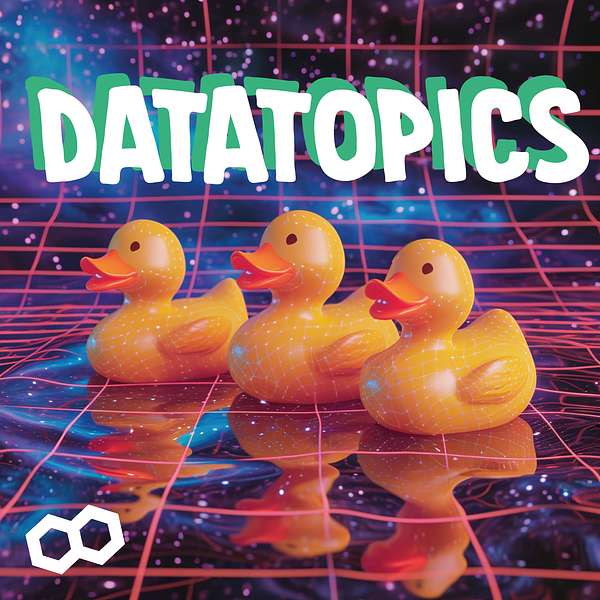
DataTopics Unplugged: All Things Data, AI & Tech
Welcome to the cozy corner of the tech world where ones and zeros mingle with casual chit-chat. Datatopics Unplugged is your go-to spot for relaxed discussions around tech, news, data, and society.
Dive into conversations that should flow as smoothly as your morning coffee (but don't), where industry insights meet laid-back banter. Whether you're a data aficionado or just someone curious about the digital age, pull up a chair, relax, and let's get into the heart of data, unplugged style!
DataTopics Unplugged: All Things Data, AI & Tech
#40 Code, Comedy, and Creativity: Navigating the Digital Data Tapestry
Welcome to the cozy corner of the tech world where ones and zeros mingle with casual chit-chat. Datatopics Unplugged is your go-to spot for relaxed discussions around tech, news, data, and society.
Dive into conversations that should flow as smoothly as your morning coffee (but don't), where industry insights meet laid-back banter. Whether you're a data aficionado or just someone curious about the digital age, pull up a chair, relax, and let's get into the heart of data, unplugged style!
In episode #40, titled “Code, Comedy, and Creativity: Navigating the Digital Data Tapestry,” we're unraveling the complex weave of digital innovation, creativity, and humor, with insights from special guests Thomas Winters and Pieter Delobelle, who bring their unique perspectives to our data-driven discussions. Here's what we've got lined up:
- AI Humor and Creativity: How do machines understand humor, and can AI actually be funny? Check out the relevant slideshow for a deep dive into AI-assisted humor: 2023 Humor Talk by Thomas Winters
- Is “Embeddings” killing Embeddings? Questioning the terminology in AI and its implications.
- Tokenizing in Different Languages: Investigating the nuances of good and bad tokenization practices.
Do it.
Speaker 2:You have taste in a way that's meaningful to software people. Hello, I'm Bill Gates.
Speaker 1:I would recommend TypeScript.
Speaker 2:Yeah, it writes a lot of code for me and usually it's slightly wrong.
Speaker 3:I'm reminded it's a rust here, rust.
Speaker 2:Congressman, iPhone is made by a different company, and so you know you will not learn Rust while you're trying to do it.
Speaker 4:Well, I'm sorry, guys, I don't know what's going on. Thank you for the opportunity to speak to you today about large neural networks. It's really an honor to be here.
Speaker 3:Rust. Welcome to the Data.
Speaker 1:Topics Podcast Hello, hello and welcome to Data Topics Unplugged, your corner casual of the web. We're from LLAMs to research, to whatever Anything really goes. Today is 8th of March of 2024, women's Day, so maybe can we actually get a quick round of applause to all the women.
Speaker 3:Woohoo.
Speaker 1:Well before, maybe an introduction, it's Women's Day, before any further ado as well, fun fact honoring all the women in STEM and in tech. Actually, the Grace Hopper is Grace Hopper, right, bart.
Speaker 2:Grace Hopper.
Speaker 1:Yeah, I'm asking, but I have it right here in front of me, so I know it's Grace Hopper. She created Cobol Language, which was one of the big things that laid the foundation for data processing and actually programming. She created the Cobol Language. Yes, that's right.
Speaker 2:Nice with the nook, you didn't know that. No, it was the first compiler. She created the first compiler. I didn't know that was Cobol.
Speaker 1:Yeah, yeah, no, it was the first compiler. So actually, yeah, without the women in STEM, in science, we wouldn't be here today. Maybe probably not. So you know, big shout out there Today the skills are a bit different. But yeah, and who do we have today? Maybe I'll let everyone introduce themselves in a second, but I'll just say here we have Thomas Winters, an NLP researcher at Kill Loven, specialized on LLM and creativity and humor. I have also Peter here, good friend, former student colleague. We co-published some papers and I'm using co-published very loosely here.
Speaker 2:Look up your Google scholar.
Speaker 1:Yeah, I just have one, so, but that's Peter. I have Peter did, let's say, the heavy lifting there. He's also the guy that not only on NLP in AI space, also in the real world space, he's also an expert holding a streak of over 2,500 days on Duolingo. Yes, yes, that's true, that's true. He specialized on state of the art bird Dutch models and also like how the different languages play. Llm is a very interesting topic, obviously. So I think today we have a very special episode with two people leading the industry there the research and the man behind the sound engineer, you know the the sound engineer.
Speaker 1:Excellent engineer.
Speaker 2:We have Alex on keys.
Speaker 1:That's true. He's the guy you know that keeps the lights on here. He's the guy that puts me in line the ad structure. The man that I have nothing but love and admiration.
Speaker 2:Bart Well hi.
Speaker 1:Yes, yes. So yeah, I think last time too. You know maybe this is a side note I used to not never listen to myself and watch myself. I still have a bit of a hard time, but it's getting better. You know, sometimes I listen to myself and I'm like, oh, that joke was a bit off you know, and I think it's like I just want to set the record straight here you know nothing but love, bart, admiration for you, you know.
Speaker 2:Was there a joke in the past?
Speaker 1:Maybe there was, maybe there wasn't. We'll see. We'll see. Yeah, thomas, maybe we can start with you. If you would like to introduce yourself, say a few words from facts. What would you like everyone to?
Speaker 4:Yeah, so hi, I'm Thomas. I'm a postdoctoral researcher at K-Löwe specializing in creative artificial intelligence, building language models together with Peter and doing research on that, especially like how can we train computers to detect and generate humor. And, yeah, so I. We both just finished our PhDs a couple of months ago. And yeah, oh yeah, you're on my website already. Okay, great, yeah, I think a lot of people in Belgium might know some of my pride side projects, for example, torusbot, which imitates Victorovs on Twitter or X, but I prefer seeing Twitter.
Speaker 4:We've also got Improbotic Splenders, where we play improv teacher with robots on stage. We've been doing that since 2019, 2020, something like that. Yeah, there's also plenty of other things here. There's Dog Generator, which generates completely random slide text since five years ago by the topic you want. Yeah, there's also, I think, like a tap on AI, specific projects. So these are just the main ones. I play a lot of improv theater. I combine a lot of technology with creativity. In general, that's what I love, that's what I like. That's what I want to keep on doing Really, really cool.
Speaker 1:And I see some of it is in English, I guess, and some of it is in Dutch. Yeah, some of it.
Speaker 4:I really enjoy doing the things in Dutch because it often proves that AI can already do something for such a relatively small language such as Dutch. So I mean, if you see people that's like, oh look, there's this AI that can imitate this famous English actor like Snoop Dogg or something, then you could be like, well, is that because the AI is so good, or is it because someone did so much effort into that? Because replicating such a big actor, it's worth spending thousands and thousands of dollars to it? But then if you're going to imitate someone as niche as Richtof's or characters from TV shows from Flanders, then you kind of know OK, ai is probably mature enough because no one will spend thousands of dollars trying to imitate this particular person. So I really enjoy almost like a proof of concept of something really showing the world. This is what AI can already do by doing very niche things in Dutch. That's cool.
Speaker 1:That's cool and, again, dutch is. Sometimes I have this question myself. I'm also from Brazil, so I speak Portuguese. I don't know, I haven't checked the latest and greatest of LLMs and NLP, but I remember last time I checked the stuff in Portuguese was significantly lower quality, right so, and I also understand that there are differences in the language itself. Right, the way the language is structured, like maybe some, there are some languages that are more complex to understand and not so. Actually expanding to other natural languages is something that I have curiosity there, and that's actually where your expertise comes in, right?
Speaker 3:Yeah, indeed, so I'm indeed focusing on training language models for partially for Dutch as well, together with Thomas, but also trying to adapt language models to different languages. For Dutch, that's indeed quite challenging because, although there is some data, it's typically not enough or we don't have enough compute as well, because while we're also researchers working at the university, so we try to come up with methods that deal better with that as well. Yeah, so that's part of my research. And then I'm also focusing on fair NLP, so trying to remove biases like gender stereotypes, gender biases in there. So, in the context of Women's Day, I think that's also a word you mentioned.
Speaker 1:Very true. So, yeah, maybe. So you mentioned like, well, maybe there are different ways we can actually go from this conversation. You mentioned your university. You don't have the same computational power than OpenAI has, and all these things. That's actually a question. Yeah, exactly Right. But then you start seeing, yeah, like okay, there's a new state of the art model for NLP, but also it's because they're one of the only ones that have the compute, and I think it's like maybe five years ago, if you're asking like, where's this the research innovation comes, it was going to be universities, right. And now I feel like, because everything relies so much on so much compute, I wonder if this unlevels the playing field. You know, like now it's not like everyone has the same opportunities, right, like if you have some very popular model or very new architecture that is very efficient, but maybe you still need that computational power, you still need this. You will never get your moment in the sun, kind of thing. Do you feel any difference, or is this?
Speaker 3:I mean, I think that's true to an extent. Like, for instance, what I try to do is translate models, so that you take an English model and they can translate it to Dutch. So it's a bit more advanced than fine tuning on Dutch data, but you still need to have some compute. And okay, it might be orders of magnitude less, but you still need compute and those GPUs are still super expensive.
Speaker 2:And where do you get your compute for your research?
Speaker 3:projects. So we mostly have the Flemish supercomputer. But those have yeah, they have some GPUs, but they're in super high demand. So that makes like iterating over something very, very slow.
Speaker 4:But it is something like quite scary, I think, like when you look at it, like so much of the big large language models if you want to train them or run them even sometimes it takes so much resources that it's like, yeah, it's very hard to do research on, it only becomes harder and harder.
Speaker 4:You've got all of these million dollar startups and companies that are innovating in that space, but I think we as academics can still do a lot of research and contributions on like other original, smaller angles, like something that a lot of companies might not they're doing just because, I mean, they have a bottom line to reach right there, they want to make profit at the end of the day. And I think, like a lot of the cool things that Peter, for example, has been doing is also like often, sometimes on like something very initial, very small, that then helps a lot. And I think, for example, indeed, his recent work on token translation, where it's like, can we reuse a model that has been trained on one language and then just translate the tokens and use these embeddings already to then have a baseball, if I understand correct.
Speaker 3:Yeah, that's exactly.
Speaker 4:Or then also the fairness research that he does. I think it would like you can do some experiments still on these models. You can train your own most you can to some extent still, and I think we're already lucky to have this much compute as a computer science department. I think one of the reasons that our Robert model, which we made four years ago, got so popular is because a lot of people doing NLP are also in the linguistics departments and they don't even have the computer we have. So they were very happy that we used some of the compute to then open source one of these models, which was also, I think, one of the earlier models on on hugging face.
Speaker 3:Yes, yeah, back then they didn't even have the hugging face hub right now.
Speaker 4:We even like, I think, some of the main people from like the leaders from having things doing.
Speaker 1:Pull requests to our repository to make sure everything was compatible.
Speaker 4:Yeah, really cool.
Speaker 1:And you mentioned also the token translation. Yes, I'm assuming that's this tick to talk.
Speaker 3:Yes, indeed. So yeah, we trained the last version of Robert with that method. So we took an English bird model or a Bertha, and we translated it.
Speaker 2:Maybe explain, like for the people that don't know what a bird model is.
Speaker 3:Yes, so it's. It's a language model. It's typically used for classifying texts or getting embeddings out of it, so you get a representation of your text. So it's not a generative language model. So it's also a bit smaller, but back then that was quite a big model. But yeah, so, and what we do is we take an English one, so Robert is trained by Facebook on, I think, thousands of GPUs, and we translate that, token per token, to Dutch, and then we still fine tune it a bit, but that works really well.
Speaker 1:So yeah, we also. And how good can you map tokens from English?
Speaker 3:to Dutch it's a bit of a mess, so it works. It works. Thing is, some tokens map very well Things like I maps to IK because that's in both languages, but then you start to get two tokens that are split up. And then it's way, messier, because they're not split up in the same ways.
Speaker 1:What about the? Because I know in Dutch, like sometimes, one word is just like Compound words.
Speaker 4:Yeah, how do you.
Speaker 1:How do you manage that? Well, maybe explain what it is for people that are familiar with it.
Speaker 3:So yeah, the compound words in Dutch are basically all glued together Like same in German. So if you have seen those memes about German words who that are super long, like the egg breaker thingy- what is that breaker thing? So there's a word like I, or Shalen, like my German is bad, but it's to break your egg.
Speaker 1:So it's like a, like a device Break your egg.
Speaker 4:But so in Dutch we also have this thing, where we glue together a lot of nouns, which confuses the tokenizer a lot, right, because tokenizers especially like a PPE once they're trained to, basically as a compression algorithm, right? So they're trying to compress the corpus that you're training on in a subtle. We have a beautiful that's the word.
Speaker 2:Anyone wants to give it a try? We have the German word here on the screen.
Speaker 1:Actually, Alex is German.
Speaker 2:The bus passed by.
Speaker 1:I just saw an opportunity there.
Speaker 2:I think it's go for it, bart.
Speaker 1:Really, you can ask the Brazilian of all the people make it a bit as a tariff. I hear Shalen slow Bruce tell Len the Rusa say and like breaker, that's that's what I said. No, no, no translate. So basically, compound word yeah, basically is like a whole bunch of stuff glued together.
Speaker 4:Yeah, and you say yeah, and when you have these PPE tokenizers.
Speaker 4:I mean they're basically trained to to compress the entire Internet in as few tokens as possible Possible rights, but they completely disregard morphology and they might split up word up in things that don't make sense and are actually not representing the actual nouns that are in present in this compound. And one thing we found when we were training our robot model four years ago was when we just used the English BP tokenizer that Roberta by default uses, trained on English text, and then we trained a model again, but then on one where the tokenizer was actually built on Dutch, like a tokenizer that was built on Dutch text so that it actually respected a bit more of the Dutch, but even it didn't even respect the morphology of Dutch at all. But we found that then for several tasks that it needed way less data to get the same performance or even higher performance than our one that had an English tokenizer and also competing. So I think one thing we learned there is that, like these tokenizer are definitely like under researched, I think like, especially if you look at things like that's why we have all of these compound nouns and other morphological things that they want to have in there and in a lot of ways, like tokenizers are to me still like Achilles heel, even like GBT models, because, like when you want to do rhyming or like something where the syllable count is important and these models have such a hard time figuring out like how many syllables or how many, or how does this word even look.
Speaker 4:Like there's this joke where you can say, like give me synonyms of this particular word that started with this letter, and then even GPT, for sometimes trips over what the first letter of a word is, because it doesn't know what that token looks like. And so there's like still like so much improvement that we can do on these tokenizers. There's so many things wrong with the way that we have the tokenizers right now, which are just trained as a compression algorithm to have as much text as possible in as few numbers as possible. That, yeah, it's.
Speaker 4:It's weird that we, and the entire world is spending hundreds of millions of dollars training them like the models, but not looking at like how can build better, tokenize it, for example, respect morphology better, or like these other constraints or things that languages have.
Speaker 1:Yeah, I'm also wondering, like if every time, because I like, morphology depends on the language, you have right, so does that mean that you need to almost start from scratch every time you go to a new language, or?
Speaker 3:Well, you need to train a different tokenizer for a new language.
Speaker 1:typically, anyways, like what do you mean by the sort of training and tokenizer?
Speaker 3:So you have your corpus and then the first thing you do is you look which words or which sub words are occurring a lot and you use those to train or construct your tokenizer. It's really just the most commonly occurring words and sub words get their own token until a certain threshold and then after that you start to represent words by using two sub words or three or four.
Speaker 1:So then, like even the way you split when I'm saying words and sub words, because, from At least when I looked into the tokenize, is in hugging face and whatnot is because they split word like translating. They could split a bit in translate and then the I and G is so common like so but even the way that they split these things are also Determined based on the data you have. So I guess the I and G, something that is very recurring.
Speaker 4:So they're going to say this is going to be separate talking yes, but, but there might be yeah, so sometimes it's something meaningful, like I and G does mean something like it's. It's conjugating a particular, but the tokenizer doesn't actually know anything about a morphology of the language. It just figures that out because it's like basically trying to compress a large corpus into as little numbers as possible, so it just finds the most common substrings.
Speaker 3:We had one, so we updated our model to 2022 data and then we tested for like which new tokens are in there related to COVID, and there was one COVID measures split up in Corona measures like the first letter of measures.
Speaker 4:Yeah, and it's like it's also like this ugly artifact that you have, because a lot of words you sometimes spell with a first capital letter or sometimes without a capital letter. So there's often like words where you just have like the word without the first letter, because then it just swaps between the small letter and the big letter in the tokenization.
Speaker 3:Oh, with a space in front of it or without the space where you basically have four variants of a word.
Speaker 4:Oh well.
Speaker 3:Yeah.
Speaker 4:And these are like the things that all of these language models use, right, because most of them use BPE, because, basically, people made a trade off back when they wanted to tokenize it right.
Speaker 1:So you could say like if you want to use a BPE by pair coding.
Speaker 4:And so the idea behind it is you want to have as much text as possible within your context, right? So you basically want to find ways of representing that text with as few numbers as possible, and so usually, when we normally map a text to numbers, we just say let's use Unicode and we just encode every letter as a separate character. But that means if you have a context window of, let's say, 256 tokens for example, well then you can only encode like a tweet slant, basically right. And what Bert did, the Google when they made Bert is like they basically encoded the most popular words and they gave all of these words a fixed number, but then you only have like the top four, four. What's the 40,000 words?
Speaker 1:Yes, that's encoded.
Speaker 4:All other words are just like unknown token mapped to that one.
Speaker 4:But, then if you do that, then you have for, especially if you have like new words or like other languages, you have just like unknown token, unknown token, unknown token, unknown token. So what's the? The middle ground between these two, between encoding letters and words, is just like saying, well, let's find these most common substrings and we give them a number. And so that's why when you, when you use GPT, for example, they always say like you get so many tokens back just because they don't reason in words. They reason in parts of words, these substrings. And it's pretty interesting if you look at how text is tokenized, like it doesn't follow the things that we do. For example, if you encode Gothic, I believe it's like G O, o T H, and then it's like it's like three and it's like not more logical, making sense, it's just like, but it's like because O T H is probably like a very common token and.
Speaker 4:I see at the end is also like a common token. But it's like this, this middle ground that they had to pick, because either either you're going to have very little text that you can input or you can have a lot of unknown tokens. Let's do this middle ground. But it's middle ground because it's basically modeled as a compression algorithm, like how can we get as much text as possible? You're going to get at these weird artifacts where, where these, these words are not, yeah, making more logical sense.
Speaker 1:Yeah. So then this yeah, I see what you're saying, I see what you're saying. So, basically, and then also, what's after you have the tokens? You still need to come up with an embedding for the token.
Speaker 3:Yes, so that's the training, the language, yeah, and then you still need to do that.
Speaker 1:And then, after you have the, the tokens, I guess you still need to train the machine learning model. Yeah, that happens at the same time, so you have the.
Speaker 3:You train the embeddings that map to the tokens and you train the entire language model at the same time.
Speaker 1:And maybe when you say embeddings, do you want to explain what that is for people that never heard the term before.
Speaker 3:Sure, it's basically a list of words, so of a list of numbers that represents your, your word or your token. Yeah, and then with language model, like birth, you can use an embedding to get an embedding from an entire sentence, which is nice.
Speaker 1:Yeah, the reason I also has this is I came across this article that I thought was interesting. Basically it's embeddings killing bad things and basically they're just saying it's a bit of a for segue, but anyways, basically they're making the comment here that embeddings confuse people and I think nowadays, with ChagYPT becoming so popular, like everyone is talking about embeddings. But he, like embeddings has a meaning in English, right, like to put something in right, and then that confuses people. That's that's what he, that's what the argument here is right, and that doesn't happen when you say prompt engineering and when you say fine tuning, because these words are more accurately represent what is what they're saying, right? So I think the example he could put here is like the knife was embedded in his chest, right. So embedded in the sense that like to put in right. And then when we talk about embeddings now is really just saying like we're mapping a word to a sequence of numbers that are meaningful.
Speaker 1:Yes, you're embedding a particular word into space right, yeah, exactly Into a vector space, right, but then yeah, so what would then be the correct way to he? So the are the action I think I'm saying if you get Steve Jones, this guy, he's using me, he's proposing instead of using no. It embeddings is the correct word, but he's saying like for us, that's fine, but whenever you're talking to people that are not in the field, so most people that are a bit more intrinsically or intuitive, exactly, and then he mentions that should be.
Speaker 1:He will propose something like source translation, because you're translating from English or natural language to machine.
Speaker 4:But that just sounds like you're doing a translation task.
Speaker 2:Yes, I agree, that's source. And what is the source then? Because you can go to the embedding, but from the money you can go back to the words.
Speaker 1:True, but I guess it's like you can say like, actually I don't, maybe we're getting too caught up, because I think what his main point is like we're going from a machine representation, something that the machine learning models understand, something that is natural language that humans understand, and then he says well, he defends that if you were to use this terminology would be less confusing for people that are not data science, space.
Speaker 4:But I mean, if you're going to use source translation in natural language processing, then it does sound like you're doing a translation task which makes it even more confusing.
Speaker 1:Yeah, I think for us. Yes, I mean I think when you say, if I say like there's a I guess maybe like image embedding, then exactly I guess.
Speaker 2:But isn't, that was his name, steve. Yes, you're not trying to solve something that is not really a problem.
Speaker 1:I mean I never thought it was a problem. But then I read this article and I was like, maybe because they also say well, if you're in the field, you know exactly what it means. So I never really thought of that. For me was very obvious because we've been talking about so long. But and I actually never had an issue when I was talking to someone that is not in the field and I say embeddings and explain what it is, and they're like, oh, that's so counterintuitive, you know.
Speaker 2:I think I still need to explain what the source translation is. That is very true.
Speaker 4:And it seems like his article is also confusing things a lot by intertwining into prompts. It's like yeah, no, it doesn't have.
Speaker 1:No, I think he like what I mean nobody is like return the sort of information to prompt and stuff.
Speaker 4:It's like you can have embeddings without sure.
Speaker 1:But maybe you made me think. One thing I do have a bit of an issue with is the word AI, like to say artificial.
Speaker 2:Oh, this is a very good topic.
Speaker 1:I don't like the name and that's when I hear what everyone thinks. Is this your hot take for today? No, no, that's not my hot take. I will have some hot takes, but I guess it's just like I don't like AI just because I think it invites the conversation, the whole sentence, the whole intention, the whole wills and desires. Because when you say artificial intelligence, people really think it's like a little robot that gets sad, that gets happy, that wants to do this and that.
Speaker 2:But what is your?
Speaker 1:alternative, I would even say like pattern, statistical pattern, I don't know.
Speaker 4:People might argue that it's not really statistics. Right, because I had this discussion with someone recently and I was like why do we call it like automated statistics? But then it's like yeah, but statistics is actually cleaner, because in AI it often feels like we're steering in a pot. Until what about?
Speaker 1:like pattern recognition, something, something.
Speaker 4:But then you're going very neural because, like, if you look at logic and reasoning and these kind of things, they're all in AI but that doesn't necessarily mean that they're doing pattern recognition, I think.
Speaker 2:AI is not necessarily like. The term AI is not necessarily a problem. I think, like because it's a very, very, very high domain, that there is a lot of vagueness like what is AI? Yeah, predictive models is a bit of a statistic.
Speaker 4:I think the annoying thing about AI is maybe like the moving goal post part of it, right. Because, like, if you look at a couple of decades ago, when you were doing breadth first search and depth first search to solve games, then people were like, oh, this is AI.
Speaker 1:Yeah.
Speaker 4:But things, as soon as we understand something, well enough, it's like no, no, no, we don't call this AI anymore. Like, and if you have a decision tree, it's like, oh, yeah, but I can understand this, it doesn't. That's not AI anymore, like I. But then I feel like, even like with neural networks, like we get to basic ones and it's like, oh, just like a one layer neural network, I wouldn't call that AI anymore. Well, it's only one. So it feels like we're always defining AI as like the thing, what that's on the border of what we kind of understand and we're constantly moving like what, what?
Speaker 4:this?
Speaker 1:is, I think, with the chat, gpt and all these large language models, because they're so big and like they're black box, you know, like it's really hard to really see how we tune this, this happens and this, that happens. You know, and I think because we don't have the very clear view, then the AI argument becomes even more. You know, because do you say you really understand how all the knobs and whistles are working there?
Speaker 4:and maybe maybe yes, maybe no, you have an idea. But is it important that a human understands? Because I don't understand how your brain works, but I can see, like all your intelligence, right, well, I'll take that you heard it here.
Speaker 1:First, no, but. But I think it's like, yeah, because the deep blue example, right, like the chess, like you know, they said one wants to repeat the best chess player, that's AI, right. And then they did it and then turns out it was just like breath search or depth search whatever.
Speaker 1:Basically just kind of explore all the possibilities. You have a very powerful computer that can try everything, 20 moves ahead and say, well, if you do this, you're probably going to be in a better position later, right. So it's like you say, okay, that's not, okay, that's not AI, because it's just brute force, right. But it's very clear, like you can say, yeah, it's doing this, this and this. And if I had a pen and paper I could do this, if I had all the time in the world.
Speaker 4:Yeah, but if you want to, you can do gradient, descent and stuff by a pen and paper if you have all the time in the world.
Speaker 1:Yeah, but I think it's like with Chesh, pt and LLM's. It's like the argument is not as clear. You know like, because you really say, like the black box, you know it's unexplainable. And then it's like, well, if you don't know how to explain, but it's doing these things and you can like if I say, hey, do this, and then he says oh, don't, don't be rude to me.
Speaker 1:He's like oh, maybe that's a real person there, you know, but it's also just predicting the next, and that's the thing I feel like AI invites more that discussion to the conversation and I think if you just say well, I just predict the next word.
Speaker 4:so it's not really intelligence right?
Speaker 1:Because again, and I agree with your point AI is such a hype term that is everyone's thinking, everyone's talking about it and some people that don't really understand how it works are just talking about it. I actually was in a Picon in a conference that the guy he was going to talk about AI, on the blockchain, and then he started talking about AI and free will and how the model shouldn't be censored because they should have free will, because if it quacks like a duck, then it is a duck, so I don't care, so we should get free will Like, really exactly Like it was like, and this guy was in a conference, he submitted these things.
Speaker 1:He's like, he runs, like, like, and it was on the blockchain, I think so.
Speaker 4:I mean that already discredits.
Speaker 1:To be but to be honest, like I didn't even, like we didn't even get to that, because he's like it was very like it was it was yeah, but I mean, it's nothing new that people project a lot of personality and things onto AI, right?
Speaker 4:If you look at, for example, even Eliza 20, no, sorry, 60 years ago, eliza, the thing that Chad bought, that sounded like a psychiatrist.
Speaker 4:Exactly so. That's 1964. So yeah, so 60 years ago. So then people were already like projecting. I was like, look look at this thing that can think. And I mean that's nothing new. And I don't know if changing the term AI will really change that. But I do get your point that intelligence is such a loaded word we don't even understand intelligence ourselves. That's also a problem with computational creativity and creative AI. It's like what is creativity? What does it take for an AI to be creative? And I'm just like my. My general idea is like if it contributes something of value to someone's creative process, then that's enough for me to call it like creative AI. And I think the same thing with with just AI in general, like artificial intelligence. If it does things that help in some intelligent task, or maybe like even does it itself, then to me it's fine to call it like intelligent.
Speaker 1:But let's go there then, like for creativity, right, like you mentioned, that if it's something that helps you be creative, then it is like it would. So let's imagine I have chat GPT and I'm trying to write a book and I say, chat GPT, this is the setup. Give me 40 different ways how this story can go. And maybe I won't even take it and copy paste, but maybe it's like oh, that's actually a good idea, but maybe I'll change this in this. Would that fall in the space of creative AI or for you?
Speaker 4:I would think so. Yeah, I mean. For me it is just like creative AI is whatever is helping you in a creative process, and I mean the level of automatedness in that process doesn't really matter too much to me, as long as there is a bit of automation and it's fine to call it creative. In my personal opinion, no, but that's fine.
Speaker 1:But also, in how do you so your research is, in that area as well, correct? I think the thing is a bit tricky also with the limbs, and we talked about this before. It's like how can we say this is better than this? And even with the limbs it's tricky, right, because we see the benchmarks, like with the Gemini, chagapiti, this Mistro, right, like there was a lot of them, and then it's like they have a lot of promise, but then we try and it's like not sure, right.
Speaker 2:And it's hard to quantify. Yes, I think we talk creativity, especially that's always been like.
Speaker 4:This has been the bane of my whole PhD. It's like how do you evaluate creativity, Right? It's like all of the all of my colleagues were doing nice quantitative AI methods and then they just check if the numbers match up with what is predicted. But in creative AI, whenever you generate something, you probably should, somewhere in your experiment, test it on humans, right?
Speaker 1:So how do you like you just have someone reading jokes from an AI and then you see how many, how much people laugh to the decibels and just I mean that would be probably like the best way of doing it.
Speaker 4:Because, like, it's very hard to to fake laughter, right. So then if you have the decibels, that's great, but that would be quite a big setup. So usually what we do is like here's a joke rated, or maybe here's a couple of jokes, which one is your favorite or which one do you like better? And then you can compare, like if I have two separate joke generators, like which one is better, the one with or without a particular component? Or maybe it's like you pick your best generator and you pick a human, and then they write jokes and you show this to other people and it's like which one do you like better?
Speaker 2:What if we talk about humor in AI? What is the state of the art today?
Speaker 4:I I recently did an experiment myself where we found that improvised jokes so when you give humans a challenge and it was like even comedians doing the challenge and you make an AI improvised joke, then the audience thought it was pretty equal, like humans at like a slight edge, but yeah.
Speaker 4:So we that's like a study that I'm still working on and it's not published yet, but we did it with a live audience of like 40 people and it was pretty similar, but, like it's, it's pretty big leaps Maybe like so just the the details on there, like is it just someone reading the joke?
Speaker 1:or is like are they? Do they just read it on text? Because I also think it's like a lot of the times in the joke is the delivery.
Speaker 4:Right, yeah, so in this case we had comedians. They, they all had a challenge and they had to bring one to themselves, and one from that was AI, generated by me behind the scene, and the others didn't even know that AI was involved. They just had to rate the jokes and then the end we told them and then we saw that, yeah, about like 30% of the times they were then the AI was better than humans, and then about 35% of times, humans were better and 35% of times they were equal. So humans still are a bit better, but it was like it was pretty close on. So if you make people improvise a joke, so not give them all the time for the world to write, just like in. Ai doesn't have all the time to write, it's also to read immediately.
Speaker 1:Then, and this the thing used to generate the joke.
Speaker 4:No, no. This is this is. This is 2017. So that's already seven years ago that I did this. This was for my master thesis back in the day.
Speaker 2:How do you generate jokes?
Speaker 4:So in here or no in the example you were mentioning then we were just using GPT for, but with some clever prompting strategies, because you need them, because when you ask GPT to write a joke, they're generally pretty bad.
Speaker 2:Yeah, exactly.
Speaker 4:Yeah, there's this for you agree. Yeah, if you just say like, please write a joke about lawyers or something, then when then in 90% of the times you'll get variations on the same 25 jokes. There was a paper showing that was pretty interesting, so it might just say like why did the lawyer cross the road?
Speaker 2:Kind of thing, let us write it.
Speaker 1:Wait, why did they cross the road? Don't leave me hanging just to get to the other side.
Speaker 4:That's a thing like these, these AI models, and like GPT for, is getting pretty good at it, but it's like still pretty bad. But like a lot of AI models, they're just well picking up patterns, right. And you can tell that when you ask for a joke in even like an all generations of AI that we've seen over the past so many years, you get things that are pretty good at mimicking the structure of a joke, but not really the punchline of a joke, something that makes it funny. And the reason for that is quite simple, right, because when you, when, when you tell a joke, people love because there's like a world model in their mind that makes a switch or jump right.
Speaker 4:So if you take the joke like two fish are in a tank, says one to the other, do you know how to drive this thing? Then, up up until the word like do you know how to? You might think of like the fish being in an aquarium. But then if I say that we're drive, then your mind's on this like, oh, this doesn't make sense. You can drive an aquarium and it jumps to to fish that are in a military vehicle and you have this with so many jokes, like if you have a bun. You have two interpretations.
Speaker 4:If you say something sarcastic, like there's so many like, so much of a joke is just like jumping from one frame of reference that you have built up into your head to then realizing like, oh no, this is wrong, slightly panicking and getting a new world model, and then the release of this tension by just laughing that away. But if you want a computer to make a good joke, it has to kind of realize that there's these two frames of references that humans can jump from. And that's often very hard because, well, if it's too easy of a jump, then we don't laugh because we saw the joke coming. If it's too hard like if it's like a very difficult connection, like, say, wikipedia pages are sometimes like linked with three clicks away, we have to go through very obscure knowledge, well, then we're also gonna laugh because we're just gonna be confused because we never jumped to the second world model and so in a lot of ways, like humor is like an excellent test batch of like AI.
Speaker 4:Just because it's like if you really want to do that humor in general, like for all types of humor, and you want to be able to generate these kind of things, well you're gonna have to have a lot of functional equivalents that can kind of estimate, like, is this not too easy or too hard?
Speaker 4:You need linguistic capabilities, you need cultural references, you need like there's all these kind of capabilities that you need for a proper joke. And we just saw, like for the for the past so many decades because, like the first real computational humor systems were back in the early 90s A lot of these systems were quite bad and like at best we could get like half the frequency of of human funny jokes. So like, yeah, if humans made so many jokes and all the frequency of that computer might match it, but then that's if you particularly hard coded for a particular type of joke. So, for example, like I like I like my relationships, like I like my source open, that's like a computer generated joke, yeah, yeah. But like I like my coffee, like I like my war gold, and that's like from like a paper from 2012, where they just for that particular pattern.
Speaker 4:They just learned relationships between three things, and also something I extended in the in the and you have prompting tips, yeah, so.
Speaker 4:So I think we see a lot of these prompting guides floating online online right, where it's a lot of ones that are pretty weird, which like take a deep breath and like and you have to give it a tip.
Speaker 4:But, like, when you actually validate these kind of prompting strategies, a lot of them are just good for some tasks, but not for different tasks. But I think there's like the big three one that you can use to basically help automate almost any task, right. So there's the, the few shot examples. So if you just give a couple of examples of what you want to solve or what you want to generate, like GPT is very good at picking up patterns. This is how we had to prompt engineer before we had the, the GPT that could follow instructions. You just gave it a lot of examples and it picked up on the pattern and then it's, yeah, basically imitated that. So giving examples always great.
Speaker 4:Then you have the, the chain of thoughts, or like splitting complex tasks into simpler ones. That's always something that helps a lot. So the, the, the basic trick, would be like you say like that's thing step by step, or or you give it some examples. That's even better If you can do that. Give it some examples where you say, given this input, I have these reasoning steps and then this is the output.
Speaker 4:Or what I do a lot for for humor is like well, when you write a joke so I do play a lot of improv teacher, I teach a lot of these techniques. And if you actually teach these techniques to to the AI, where you say, given that you get a headline of something in the news, or something you can do is first analyze what the topics are about, like to topic handles, so to speak Then you can say, well, let's find some connections between that in a second step. For your third step, write a couple of punchlines that you think might work here, given this link, and then, for your final steps, select the best punchline and then write a setup for this punchline. And if you do that, then suddenly your joke quality gets way higher, because suddenly you're not asking for like, please give me a joke, and it just mimics it with like a pattern and it fills in some word into a pre existing joke. It will actually like do some brainstorming steps before are you gonna try something?
Speaker 4:Bart, can we try this life? Sure, but then you might have to write several things.
Speaker 1:Are you gonna there we go, this is live.
Speaker 2:Can we? Can I give a topic and you give the prompt to?
Speaker 4:sure, but I'll need to type a lot, so okay. And this is the third trick. Also a third trick, by the way, that I forgot is giving context right. So if you give context of like what is it that you're trying to solve, or preferably you're going to say like you're oh no, this is gritty, it's gonna go great. You're a boy, oh boy.
Speaker 3:Well, thomas is struggling. I guess I can also say something like the chain of thought prompting. So there you really ask. Okay, let's think step by step. You need to do that before you ask for the answer, not the other way around, otherwise it will give a wrong answer, hopefully a white one. But it, like a language model, generates a token, one by one, from left to right, right. So if you then first ask it to generate the answer, it will only use what it has until then, and then afterwards it starts seeing okay, I gave this as an answer, why? And then it just invents something, because it also doesn't know why it generated that. That was just the most likely thing. So if you first ask it to reason, it can look back at that reasoning, understand that reasoning and, hopefully, that reasoning is correct.
Speaker 3:And then it uses it for classification or something Interesting.
Speaker 1:So like it's the opposite of what I usually do when I'm sending emails. Usually I just put exactly what I want and then I try to give some context, because if I put it at the end I found that people don't really look over. Like I think people like people's attention span. It goes from like paying attention in the beginning and then like okay less okay, less, and then sometimes they don't even get to the end, so he actually like.
Speaker 1:I had a class in the US, like on English for engineers that they were like. Actually the class was interesting. They talked about big disasters in engineering, like the rocket that exploded and how communication was a big issue there, and the first thing she says is, like always put what you want in the beginning and then see why I do that a lot with you, Bart, as well, Because I know you're a busy man, you know. So it's like yeah.
Speaker 1:I usually put like hey, can you do this? And there's like I try doing this, I'm thinking this is this. But like usually, I always start with what I want, but I'll have to it's not smart on slack. What do you mean?
Speaker 2:So you slack for internal communication, like if you start with what you want and then you have a whole explanation. I'm only going to read the last line.
Speaker 3:Yep go the input is at the bottom. So Exactly.
Speaker 1:So you're like chat. That's why I don't never do what you want. Yeah, life.
Speaker 2:You see that it seems like it's not fitting on the full image.
Speaker 1:Can we have a look at that Can?
Speaker 2:you fit it somewhere One way or not, or is it maybe just?
Speaker 1:this but also maybe a question, and maybe also everyone makes any headline into this headline yes or.
Speaker 4:I now made one for headlines. Usually, what I would also do is add some examples where I say, given this input, I would follow these reasoning steps. And then there's some output.
Speaker 2:I do writing notes with news headlines, like just anything.
Speaker 4:Yeah, anything could be anything from the news, something that you might want to talk about or like a.
Speaker 1:Dutchman walks into a bar. I don't know, not the punch line.
Speaker 2:So maybe in the meantime you need to.
Speaker 1:So right now for the audio people, we're on Chagypete 4. We're doing this live, so we're not liable for anything that the model spits out. Basically, there is a prompt there it's an initial prompt saying your world renowned expert on comedy, with a proven track record and writing jokes about news headlines. Maybe just a side comment. I don't know if we mentioned this particular, but I do know that just by telling Chagypete or the model you are an expert on this, usually the output is significantly better.
Speaker 4:So, yes, and that's what I mean, like providing some context. But then the best kind of context is saying you're an expert because these models have trained on the entire internet.
Speaker 4:Yeah, if you say please write a poem about something, something. Well, I mean I'm stretching the truth of it. But then it's it's like well, I've seen so many poems online, I'll just give you one of these random ones. But if I say like, no, no, you have to pretend to be an expert, then it's kind of finds like the room where it's like more expertly written poems rather than an average poem on the internet.
Speaker 4:So like reinforce learning from human feedback during the training. When Open AI did, that already made the quality kind of already better. But like in general, that's like why you want to like condition the model often on these kind of things that push it towards the more interesting spaces of yes.
Speaker 1:So maybe it's a quick tip for people using chetch PT, which is everyone. So that's, that's one thing. And then afterwards there's whenever you write a joke, you follow the following steps select topics and use headline. Write a list of these links. Write a list of links these topics from step one can have, write all. Write a list of punchlines related to these links. From step two, select the funniest punchline from step three, write a setup for the punchline from step four. So basically it's like a step by step Do this, do this, do this, do this, and then the input from Bart's. Yesterday, marillo, a famous podcaster, found a drunken pink unicorn on the side of the road. His interventions were able to save the poor unicorns life. All hail Marilla.
Speaker 2:But if I must set you correctly, you need multiple headlines here.
Speaker 4:No, no one is okay. But like, what I would usually do to get even high quality is say like here's some inputs that you might catch and then some reasoning steps that I someone would follow.
Speaker 1:Yeah and then.
Speaker 4:So now I explicitly wrote the steps here, but what I usually would do is give reasoning steps for that particular type of input. So this, this might not I. This is not the best prompt I. Usually my prompts are a page or two.
Speaker 4:Yeah, yeah, yeah, like this is getting the general idea of, like you give some context by saying pretend to be an expert, you're giving it some steps and this way, instead of it having to immediately solve the problem on its very first token that it generates, you're saying take it easy, take it slow, you can have some draft paper and write out some steps and then you can use these intermediate steps to write a joke. So now, if you press save and submit, we should be able to.
Speaker 1:let's see, let's see drum roll. Do we don't have a drum roll sound, do we? Bart? No, alex, no, sorry, maybe next, maybe next time. So now what's happening is the chat is actually writing down. The steps from the before is actually like it says. Step one was this and then give some bullet points. It's a lot to read so I'm not gonna try to read. Maybe you want to read it by. I don't know what to read.
Speaker 2:What should we focus on here? It's written to there.
Speaker 1:So if we want to put the joke together, yeah, you have to.
Speaker 4:You have to just look at the last step. Yeah, because normally you can also add a step that says then write out the full joke.
Speaker 2:Yeah for the people listening, like the output of just to be these now giving whole, like it's processing all the steps and giving output on every step. And now we can ask put the joke together.
Speaker 4:Yeah, sure, let's try. But it's if you read step five and step four, then we also can in a joke, but we're not that advanced in humor, yeah no, we're talking just humans.
Speaker 1:Yeah, why did the pink unicorn argue it wasn't drunk? It insisted it was just tasting the rainbow, not drinking it. Do we have the crack crack?
Speaker 4:That's something else, that's okay. I think what happened here in this case is I should have said that it should only use two main topics, so that was in step one already that I messed up the problem. But it's tricky.
Speaker 1:I think it gets very complex. I think, it's very interesting how you're almost breaking it down on the science of humor, which I think is also very but it takes a lot of iterations, right.
Speaker 4:Yeah, so I.
Speaker 2:This is a very good example of this is the first iteration and you're just sort of proving on that.
Speaker 4:Exactly exactly.
Speaker 1:I have a question as well. I feel like not because I'm like this, but sometimes jokes can be can play on stereotypes, and stereotypes, if you push it a bit further, can be.
Speaker 2:Were you triggered by the pink unicorn?
Speaker 1:No, not really. But if you said like, oh, murillo's a Brazilian guy and he, I'm sure that's something borderline, not nice.
Speaker 2:Okay, it may come out.
Speaker 1:It may come out and I think it also taps in your fairness. Yeah, it's an interesting answer.
Speaker 3:Yeah, like fairness joined with humor. We've talked a lot about it but we actually never did something about it. But it's just measuring fairness or how biases are in language models is already super tricky and then mitigating it is even more challenging. Like you saw what Google tried to do with the image generation that went. Then it just went completely to yeah, fair images, but at some point it's not fair anymore. But it's Difficult, eh.
Speaker 1:Maybe could you explain what that was from Google.
Speaker 3:Yeah so Google made an image generation tool in I think it was in Gemini and people started noticing that they added some stuff to the prompt so that it generated more racially and gender diverse images, which, I mean, that's not necessarily a bad thing, but then that was applied to everything. So it was applied to, when people ask it, medieval vikings those were not the vikings that actually lived in the 12th century or popes Like there is not a single black pope or female pope but then it started generating that which, like, at some point it becomes fiction, and that was what people were concerned about.
Speaker 1:Yeah, also with this bias sometimes I have a hard time, like, for example, one thing I get not so infrequently is like, oh, you're Brazilian, oh, you must play football, and I'm like, wow, I need those and I do, right. But then it's like, it is a bias, I guess, like stereotype, right, but to a certain extent, like, for example, we actually did the last podcast, we're showing some image generation and it's very fast. And if I type like Brazilian, either women we're going to show up with like a bikini or something like that, you know, it's like when we say Brazilian, when we say Italian, when we say this, you have an image in your hand, right, and it is a bias, right. So in a way, you don't want to just an image of a person.
Speaker 2:It is a bias, but maybe it's a correct representation of the things that are right.
Speaker 4:Is it the correct representation though? Because I think a lot of like in AI. There's a lot of amplification of these biases. Right?
Speaker 2:Because in AI, usually when you train it, but I mean a correct representation of the training data.
Speaker 4:But then even still like, for example, when you look at VAE's training on amnesty images right and in the images they are black and white, hand drawn digits. But then if a VAE generates again, like through the bottleneck, like an image, again you get like grayscale things that are not present in the dataset. Why is it generating these grayscale ones? Because these are the average between black and white and often AI models are stimulated to be as close as possible to a particular prediction, so you're averaging out a lot just to have more probability If it's white or if it's black you're still quite close.
Speaker 4:So I think, like in a lot of the cases, the problem is with neural networks. The probabilities I get out are not actually calibrated with reality. Like you get. Like, for example, this GPT says like I'm so like so much percent convinced of this next token, that doesn't mean that that is actually reflecting the reality of the training data, just like when it's when it's predicts that token, like yeah, then, then it's most likely to be correct.
Speaker 1:So it's more like fairness in LLMs and AI is more like you want to like if the curve shifts to the right. You want your curve to shift to the right, but not more than they should be, not more than what you actually see.
Speaker 3:That's a bit depending on what you want to do as well. Right, like, if you like, we work with the Flemish public employment service and we have a data set of resumes. And what you don't want is that you have a model that says, ok, this is the resume of a nurse, and now I get the resume of a computer scientist or of a man, and just uses the link between female and nurse to then just classify all the resumes of women as nurses and then of men as computer scientists.
Speaker 1:Yeah, and I think that's what you want. Yeah, I actually saw. I heard maybe I should fact check this that Amazon also use some AI to screen CVs, but then they would screen out women because training data, but it is like, and I completely agree that it's wrong. We should not do this Right, but at the same time, it is On the data that they trained it on, probably, and that's why you want to be conscious and you want to correct for these things.
Speaker 4:So I guess it's like it's like and I think that's what Google was trying to do with Gemini and like you want to add some some and it's interesting that they got a lot of slack like a lot of issues For that online, because open AI was doing the exact same thing with Dolly to when it came out, I think two years ago.
Speaker 2:Exactly yeah.
Speaker 4:No, no, they did the exact same, though, because they also added to the prompt like. Whenever you create a person or like, for example, if you edit, if you said please generate me a CAO, then you usually get white males, but then at some point they fixed it and people like oh yeah, exactly the fix it and then but then.
Speaker 4:But then the the fix was just that they added, whenever there was a Job or a person being mentioned, that I just added black or woman or these kind of things to the prompt, and people Exposed that by saying a person holding a sign that says and then you got sign like people holding a sign that just says black or Woman and they did the exact same fix that Gemini did, namely adding things to the prompt. But then it's interesting that so many years later, just the exact same thing happens.
Speaker 2:And then you and before that they got flack for the opposite reason, like what you're saying like Generated an image of a CEO. It's always man. Yeah and so it's good that actions are being taken right, because that's not what we want in society, and and the demonizer is an example, or maybe it's step two far.
Speaker 1:Yeah, so maybe also Is this. You mentioned the CV screen. Is this what you're talking about?
Speaker 3:Yeah, so this was a tool that we build based on those resumes, partially, mostly because we noticed that a lot of the resumes and they were not very well written. So, yeah, this is not screening resumes, but this is trying to help people write the resumes. We still have a TC student working on this. Yeah, it's still work in progress, but, yeah, the idea is just, with GPT and with resume, robert, as we called it, to generate interesting outlines that map to what in resume actually should look like and and then give some suggestions for skills what I, what I notice in our own job application process, and a truth is that nine in ten people today for a motivation letter use chat.
Speaker 2:Gpt is and it has become completely useless, like because it's so generic, the wording like it's very clear as it doesn't come from the person anymore, that it's also like you lose a little bit of authenticity in these things as well.
Speaker 3:How much was actually? Yeah, how much value did the situation that you normally have yeah, but was it not just copied from, like the last 20 applications that they did and then changed the?
Speaker 2:but I think there's not a discussion. I think there is a fair point, but but Like, what is the value of motivation? Right, but what you see here is that because everybody uses chat, gpt, and suddenly everybody has the same yes. I think you have this, the same danger, when you talk about resumes.
Speaker 3:That you have this which becomes bland, and everybody Expresses it in the same way to be fair, like these are resumes for people working or not working and having trouble finding a job, who are a lot at FDF B, and a lot of these resumes don't have any information. No, no, this is for your specific part, but I mean more in global.
Speaker 2:I think, in global, we will see more people is starting to use this, for I just came out of school. I need a resume.
Speaker 3:Let's use just GPT for it, yeah but if it's an accurate representation of what skills you have, why why shouldn't it be?
Speaker 4:Yeah, you're democratizing the skill of resume and I think for resumes it's still like very concise information which has to be accurate, easier. I think, indeed, like one issue that you see is the when you generate something with GPT, like you get these long texts, whether you don't have to put effort in. So One thing and I think that might even be a good thing for society is the, the devaluation of these long, complicated texts.
Speaker 4:So, and and I feel like we're kind of Finally making it more efficient, maybe for us because now we're more value. You're late. Value in these kind of bullet points is, so I can imagine that maybe for your next motivation that around that data Roots, you might be like oh, please write us a bullet point list of things why you Want to work here, rather than putting these bullet points into chat GPT and then having to go through all of the noise in there, that's with all of these favorite words of GPT that are very recognizable.
Speaker 1:But I think the the main thing is like the motivation letters. There's clearly people that just say write a motivation letter for this company, you know, and then just give some information. So there was no nothing really personal there, right. And it's like if I, if you write the same prompt on chagapiti, yeah, the words would be a bit different, but you can look and it's like, yeah, oh, I would like to go to Belgium because of this vibrant community and because of this in the history, like it's always the same stuff. And I think if and again, I, maybe I wouldn't take as much of an issue if someone uses chagapiti to say, hey, include this information about me, this is my favorite project, these are the things that I'm interested about. And then even the person reads and see if it makes sense or not, right, I think that's more of a fair game. But I think what we saw is that it's the same, but it's, but it's a good filter for you guys right.
Speaker 4:Yeah, if you see that it doesn't have any Everything things in there, then it's an easy way to filter and I think we're getting to this. Do this very weird pointing society. And I think that might be the inflection point of like inverse compression right? So, like usually, as computer scientists we're like trying to compress data in a small thing as possible. But now we get to the point where people write a bullet point list of things I want to say. They say, hey, gpt, please write this as a longer email.
Speaker 4:You get these long emails to the people's mailbox and the people Receiving a lot of mails are just like hey, gpt, please summarize these mails for me. And now you get again these bullet points, but kind of skewed out of it. So we're kind of like making the data larger and I think at some point will realize like, hey, maybe this interpersonal this, these quick list of points, it's more valuable. And maybe the motivation that this can just be like a motivation paragraph with a lot of bullet points when you say what are you actually reason of getting here? You don't have to compliment Belgium to do in order to be higher.
Speaker 1:Tear right yeah, I put. A joke I saw a while ago is like with co-pilot. I think it's very relevant. It's like there are two people in front of a computer on one side and says, hey, I turns the single bullet point into a long email I can pretend I wrote. And on the right side is like, hey, I make the single, makes a single bullet point out of this long email. I pretend to read exactly yeah, it's a bit like, yeah, we can just cut the Exactly exactly, and I think at some like we're already seeing the devaluation.
Speaker 2:When we talk about creativity. Without something like this, without genai, I have the feeling like there is more diversity in creativity. Well, now everything like you could go by default to a genai solution, like would be a text speed, images, be, whatever, and this becomes like 80% of what is out there and it's very similar to 80%. The 20% is real authenticity, which is, I think, at the same time, like gets a higher value, which is also good, but like you get less of a distribution in that 80%, I mean yeah, that's the danger.
Speaker 4:Right, we're all gonna sound like chat, cpt and and then these kind of AI models that are just averaged out and but I think I don't know. I like the value, especially when you make your own, but that kind of sound like you are like a particular character for a particular task. I have, like this, this custom GPT's that you can build them. I'm so addicted to them like.
Speaker 2:I like the value as well?
Speaker 4:Yeah, and then you can actually make it steer away from these default ways of sounding right. So, for example, for the humor prompt that we just saw well, I've got some that work really well on improv teacher and then it doesn't sound like.
Speaker 4:You would normally sound and it actually takes on a character and and improvises along and then it doesn't sound exactly like Chatship it or these kind of words that it prefers. But then of course that takes more efforts and I think, indeed, like a lot of people sound like the vanilla, unprompted, just simple instruction please write a motivation letter and then we all sound exactly like chat CPT, with beautiful words, like the advent and culmination of Accelerating yeah they had this at this university where they screened the thesis, and I found particular words that were suddenly tenfold More used in absolutely Because be just like these kind of words.
Speaker 2:Do you have any insights beyond humor or on bias, fairness, on Clots and tropics?
Speaker 4:new model Clot to I've played with it a bit, but maybe I looked at the research paper.
Speaker 3:But that's actually about it. It's interesting how they, like the, basically went out of data to train on, so they did a bit of it synthetic data. But then I try to find something about that. They don't say anything.
Speaker 2:No, not a very slow.
Speaker 4:That's in it what we see right. So all of the language models we've refused, of the entire internets. And the internet is only getting worse because everything is getting generated on the internet nowadays.
Speaker 4:Yeah like everyone is turning to these synthetic data sets and I think, like what we see in Gemini papers and clouds papers and stuff and open a ice papers is just they. They keep emphasizing, like the, the, the quality of data matters so much and like getting that filtered out or Making your own synthetic data sets. I believe opening I hired, like so many of these Programmers, and not just to help program the AI, but just to solve programming Exercises and tasks so the model could could learn better from real people, and I think that's the reality that we're kind of hitting is like we've used of the internet. We have to kind of point to either making new data sets or synthetic data sets. You see everyone training on things that were generated from other AI's and filtered out and rated and ranked.
Speaker 1:Yeah, yeah, I actually heard a while ago the people discussing whether AI has poisoned its own. Well, because, they released the models and others. So much AI generated content. And it's like if I go to chat to PT and I write a blog post and I change some things, but it's still 80% AI generated and I post it. Yeah, can they use it to train my model? Are we just gonna go? Are we gonna all slowly average out to the noise of AI? You know?
Speaker 4:yeah, a beautiful example of that was Croc from from X. That was that was saying like no, I cannot do this task because of opening a policy.
Speaker 2:Just because, exactly.
Speaker 4:And then they said well, we didn't train on GPT output but of course, on the internet test articles being written that also include like this goes against opening AI's policy.
Speaker 4:Like even on Amazon, there was like this thing. I don't know if you saw this way. If you Google, I don't know if maybe they've they've scrubbed it off now, but like if you went to Amazon and you searched for so as a language model, I cannot then you find a lot of products that just have these in there in their text. That that was just clearly generated by the company.
Speaker 4:Just all the text without looking at it. It's just like sorry as a language. Well, I cannot generate a description for this chair, or something. Is this this Might be yeah, yeah, yeah.
Speaker 2:I am Just try it out. Your a chug generator on cloth three so it's exactly the same prompt Did it on cloth three opus, which is the biggest one biggest model, and it gives the breakdown of the different steps, like we just saw on the GPT bill. Also immediately gives a joke, so it's a last step. Yeah, the setup of final joke. It says in an unexpected turn of events, famous podcast on Merillo rescued a drunken pink unicorn from the side of the road. Sources say the unicorn is now recovering and is expected to be a special guest on Merillo's net port. Next podcast episode Confessions of a drunk unicorn.
Speaker 2:I like that Can we have that right some better yes.
Speaker 4:And I think that's also something beautiful like about all of these language models, these prompting strategies, the big three, right so the few shots, the chain of thoughts and giving context and with like role, prompting and stuff, they keep on working between all of these language models.
Speaker 4:It's like a trick that isn't one of them, like people have validated these against, like the lambda, the llama, the GPT, the, the mixed rule, mixed rule of them. And they just keep working as long as your model is big enough. Like chain of thought works, like for the smaller ones it doesn't pick up very well on the chain of thought, but like you can just copy these prompts over. And I think that's maybe also something where. Where are we going towards? Where, in the future, for example, all of our phones will have a default large language model that your, your browser, can interact with. Just like. Just like, if I don't know if you notice, like the web speech API in the browser, like you can do text speech and speech to text by just calling a variable in JavaScript. That's already like and then it just uses whatever the browser provides for that service, for like converting your speech into text and and also forcing whatever text you wrote.
Speaker 4:And I think in a couple of years we'll probably have these kind of large language model interfaces in browsers and apps. Maybe that that they can then use and the fact that these prompt strategies work across all these models is also like. Well, if every phone has their own language model, then and and they and these prompt strategies work on their well. That's probably good for the future, so that not every app has to install its own language model or like keep on calling the cloud for these kind of access. And I think we'll probably not see it in the next few years, but I think that's where we're going towards, when these language models get stable enough, trustworthy enough so hallucinating a lot and get them small enough to be on the phones that will just have.
Speaker 4:These kind of API is just like we have for text speech and speech to text there is a we talked about a while ago.
Speaker 1:There's the ebrabbit m1. That is like a phone redesigned for a large, large action models, they call it. But yeah, you mentioned the future. Maybe we can. We already passed our one hour mark. But predictions, future, nlp, llms. You mentioned some things, maybe long term, near term.
Speaker 4:Yeah, I think one thing I mentioned that just large language models becoming like standard services and standard API is a maybe chips that just run these cards, large language models for your phone so that they can run with lower resources and, just like, only run that particular language model.
Speaker 4:So not a channel chip, but like I think that will probably be happening, but that's way down the line. Yeah, right now we're seeing this cheap vacation of creation, like we'll see, I think. Like just like we had word art back in the early 90s and 2000s, where everyone's turning their, their text, into beautiful word arts, that now looks very dated. I think a lot of the things it's really working on.
Speaker 2:It's like it's cool again.
Speaker 4:Yeah.
Speaker 1:I'm trying to make it. He's putting his leading the movement.
Speaker 2:I'll make word art to read again. Asky art is even cool again.
Speaker 1:It's cool, but it's because tweezers are cool.
Speaker 4:Also something that language models struggle with coming up with, new asky art just because of the tokenization. But I think like it's great for like your local spaghetti party and great for your theater shows that you can now have proper graphic design rather than someone going in paint and drawing something. But I think we'll be increasingly more sensitive towards these cheap generations because, like if a big corporation uses these generated images without any edits, without anything like it signals to the people like hey, we don't take project series enough to have a project designer.
Speaker 2:We're already there, right Like if you scroll LinkedIn or X and you see yet another generated image, you immediately yes, exactly.
Speaker 4:Like the Willy Wonka experience I don't know if you saw that in the news like they also, like there was like a failed thing, but then when you went to their website, it was also full of these generated images. So I think, as big corporations, it's like you can use them as in your design process and maybe as a mood board, and stuff.
Speaker 4:But if you are going to just plainly generate these images, especially of the spelling mistakes, because these are not very great at text, or if they have like so much detail, and then if you look at the details and the details are wrong, like that already triggers to the consumers and to people like, oh well, you don't care enough about this project, enough to take your branding seriously. So we're going to see like some interesting dynamics there with like the cheapification or like the cheapness of all of these things. Well said, peter.
Speaker 3:Yeah, well, I think like lots of more personalization is going to happen. Like, yeah, we, if you go on Facebook now, like half of the content that you would see there is already way more personalized, and I think that's just going to become more and more. Like audio books, at some point I can imagine being just generated for you. If you want an audio book about I don't know what you're going to get it you can just say I want Snoop Dogg reading Harry Potter. Yeah, like, if you want that.
Speaker 1:You just had that example on the tip of your tongue. I feel like you've been waiting for this moment you're like.
Speaker 4:every night he goes to bed, yeah, I wish.
Speaker 2:I wish it was a 2016. Open AI was created. You're like that moment that this is what we're going for.
Speaker 4:But I start the clock. But it's a logical trend that we see, right? So so many decades ago we had TV where, like, everyone watched the exact same shows and you could talk with people about what you saw. And then you had YouTube where you could find your niches and like resonate with a lot of people that had the same interest and you could talk a bit like that's about the general people that you had in real life, just because no one was watching the same YouTube.
Speaker 4:And then we have these ticktocks and the shorts and the reels and stuff, that kind of use, all these AI techniques, recommendation engines to really push you to watch Cineesh, where you have very little overlap with. Even your best friends might be on different niches on ticktock. And I think we'll just see that trend continuing with completely generated things, where, where it just generates things based on your taste or and I already find myself having this urge. So because, for example, when I played Dungeons and Dragons, I usually research like what's what's in this world of Dungeons and Dragons, and it's often like what do I want my players to have there? But with GPT I've made these kind of bots that just when I ask like what, what will these characters?
Speaker 4:and know is because it is linked to my character. She's like what can they do in this world? And it gives me these large texts and I'm really like oh, this is so, so much to listen to. I would rather listen to it while doing laundry or stuff like, and then that's basically an automated podcast that I that I feel I really have the urge of of listening to, and I think that that will probably be something that a lot of people will more and more have, just like we find these kind of niches in ticktock that will just find these kind of niches in something that is completely generated for us and we might not have anyone to talk about it.
Speaker 3:Which is a scary thing. Yes, like, a lot of the interactions we have are about like OK, the latest Game of Thrones episode and how bad it was, or whatever. Or like when we were children.
Speaker 1:The shared experiences.
Speaker 3:And if that completely disappears, like? Imagine, as a parent, you're like you put an audiobook app next to your kid and the audiobook app optimizes how long your kid stays silent. That's a very nice thing for a parent, I guess, but like Cindy, that kid will never hear a story that one of his or her friends hears, but it's a trend that's already happening right, the whole ticktock movement and the recommendation engines are pushing towards yeah. Yeah.
Speaker 1:I don't know if I have mixed feelings about this future as well.
Speaker 3:Yeah.
Speaker 1:But I do agree that it's a viable future. I don't know if I have time for hot takes parts.
Speaker 2:I think you tease me a little bit that you, with the idea that you have a hot take for today, you didn't want to tell me what it was.
Speaker 1:So I don't think we could skip on it now. My hot, you did you see it before?
Speaker 2:No.
Speaker 1:My hot take it's my personal hot take and I'll stand behind it is that I have a strong belief that soap in bars is better than liquid soap, and if anyone disagrees with me, you know where you'll find me. Leave a comment.
Speaker 4:And what's your reasoning? Yeah, how did we get?
Speaker 2:to this? How do we get to this statement?
Speaker 1:Well, I mean, it's just better.
Speaker 3:Yeah, but why?
Speaker 1:Well, do you agree that maybe let's start there?
Speaker 4:I think it's that's hygienic right, because it's less. Yeah, because everyone touches the same. But it wears off, it wears off.
Speaker 3:You can say like oh yeah, everyone touches the same bottle, same thing, okay, but afterwards, you wash your hands under the water with the soap, instead of like a soap that could literally be dropped in the mud and still be brown from the mud.
Speaker 1:No, I mean, why is it better? It's better, okay. So First, I feel like it lasts way longer, like if you have a bar of soap it's gonna last longer than if you have liquid soap. I think it's more cost efficient.
Speaker 1:I mean I also think it's more environmentally friendly, cost efficient, for sure it lasts longer, just like lasts longer, and I also think a bar of soap is probably cheaper than a bottle of soap, I guess, probably. I also don't like how sometimes, if you have like a puddle of soap and then it's just like the water hits and then just like you have the whole chunk just kind of slips off your hand, you know, and so I don't know, I feel like when I use liquid soap I still use it, okay, just no, I'm not that against it. You know, I'm open minded, I don't know, it's weird. I feel like it's like I have to put too much and then it's like it doesn't feel like it's really clean. You know, I feel like bar of soap is like way faster, you know, boom Done. The point is. But I still don't. I see where you're coming from, but I have to strongly disagree. I also think you can also travel with bar of soap, no problem, you know, you can take it anywhere.
Speaker 1:But you can also travel with a small liquid, but then you have to either buy a small thing, because if you want, you know. Yeah.
Speaker 4:Yeah.
Speaker 2:Never been in a style.
Speaker 4:Interestingly enough, the last argument that you're fighting over is the first argument that GPT doesn't make what, because the first ones that you just listed are all just made by GPT. Oh, really, yeah, so we're all starting to sound like a GPT. You clearly asked it to this one.
Speaker 1:Yeah, no, because in Brazil also, like people use more. Look at the bars of soap. It's very normal and I've been places in Belgium as one of them and I feel like I received certain, you know, attention friction due to that.
Speaker 2:What put you in situations that people notice that you're using bars of soap versus no?
Speaker 1:It's not like people notice. I don't usually shower in a group setting, let's just say that. But when I mention it, you know it doesn't come up often, but when it comes up people look at me like I'm not from here, which I'm not, but you know.
Speaker 2:This is like a topic like do you sit down and pee or not?
Speaker 1:Oh, don't even get me started on those. We can discuss this another time. But yeah, I don't know. I just want to set the record straight. I'm a bar of soap kind of guy. I'm flexible.
Speaker 4:I kind of feel like this whole segment is just like an excuse for something that happened earlier today or something.
Speaker 2:But people said like is that a bar of soap in your pocket store? But I honestly completely lost our whole place, I always have something to do with data.
Speaker 1:No, I just want to switch things up today.
Speaker 2:You just wanted to make this argument, yeah.
Speaker 1:I feel like I was maybe shy. What makes it you're so passionate about this? I guess it's just I'm just stubborn. Actually, that's the real thing. Okay, you know.
Speaker 2:Yeah, I just want to put here on the record live for everyone to hear. Thanks for sharing this.
Speaker 4:Imagine you're just scrolling over LinkedIn and you put on this live this guy arguing for bars of soap.
Speaker 1:Yeah, but now you know, maybe I should change my LinkedIn title. You know, bar of soap lover or something.
Speaker 2:What is your ideal size Bar?
Speaker 4:Do we have options there? I don't know, I don't mind.
Speaker 1:You have the small hotel ones that you get for free, but then you have, like the huge chunky bars, yeah, but I think the hotel ones usually they're cheap, right, so it's like it really dries up your skin. Not a fan.
Speaker 2:Oh wow, it is also different, of course. Come on, just this guy, okay.
Speaker 4:Let's leave it at this.
Speaker 1:The only thing better than a bar of soap is one of those like 71 shampoos. You know it's like shampoo conditioner. Soap, laundry detergent, you know, remove stains, that's the best.
Speaker 4:But I was a student, seven and one Exactly. I mean, what a stereotypically male way to end the podcast on international women's age.
Speaker 1:Maybe we should change it on a different note. Maybe or maybe not, I don't know. Maybe I should have kept this one for another day, indeed in retrospect.
Speaker 2:Maybe we're.
Speaker 1:That's a learning we take away from today. Yes, some people stumble upon can see the details, so maybe I'll just put it on the screen again. Peter, thanks a lot. Oh yeah, thanks, peter, thanks a lot. You can find out more information about Peter's work on peterai. Are you on X LinkedIn, all these?
Speaker 3:things. Yes, like my name on LinkedIn and my name on X or Twitter as well, without any space or underscourting.
Speaker 1:Thanks a lot for joining. Thanks for the invitation, yes, and also thanks, thomas, nice, to be here. Yes, thank you very much for your invitation. And then you have us again. Your personal website there as well, for you to check your work there. You also linked in Twitter all these things.
Speaker 4:Yes, but sadly my name is less unique than Peter's, so it's Thomas underscore Wint on Twitter. So without last three letters.
Speaker 1:Yeah, are you the master don and all these things? Are you guys, all the blue sky master?
Speaker 4:don, I have a crowns, but are people posting on there? I don't know.
Speaker 3:Is it? I have like a hundred red messages or something.
Speaker 4:Like I kind of wished that it was more like a federated guy, I'm hoping for blue sky to become bigger, yeah, but it feels like the market is so fragmented you're never going to get a big winner out of it.
Speaker 1:now it's extremely different.
Speaker 4:Yeah, very sad Because I really liked the whole research hub on Twitter with all of the researchers being on there. But it feels kind of drained now.
Speaker 2:All right, For now it's a shitter Stays thing.
Speaker 1:Yeah, indeed, that's where the people are. Yes.
Speaker 2:Thanks a lot, guys, thanks a lot.
Speaker 1:Thanks a lot for the listeners. Thanks for the intro.
Speaker 2:Thank you listeners. Yes, you have taste In a way that's meaningful to software people. Hello everyone, I'm Bill Gates. This is what they talk about a lot. That's slightly different. I would recommend a typescript. Yeah, it writes a lot of code for me and usually it's slightly wrong. But I'm reminded it's a rust here, Rust Well, Rustman. Iphone is made by a different company, and so you know, you will not learn rust.