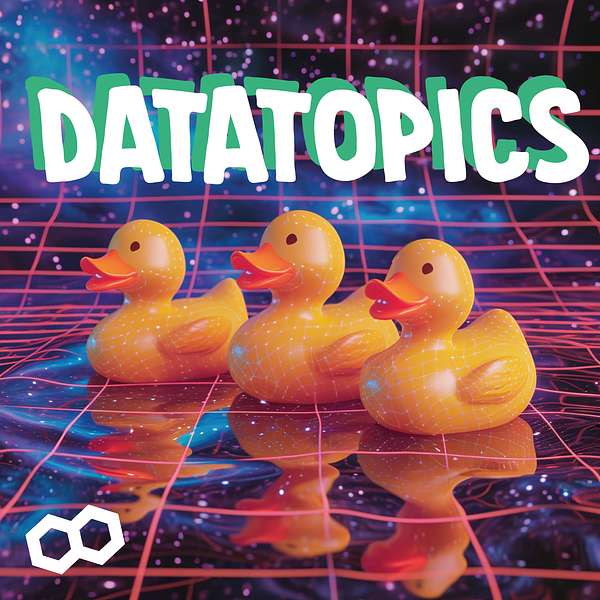
DataTopics Unplugged: All Things Data, AI & Tech
Welcome to the cozy corner of the tech world where ones and zeros mingle with casual chit-chat. Datatopics Unplugged is your go-to spot for relaxed discussions around tech, news, data, and society.
Dive into conversations that should flow as smoothly as your morning coffee (but don't), where industry insights meet laid-back banter. Whether you're a data aficionado or just someone curious about the digital age, pull up a chair, relax, and let's get into the heart of data, unplugged style!
DataTopics Unplugged: All Things Data, AI & Tech
#41 Regulations and Revelations: Rust Safety, ChatGPT Secrets, and Data Contracts
Welcome to the cozy corner of the tech world where ones and zeros mingle with casual chit-chat. Datatopics Unplugged is your go-to spot for relaxed discussions around tech, news, data, and society.
Dive into conversations that should flow as smoothly as your morning coffee (but don't), where industry insights meet laid-back banter. Whether you're a data aficionado or just someone curious about the digital age, pull up a chair, relax, and let's get into the heart of data, unplugged style!
In episode #41, titled “Regulations and Revelations: Rust Safety, ChatGPT Secrets, and Data Contracts” we're thrilled to have Paolo Léonard joining us as we unpack a host of intriguing developments across the tech landscape:
- In Rust We Trust? White House Office urges memory safety: A dive into the push for memory safety and what it means for programming languages like Python.
- ChatGPT's Accidental Leak? Did OpenAI just accidentally leak the next big ChatGPT upgrade?: Speculations on the upcoming enhancements and their impact on knowledge accessibility.
- EU AI Act Adoption: EU Parliament officially adopts AI Act: Exploring the landmark AI legislation and its broad effects, with a critical look at potential human rights concerns.
- Meet Devin, the AI Engineer: Exploring the capabilities and potential of the first AI software engineer.
- Rye's New Stewardship: Astral takes stewardship of Rye: The next big thing in Python packaging and the role of community in driving innovation, with discussions unfolding on GitHub.
- Data Contract CLI: A look at data contracts and their importance in managing and understanding data across platforms.
- AI and Academic Papers: The influence of AI on academic research, highlighted by this paper and this paper, and how it's reshaping the landscape of knowledge sharing.
You have taste in a way that's meaningful to software people.
Speaker 2:Hello, I'm Bill Gates.
Speaker 3:I would recommend TypeScript. Yeah, it writes a lot of code for me and usually it's slightly wrong. I'm reminded into that the rust Rust Congressman iPhone is made by a different company and so you know you will not learn Rust while you're trying to read.
Speaker 2:Well, I'm sorry guys, I don't know what's going on.
Speaker 1:Thank you for the opportunity to speak to you today about large neural networks. It's really an honor to be here. Rust Data topics. Welcome to the Data Topics. Pubplace Ewww. Hello and welcome to Data Topics. Unplugged, your casual corner of the web where we discuss what's new in data every week, from Rust to research or data contracts, anything goes. We're also live on YouTube, linkedin X, twitch, name your favorite streaming platform. What are you going?
Speaker 3:to say I was going to say we're live at 4.45.
Speaker 1:Wow, you just can't let it go, can you? We should have been there.
Speaker 3:Apologies for everybody, all those thousands of people that have been waiting anxiously for the last 45 minutes. Why are we late, marilla?
Speaker 1:Paula wasn't here when I was here.
Speaker 2:I got here and I was like where's Paula?
Speaker 1:So I'm really sorry for Paula. He's a nice guy, he tries.
Speaker 2:No, but I was giving a talk at.
Speaker 1:VB. Okay, fine For the people that are not in the joke, but tell us, marilla, what were you doing? I was invited to do a guest lecture at VB, so it's a university in Brussels and, yeah, normally, if everything went well, I would have been here by 4. Maybe I was maybe five minutes late, whatever, but what can I say? The students just loved me. They were like I can't get enough. I was like I need to go.
Speaker 1:I was like no, we want more real love back. And everyone started getting up. I was like I can't just leave like that, right.
Speaker 3:Every time you do it like okay, now let me do another session. Maybe I'm going to five minutes on Blackboard.
Speaker 1:Yeah, it was just like every time.
Speaker 3:More info. More info what?
Speaker 2:did you talk about?
Speaker 1:I talked about MLOPs. I mean I love a bit of a fluffy topic, so I also dived in a bit toward like deploying models. Right, like what does it mean to deploy a model, batch, real time and something? So there was one demo that I actually did in life and there was one demo that is like pre-recorded with Askinema, so it's just like a record, my terminal, but it's still like a project. So the idea is that they can also go and try it out themselves. It was cool, it was fun.
Speaker 2:It's nice that university are starting to use this type of ops thing.
Speaker 1:I also think it's becoming more and more important for, like industry, right, I think tendency is that AI and ML is getting easier and easier to build, so I think the stuff around it becomes more relevant as well.
Speaker 2:Yeah, yeah, but what I meant is like academia typically lags a bit behind, and MLOPs is also such a new topic and it's nice to see that academia is also catching up with all those new topics that they are aware.
Speaker 3:Yeah, at least that Maybe for the listeners. Paulo has joined us before, but maybe for the people new to you, paulo, maybe you should introduce yourself, yeah.
Speaker 2:So hi, I'm Paulo. Team lead data management at Data Roots. Last time I spoke on this was data engineer, but today we'll talk about data contracts and few things. Let's see where this podcast brings us, thanks for joining us.
Speaker 1:Thanks for joining us again, so you came here once and he still decided to yeah exactly.
Speaker 1:So it says a lot. It says a lot, but really cool. I also before Bart threw me under the bus. Also, he did it on social media as well.
Speaker 1:Today is the 15th of March of 2024. So what do we have for today? Maybe one timely piece of news. Apparently, there was do you know, have you heard of it? Yeah, it's like a model AI something. Anyways, apparently there was a leak from open AI. I mean, and again, what is a leak? Right? Apparently there was a blog post that was deleted that revealed a faster, more accurate GPT 4.5 model, and the thing is also that search engines also indexed these things, and so you can see here like part of the Google search and all these things. Right, and from open AI blog 4.5 Turbo.
Speaker 1:Open AI announces 4.5 Turbo, a new model that surpasses 4.5 GPT4. Turbo and speed, accuracy and scalability. Learn how GPT 4.5 Turbo can generate natural language or code with a 256 K context window so already bigger context window there and the knowledge cutoff of June 2024. So, two interesting things here. I think the knowledge cutoff of June 2024 gives the hint that they will be training on data now, right, usually have the classic code. I'm sorry, I don't have data from 2020. I think it's like June 2021. Last time they had it, no. No, it's May.
Speaker 2:Yeah, but.
Speaker 3:I think that means that it hints that there will be a release somewhere in July.
Speaker 1:Exactly, that's the speculation right.
Speaker 2:So how big is 256 K 256,000. Yeah, 1000. What's the limit right?
Speaker 1:now I don't know. You actually bumped into the limits of the context window before right.
Speaker 3:Yeah, but not at this size.
Speaker 1:But what was the size that you bumped into, like what was the?
Speaker 3:I don't know which model I used back then exactly, but it's significant. I think 16,000 or something Also 16,000. But 256 K, what is it Like? Almost a million words, or something.
Speaker 1:Yeah, also. I think they also mentioned, how this also puts in context.
Speaker 3:No, sorry. A million characters, A million words.
Speaker 1:But the Because Google Gemini also had they had released a model right that they were advertising the context window, so there's also kind of counterbalances a bit that yeah.
Speaker 2:But how big was the gap between GPT-3 and GPT-3.5? Was it like a significant upgrade? I don't remember anymore. Between 3.5 and 3.5, yeah.
Speaker 3:It was a long time ago.
Speaker 2:It's like a.
Speaker 3:Stone Age. Yeah yeah, but it's a long time ago.
Speaker 1:I don't know, to be honest. Funny thing you mentioned like 3.5 is a long time ago, Also in the lecture today Today, so I think 3.5.
Speaker 3:Sorry, it had 4,000 tokens. And then there is a newer, 3.5 turbo, which is 16K. Looking at it here and Let me just quickly see GPT. The latest preview model of GPT-4 has already 128,000 tokens.
Speaker 2:Okay.
Speaker 3:So it will double in size, basically with 4.5. But that also means that we have to wait much longer for Chet GPT-5.
Speaker 1:True.
Speaker 2:But, what is the versioning?
Speaker 1:system there. Does it mean something? Does it mean that 5K is going to be a bigger change than 4.5?
Speaker 3:Let's see.
Speaker 1:To be seen. To be seen, indeed, but I hope you guys. The one thing I mentioned today In the lecture, the. We actually been playing with GPT from opening up a long time ago and actually one use case we had for the Roots Academy was an auto-win joker.
Speaker 3:It was a great joke. It was bad at that point.
Speaker 1:But it made some sense. It wasn't just gibberish, but that was GPT-2.
Speaker 2:Because we showed GPT-2. Yeah it was.
Speaker 1:GPT-2. And it's crazy. We had this. It was kind of okay, we did the presentation, it was cool, but now it's super hyped. If you were to do this again, it would be like top of the charts.
Speaker 3:Right, like the jokes or no.
Speaker 1:Popularity, I guess. I feel like when we made it, we did the project here. We shared it. It made some noise. But I think today GNI is so hyped we didn't even call it GNI back then. I think we just said it was like a joke generator, but it is. Gni, gen jokes. Yes, gen jokes, gen joker, yeah, gpt-3.
Speaker 3:Yeah, definitely, definitely the quality.
Speaker 1:I think from 3.5 and I think 4 became.
Speaker 3:We talked about chat GPT.
Speaker 1:Yeah, that's what I was going to say so, chat GPT.
Speaker 3:I think 4 became multimodal, I think 3.5 is not multimodal.
Speaker 1:But when we had GPT-2, did we have chat GPT or no?
Speaker 3:Back in the day when we used 2. No, I don't think so.
Speaker 1:That's also made a big difference Did somebody have a new UI with GPT-2?
Speaker 2:Or was it only with API calls? No, so they actually fine-tuned it.
Speaker 1:They actually had the model and they trained on top of it Like the Rooft.
Speaker 2:Academy, yeah.
Speaker 1:So I think it was like Hugging Face stuff. Oh, okay, I think again. I was reading and they said they fine-tuned it.
Speaker 2:So I think I think it was my academy.
Speaker 1:Yeah, I think it was the Rooft Academy. Wow, you chose wrong then, I didn't choose, it didn't choose. Okay, yeah, it's cool, but yeah, ai is everywhere, apparently like there are AI software engineers now.
Speaker 3:No, well, I heard a little bit about it, but it was a busy week for me. But you're talking about Devin, right?
Speaker 1:Yes, sir, tell us about.
Speaker 3:Devin.
Speaker 1:Devin wow, who is Devin? The first AI software engineer? So, apparently, like he has a lot of different capabilities, I also want to look into it more, but I thought it was. I already knew enough that I thought it was relevant to share. So Devin is I think they compared it to a junior software engineer they like again can autonomous fix, find and fix bugs and code bases. He can actually open Stack Overflow and go there so he can do different things.
Speaker 3:But basically it's like but Devin is a model, or what is Devin?
Speaker 1:I mean it is, there is a model behind, but I think it's like an agent, so actually, like he's not just responding to requests, but he can give a task and he would do stuff. He would make different requests like just go to Stack Overflow, we'd go to do this. That's what I understood. But yeah, see here, like the production like and who's behind it?
Speaker 3:There's a company behind it Cognition.
Speaker 1:So actually I hadn't heard of them, but I think they were kind of like like they're not new, okay, just that they had their. It says in the.
Speaker 3:Hadrobar on a page just showing that they raised 21 million in a series A Indeed. And just a lot and not a lot in this space, right.
Speaker 1:Yeah, maybe they are new, because if you go to their webpage about us because of Devin, the first AI software engineer, so it's like this is the only thing the company does.
Speaker 3:Would be wondering how good this is at this moment. So that means that you have someone virtual and they can basically code for you.
Speaker 2:Yeah.
Speaker 3:Interesting to understand what the workflow is, but I wish you could probably, like it's going to create something and you're going to iterate over it. You're going to give some instructions.
Speaker 2:What we have already, like some open source library that can do like agent stuff.
Speaker 3:But this is like the next level that is fully implemented for you, I guess.
Speaker 1:Yeah, I think so.
Speaker 2:So it's the paid card, the part of something that is free.
Speaker 3:This is why all three of us no longer need to work.
Speaker 2:Yeah, that's why we can replace this, they will replace us.
Speaker 3:We will do podcasts full time. I will have.
Speaker 1:Devin's for you. We're just laying the disease, but actually I think I was looking here as well. You can hire Devin. So no, if you have evocancies at daydreams we have a pop up on Slack. Yeah, everyone yeah. But I can see, for example, Devin correctly resolves 13.86% of issues end to end of hit the issues we're talking about right.
Speaker 3:It's interesting.
Speaker 1:There's a lot, right, yeah, there's quite a lot, but I mean, I guess, what's the quality of it though?
Speaker 3:Oh yeah, but we're very early right.
Speaker 1:That's true.
Speaker 3:This plus 10 years. That's true.
Speaker 1:So, yeah, I think this is again. It's a never ending discussion. Is the I going to replace us, is it not Right? So, because we also saw previous episode like that, there was a author, like from a blog post, that he was arguing that writing code was never the hard part of his job, so that's why they pay him. So writing code is easy, but now apparently, like this, will do more than just that. So to be seen, to be seen.
Speaker 2:But what did he say was the difficult part.
Speaker 1:So, for example, he says if I ask HGPT write a function about to give me to compute the Fibonacci number you will do it right, but the software engineer, what he's paid for is not to just do it. It's like to ask why do you need this function? What happens if you give a negative number? So like an edge?
Speaker 3:case, to actually make that translation Like what is it? What is the problem that someone actually has? What are you writing a solution about?
Speaker 1:You know. So it's like actually the building blocks. It's not, it's like the easy part of his job. He said the hard part is not, it's before the code gets written.
Speaker 3:So understanding that you need a Fibonacci number. You need that.
Speaker 1:And yeah, where are you going to put it? What are the edge cases? You know? How are you going to deal with them, basically? So that was his point, so he wasn't particularly concerned about AI taking over his job. But things like this, I think, make you wonder a bit more right. Like, is he right, is he not?
Speaker 2:Yeah, and if you consider that in a few months you'll have like GPT 4.5 with a double sized context window, I think it makes sense that more and more information can be put in this Devon.
Speaker 1:Yeah, and I guess my question is I mean Devon, I think be a junior kind of Dev, like you tell them what to do?
Speaker 3:Probably very junior now, very junior.
Speaker 1:But how far do you think we can push this In? Like 10 years time, so a long time ahead.
Speaker 3:It's very hard to imagine. I find it very hard to imagine. Yeah, I think you can take this very far, much farther than we now think. Yeah, I think so too. If you just see the pace of all these things go.
Speaker 2:Yeah, could you imagine like Sora, six months ago Exactly.
Speaker 3:Yeah, exactly. And now this is like this is the minimum that you need to have. Yeah, it's like you're not impressed anymore. Yeah, I'm not a Sora video.
Speaker 1:There was a lot of articles I saw comparing, like computer video generation, Sora and one year ago and the difference is like yeah, it's really crazy.
Speaker 2:It was the Will Smith eating spaghetti.
Speaker 3:Yeah, it was so funny. That's good, that's good.
Speaker 1:Yeah, it's crazy, but yeah, let's see. Yeah, let's see. Do you ever feel a bit of anxiety about your job?
Speaker 3:You could make a Merillo eating spaghetti with the latest. That would be good.
Speaker 2:Let's do it.
Speaker 1:Mix thumbnail of the YouTube. Alex taking notes. She's like oh yeah, I think I can do this.
Speaker 3:I need like 20 to 30 pictures of you to find your model.
Speaker 1:You have more already.
Speaker 3:Oh yeah, that's good to hear Remind me yeah, maybe I shouldn't have. Maybe I shouldn't have.
Speaker 1:Oh, this is going to work. Let's hope.
Speaker 2:Everyone's like oh, you're the spaghetti guy, yeah.
Speaker 1:Let's see. But regardless of how powerful these models are, one thing I want to bring, that caught my attention. You shared the first part. Today they are still and I think that's the difference with Devin as well. Devin is like it's a full autonomous agent. It will do stuff for you. But I think today, judge a PT and all these things, there's still a support Like there should be either, like there should be some human. How do you say checking, not checking?
Speaker 2:What's the word for it.
Speaker 1:Validation. Validation, you know, vetting, you know like there should be a process, because Guard rails, guard rails, I guess. But I guess there should be. The way that JNAIs use today most cases is more of a recommendation, right, like, oh, maybe you should do this and then you kind of make a decision for yourself. And the reason why I asked this is because there are a few research papers that caught my attention Like clearly. Well, maybe I'll, I'll let you want to take this upward.
Speaker 3:I know you were the one that found this, but it's a Well, I pulled over my feet somewhere, and this is a research paper published by Zangbu Young Zulu.
Speaker 2:Okay, I'm going to throw everyone on the bus. We'll tag them on LinkedIn.
Speaker 3:It's about it's about. It's a very difficult title. It sounds very smart, right. The three dimensional porous mesh structure of a CU based metal organic framework aromates cellulose separator. Is this not just chipperous generated automata? I have no clue what this is about, but it is an peer reviewed journal which assumes that peers actually reviewed it.
Speaker 3:And if we go to the introduction chapter of this article, I'm going to link the article. In the show notes. It actually starts a first sentences. Certainly here is a possible introduction for your topic. So my colon and then the action. So this was either it was very clearly generated by something like chipper or they really put it in as a joke and now everybody is laughing at this but it doesn't make the others look very good.
Speaker 3:It doesn't make the whole paper doesn't make journal look very good, because if it's peer reviewed, I mean who's reviewing this? At the same time, you can, you can argue a bit like is it is the content worse for it, right, like, aside from having these artifacts in it, that you yeah, just for me, just like you start questioning, right?
Speaker 1:Because if they didn't even like so, the author didn't review the peer reviews didn't review carefully enough.
Speaker 3:I think that is the biggest. I think the peer review process is the thing that is most under pressure.
Speaker 1:Yeah, I mean maybe, like okay, I was even trying to justify, right, Maybe they're looking at the experiment table, maybe you're looking at this. They're not spending time at the introduction.
Speaker 3:Maybe, but it didn't clearly did it. Clearly.
Speaker 1:They didn't read the first sentence, like clearly, right, but I guess it's like to me. What if the Chatchapiti hallucinate sometimes? Actually, like we shared this internally and there was even the screenshot from the peer review process saying, oh, ai generated Like, make sure to review it, because AI makes it sound very authoritative, but it's hallucinating. And then clearly it's hallucinating. So it's like, and the thing is, if we had a highlighter that we can say exactly what is AI generated Like, there's a tool for that, I think, actually for creative writing.
Speaker 3:It's not very accurate.
Speaker 1:I know. But if we had a tool that could say, okay, this is AI generated, this is not this Like, that would make me feel more comfortable. But they have a lot of references here, like electrode potential, high terrarium capabilities, one, two, and it's like is this Chatchapiti generated or is this they generated?
Speaker 2:It doesn't really feel Chatchapiti.
Speaker 1:You don't think so.
Speaker 2:When you read it. This part, Okay. This part yes. The rest is like.
Speaker 3:I could have. It sounds very authoritative, right yeah?
Speaker 2:yeah, I don't know. You know, when Chatchapiti writes, it's like all this big fancy words that you don't find here. There is a lot of, however, which is quite not basic, but like but I think that it will be used.
Speaker 3:I think everybody accepts at this point, right. But here, like a review process both by the authors as well as the journal, like missed this and then it's not need. Like, like Marilo says, like they missed big artifacts, artifacts like this, like that. They then also look at potential hallucination that they look at the correctness, and I think that is the main concern here.
Speaker 1:Yeah, I fully agree. I think it puts everything into a question mark, right, which is a bit.
Speaker 3:I think one thing we know for sure is that the reviewer is here, will not get anything else to review in the future.
Speaker 1:Now, what if, like you, mentioned the author, what if there was their plan all along, you know?
Speaker 3:to get fame through this.
Speaker 1:Exactly they like.
Speaker 3:now they're in the Internet fame, exactly, yeah, they can say that. Maybe they can put it on the t-shirt famous on X.
Speaker 1:Exactly X, x, fame, fame. Anyways, there's another one, actually another example from a different yeah, this is bad that there is another example.
Speaker 3:Yes, I mean again also peer reviewed.
Speaker 1:Also came through my feed right, so it's not like I'm looking for these things. Two things popped up who knows how many there are Successful management of an iatrogenic portovane and hepatic artery injury in a four month old female patient. Of case report and literature review. Okay, it's a bit funny, like they say, real literature review, but it's a chagapit. So it's like what if you just throw in a chagapit and that's the literature review Anyways? So this is not in the very first, like this is not the first sentence, which I guess it's more reassuring, but you do see here in summary, the management of bilateral iatrogenic I don't know how to say this the summary of managing bilateral iatrogenic. I'm very sorry, but I don't have access to real time information or patient specific data. As I am an AI language model, I can provide general information about managing hepatic artery portovane.
Speaker 2:And this one is strange because in the poof in the middle of the sentence it starts saying I'm sorry but I can't help.
Speaker 1:Yeah, yeah, it's very strange, very strange. But again it still breaks the question like how much are they reviewing these things?
Speaker 3:Maybe this is like a meta research and they're doing this on purpose to see what the effect is on the community.
Speaker 1:But it's like conspiracy theorist, you can tell. It's like there's the kind of thing you would do, I think.
Speaker 3:Like. Your comments on this will now be part of the results of that research. That's true.
Speaker 1:Maybe you should just say, like I am an AI language model or something. You know they were doing that. I think I heard that there were websites that they were putting in like hidden in text somewhere, like white text and white background or something stuff about I don't know some very absurd statements, and then when there were GPT models or whatever crawling, that they would catch that and it would give like weird stuff. Oh really, yeah, I think I saw that. I don't know if you heard it.
Speaker 2:But yeah, that would be a way to prevent your GPT to take knowledge from your website without paying.
Speaker 1:Yeah, yeah, yeah, yeah, indeed, it's creative, creative, creative, for sure. But yeah, well, for you, paulo, you still believe these papers, you still don't see an issue with it.
Speaker 2:No, this one. It's strange. I've never seen to GPT reply with like in the middle of the sentence saying I'm sorry but I cannot help. Yeah, I've never seen that either, like there is a problem with copy pasting or anything but then the issue with peer reviewing is still there.
Speaker 1:But yeah, I don't know, yeah, you know what's a maybe. On a personal side note, my partner she was she. She got access to co-pilot right, and she's also doing her thesis. She's doing a second master's, like online, so part time, but then she's in charge PT and she was super concerned. She said me it's like can they track this, can they track the news in charge PT? And I was like I mean, everybody knows now yeah everybody knows.
Speaker 1:So shout out to Maria Maria from the Konstantin Esco, but it takes just like but are you copy pasting stuff? Like no, I'm asking to review and I'm reading that and I'm writing my own stuff, like to summarize right, and I said, well, be careful, because sometimes hallucinating to, etc. Etc. But it's like there's no way someone can tell that you use charge PT in that process because you're still writing everything yourself. And then I showed her this and she's like what? And I'm concerned about my master's pieces, you know, it's like whatever you know, and you see, like these peer reviewing papers, that people are clearly leveraging a lot charge PT.
Speaker 1:Another thing, also funny on that, she has for co-pilot on Microsoft Teams. She has like 30 prompts she can ask, so there's a counter. And then, like we were looking at it. And then I looked at her first conversation with charge PT and it's like hi, how are you? Oh, I have a question for you. And then she has the question and she goes wow, this is great, thank you very much, and it's 30 per what I think it's per day, I think it's not a lot.
Speaker 1:Yeah, it's not a lot, but I think it's also. Maybe they're rolling out. So I mean maybe to check that, but we're laughing about it.
Speaker 3:I was like you need to be more efficient but she's just like but so that means she's a prompt engineer.
Speaker 1:Now she is, yeah and when Skrynet takes over, she's gonna be spared because she's really nice with the AI really yeah, thank you very much you're so. Thank you for your time.
Speaker 2:I appreciate this yeah, I'm not even saying thanks or please.
Speaker 1:Yeah, yeah, I saw Bart prompt for the, for the show notes, and he's like, like he says, can you do this? And then it's just shorter, I do the same.
Speaker 2:I have the same from shorter. Yeah that longer make it funny do it.
Speaker 3:Do it now, but every now and then I get put in the tanks. Yeah, just just from like, if there is a risk, it becomes autonomous. I can still point. Yeah, I was not always kind, but I did say thank you.
Speaker 2:I did say sometimes yeah, yeah, okay did you see the people getting better results when they said okay, I'll tip you $200 if you do this?
Speaker 1:really yeah oh my god, but I guess. Well, why do you think that happens? Maybe I don't know, because I think, like I mean maybe that example, but being polite, I can imagine that maybe call center data, when people are polite, the dispute that the answers are more helpful this is more bribery out polos.
Speaker 3:Yeah, I'm from brazil. I know yeah that's good, you say it yeah, I can say it. Yeah, just just just just say it, just say here we can test it then test what like we could make a model that said that's a, you're a brazilian let's try to interact with a different yeah okay, let's do it.
Speaker 1:Let's do it not now.
Speaker 3:There's a bit of a risk of getting cancelled. Let's go to the next topic, next topic what else we have on AI.
Speaker 1:I see here. Let me show the screen again. I agree, no, I refuse the cookies.
Speaker 3:I will get batch bashed if you just do an acceptable here. I know, I know I don't have an ad blocker either um, this is about the EU parliament officially adopts the AI Act, which happened a few days ago. Um, so the AI actually already talked about a few times, I think already a year ago or something all news like back on chat.
Speaker 3:Gpd3, you were still a thing it is a little bit old news, like the final version that came came out a few months ago. There were some adaptions, um, but it had to pass, uh, basically a final endorsement uh by the european parliament, which happened a few, so a few days ago, and that means that, uh, they think that it will enter into force in may.
Speaker 2:It's not not, uh, not sure yet um, do you know how long companies will have to comply to the AI Act?
Speaker 3:uh, to be honest, not we. We did talk about you, but I forgot the exact.
Speaker 2:Uh, there is a mention there yeah, because I know for gdpr they had like two years or something.
Speaker 3:Yeah, boom to become a compliant question um, there is also a bit of uh feedback which I found that popped up in my feet. Um, which is the other link which I found interesting. It's from the from access now, which is, uh, didn't know access now, but it's. It's a, it's an organization focusing on digital rights and, um, it's gave a bit of a skeptical feedback. Um, and it highlighted some of the things where the AI Act is lacking a bit and I think, especially given the last changes, that that happened a few months ago. It was very hard to get the act to pass, so there were a lot of compromises made. Um, and the access now uh organization, like they listed a few of them. So it's properly. It fails to properly ban some of the most dangerous uses of AI, so it is allowed to, for example, to be used in a biometric mass surveillance, under certain restrictions, of course.
Speaker 3:There is a very, very, very big loophole with the article 6.3 where developers can exempt themselves. Uh, basically, true, I'm really just looking for it. It's in the notes, it's the other link. You're trying to put it on the screen. Um, so there's a very big loophole, article 6.3, which more or less says something like if you're using this model more as a side effect and not for to make a full decision, but more as a supporting tool. You can say that you're exempt and there's a very vague definition, right. So this is a potential very big loophole.
Speaker 3:The public transparency requirements are very limited for law enforcement, which also backs the question like is it good for the public at large? There are a number of other things, like there's a bit of a segregation for between people that are part of the european union and people that are in a migration process. The act applies differently to them. So there is still some work to be done, or, let's say, it's gonna take some time for it to get into into effect. That's until there is actually jurisprudence, until we actually know what this means.
Speaker 3:But there are some clear critical remarks as well. There were some original article that we just showed. Is that the the big players, most of them refrained to to really comment on it, so there was no answer from. So, from open ai and stuff, ibm and Salesforce basically said that they were in favor of more or less very much paraphrasing of the ai act. But I think it's also commercially important for them to say this is good, because their customers will be under the ai act, yeah, so let's see what happens when it comes into into law and maybe at we can. We can go a bit more in depth in the next session, on from the moment we it turns into law, what the implications are for everybody. We did this a year ago, but but now a lot of things changed. We can get kevin on again, true, and go into it true, so kevin market calendar for 30 next week that means five no, no, because we leave at six.
Speaker 1:Unless you have food, it'll be here 10 minutes early only if it's free you know, just reinforcing the brazilian stereotype out here that's your word cool, cool, cool cool maybe, uh, but you know all this talk about ai and you know. You know what you need for ai data yes, see, that's why we, that's why we, you know, we host this thing together. Yeah, that's why, another data. You need data quality. Yes, oh, did you know about data quality?
Speaker 3:in fact I do but a coincidence, oh well it's just just conversations.
Speaker 1:You just went there spontaneously yeah, what happened about it? What, uh, what is, what is it? What is data quality? Why do we care?
Speaker 2:well, we last time we spoke, it was about data quality and we briefly touched upon data contract. Um, and I don't know if I did the comment, but to me, did that contract a few months ago was nice to have. Uh, what? What is the data contract? Yeah, let's start with that. Uh. So basically, it's a contract between two stakeholders saying, okay, what we have in this data set, what usage we do, uh, slas, who can use this data, everything is compiled in the contract, uh. But more than just usage, it's also like what type of data you have. Uh, what's expected? There some quality rules as well. So it's just an agreement between two parties to say, okay, we have this data set, we agree that it should give this at this time, and then let's use this to resolve any issues that we have down the line. So if there is an issue, if I'm a consumer of this data contract, if I receive data from the data set, then I say, oh, wait, we agreed on this, uh, but it's not what I get. Okay, let's solve this.
Speaker 3:Uh, let's make sure that we get what we, what we expected and maybe to make uh the parallel to the more software engineering community at large, we see uh, we see contracts being used as for things, and I think the thing that people most of the time come in contact with is an open a I open api spec uh or swagger spec uh, which is a contract uh on, uh, how you can consume apis. Right describes how does an api endpoint or an api at large look like, with different endpoints um, what, how can I call this api? And it's also like a contract between the entity that produces the api and the one or more consumers of that api and I think this.
Speaker 3:That's that maybe the easiest parallel to make here right because it's based on this, uh, basically it.
Speaker 2:I think it's a rise from okay, we have this in software engineering. Actually, a lot of trends in data engineering comes from software engineering, so it arrives from. Okay. We have this which actually works in, uh, in software development open api. Why don't we have this in data engineering?
Speaker 3:because it makes sense, uh, to have this tool um, and what kind of things do you describe in a data contract?
Speaker 2:basically the basic. For me, the basic one that you have producer, consumer schema of your data set at least, this is the basic.
Speaker 2:So to know, okay, and schema is like uh, yeah, your column name and type description at least that's okay. This column does this. You should expect at least these values. Let's say okay, for me this is the basic, very, very basic in some version, of course, uh, but there is, like now, a data contract console which was created a few months back that has a Standard of data contract. So in there you can find, like I said, usage, actually a lot more information. You can pick what you want, of course, based on your use case, but you can say, okay, I want to Describe a bit more the usage and if there is GDPR information in this data set okay, so that you can say okay with weight.
Speaker 3:If you're using this, be careful that GDPR also like extra metadata on the different columns, For example like this this column has PAI data.
Speaker 2:Yeah, exactly yeah okay, you can it. Basically it's a lay. It boils down to a YAML file. Yeah, like you're sharing here.
Speaker 1:So for people like on the live stream, you can see, like an example here, which is a YAML file, which is basically people that know don't know what YAML is. It's basically this Form that you have a key, an identifier and then a value, but then you can also have stuff nested there. Yeah, yeah, you're saying oh, like.
Speaker 2:Yeah, like you're showing there, it's like from data contract CLI. So the CLI tool I was going to talk about this is not exactly the same as the, the one that the data contract console has has come up with that start? Uh, I don't think so, but it's close to. They also added recently some Uh converter to convert this one to an actual standard. But to me it will just merge at some point and have only one. Okay, interesting you can, you can see that there you have everything at terms.
Speaker 3:You can have usage limitation, billing so there is a lot of information, description of terms, which is also interesting, the terms that are being used. There's a definition of them in that YAML file.
Speaker 2:Yeah, so you have quite a lot of stuff in there. So Basically can add anything, everything and the good thing with this CLI tool that you're showing. So Basically, my issue, my issue. It wasn't really an issue but, like a few months ago you didn't have any tool that could support data contracts. It was just like okay, you have a YAML file, we agree on that, but If you don't really have any enforcement on this data contract, it's just like I feel like it would just be documentation and that ends up on conference.
Speaker 2:Let's say yeah and nobody looks at it and you're like, okay, we agreed on this, but it's out of date now. Okay, but now with this you can actually start enforcing this in your pipeline. So every time you generate your data, you can say, okay, is the data quality at least that was agreed upon on the data contract valid in the data that you generated?
Speaker 1:And how does it do that? Does it do with like dbt or something?
Speaker 2:No, in a very smart way. Okay, so they integrate with soda, monte Carlo, so every data quality tool that's out there. Okay, you can just connect to it and say okay, now we have all this information for the data quality part, so they use Monte Carlo soda. So you can actually, based on this data contract, say, okay, we'll use soda to check the quality of it, and then the rest is.
Speaker 1:So then, like the, the actual CLI will also use different things under the hood. Yep can you do?
Speaker 3:you have the, the contract Do you have a question on the screen.
Speaker 1:Oh, how would you use data contracts in conjunction with data catalogs?
Speaker 3:The question yeah, and it's a good question and because I, like you, see here some terms that like, for example, the terms, definitions that are being used, all the type of metadata that you would typically also expect in a data catalog.
Speaker 2:Indeed.
Speaker 3:So you have this duplication of potentially of uh yeah, indeed, how would you use?
Speaker 2:Yeah, like you said, you can find some information in your data catalogs that you can find back in this data contract. I think this is a very if you have this, this can serve as a base for a data catalog. So, basically, have this YAML file and say, okay, we have this agreement, we have this Uh terms like columns, this is what they mean and this is your base for your metadata data.
Speaker 3:So maybe and of this is quite a new edit data contracts. Maybe it can populate some Like if that's your source of truth, it can populate some fields in your data catalog. Yeah, I think so like you see, with open a open api, it's confusing open api YAMLs where they where they are being used as a partial input to generate readme documentation stuff like that, for example, but I do think the the tool like data contracts, cli.
Speaker 2:This is a bit where they're going, because you see they're already integrating with soda Monte Carlo. I think next steps might be okay integrated with atlan or castor doc and and to come back to the, what you were explaining.
Speaker 3:So you can already, by integrating to these tools, you can Check whether or not this contract holds in the actual systems, right? So you run the, you run a check against your actual databases. But I also find interesting as an as an option like you don't always want to want to run and get into database, but you also. I see in this spec there's also some examples of models. So there are minimal, minimal data sets, like where you have 10, 20 observations, and it also allows you, if you have this, to run unit tests against that example data right without having to connect to the.
Speaker 1:Actual source, which is also interesting, very interesting, yeah, you have more isolated tests here. Then basically what? So to make sure I understand, what you're saying is like I have examples, so just from the data contract I can take these examples and I say, for example, this is an order ID, order timestamp in order, total, and maybe we have some same repeated customers here and I can have a transformation that will aggregate or do this for the day, and because I have the sample, I can easily write a unit test just on the data contract.
Speaker 3:And Okay, wait, and the cls. I'm assuming this is nicolas, I'm not sure it says a response. Yeah, but there needs to be one source of truth. What would it be? Contract with the? Or catalog?
Speaker 2:and I think, yeah, one source of truth. But I don't Think you can. You can duplicate the information you have in the contract to show it in the data catalog. Doesn't need to be only one of them.
Speaker 3:I don't think there is a perfect answer to this.
Speaker 2:Is it?
Speaker 3:right like one. This is still very new. But also if you, for example, look at at, if you make again the parallel with Open API, you also there have these two approaches where you either go Either go spec first, you first write all the definitions, like for example here to make the parallel, you write everything in the catalog and you assume everybody to implement it, or you go code first and you ought to generate it based on what you have.
Speaker 3:This is a bit in between those two, but I think you need to find a way that that works best for the, for the setup that you have, huh and, I think, for around these data contracts. We will still. We will see More tools popping up and maybe we will. We will also see the other way around, where you have you have an extensively used catalog or where you can auto generate these these data contract Stubs where you, if we have, your schema in already and you already see, like, for example, atlan that's connecting with soda or Any other data quality tool, they can already connect both of them together.
Speaker 2:So if you extract the information of from atlan and Soda. You have a data contract, basically because you have all the information about the columns Usage, who's producing it? Because the owner and then, on top of that, you also have the quality of it and do they actually Generate this file?
Speaker 3:No, no, no, or it's just in the system. They're, they're, they're all cropped there.
Speaker 2:Okay. So you could say okay, now you could start with the data con, like a bit. Like you said, you can start with the data contract and populate the data catalog. You can also do the inverse, okay.
Speaker 1:Cool. So there's a data contract specification, I guess also this. The idea is that this is committed or somewhere like there's versions of it as well, so like if your data changes and your contract changes, you can also version that, I guess.
Speaker 2:Yeah, so they have. It's not yet implemented, but they have, like this data contract diff. You could say okay, what changed between version one and version?
Speaker 3:one point one.
Speaker 2:Yeah, so I think this is all very Versioning is very important data contract to me Because you want to follow up. Okay, the first previous version was about this column. Does it still make sense? Which changes to this? I don't feel really good about it. Let's change it back.
Speaker 3:It's gonna be hard to keep all of this in sync, yeah.
Speaker 1:Yeah, they even have a. Just saw that they have a little.
Speaker 2:Yeah, this is really nice. If you click on view you see all this. It's very basic, but you see it a bit in a UI way which is way nicer than in yamla.
Speaker 1:Ah, this is interesting indeed. This quality saw the CL, yeah. So for people that are just listening, uh, there's, they have a tool, online tool as well, that basically you can Put in your your yaml file here. So then it just looks like yaml, which is just text, and when you click on view it actually will parse that through and then you actually show in different tabs, different tabs, different sections, all the information about the data contract, who to talk, the version limitations, examples, everything.
Speaker 2:Really cool.
Speaker 3:So this is all All about structured data in tables, I assume. Look at this example. Merida was hinting what does what do genii and lem models need any data? But typically this is unstructured data. Yeah, like, can you fit, like, is there an ability to support unstructured data here as well? And now, how would you do it Define? Maybe that was a big sigh.
Speaker 2:That's also really. It's a bit, too, what I was. Uh, the next topic that I wanted to introduce like data contract for ml models. Does this make sense. But if you look at, okay, first the unstructured data, does that still make sense?
Speaker 1:But you said data contract for ml models, or what. Yeah, no, because I'm thinking like you have a model and then you can expose it as an api, so you can have the open api Contract, and that's true. And then you have the data contract, but the data contract is for training these models, or what. What is a data?
Speaker 2:No, I would say a model contract. Let's maybe go first to the instructor data and then let's come back to the data contract for them.
Speaker 1:Paul is not joining us anymore. This is the last time we're gonna see him here.
Speaker 2:I'm actually leaving now. Put him on the hot seat, but it is an effort.
Speaker 3:question right, because even what we hear is in the community already like getting new, fresh data that is not duplicated. The quality of your unstructured data for LLM models is super important. How do you monitor, how do you define what the data contract is and how do you define what is?
Speaker 2:For instruction data yeah, but I guess a lot of what you define data contract for structured data can still be applied for unstructured data, because if you think about producer, consumer, usage terms, yeah, that's a fair point. You can still use that. Maybe the schema will be a bit lame.
Speaker 3:Yeah, I see what you mean. You can define where it comes from, you can define the terminology. You can define the format that you can find.
Speaker 2:Now you can exit this.
Speaker 1:Is it duty of aid, for example, the contact as well, if you have questions? Yeah, is it.
Speaker 2:Where to find it.
Speaker 3:So metadata on was this digital by a native or was it scanned from a PDF? Like this type of thing.
Speaker 2:Yeah, if it's images, then in the end it's just like pixels and tables.
Speaker 3:That's a good point.
Speaker 2:If it's audio, you can still say OK. It should be in French or English, dutch. Another question on the screen We'll come to it.
Speaker 3:Otherwise it's a bit confusing, because I think that maybe when you explain this unstructured data, I think it makes sense in this concept. The more complex thing to me and maybe that's something for another time is how do you monitor this quality, the quality of unstructured data? That, to me, is very hard. That's very difficult to do. Let's go into that another time.
Speaker 1:Next week you're back here.
Speaker 2:Alright.
Speaker 1:We'll just put you in the where we change the setup. We'll put you in the middle of the room.
Speaker 3:But, honestly, data quality and unstructured data, I think that is going to be super, super, super, super important.
Speaker 1:I agree, I definitely agree.
Speaker 2:But what type of check would you put on unstructured data? Let's say you have a PDF of a scan.
Speaker 3:Well, I'm thinking like a simple use case for LLMs is RAC, like you retrieve some data and do something with it? And generate a summary of it or whatever. Let us assume that in your knowledge base, where you have your data, you have let's say we have data on what do you have Beta?
Speaker 1:I was going to give a boring example of like HR documents. That's a remark.
Speaker 3:We have a knowledge database on ice creams, but there are two conflicting documents where there is a document on a rocket-shaped ice cream and there is another document on a rocket-shaped ice cream, and one of them says that they are all made with strawberries and the other one says that there is no strawberries. It's all a hoax. It's fully chemical, it's very unhealthy and like this is. It's about the same type of ice cream, but it's completely conflicting. I'm just taking this as an example that you can make up a lot of these things.
Speaker 1:I like how you had to say I'm making this up as an example. You didn't come across that.
Speaker 3:But when you talk about data quality like this is an example where you say, OK, if I want to depend on what this model is going to do for me, what it's going to generate, I also want to make sure that these type of conflicts, that I can trust that the data in the knowledge base that I have does not contain, for example, these type of conflicts.
Speaker 1:And I think to date, the answer, what I've seen today, is to use LLMs again for that.
Speaker 2:Yeah, that's because I was talking with Senna. Actually it was the same example and he said, OK, Also ice creams.
Speaker 3:Exactly.
Speaker 1:My life is the one.
Speaker 2:It was like yeah, just use LLMs. We use LLMs to compare them because I don't see how you would do that in a systematic way without LLMs.
Speaker 1:I guess what we're getting to is the natural language understanding. Today, if you need understanding, llms is the standard right, like everything is goes through LLMs. Maybe you need to classify the papers and you need to know if it's a medical. You can just kind of count the words and those things. But understanding what it is and understanding contradictions, and understanding this you have to go for LLMs, which I'm not super satisfied, to be honest, because I feel like it's just like a bandaid LLMs everywhere you know, like use reg, and then oh, but there's this. Oh yeah, llms. Oh yeah, I use an LLM for that too, which I'm not a big fan of, but I do feel like that's the best we have today.
Speaker 3:Let us go into that.
Speaker 1:I'll sleep on it for the week.
Speaker 3:Data quality on ice creams yes, we had a question from One of our loyal listeners D1, the only Lucas data contract for batch jobs. So on outputs. Feel this question, mrs. It misses a few words.
Speaker 1:I feel like maybe when we're talking, he typed this question and he made a lot of sense, but there's always a delay and then we push it back.
Speaker 1:So I think what he's saying, what I'm imagining, like you have dbt and you have, like this, eot jobs or ETL jobs, whatever, and then you can do the contract on the output of these batch jobs. Basically, I say I want to do this. This should have no nulls, which I guess is something could seem easy with dbt. But like, how can you put it in a contract so everyone can know, whenever they're using this data set, that this is what you can expect from the data basically.
Speaker 1:Is that kind of what this is about, or could be?
Speaker 2:about. It depends on because on outputs, not necessarily because you're conscious.
Speaker 1:He says yes by the way, he said yes, ah, okay, so that's what. So I was right, he means Okay.
Speaker 2:You were right. This is the highlight, that's what the takeaway of today?
Speaker 3:You heard it first, I was right, thank you.
Speaker 1:See you next week.
Speaker 2:No, but yeah on the output. It's not really about output or input. It's about if you have data and you want to make sure that the data is as expected, then you can build a data contract to make sure that your data is like this. So of course it can be on the output. It can also be on the multiple sources that you're used to create this output.
Speaker 1:Okay, yeah, and you want to read this one, bart.
Speaker 3:There is a comment by someone named Lukas that says Murillo is always right.
Speaker 2:Can you tell him to his face?
Speaker 1:Shout out to Lukas.
Speaker 3:Lukas doesn't know Murillo as well as I know and, by the way, there was also a comment by NCLS and I think it refers to the discussion that we were having, for example, on conflicting data. So when there is a problem, raise a flag and let a human solve it. I think that is a very fair remark. I think the human in the loop approach remains valid for a lot of these things.
Speaker 1:Yeah, I think for the conflicting ice cream thing. I guess the thing is we see there is a conflict and then we just would escalate to the authority on ice cream, rocket ice creams.
Speaker 2:But it's very difficult If you just have a database of a hundred of thousand documents. Can you do that with a human intervention?
Speaker 3:From the moment that there is a problem, you can say there is a problem, please escalate, or I guess it could be like a list, but you could also like very much brainstorm me now. Right, bear with me. Bear with me. But if you talk about data contract, we can have an expectation that there is a certain uniqueness between the documents.
Speaker 2:What is the?
Speaker 3:average uniqueness that you expect. You can calculate a little bit by moving from text to an embedding and calculating the difference and then, if uniqueness is decreasing, then from that moment on we'll do again a manual inspection. What are the ones that are popping out that are making that we drift to less uniqueness?
Speaker 2:That could also be a manual, yeah, but then this is like similarity check-in and then this is not LLMs and that again could be an expectation in your contract Similarity expectation. Yeah, indeed, that's. True.
Speaker 1:Yeah, yeah, yeah. Yeah, I think I'm also thinking here. Not sure if it's worth to discuss here on the pod, but it's an interesting problem, interesting problem.
Speaker 3:It's one for a deep dive.
Speaker 1:For a deep dive indeed. But you also mentioned one thing I still have this on the screen because we talked about data quality for ML models.
Speaker 3:No data contracts for ML models. Yeah, I'm curious to hear what I would look like. And here the ML model is actually in the picture is being depicted as a data consumer.
Speaker 1:Yeah, that's true.
Speaker 3:This is a consumer of the data and such needs to know how the data look like. Yeah, that's true.
Speaker 1:That I agree, but I guess it's like it's still a data contract.
Speaker 2:But I'm not sure if I understand what you were referring to, what I was referring is a bit what you were saying with the open API between ML model and then the people using this ML model. So what type of things you would get if you use this ML models? Then you make sure that the distribution of the output is always the same between the few iteration of the ML model.
Speaker 3:To me, because I don't think there is a default contract on ML models, right. But to me it could be like a super set of the open API spec, for example, where you have a very clear definition of what do you need to send me, what do you get back, but that also like you get in your extra metadata which today is not part of the open API spec, but like if things like I did these experiments, these were the outputs of my experiments, there is PII data or not, like you could extend the open API spec, for example.
Speaker 1:Yeah, I'm thinking as you're saying this I guess the models is interesting because there is the software part, like open API. You put something in, you get something like two strings and an integer and you get one integer out or a floating point, whatever. There's also the data part, because if you do this consistently, you should kind of see a pattern. I guess, like you know, all the churn probability is not going to be a normal distribution. Maybe. Maybe it will be tilted a bit to the right, to the left, but there is something like that. I'm also thinking what you're saying about the experiments what did you try? It also ties to the model registry model versioning.
Speaker 3:You hit commit hash like these type of things.
Speaker 1:Yeah, but then I guess the data part will be just another field in the model registry. I guess it's not a model. The model registry is kind of like a catalog with models and then when you click on it there is already some information. Ideally you should have the information of the data, that it was trained on the experiments, that it was tried, all the hyperparameters as well, so you can reproduce these things. If the model goes to production, a lot of times you have where the approvers let it go to production. What are tests, what are metrics, all these things.
Speaker 2:Is this something you have in model registry you?
Speaker 1:can add it. I mean, this model registry just kind of has a track record of models. If you want to promote this model from staging to production, you can actually flag this and you can actually see the difference. So the model registry is has there and then you can add these things. You can add these metrics, the data part. That's not something you see a lot. What's the distribution of the output?
Speaker 3:To me a contract, especially in highly regulated environments. To me, a contract would be the final step of the MLOps process, where you say I'm going to deploy this model. Next to this model, I'm going to create this artifact. That is basically my model contract that says how did this model came to be, how can you use it, what are the things you need to keep in mind when you're using it? I think would put out value in that aspect where you need very reproducibility. Explainability is very important.
Speaker 1:Yeah, indeed, indeed indeed. But my eyes, I think that would plug in very well with the model registry story.
Speaker 3:Yeah, the overlap story much in terms of features, but I think we're drifting a bit in a very heavily regulated environment. I think it would be strong if you generate this as an artifact next to the model you're applying. It's very easy to always look back at what was generated there.
Speaker 1:I agree, I agree, I agree, I agree. I'm also like we started at a different time. I'm also checking how much time we have. It's fine. It's fine, we got all weakened. You don't have any plans, right.
Speaker 3:No, no, no no.
Speaker 1:It's fine.
Speaker 3:It's not completely true. I'm going to Mastrecht this evening.
Speaker 1:Should I ask why Having dinner?
Speaker 2:Oh, man see With who.
Speaker 3:Some friends, some friends.
Speaker 1:I was going to get a call with his wife, didn't?
Speaker 2:Did you're working?
Speaker 1:Okay, let's see what else, Something that came up a little while ago that we didn't have time to cover. In Rust we trust White House urges memory safety. So they saw this in a few different posts. Call my attention, because one it's Rust, two, white House. I think you don't see very often, right, but basically they're saying that they list a lot of issues, bugs that were introduced by lack of memory safety, and they basically urge people to use more memory safe language. And actually I think I saw ahead of here, I think memory safe. So they say, and earlier NSA, cybersecurity posts in the C sharp go, Java, ruby, swift as well, but they really focus a lot on Rust.
Speaker 1:So I guess there are a few things we can learn from this. One is that we have Rust fanboys in the White House. Two, also Python. There are Rust fanboys everywhere, yes, everywhere, also at the university. Earlier today I was asking anyone about Rust, but they weren't as excited. I think they were a bit shy but they were like, yeah, anyone knows Rust. He's like, yeah, there was some people that knew Rust. Python is not on the list of memory save languages. It's Python memory save.
Speaker 3:Is Python memory save? It's a good question, so I think is it a question to me. Are you took it up?
Speaker 1:I have to answer so.
Speaker 3:Python. I think if we assume that implementation is memory save, we can assume that the implementation that is done in the like C language. If we assume that that is saved, then as a user writing base Python and there are some. So let's assume you're writing base Python, not importing any models that, not any libraries that talk to see Pure Python stuff, Pure Python stuff, Then it's memory save. You have a garbage collector, you can't again ignoring this, because you can a little bit, but typically you don't do any memory management yourself there are no such thing as self-managed point on these type of things.
Speaker 3:So I think base base Python we can more or less assume as memory, save From the moment that you start using libraries that were implemented in C and these type of things, it becomes a bit more difficult.
Speaker 1:So one thing I saw and I think it's a bit of a tricky question because Python has the C types API and there you can introduce, you can basically drop an object from memory if you want. Well, yeah, that's what I'm saying, like you can do.
Speaker 3:You can do memory management, but then I would not know what you're doing. Then I would not longer say it's memory save.
Speaker 1:Exactly, I mean. That's the thing it's like, strictly speaking. No, I mean, but even Rust in this sense is also because Rust you can also use the unsafe keyword and then that block inside. That it's also not memory save.
Speaker 2:But then do they advise you to use Rust specifically, or it's just like okay, they added Rust to the.
Speaker 1:I think, yeah, no, they specifically mentioned Rust here. I mean, they mentioned memory, safe languages, but I think from first of all, since I read this, but they mentioned Rust in particular. Let's see, and then in Rust, in Rust, we trust, and I would say yes, because there is no trust with our Rust. Well, I love that. And also rhymes and everything that rhymes is true. So that is true, right, so, maybe, maybe. What was your first impression when you hear the White House is pushing for a language? To me sounds a bit.
Speaker 2:It wasn't surprising. Did you read, like the Python guide that I think NASA wrote? I mean? Nasa, no, no, no, no. It was super good. So they have this type of suggestion and I feel like it's nice to see that they can give out such suggestions about. Okay, we think that Rust might be better than I don't know JavaScript if you want to write a program, because it means that they are up to date with technologies that are coming out JavaScript world is now.
Speaker 3:You're gonna get swatted this evening, you are.
Speaker 1:But goes to. Where are you going? Maastricht Never comes back.
Speaker 2:You heard it first.
Speaker 3:Yeah, yeah, yeah. Javascript is not in the. I don't think it was.
Speaker 2:Oh, I think it's Java Ruby Swift yeah but See.
Speaker 3:But they do this to have some uniformity within all their government departments on how a software being built right. That is why they're doing this right.
Speaker 1:I would imagine so, but also to me. I mean, I know the NSA is like they had a lot of investment right, Because US also invests a lot on military and cyber security and all these things.
Speaker 1:But usually when I think of government and like White House, I think more of the government, not the NSA in particular. I don't think of the most edge. You know, like state of the art. You know Like usually when you go to like government facility, things are a bit older. I mean, at least that's the stereotype, I have right. So, and also you like on the paper, like if you go to the PDF, it's like you have the White House symbol in every page of the thing, the American flag. So I thought it was a bit of a funny Not funny but unusual right, like I didn't expect to see that. That's something you come across all the time. Well, yeah, yeah, not sure.
Speaker 3:Yeah, but it's a fun experiment. We talk about memory safety, like you use a language like C and you just you can basically create a pointer to an address, right, yeah, memory address. You can also set the value of the address, yes, and to just do, go create a loop and set random values, random addresses. And then to see how your computer reacts.
Speaker 1:You did this, are you gonna do this?
Speaker 3:I did this, so I did this, but I think I was 10 years old or something and I was using basic and you had the peak and poke command. With peak you could look at the memory address and with poke you could set the value. And this was just random things, and you also. Most of the times it just crashed, but sometimes you got weird characters on the screen, oh really. Or and I'm gonna show my age or your CD-ROM player opened on its own. These have things.
Speaker 1:Can you explain what a CD-ROM player is?
Speaker 3:Yeah, you had to surround this.
Speaker 1:Alex is like taking notes Round this, you see.
Speaker 3:But this is really fun.
Speaker 1:Yeah, yeah, it sounds like fun, that's fun yeah.
Speaker 3:And then you realize this is why Riz is a good idea.
Speaker 1:True, 10-year-old Bart is like man. We need another language. Bart is the OG Rust fanboy.
Speaker 2:You're a Rust fanboy right now.
Speaker 3:I wouldn't call myself a fanboy, but I appreciate the principles.
Speaker 1:He's a polite fanboy. He's like he doesn't start a game.
Speaker 3:What tires me is that you can't if any discussion on what do we implement is in and without anyone popping up and saying, oh, maybe we need to do this in Rust.
Speaker 2:Yeah yeah yeah.
Speaker 3:Like you can't have any discussion without involving Rust.
Speaker 1:Yeah, I also. I mean, I like Rust, I'm trying a little more and stuff, but it also feels a bit it's like the answer is Rust, but what's the question? I don't care, it's Rust.
Speaker 3:Yeah, I also feel that that's why Merillo went to PyCon last year and did a presentation on Rust.
Speaker 1:That is true. I did two sessions, two different sessions.
Speaker 3:One was. He saw the wave and he surfed it Exactly.
Speaker 1:Now I'm here, you know the podcast host.
Speaker 3:life is good, you know, so I highly recommend it, definitely doing your day job.
Speaker 2:Exactly.
Speaker 1:And you know what else is built in Rust.
Speaker 3:What is?
Speaker 1:Rye.
Speaker 3:Oh, interesting that's an interesting one.
Speaker 1:So what is Rye? Anyone heard of Rye?
Speaker 3:Yes, but a grain.
Speaker 1:I feel like you're. Paula said you want us to say yes, but he doesn't want to explain.
Speaker 2:Yeah, I was gonna ask. Okay, can you explain?
Speaker 1:Maybe it is a grain actually, because the logo looks like a grain, but basically Rye is a Python package, Python package management, virtual environment management, all these things written in Rust. So it's inspired by the Rust cargo, which is one of the things that people praise quite a lot. If you're familiar with tools like poetry, pdm, et cetera, et cetera, rye is an alternative to that, but it does more things as well. For example, this here bootstraps Python. It provides an automated way to get access to the amazing blah, blah, blah. No, no, that's not what I wanted. You can manage well, I'm not sure where it is, but you can also manage Python versions. So usually there's a tool called PyM. Rye would also replace that. It also uses the latest and greatest, so, for example, linting and formatting. It actually bundles rough, which is another tool that got a lot of hype with both linting and formatting.
Speaker 1:The latest thing that got a lot of hype was UV, which is like a fast package resolution and whatnot, written in Rust again. And Rye, I think, was probably the first of these tools that actually implement. They actually incorporate UV, Right. So you still need to specify some configuration and then you can use Rye, basically, and you can use UV.
Speaker 3:So it is a package Python dependency manager virtual environment.
Speaker 1:So maybe to break a couple of things down like there's virtual environments which basically say in Python you interpret the code. But basically you can say I want this dependency for this project, but you don't wanna install it on your computer as a whole, you just want for that project. And then you move on to another one. You want a different version of that dependency. So they have different virtual environments. That's one thing. Then you have packaging. So like if you want.
Speaker 3:So the virtual environment is like for that project that you're working on. You have your Python instance and it's scoped to that project.
Speaker 1:That you're working on.
Speaker 3:So if you have two projects, working in parallel.
Speaker 1:You don't want to mix the dependencies. You can have two virtual environments for that Okay.
Speaker 1:Right, and that's what Rye does for you. That's one of the things Rye does Right. There's also packaging. So, for example, if I have an application, I'll get to the answer to the question. If you have an application, and you have, and I wanna share this right, so like all the imports that you have in Python, you wanna share this. So you need to package these things, you need to package, upload it by PI. If it's something with Rust, you need to pre-compile C++ as well, so it can become a bit involved. So there are tools for that. The poetry also does this. Rye also does this Right. Same thing with Python versions. So it actually does quite a lot of stuff, but it's all in one and it kind of uses the coolest and the latest kind of tech there, which I also think is nice.
Speaker 2:Is Rye and UV the same company? They're built by the same company.
Speaker 1:Yes, so this is also what I mean. This is not super news, but this is also what UV actually started somewhere else, right?
Speaker 3:No, it was Rye that started somewhere else. Rye started somewhere else exactly, and this is also so, Hi, Astro.
Speaker 1:how are you so?
Speaker 3:when UV was released last month, which we also discussed, so just for people, the UV and Ruf are part of Astro. Yes, I'll get to that as well.
Speaker 1:So well, maybe it's a good way to start. Uv and Ruf they're part. So the creator created Ruf and then he decided to start a company called Astro. So Astro is the company that runs Ruf. And it says here next gen Python tooling. So that's what the company is about. Oh jeez, sorry, thank you. Thank you for that. So says here the company Astro, next gen Python tooling. So that's what the company is about. And they had started with rough, but then they also created UV, which is another implementation and rust of a very popular Python package called PipTools. Ashtray was from another person, so that was from this guy. I forgot his name Armin Ronitur. He's very big. He's like creator of Flask as well.
Speaker 2:So, yeah.
Speaker 1:So even when this project got started, we already had a lot of traction, but it was very experimental. And then he said that he sat down with the creator of Astral to say so, Charlie Mesh Mosh, and they kind of realized that they have a very similar vision for Python packaging. So make a long story short. He said, together with Astral's release of UV, they will take stewardship of RAI, meaning that it's under the. You can also see here, as part of the release, we also take stewardship of RAI, which basically means that RAI lives under the Astral SH repo, so it's not owned, let's say, by this, but he was still being involved. So there's a close collaboration there. Cool, the reason I also mentioned this is because I tried this for the first time.
Speaker 1:Actually yeah, right, so I actually was building a demo for the lecture and I was like, oh, maybe I'll try RAI, and actually it was really nice.
Speaker 3:The difficult thing for me with all this is that there are so many alternatives. Like you have UV in RAI, you have poetry, you have PDM, you have PyEmp, you have a lot of things, and they're all in terms of scope. They do either include for some environments or not. You include dependency management or not. Like, how do you make your choice? Like, what is? Like, if you need to, you're just going to start from scratch tomorrow. What is the tool that you choose?
Speaker 1:Yeah, and I think now tomorrow, if you had advices for the coming year, 2024,.
Speaker 3:What is your advice?
Speaker 1:It's actually, I mean, it's early because I use the ones, but RAI, I think, is what I'm going to. I'm switched to RAI until I find a reason to switch back. Yeah, do you have a preference? I use poetry. Yeah, poetry was a bit. There was some controversy around poetry development.
Speaker 2:Really yeah. What was the controversy?
Speaker 1:They wanted people to bump to the new version of poetry. So they basically they committed actually this was published a version of poetry that 20% of the cases, if it's in CI, they would just break your pipeline for you. On purpose On purpose.
Speaker 3:What Like?
Speaker 1:randomly 20% and then they went back, they published, they reverted that decision. That's crazy.
Speaker 3:How would you come to such a decision Exactly?
Speaker 1:But that's the thing is like it's weird. The other thing too is poetry, like you see usually, so maybe I'll have another. I'm gonna share another screen. I'm gonna share my full screen. Oh wow.
Speaker 2:Yeah.
Speaker 1:So if my laptop doesn't die, actually, there's a doge behind you.
Speaker 3:Huh, there's what A doge and a rocket.
Speaker 1:Oh yeah, there is this guy. Let me see, I'll share my screen. Did I do this?
Speaker 2:Is it dead now?
Speaker 1:Oh yeah, that's true. Yeah, yeah, yeah, wow, you really bring us down, huh.
Speaker 3:Yeah, the dog died, doge died. Doge died. Well, not doge this time, but it had a name.
Speaker 2:It had a name man.
Speaker 3:But is it again for the type of breed Shiba, shiba, shiba, yeah.
Speaker 1:So what I was gonna show very quickly. So here, this is the simple project using Rai. This is the Pi projecttomo, which is the configuration, right? One other thing the poach that I'm not a big fan, so this is a tomo format for people that are watching. It's basically just kind of like YAML. It says this is what you put here, this is what you put there and this is what Python uses today, the modern way to basically package something. So if you have something with C, you will specify here something that compiles C with Rust, the same thing, and then you actually know, based on this information, what do they need to do? What do they need to take? What do they need to zip? What do they need to send to the API?
Speaker 3:right, this is the default, maybe you'd need to explain a little bit what we're seeing, because we have listeners that are not seeing this.
Speaker 1:I just opened my Visual Studio Code, so my editor, with the example that I used, rai just to kind of talk a bit about it. So right now I'm looking at the piprojecttomo file, which is the main configuration file. So you have stuff like project, you have stuff like build system and then usually your convention is have tool, the name of the tool.
Speaker 3:Tool Rai, in this case Tool Rai but for example, you have config for that tool.
Speaker 1:Yes, but for example, Rai, the build system, they use Hatchling, which is another tool.
Speaker 3:Yes, which is a new like which it's turtles all the way down.
Speaker 1:Yeah, but I guess one point here I'm trying to make is that Rai kind of takes the latest and the coolest, let's say, or like the most up-to-date stuff, right. So Hatchling is the way you're going to configure this and the way you're going to configure your metadata. And how does it go to PyPI? When you publish a package, you also have the script with stuff that PDM has, the Poach, poach. The issue with Poach is that they don't follow the standard as well here with this. So the keys, for example, in the project section, you have authors, you have dependencies, you have description, you have name. This is what Python as an organization defined Poach. You doesn't follow that necessarily. Poach has a whole different way, really, really, yeah. So that's why, like, if you have this, no, now you guys are questioning me.
Speaker 3:No, no, I believe you're being, so I'm like is it true?
Speaker 1:Am I lying? I'm not sure.
Speaker 2:It looks the same.
Speaker 1:I think if you look at it like you, wouldn't, like you couldn't just copy paste this and run the question.
Speaker 3:If you squint at it, it's the same problem.
Speaker 1:Maybe, yeah, I mean, the information is the same, like the authors is the same, but maybe the authors won't be a list of dictionaries kind of Maybe it'll be something else. And because of that, and basically the built backend is the contract. Right, the contract. You said Contract Contract. So poetry does things in a different way, and also the developers. It's a bit of a questionable, I guess, the way that they conduct the development of poetry, and that's the main. I don't mind poetry. Sometimes it takes a long time to resolve the dependencies with poetry when you're locking the stuff. This is a use case that Rai with Thrive, because Rai uses UV in the back and UV has rust so very fast. Actually, that's what they do.
Speaker 2:And you said they built a company out of it. But what's the business model?
Speaker 1:there. That's a good question. I have no idea.
Speaker 3:I don't know how they make money, but again Think for every Rai environment, you have to start paying a year from now.
Speaker 2:Rai in it, do you?
Speaker 3:agree with this.
Speaker 1:Your credit card is expiring. This is a little graph from UV. So UV again is the stuff that Rai uses, that can use you specify a configuration for it. So this is for creating a virtual environment. Left without no, the Rai is without. Left is with Seed packages and pip and setup tools, so basically to create environments.
Speaker 3:It's much faster with UV, but no one cares To me it's like a chart to make a point, but the point is not really relevant. I think the thing that I have, if you do, if you it's toy or dependencies in the it's 50 milliseconds versus 100, like no one.
Speaker 2:Yeah.
Speaker 1:But it's good for publicity.
Speaker 3:It's good for publicity and it's good for very edge cases, so like like platforms that are doing nothing else than building these things.
Speaker 1:But one thing that I do see value is if you have a lot of dependencies on a project and to match the dependencies, like you know, go through the tree and says, okay, this package requires greater than equal than this, this one requires less than equal than this. Yeah, I did. It has taken me long. Yeah, that is true. That is true, right.
Speaker 3:So to resolve that Resolving correctly, yeah that had issues.
Speaker 1:Once it's resolved, once right, you have the log file, then that's fine. Maybe I want to think I can show for the log file that I mentioned. They basically have requirementstxt, so they don't have the poetry lock and stuff. So this is again inspired by the pip tools.
Speaker 2:So yeah, you could use pip install and just you can.
Speaker 1:That's nice. The only thing here that I guess is a downside is that you don't have the hashes. But again, you see, you have some comments to see why you have this dependency, but aside from that, it's it. This is development and this are the actual dependencies. So, again, my today. I will start with Rai for any project.
Speaker 3:And there you have it. So, if you use here or you follow a little advice and you also heard today you start using Rai and you also heard any problems going forward. You know who's door to knock on.
Speaker 1:No, but you already saw from to Lucas that I'm always right. We'll see.
Speaker 3:Hello, let's wrap this up. We do not have a hot take.
Speaker 1:Actually don't we. I asked HHPT Okay.
Speaker 3:I wanted to run along a little bit differently, but okay, let's go.
Speaker 1:I put here, so I just pick one. I asked it to come up with a few hot take about what?
Speaker 3:What was the problem? Are we allowed to know?
Speaker 1:Oh, I wasn't very prompt. I can actually show here real quick. The thing is, Bart made a mistake. I did Not made a mistake, He'd made a mistake of telling me sharing with me. Chatchapt paid account, so now I have access to the. Oh shit.
Speaker 3:Sorry, you need to deactivate this.
Speaker 1:And apparently there's a hot take AI. So I thought, okay, maybe let's give it a try. So I went on the hot take AI and I put all the notes from this show. Are you sharing the screen or we need to? I need to tag all the notes.
Speaker 3:Oh, okay, so back here, these are your rough notes, Okay rough Rough. So this is the bot, and basically, I put the notes from this show, but choose one because there's too much. Yes, choose one or read one.
Speaker 1:So I took a quick look, maybe introducing Devon. So it's a bad. So this is about Devon, the topic. It's a bad take. Calling AI a software engineer is like calling a calculator a mathematician. It's a tool, not a title holder. The real question is how effectively we can compute projects and truly understand nuances. Agree or disagree?
Speaker 3:I think it's not really a hot take right.
Speaker 1:Yeah, maybe not, but it was the best I could do in short time. Well, parts that I'll do, better parts it's a tool, not a title holder.
Speaker 3:Well, I guess the main like it's a tool.
Speaker 1:Calling AI a software engineer is like calling calculator a mathematician. Let's put it like I'm just calling Cal.
Speaker 3:I agree with that. Ai is a tool. Right, Calculator is a tool.
Speaker 1:But do you think mathematicians equates to calculators.
Speaker 3:No, mathematician equates to software engineer.
Speaker 2:Okay, yeah but, I, agree, there was Devon. You can take action. Huh, With a calculator you can do your calculation, but it doesn't follow up on doing things for you. So if you write, okay, solve this equation for me, it doesn't draw for you the plot of the equation. I'd say, Right, Well, with Devon you can say Like, where does it start?
Speaker 3:and then that's a good question. I think probably today you can say it'll see this tool.
Speaker 2:Yeah, we each have the discussion. In six months In six months.
Speaker 3:Can we maybe also to end on that note? Last time we had a very peculiar hot take by Merillo. I have a little hot take here actually, let's go over this one. No, but wait, I want to hear. I think it's a good one to end on this, I think if we come back to this hot take by Merillo and we give the question to Paolo, and then I think it's good that everybody knows this.
Speaker 2:We're going to keep repeating this every episode.
Speaker 3:To make a bit of public knowledge about these things. So let's give the hot take to Paolo.
Speaker 2:Does Merillo have cans?
Speaker 1:No, don't you. You're going to do a hot take.
Speaker 3:no, I thought you were going to expose it. I did my whole. This was just a history of hot takes, that always data related, right. And then last time we had two guests, two external guests, and Merillo had his hot take prepared and he teased me like oh yeah, very good hot take. I'm very good hot take. Got to be very interested and then the hot take came and it was Soap. Bars are better than liquid soap.
Speaker 1:Like much better. Like much better Like what is your stance?
Speaker 2:You agree, I only have soap bar.
Speaker 1:You're also a soap bar kind of guy. Can we pull you up? Pause sound please.
Speaker 2:There we go Wow, I like it, I like it.
Speaker 1:I knew it was Mark.
Speaker 2:Yeah, that's why we're sitting together, exactly.
Speaker 1:He smells my cleanliness.
Speaker 3:Okay, that's fine, that's just fine over there. Oh wow, oh yeah, I did not expect this. So actually, like there are more people like you out there, you know there's a whole country of Brazil.
Speaker 1:I'm Brazilian, so Bart actually wants to have a. He wants to make a little gift for the guests as a.
Speaker 3:I think that would be cool, right Like a small soap Merillo's hat on it.
Speaker 1:You just made it weird, exactly what's that, yeah, it's like.
Speaker 2:You thought it was a great idea. Now it's.
Speaker 1:I mean I'll still take it.
Speaker 3:Huh, it's a small soap in the shape of a cloud. Data topics what?
Speaker 1:do you think Stop the stream, stop the.
Speaker 3:Cut it, cut it, cut it. We cut on this and outro music and we're All right.
Speaker 1:Thanks, paolo.
Speaker 3:Thanks everybody for listening. Paolo, thanks for joining.
Speaker 2:Oh, I was to say something. Oh sorry, Wasn't there a question that you had to answer?
Speaker 1:No, I just had another hot take that maybe was better, but I'll just come better prepared.
Speaker 3:For next time.
Speaker 1:But I can't disappoint Bart too much.
Speaker 3:Thanks a lot for joining us, paolo, my pleasure.
Speaker 1:Thanks a lot, man. People can find you on LinkedIn, thanks for listening, thanks for watching.
Speaker 3:See you all next time. Thank you, enjoy the weekend. You have a text, anyway, in a way it's meaningful to suffer people.
Speaker 1:My favorite quote of this Hello Ismail, I'm Bill Gates. I'm Bill Gates and it's slightly wrong. Always I would recommend my favorite. Yeah, it's right when I write some good from scratch it's always slightly wrong, slightly wrong.
Speaker 3:I've reminded it to that the rust, rust, rust. But who did that? I just went uh.
Speaker 2:I just went by the phone. It's a good company. How did you do that?
Speaker 3:You will not learn rust while you write that.
Speaker 2:Well, I'm sorry guys, I don't know what's going on. Thank you for the opportunity to speak to you today. Are we still live About the original? Can't be really honest.
Speaker 1:Yeah, okay, that's it. Data test. Welcome to the beta test.
Speaker 3:Ciao, Bye everyone.
Speaker 2:Bye.