaker 4:he says that ai has compute or compute, and we have intention right. So, and I also think it's very valid here, like I, when I was pitching, it was you versus Chachapiti, but I think, hands down, who will win is a regular guy, with Chachapiti just covering stuff away, right, which is the reality of what we have today. You don't need to choose one or the other, right? That's the thing.
Speaker 3:You have to choose the good person that can use the good tool.
Speaker 4:Exactly, it's a different skill, I guess, yeah.
Speaker 3:And you have to see the two as really a system and not something. You have to choose one or the other, just the best combination of both. Completely agree, completely agree. And that's also, I think the quote I put in the first subject, what my thesis promoter always used to say is like AI will not replace people, but people who use AI will replace people who don't use AI. And I feel, like that's exactly on point.
Speaker 4:Amen to that, amen Preach, maybe going further on that path. It is Gen AI assisted as well. But I think it's also true for any profession, right, like technologies evolve and you have to adapt to these technologies. Right, there are new things now and I think for now it as well. But I think it's also true for any profession, right, like technologies evolve and you have to adapt to these technologies. Right, there are new things now and I think for now we're living with gen ai and software development. It's a bit more accelerated, it's a bit more more attention, right. But even the feel like data science, I feel like it's changed a lot, and I think this is something that I personally witnessed with these AutoML frameworks, with these off-the-box models, even including Gen-AI, in the years that I've been working. I noticed that in the beginning we were expected more to actually open a dataset, explore and build custom models and do the A, b and C, and nowadays there's so many things that kind of do these things for you because compute is more available and all these things, that I wonder what's going to be in the next five years. How's data science field going to be in the next five years?
Speaker 4:And I came across this article, which I think there's even a whole medium community low code for data science. The question is is data science dead? In the last six months, I've heard this question a thousand times. Is data science dead? This is from low code data science. So basically the author is just saying there's a whole bunch of people asking about this. Now there's AI. Is it worth to train your machine learning models? Now there's AI. Is it still worth train your machine learning models? Now there's eyes, you still worth to learn python, etc. Etc. Etc. Right, low code ai for our low code, low code data science.
Speaker 2:I guess I don't know um like nine, like uh data queue, like exactly so it's like frameworks that you don't need to really write everything.
Speaker 4:there's a lot of user interfaces that you can drag and drop to connect services and a lot of times you can press a button and train a model and all these things. And I guess what they're saying is with all these things, is this going to replace, quote-unquote, traditional data science? The article go on to go. I think it's like this Knimi, I don't know what this, how do I, how can I Knime, knime, knimi, I don't know what this? Uh, nine, nine, there we go. Um, so I think it's someone from that. So they kind of really focus on this tool per se and they also have with gen, ai and the ui and whatnot.
Speaker 4:But I want to hear from you what is your opinion on this, and I know you have a hot take somewhat linked to this as well. The author's takeaway here is that data science is probably not dead, but it surely is changing. The best data science will not be the one called faster, but the one that can better direct the assembling of the data science project, taking into account the integration data quality which is I think it rhymes with what you said earlier the best data science is the people that use the tools better, but it won't replace them necessarily. Do you agree with this?
Speaker 3:Totally. Yeah, I think it's exactly what we say. What's evolving is actually the tools. The technology and people that can better use those new tools are the people who will be most successful. What?
Speaker 2:do you think Bart?
Speaker 2:Theological evolution I think I agree with what Martijn is saying Data science. If we say data science is understanding data, understanding trends and data evolutions and data, that is definitely not that right. I think the underlying tools are very much evolving. I think also the more typical components also make that it's easier to do these things without having a very specific setup like um. If they have been saved in the realm of low code, for example, like we use zapier, yes, which is just like there is an event happening, do something with it. It has a lot of uh llm integration. Like you, with very limited effort you can do a lot of things, a lot of the quote-unquote data science related stuff where before that, to make that a reality, you needed a very extensive suite, like KNIME or Light Data IQ.
Speaker 2:I mean there are still very good arguments to use those platforms, but I think we are evolving away from where it just becomes like it's not that complex anymore, Like the typical technical setup you have is ready to do a lot of these things more or less out of the box, where maybe because of that, you don't need as specific tooling like you needed five years ago.
Speaker 4:Yeah, so I think I agree with what both said. I think also it's a bit there's a hardship that I noticed from fresh graduates because data science role changes, because now it's not the modeling as much as more the data understanding, I guess. But usually when people well, back when I was a student, most people were really excited about the modeling part yeah, you were gonna be the next.
Speaker 2:Uh gemini engineer, right?
Speaker 4:exactly right, and I think that's a bit of a there's a, it's like reality misconception about yeah, you know, I think what now when you go, when you have more and tooling, you kind of see that it's really more about checking the data, checking that it makes sense, checking with people to see that you're doing what you're expected to do, and less about tweaking parameters, creating all your network. And I do still think that there is a space for people modeling, creating these very custom things. But it's really when you have a very, very niche use case.
Speaker 2:you know, and yeah, but there is a disconnect there, things. But it's really when you have a very, very niche use case, you know um and yeah, but there is a disconnect there, right, like you learn about your network architectures, propagation optimizers, this type of thing, and then you come get into the working force these days with all these models and service and it's just yeah, you need to do a post request. Yeah, very much simplified like a dot fit a dot predict yeah, even before.
Speaker 2:Yeah, it's like use this library and call dot fit on this object even then there was a disconnect.
Speaker 4:Yeah, and I also think that, for data science, is particularly appealing because there's a lot of things that are very empirical, like if you're building your own neural network, it's like, okay, how many layers you put? Oh yeah, maybe you can put 10, maybe you can put 20, maybe this, maybe that. Okay, what activation layers, how many neurons, how many of this? And I feel like I had a lot of discussions with people.
Speaker 4:It's like, okay, why did you put this one in particular? And they were like, oh, experience, which is like I think it's a very polite way of saying I didn't really know. I just kind of have an intuition, you know, and it's, but it's very non-reproducible as well, which I think is very tricky. I haven't heard someone saying that, like data science is more of an art than a science, right, which I thought that was interesting, interesting, um, and I also feel like, because it's easier to build models, data scientists today are expected to have a wider range of expertise, you know, like building dashboards or building simple pipelines, or I think even like the mlop stuff, like putting stuff into production is something that for machine learning engineers, you know it's more and more and more expected now. So I also think that that changed the role of data scientists or machine learning.
Speaker 3:Yeah, it's what they say. It's becoming more and more generalist, but we can also see that with the tools like tools like fabric.
Speaker 4:Yeah so general.
Speaker 3:It's so general and if you are like, you could imagine someone being a fabric engineer and he would just be able to do basically anything.
Speaker 4:Yeah, indeed, and I think, even like an analytics engineer can do quite a lot of stuff as well.
Speaker 2:You need to have the basics in place, business understanding in place.
Speaker 4:Indeed. Stuff's evolving on the hype domain. Yes, yes, yes, yes. And talking about hype, is there a lot of hype still on rag architectures?
Speaker 2:um. I think there is.
Speaker 4:You think there is.
Speaker 2:There is yeah, I put a few in the in our show notes, right, um, and it's just something that I noticed. And, to be honest, if we're being critical about uh, our, our, uh investigative journalism here, like, uh, this is very much uh didn't do much investigative journalism, but what I do see is like almost every other week there is a new RAC framework.
Speaker 4:Yeah.
Speaker 2:Which is interesting and it's very much linked to this is very much hype thing where we're in. So RAC retrieval, augmented generation. So what these RAC frameworks try to do now I listed a few of them to the show notes is rack flow. There is a canopy rack from pinecone, uh. There is verba from weviate, which is maybe a little bit more chat focused. There is a r2r from sci-fi um for rag to riches and uh, what they?
Speaker 2:what they try to do is to, to, and they all have a little bit of a different setup, but to provide you more or less with a service, sometimes really a microservice, that allows you to, in a very easy way, to send some documents to it Please index these for me and that you can then basically query these documents. And it tries to abstract away from you all the complexity routes. You need to have this document, you need to have a Maddox product, you need to have a database, you need to do vector distance searches, and it abstracts away all this logic, basically to allow you to get up and running with Rack queries.
Speaker 4:But do you think it's just because of hype or do you think it's because there's like a little race going on, because people see this use case? Because I think rag is it's a very um. In portuguese we say like sacado. It's like people know exactly what it is like. It's very easy to sell.
Speaker 2:It's like maybe give a small explanation of what it is. It's very easy to sell. It's like a box. Maybe give a small explanation of what it is for people listening that don't know.
Speaker 4:So, basically, you have a lot of documents, or you can think of an easy use case.
Speaker 4:Example is like HR documents right, big company, they have a lot of procedures, and then people want to know exactly what it is.
Speaker 4:So a lot of the times people end up asking like, hey, how do we, how can my holidays be used next year in Belgium, right? So instead of having you going through all the documents or instead of having someone that answers all these questions, you can actually put all the documents from all the company, hr manuals, whatever in a vector database. The vector database would take, basically, will create it, will plot the pieces of text, the chunks of text, and then, whenever you have a question, you will be able to say, okay, this question, you will plot it on the, it will have a numerical representation that you plot and you say, okay, this question looks like it may relate to all these other chunks of text. And then you can actually extract those chunks of text and then you can go to Gen AI and say this is the question, these are the related chunks of text that we identified. What is the answer to this question? And then it will give you a digested answer, exactly what you expected.
Speaker 3:So if your question is not about holidays in belgium for 2024, maybe your question is that, and if you can uh save them for 2025 okay, but how is it different from, like just using chat, gpt, putting a lot of text document and saying okay, based on all the documents I just gave?
Speaker 2:it is actually more or less the same. The problem that we face, and especially that we faced in the past, is that the context of our prompt is limited, so you can't put and maybe with Gemini 1.5 you can, but you can't put thousands of pages in your prompt, simply because there's a limitation of the context length. Or and there's something that there is still a lot of discussion going about if you make your prompt too big, like you see a degradation of performance. So what you do with Rack is that you say I'm going to index all my documents, I'm going to translate these text strings, like Mariela was saying, to embeddings, which is basically a numeric space that you can easily uh compare to something that you're typing in. I want to search for this, uh, so you're going to have this matching of I want to know, um, how much cows I have, for example. We're gonna already segment to the next next topic, how many cows I have.
Speaker 2:And there's gonna happen this search in your backend, a vector search to it's gonna see what is the document that also talks about this. That is the closest to my query. It's gonna take that document and it's gonna inject it in the prompt. So it's still in your prompt, actually, but it's not all the information that you have okay so you make a, make it basically a selection of what do I want to add onto my prompt.
Speaker 3:Okay, through that, yes, but so it's a solution to a technical limitation that we have right now.
Speaker 4:But if we imagine like a context size that's basically unlimited, we don't really maybe because we don't know how good chpd is to find a needle in the haystack yeah so again, I think today this is the very big context window is like something new, so I cannot say with a lot of confidence that that's a problem, but well, it came up because there was this limitation that we couldn't even put.
Speaker 2:If you have like one, if you have the whole wikipedia and you want to ask a question, you cannot just put that in the context and even then, like if, if there would be a good performance, like recently I saw this post uh, someone that dropped all the harry potter books in gemini and asked them to to, like, generate the links between all the characters was extremely good at it crazy good, um. But even then, like, if we would say, like there's no degradation in performance, um, like, it's still very inefficient.
Speaker 4:Right, you're gonna have such a huge query that, uh, that's uh yeah, yeah, yeah, yeah, for sure, and I think, well, pinecone, this is the. I'm sharing the, the canopy rag framework, that's the one from pine pinecone um, pinecone is a vector database, right. So I think that's why it makes a lot of sense for them to share the use case. And what I'm saying here is like this is a very you have the components, the value is very clear. It's very clear for you to say well, before you had to raise a ticket and someone from HR would take 20 minutes, and now you just have this, so you're saving this much money per questions. You have a hundred questions per week, so you're saving this much money per week.
Speaker 4:It's very, very concrete. The components are very well defined, the requirements, what you need to give is like okay, you need a whole bunch of documents. This can be pdf, this can be html, this can be markdown just dump it there. It's like everything's super well defined, right, which I think the other gen ai use cases are not as clear, right so I think it's neat as a back-end for their service or a back-end for your yet another chatbot service.
Speaker 2:Yes, it's super relevant, right? Because as an end user, then you have just a ui, you drop your documents in and then it does things for you indeed, indeed.
Speaker 4:So I think it's like it's very clear. The value is very clear as well, but I wonder if this is just people racing to because they're like, oh, this is very something, we should build something. But if I'm being a bit skeptical, azure open ai has a service like this, right and meaning they have more or something like this in their backend right exactly right.
Speaker 4:So it's like I see all these frameworks and I it's cool to look and see the ideas, but how different are they? I mean, yeah, maybe one optimizes for this, the other one optimizes for that, but, like azure and open ai, there's still the big names in this true right. So for me today if I have a rag use case, I'd say like you're my client, you say, hey, I want to do rag.
Speaker 2:It's like, okay, give me 50 documents, we'll dump it there and let's see if it works like and I'll do it in you know, like an hour max I think, if we talk about open source, because these are open source alternatives, the challenge of open source in this domain is that it is such a high driven domain yeah that you have to see all of these, the initiative popping up, and I have no clue which one of them is still alive three years from now. True?
Speaker 4:yeah, sure, but yeah, I think, because the thing is also it's open source, but the models you're probably still going to host them somewhere, so it's not like you're going to deploy everything yourself. So even if you are taking open source framework, you're probably still going to need a service for the. So it's like it's still like not, it's not like everything is open source, right.
Speaker 2:This one is probably optimized to easily deploy on or integrate with BindCode's service.
Speaker 4:Yeah, yeah, I think Verdict is still out. I'm not. I mean it's cool to see, but I still think it's more like people are.
Speaker 2:I think it's From the moment you say I'm not going to leverage immediately like a managed service like Azure or like OpenAI Studio on Azure. To me it still feels today very simple to build these things. Maybe I'm a bit hesitant to immediately leverage a framework which I don't know what the future is going to hold.
Speaker 4:Yeah, I can resonate with that. I also see like something similar, and I know you're very anxious about the next topic, but I'll just say this Another thing that I also see is like the unstructured to structured. Like you have text and you say, okay, from this text, give me the person's name, age, height, whatever, right.
Speaker 4:So you have like a blob of text and from that you're trying to get the semi-structure kind of thing. And I also see a lot of open source frameworks popping up for this. There's like Magentic, there's. I forgot there was like a LinkedIn post that was listing all that. So, yeah, sometimes I feel like people see this use case and they see that this is Gen AI, it's hyped and it's very well defined and people just really jump on it, but then it's really just a race and that's why you end up so many things.
Speaker 2:Yeah, but cows let's bring the topic back to cows.
Speaker 4:Yeah, yes, yes, uh, unless the farmer in the group, martin. What about cows, cows?
Speaker 2:so that's maybe maybe before we go into the. In the talk I hear you talk about cows quite a bit like. Is this something you think about every day? Is this?
Speaker 3:like an everyday thought. Honestly, I think I think about cows more than you think about ducks, so that maybe says a lot already.
Speaker 4:That is a lot okay, interesting, interesting okay, okay.
Speaker 3:Basically I go every weekend to my girlfriend's place and her father is a farmer and I help him take care of the cows. So, yeah, okay, I spend a lot of time with cows what about tractors?
Speaker 3:I also try to spend more and more time in tractors and I I try to learn how to ride them, but it's it's kind of hard really, but it's really really nice. Yeah, because the first time you go in you just look at the pedals and you have four of them. Oh so it's already strange. What's the x like? What are the four pedals for? You have two brakes actually two brakes, yeah, one for the right part and one for the left part.
Speaker 3:You can break the whole tractor yeah if you're stuck on only one side or you want to do a really, really, really sharp turn, you can break on only one side and turn on yourself huh.
Speaker 3:So it's like if you want to break with both, you need to use both feet to just break no, so it's a pedal that's quite close so you could use one the other, or just press in the middle and use both at the same time and you can already also like lock them together and just use it as one pedal and is it like the order of the the pedals?
Speaker 4:is it the same as in a? Regular car okay, and it's a manual car, then I guess a manual yeah, with 36 speeds, but manual 36, you have six speeds and for each speed, like you have six more degrees. Yeah, all right, but what about?
Speaker 3:cows. So yeah, I just wanted to talk it's. It's a subject on how how you can use someone's interest to convince them that technology is good. Because, as much as I love my father-in-law, he's pretty much an old school farmer, really old school farmer. Like he has a Nokia 3310. And that's like the highest tech he has.
Speaker 2:Keeps working always.
Speaker 3:That's undiable, but like three, three, around two. Two and a half years ago there was big floods in Belgium and he has a lot of cows just next to a river called La Laisse, and La Laisse was crazy, crazy high that was crazy, as you can see this is you took this picture.
Speaker 3:No, but it's just just just that's in my girlfriend's village and it's just just just thanks. That's in my girlfriend, a village, and it's just next to the, the fields where all the cows were, and the problem there was that he couldn't see his cows anymore because of the water there are yeah, there was so much water and he just hoped that they were somewhere safe.
Speaker 3:But he had no idea on how to be sure about it, because his only way to go and monitor the cows usually is to go by by, uh, with his jeep. But yeah, it was completely flood, so I used my, my drone to go and monitor the cows.
Speaker 2:So so nice, this is yours actually.
Speaker 4:Okay, this is, this is. Did you really print anything?
Speaker 2:So we're looking at an image for the people listening. We're looking at an image of a field with cows, with boxes around the cows.
Speaker 3:Yeah, this is after the flooding, but during the flooding they were all gathered up in a small, small island that you had really no chance of seeing from the house from the farm. So you really have to go either by boat, but it's too dangerous, and you saw that also with your drone. Yeah, so I could reassure him and show him actually, and we could count the cows and see that they were all there and they all survived.
Speaker 4:Oh wow, can we get an applause for that?
Speaker 3:What a hero that's maybe part of a reason why the new bull is called martino that that would be it.
Speaker 4:Yeah, he was conceived on that night on that flood. That's really cool.
Speaker 3:That's really cool when, uh, so this, the tell me more, a bit more about the drone and the algorithm and how the cow is like cow detector, like he's yeah, so that was the first step, like the first time he saw that we could actually use technology for things that are actually useful, because he only hear about technology on on the TV in bad stories like AI used for bad things.
Speaker 2:AI is going to take your job.
Speaker 3:Voilà, exactly. So he's not a big fan of technology and then he realized that we could actually do nice things, and the next step is to actually automate part of his job to make his life a bit easier, because, especially at this moment of the year, the, the cows uh, it's the. It's the time of the year when the cows are are born and you have to count them to be sure that they are all healthy and not they don't fall in laless or anything.
Speaker 3:So you have to count them basically each days and it's quite a hard process because sometimes they just run away, sometimes it's hard to see if they are all a gather up. It's hard to count and they are moving and you want to count. And also each time you have to go by Jeep in the field and it's not that good. You can see, the more you pass, the more you you just uh degrade the terrain. You can see, the more you pass, the more you you just uh degrade the terrain.
Speaker 3:So the next step is to do a re, to implement an, an algorithm to count the cows, which is actually quite easy because they are big, brown, on the quite green field, so it's easy to do. And the next step should be for me to implement to a way to differentiate the small, so the cows, from the cows, so that you can say, okay, if there is any, any cows missing, is it a big cow or is it a calf? And then already see if it's something that you expect, because if you, if you lose a cow, then he, she's probably fine, it's probably just in in the bushes. If you lose a calf, that's a bad sign.
Speaker 3:And then you, you should go for it like immediately, oh well, do you need so?
Speaker 4:in the cause you mentioned, it's basically Brown on a green field. Yeah, Do you need machine learning or can you just do something? Computer vision, you know like look at the brown pixels, start green.
Speaker 3:You still need machine learning, because this is the best case scenario that I showed and this is not using too much machine learning. This is quite basic. But if you want to do something that works in less easy cases yeah, you have some works Then sometimes they are just gathered up and if you just use the pixels, three cows which will just count as one and do you know if this is being done like large farms?
Speaker 2:maybe? Maybe in belgium, or farms are not big enough for it, but in texas or probably in flanders. They are yeah, yeah and do you know if they do this?
Speaker 3:I actually don't know, but that's a really good question. You should look it up, the project that they are. Yeah.
Speaker 2:But AI in agriculture is a big thing, right? Yeah, like John Deere tractors being fully autonomous yield optimization. We've done a few projects in. It's already very much influencing agriculture, I guess.
Speaker 3:Yeah, agriculture is just a huge agriculture, I guess, yeah, agriculture is just a huge industry in the world. So as any industry, now it's also has its share in AI.
Speaker 2:Super interesting. And is it like flooding, like is it something that still happens, or was it only like two years ago, when it was that extreme?
Speaker 3:Only two years ago that it was that extreme for multiple reasons. That's management of the of the gates watergate yeah and also it's it's happening more and more with with climate change, because the family of my girlfriend is in the village for like three generations, something like that, and her grandfather only saw one or two floods in his life, and now it's happening like every two or three years yeah, major one yeah yeah, I wasn't.
Speaker 2:uh, two, three months ago I had a trail run close to the samoa. It was also water was very high and there was. It was walking next to a field and it was completely flooded. It almost looked like a lake and there was like a small sign going just above the water and on the sign was construction plot for sale and I was thinking they're never going to get this sold.
Speaker 4:Just take a picture. I'm good, cool. And while we're talking about beef, what is this hip hop beef that you're?
Speaker 2:Yeah, this is another type of beef.
Speaker 4:What is it about?
Speaker 2:It's about a lot of kerfuffle in the hip-hop world.
Speaker 3:Okay.
Speaker 2:A lot, a lot, a lot of kerfuffle in the hip-hop world. You're a hip-hop fan Marilla.
Speaker 4:Yeah double.
Speaker 2:You know what is bad I?
Speaker 4:don't know. I mean it's written on the notes here.
Speaker 2:It's about GenAI, so Are you a hip hop fan, martin? No, I don't know. I'll maybe give a very short introduction. Then I'll come to the actual point, because I don't think we should debate too much hip hop here, right? It's unplugged, so but how I understand it. Yeah, won't go too much into it, but Kendrick Lamar dissed J Cole and Drake. J Cole responded Okay.
Speaker 2:With a diss to Kendrick J Cole, apologized, lost everybody's respect, but then Drake responded. And then the interesting thing was and this is like the first time I see this in discussion when it comes to hip-hop like everybody was thinking because it was leaked drake's, drake's. This was leaked. It was no, nothing on spotify, nothing on his former channels. It was leaked and everybody was discussing like is this janay? Is this actual drake?
Speaker 2:no, no, this is janay no, no this can't be very like, and there was like no consensus like is this real or not?
Speaker 4:okay, did drake react?
Speaker 2:he did not. But then what? Someone with a bit of uh presence in the teaching academics uh confirmed that it was real, so it was actually drake.
Speaker 2:And then a bit of uh, it is being said that Drake uses a lot of ghost writers so he doesn't write his own songs, blah, blah. And then, because it's a big, it's a big thing that is happening now in the hip hop world. People started leaking reference tracks of him, and a reference track is basically let's say I write something for Drake, that I also rap this, these lyrics, so that he knows, like, in what style it should be brought as a reference track.
Speaker 2:So it's like in my voice, but it's like, and then what you typically hear is like uh, this more or less the same song then gets released by Drake or for whoever, for whoever, yeah, so there's currently a lot of reference tracks being leaked. And like on every reference track, because it's something that's not officially published, like there is a discussion.
Speaker 4:Voice replication has become so good that it's become very difficult to just hear the difference so then the debate is is this really a reference track, or is it like exactly drake song?
Speaker 2:yeah, they actually change the voice to exactly, exactly that's very interesting and uh, and it's maybe also becomes more pronounced, because those reference tracks are typically not the best quality. They're not, they're not edited, they're not master mixed um, but also, I think, in general, like a lot of music, auto-tune, like it's not supernatural. So you expect a bit of aberrations, um, but it's the very first time that I hear like like. The default question is not oh wow, there is this thing.
Speaker 4:No, is this real or not?
Speaker 2:which is interesting to see yeah because, we still have the feeling, still very early on and these deep fake level. We have uh suno and uh, what's the other name?
Speaker 3:again now, but um, and it happened also quite similar, like a few months ago, pewdiepie and other big YouTubers do like a review of their whole subreddit with a lot of fan arts. And also like a few weeks, three months ago, we began to see a lot of AI-made fan arts and the question was not, it was not anymore. Oh, that's a really nice fan art. But each time it was like is it a real fan art, is it AI-generated? But each time was it, it was like is it a real fan art, is it ai generated?
Speaker 2:and and then, yeah, people really doing genuine, uh, fan art just lose the whole, the whole value of doing it because so many people just it's weird yeah yeah, I have a hard time forming opinion on this yeah for this, uh, for the arts domain, I guess, yeah, yeah because I think for art let's say digital art, like not, not music, digital art you can still say I use this as a tool, like this is not the final product, I use it as a starter or as an inspiration. I'm gonna work on this, I'm gonna make a final thing out of this. You can still position, make this argument like this is a tool yeah from.
Speaker 2:The moment that you start cloning someone's voice. Yeah, it's more uh, more, much more invasive, right?
Speaker 4:yeah, but then if someone uses gen ai to create a reference track let's say, like there's a gen ai, like I don't know brainstorming kind of thing, and then an artist actually picks it up and makes it a full-blown song, then it will be okay as well, because you need to use engineer as a tool yeah, no never see. I mean, I haven't seen that, but uh, yeah, interesting we'll, we'll.
Speaker 2:Uh, maybe it's awesome stuff to the show, not some links. Um, if you want to get into this, where do you get this news part?
Speaker 4:you're very into it. I see you're like yeah, this guy said this, and this guy said that and this, and everyone's turning against drink I got my sources very curious my sources. Everything's gonna be like twitter, twitter, twitter but we'll have.
Speaker 2:We'll add some links to the show notes if you want to get into this. Realistic voice cloning uh, there's a realistic voice cloning. Uh, there's a? Uh big. I think maybe I should research this also more, but I think rvc2 version 2 is now the biggest model realistic voice cloning version 2 which uses a number of pre-trained models for almost any type of artist or famous person that you can think of. There are trained models that you that are hosted on hugging face, um, so it's actually quite easy to get started with this. I think, if I can give you a tip, let's say, marilo, tomorrow you want to uh hit the market with a, with a drake voice, and to have a real hit like, try to do it in the same style and cadence as drake. Or if you want to impersonate barack obama, you need to use a bit the same mannerism and then you have the best output okay I'll do that noted.
Speaker 2:Maybe that could be a nice project. Try to let you make a song let me make a song.
Speaker 4:I don't know if I'm that creative, you know, no. No, I think you can do it. You're very creative. I mean, you already generated the, the intro song of the pod. You know, no, no, I think you can do it. You're very creative. I mean, you already generated the intro song of the pod.
Speaker 2:You know that was Limit Right up your alley. Yeah, it was not.
Speaker 4:Janiai. It was not Janiai, sure? Maybe you can do that with Janiai. You know we compare the results. All righty, I think that is all the time we have for today. I'm looking at your bar I don't matter.
Speaker 2:Did you have anything else?
Speaker 4:yeah, you'll bring your sizzling take do you want to?
Speaker 3:well, I mean, it's up to you. Yeah, I can just launch it and just throw it and then set fire, and then, okay, what is it? My hot take. Yes, I've added a small word. So I think that low or no code is the future in most case for all parts of data platform solution. And then I added but that's maybe the reason why, on this data strategy, he makes like a very bold claim and then he's like yeah, but maybe.
Speaker 4:but that's just me. What do you think about that, bart?
Speaker 2:We see it was home claim and then he's like, yeah, but maybe. But that's just me. What do you think about that, bart? We see with um a lot on pack. Your answer low code, no code, future for in most case for all parts of the data platform. Um, so even before the whole ai thing, we like low code was to put us all out of a job. 15 years ago, 10 years ago, it never happened. But I do agree, and we had this discussion on the side a bit on low-complexity stuff. So, for example, if we talk about data pipelines, extract, load, transform, extract and load is very simple, like you need to define this is where I get my data, I want to push that data to there. I think this type of stuff where there is no very limited complexity and also meaning like you don't need extensive tests to know whether or not it works, I think that's a very good candidate for low code yeah, I think like zapier as well.
Speaker 4:One of the things I think is nice is that the authentication is super easy, like if I was writing code. I can authenticate Google Calendar to Gmail.
Speaker 2:You don't need to worry about it. Exactly you just click, there's the site boom done.
Speaker 2:I think from the moment that you start to do more if we stay in the realm of data pipelines, like the transformation aspect, um, from the moment that you start doing more complex things there, which you typically start doing right, like you do aggregations, you do cleaning, you do any kind of stuff from the moment that you do that low quotes for one pipeline you understand what's going on and if something doesn't work you can fix it. From the moment you scale it up to hundreds. That's a lot of complexity to have in a visual interface. It's very hard to test that at scale. It's very hard to have a good view on what is happening where in terms of observability. Then I think let's say more or less good software engineering practices, let's have version code, clear tests, a clear CICD for these complex, more complex things, things. I think that is still, for the foreseeable future, the smart thing to do.
Speaker 4:That's my so it is a hot take, but I do, I do.
Speaker 4:Uh, there is also a thing called, I think, system initiative that they were rethinking a bit how infrastructure as code would look like and it's a a bit more low code kind of thing, which I thought it was interesting. So the analogy that really stuck with me is if you go for like 3D game engines, like Unity or something, you do have a lot of the UI, so it's no code at all, but you can still zoom into the code part if you need it. And I think the system initiative it was something similar. So it's like I think they are basically saying, like the Terraform resources like usually have stuff that interacts with each other and having that in a UI is nicer, but then if you still want to go in and drill down, you can still do that. So it's kind of like an in-between space, again similar to game engines. And they were saying that this is probably the next step into infrastructure as code, maybe other things as well, which I thought it was interesting. I thought it was interesting.
Speaker 3:So it's tweakable low-code.
Speaker 4:Tweakable low-code, I guess, but I'm not sure I fully agree. I think it's a For me. I'm still on the fence on this one, but I do think that a lot of people that work today, they wouldn't be excited with this future because it's another topic.
Speaker 2:How sexy is it to drop? And rock Drop, wreck and drop, true I guess time will tell.
Speaker 4:I guess time will tell, and I think that this is all the time we have for today.
Speaker 2:parts gesticulating I told marillo uh, if I really need to leave, I'll start gesticulating and I uh, my apologies, but I really need to leave no, that's fine thanks a lot, uh, for being joining us. Martin, it was very interesting to discuss cows. It was also interesting to hear that your father-in-law named the cow that will impregnate all his female cows after you. It's super awkward, but I mean, that's an, that's an honor. Right, that's an honor.
Speaker 4:Yeah, it got so red, so quick and we'll.
Speaker 2:We'll see what the future brings for low code we'll see.
Speaker 4:We'll see. Thanks again, martin. Thanks again for rejoining us, bart. Hopefully you won't take another hiatus. Yes, yes, glad to have you here. Thanks, alex, for I just see all the sounds and stuff. Thanks everyone for listening.
Speaker 2:See you next week tuesdays yes, and maybe for people because we made some switches, we're now recording, we're streaming on tuesdays right, and we are releasing on wednesday wednesday yes, um, maybe, if anyone has any feedback, send it to respond to. On any feedback, send it to respond to it on any channel or send it to datatopics at datarootsio.
Speaker 4:Yes, sir.
Speaker 2:Thanks everybody for listening.
Speaker 4:Thank you, ciao.
Speaker 1:You have taste in a way that's meaningful to software people. Hello, I'm Bill Gates. Meaningful to software people? Hello, I'm Bill Gates. I would recommend TypeScript. Yeah, it writes a lot of code for me and usually it's slightly wrong.
Speaker 2:I'm reminded, incidentally, of Rust here, rust iPhone is made by a different company, and so you know you will not learn Rust while skydiving.
Speaker 1:Well, I'm sorry guys, I don't know what's going on.
Speaker 3:Thank you for the opportunity to speak to you today about large neural networks. It's really an honor to be here.
Speaker 1:Rust Rust Data topics. Welcome to the data. Welcome to the data topics podcast.