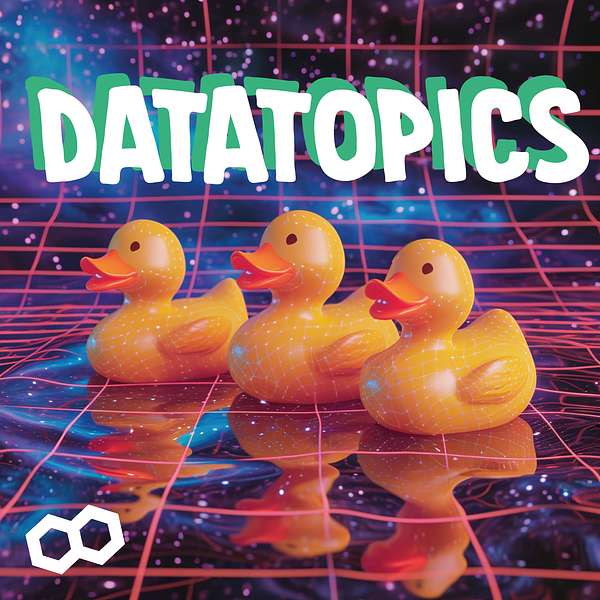
DataTopics Unplugged: All Things Data, AI & Tech
Welcome to the cozy corner of the tech world where ones and zeros mingle with casual chit-chat. Datatopics Unplugged is your go-to spot for relaxed discussions around tech, news, data, and society.
Dive into conversations that should flow as smoothly as your morning coffee (but don't), where industry insights meet laid-back banter. Whether you're a data aficionado or just someone curious about the digital age, pull up a chair, relax, and let's get into the heart of data, unplugged style!
DataTopics Unplugged: All Things Data, AI & Tech
Insights from RootsConf: AI's Socio-Historical Impact & BI Advancements (pt 2/2)
🎙️ Episode Special: Insights from RootsConf – The Data Dialogue Series, Part 2
Welcome to part two of our special RootsConf series, presented by Dataroots. This episode delves into more insightful conversations from the fifth annual RootsConf. Join us as we explore two engaging interviews, each shedding light on key aspects of AI, tech, and data evolution.
- 🤖 Rage Against the Machine with Chiel Mues: Delve into a socio-historical analysis of the AI industry. Chiel Mues takes us on a journey, linking the dots between the industrial revolution and today's tech era. Inspired by the book “Blood in the Machine”, this session will focus on intellectual property and worker’s rights within the AI realm.
- 📈 Modern BI Explored with Mathieu Sencie & Julien Dosogne: Join us as we unravel the intricacies of Modern BI. Mathieu and Julien dissect how these solutions outpace traditional BI by empowering users through self-service capabilities and data democratization. A futuristic look at the expectations and lessons learned from the past in Business Intelligence.
This episode promises to be a treasure trove of insights for anyone passionate about understanding the depths of technology and its societal impact. Whether you're a seasoned data professional or just dipping your toes into the digital waters, these discussions are sure to spark your curiosity and broaden your horizons.
Don't miss out on this compelling continuation of our RootsConf special!
Intro music courtesy of fesliyanstudios.com 🎵
Hello, hello. So, and we're back, and we're back, yes, still, we're still at.
Speaker 2:We still at the Hohen. So it's not what I meant. But in Wendell we still roots gone. We're still at roots gone.
Speaker 1:Do you have an applause there?
Speaker 2:Yeah, Wait, wait, there we go. You have to cheat there a bit. We really, really, really need to put labels on the buttons.
Speaker 1:Yeah, you're probably in the future listening to this.
Speaker 2:So in the future I will go. Yes yes, but for now. So we had an episode released last week. And follow up to that, where we will have discussions, short interviews with around two other presentations that were given at Roots Conf.
Speaker 1:Yes, but maybe if you didn't listen to last week's episode, the Roots Conf is an annual event that we kind of gather to share knowledge about what's the latest in data and everything around it. Really, we have a lot of fun. We have some workshops. So what we had? We had a lot of sessions and we also volunteered some people to come and chat with us about what the session was about. So if you haven't heard last week, feel free to hop on there and just check it out. What was there? Very cool talks, very cool conversations as well. And what do we have for this?
Speaker 2:For the continuation of that, we have two interviews around two presentations. The first one was given by Heel, which focuses on the pace of AI evolution and the challenges in that. The second presentation was by Julien and Mathieu on the modern BI stack and organization and what it will bring us.
Speaker 1:Yeah, very cool talk, very cool conversations. I feel like if I say talks, it sounds like it's a presentation, very, very cool, so let's go and listen. Yeah, let's do it All right. Hello again. Hi, it's me again, and right now we are joined with Heel.
Speaker 2:Welcome Heel Hello, thank you.
Speaker 1:So he was a machine engineer at DataRoot, but I'll let him introduce himself for us.
Speaker 4:You already stole half my intro.
Speaker 1:thanks, Send expectations for the other half. Let's see what you got.
Speaker 4:No, yeah, indeed, We've seen a machine engineer at DataRoot for, let's say, two and a quarter years, something like that, I think. Yeah, I started with an interesting statistics and that's how I got here.
Speaker 1:Okay, cool.
Speaker 4:Yeah, outside of work. What is this? I?
Speaker 2:don't know.
Speaker 4:The table is I hear it what the fuck Electric use?
Speaker 1:Maybe there's like a machine or something.
Speaker 4:But there's construction outside so there's like grains and lorries driving over like metal sheets on the road, and so if one of the heavy ones drive by, like the whole building shakes.
Speaker 2:Come change it so we can restart.
Speaker 1:We want to restart, we can restart, we can restart, we can restart. Okay, hello, hi, hello, we're here now joined with Heel. Welcome, heel, hello. Thank you, I'm a fan of your intro. I had a bit more time to think, so tell me what's the other half of your intro.
Speaker 4:The other half of my intro. Yeah, I guess the stuff that I do outside of work, yeah, one of the first things I usually tell people about myself is that I watch way too much Netflix or Apple and Disney Plus and whatever everything you have all of them Basically, yes, yeah.
Speaker 2:Okay, it's one of my dirty secrets Favorite series at this moment.
Speaker 4:At this moment, gosh, I can't say that. It's too embarrassing.
Speaker 2:Okay, that's great. It's just a bit embarrassing one as well.
Speaker 4:I'm rewatching Ugly Betty on Amazon. I don't know it.
Speaker 1:I don't know either. I don't really care. What are you?
Speaker 4:watching. It's like this show where, let's say, a nerdy, unattractive girl goes to work for a fashion magazine and she's surrounding by all these people interested in high fashion, like beauty, standards and things, and she's like challenging the norms of the company and paving her own way.
Speaker 3:Cool.
Speaker 4:Cool.
Speaker 1:What about you, bart? You said you have an embarrassing one too, and now you've got to put it back? Yeah, I think so too.
Speaker 2:I'm actually watching two series now. One is Deep Space Nine, star Trek, which is okay, I think. The other one is Working Moms, working Moms, working Moms, ah yeah.
Speaker 3:I think for me it's like my Netflix is Is it like reality TV, or is it?
Speaker 4:No no, no, no, it's a sitcom role. It's like sets for vehicles.
Speaker 1:Yeah, I don't know it. Maybe also another question I feel there's many maybe rates, but what's your best streaming platform in your opinion?
Speaker 2:Because, Netflix.
Speaker 1:I feel like it was one of the first ones, so it's kind of like the standard, but I feel like the quality of the things dropped a bit over time.
Speaker 4:Yeah, that's true. Yeah, I know it's a mix of. There's great quality stuff on Netflix as well. There's great quality stuff on Amazon. I guess quality wise, I'm very impressed with, like Apple's offering. They have some really high quality intellectual property like their own shows and movies, like Apple Originals. Yeah, like two years ago two years ago, I think, an Apple movie won Oscar the best movie.
Speaker 1:What do you know about the?
Speaker 4:movie I don't remember the title, but like Severance is like a very big show and I think a lot of people are anticipating the second season of that. All right, so if you haven't watched Severance, go watch Severance.
Speaker 2:Do we know what to do there? I?
Speaker 1:know, I think you will talk now, just kidding. So he was also doing a presentation and he also shared his wisdom earlier today with us Would you mind? Yeah, would you mind giving us the Cliff Notes?
Speaker 4:The Cliff Notes. So, yeah, my talk was called Rage Against the Machine. I think like a social, cultural, social, historic analysis of tech and AI.
Speaker 2:Do you know Rage Against the Machine or are you too young? I?
Speaker 1:don't know. I cannot say I do, but I don't know if it's because I'm too young. Are you seriously?
Speaker 4:Really, you don't know. Rage Against the Machine. Holy, oh yeah, that's embarrassing, I'm sorry. I'm sorry.
Speaker 1:Bart is trying to get back at me, because what? Was the first episode, I was like another one, dj Khal and Bart is like oh yeah, another one by Zidane, no, bart.
Speaker 4:No, bart, that's equally as embarrassing. Okay, I think it's going to come back. Tell us who. Yeah, no, so it's actually based on a book that was published a few months ago. It's called Blood in the Machine. I'm blanking on the name of the author right now. Will, other than the show notes? Yes, sure, I can recommend it as well, the book, but my session was basically following a bit the outline of the book and it's a historic analysis of the Luddite movement and I don't know if any of you are familiar with that.
Speaker 1:Park.
Speaker 2:Topaz is laptoping by name yes, but I wouldn't be able to explain so yeah, a lot of people maybe know what the term Luddite means.
Speaker 4:with someone who is not used to technology or maybe even against technology, it's often like an insult. But actually the term originates from, like, the English industrial revolution, where artisans of the time there were sabotaging machines? No, there were sabotaging machines exactly because, like the industry, titans of the day were introducing quick and dirty automation and people were like seeing their jobs disappear, their livelihoods, their income, and they started sabotaging by literally smashing machines.
Speaker 1:Isn't there like a thing too, maybe a side note, maybe fun fact of the day, or maybe the year, because I don't think we're going to be able to come up with one every day. Sabotage isn't something with like Sabotage, which is like a French thing that people would put in the machines as well, to think there's something about that.
Speaker 4:I don't know, I don't know, the loot, this they used hammers that's like also became a symbol, like these really big hammers, yeah. So I don't know, maybe indeed there could be a part of that as well, but maybe that's a different conflict.
Speaker 1:But I digress.
Speaker 2:I digress. So it's really like a protest against the technical disruption.
Speaker 4:Yeah, consolidation of power by, by resources going to the few instead of the many, yeah, people seeing their jobs disappear. And yeah, a bit drawing the parallels between between modern day as well, like, like company is like Amazon, like Uber, like Tesla, like Airbnb, open AI, even taking all this, this, these resources, this, this, this power in and impacting industries. Like the disruption of Uber in the in the taxi world. It's less in Europe, it was very actual in in the United States. Same with Airbnb, where, where the like hotel industry was like severely disrupted because they they don't have to play by the same rules that those established industries. So, yeah, they do have to play against. And then part that I added as well was was yeah, can we, can we maybe reflect a bit on on, on on generative AI, taking scraping data, what they call data, but actually it's, it's labor, hundreds of hours, thousands of hours of labor from artists, that is that is being taken, restructured a bit and pushed out again and even sold by companies.
Speaker 4:Yeah, so just just to, see what parallels can we, what can we learn from from history? Because, yeah, this disruption, that it was a very bloody time. People, people died. There was like, basically, the army got involved in those days. Yeah, that's not something that we want. I mean, it's probably not going to happen like that.
Speaker 2:But when we talk about any, we do see that the players being able to build these things are the very few exactly in terms of the resources that you need, and that's exactly.
Speaker 1:But also, the same time, if one person builds it, everyone can use it right in a way, like everyone kind of benefits from it. Well, I know I'm being very black and white here.
Speaker 4:If I'm an artist right now, I would be somewhat nervous. Yeah, artist.
Speaker 2:And I think also when you and I the interesting way to look at it, if you equate, like the data that was scraped to labor that was done, I haven't really looked at it, to be honest. Like it's the thing, is it already happened?
Speaker 4:Yeah, open AI is just everything, and now they're selling it to companies in the coming year and they the intellectual property copyright like there are laws are behind the times.
Speaker 2:That's the times. We hope that there will be a legal framework going forward, that there will be some jurisprudence, but, like I think, to a large extent the damage has already been done.
Speaker 4:Yeah, exactly.
Speaker 2:No one is going to train a model from scratch anymore.
Speaker 4:Yeah, that's a bit the modest operandi of like tech companies. Even in the industrial revolution, like machinery, automation was also ahead of its time Laws couldn't couldn't follow up quick enough to keep this rapid innovation and this rapid immobilization in check. And if these frameworks were there, this could have been avoided, like all this bloodshed could have been avoided and maybe you mentioned some lessons.
Speaker 1:Could you give me like a few, maybe top three lessons? You think that stuff that was in the book that we can translate to today.
Speaker 4:Yeah, just general awareness of like the problem. Like, like don't just blindly follow the hype of AI, like think this can displace workers and then it can impact a lot of us. So don't just only look at the good things and, yeah, next to that, be aware indeed, like we are consolidating a lot of power in these big players who have the resources to be able to train and deploy these models. So, yeah, open source is really good and some companies are fighting making stuff open source, but there's some power in open source here as well that we have to take into account or at least promote. Actually, that that's my opinion at least. Open source is really important here as well. Yeah, I think those are the two big ones. I mean, maybe I can come up with more, but no, no, but that's perfect.
Speaker 1:That's perfect indeed. I think it's something that I think with GenAI, the difference. Well, I could say that the arguments you're giving now could be applied at any AI thing, but I think GenAI is so impactful today that it becomes even more like. I think it's like GenAI. It took so much more data and it says so many applications.
Speaker 4:It's transformative as well. It has the power to change business as we know it, and with such change, you have to be careful.
Speaker 2:Yeah, and because it is so transformative, it's also very hard to understand, like how five years from now will look like.
Speaker 4:Yeah, exactly Because of the speed that is moving. Yeah, we try to brainstorm a bit about our own industry, like what does it mean for AI engineers, data engineers, cloud engineers?
Speaker 1:Yeah, it was a bit hard to predict indeed, yeah, and I think the one thing I mentioned earlier as well is the hard part is that there is hype, but it's definitely not just hype, right like I think there is a substantial change.
Speaker 4:There is substantial value in these things, but that doesn't give you a free pass to do what you want with it.
Speaker 1:Yeah, but I think, especially in the beginning, people are like is chat, gpt generating AI a hype or is it something that really is going to bring value? And I think the question in itself already posed an assumption that it is binary when it's not right. I think there was a lot of mixed hype with stuff that will change forever.
Speaker 4:Right, yeah, no we were all convinced it was the second coming of Christ. Artificial general intelligence. And then you ask it to do PySpark, and it's really not that good at PySpark.
Speaker 1:Like a little personal one. Yeah, yeah, no, man, and it still doesn't do it.
Speaker 4:These are my struggles. Yeah, no, it is very impressive, but over time I think we've been able to give it a bit more nuanced. Look as well the hype died a bit down and we got clear about its capabilities, and I think now we can see the true value with that in mind as well. Yeah, be careful how you disrupt our entire industry, especially artists at first.
Speaker 2:So, if I hear you talking like you're a bit of a cautious stance to going forward without any considerations, you also have like this movement which has been in the media for the last month, a lot. I think it's called effective accelerationism. Yes, what is your idea on that? Maybe?
Speaker 1:you can elaborate what's described a bit.
Speaker 4:Yeah, effective accelerationism means that we should do as much as possible to drive technological advancement forward so that the future lives of people can be improved, so that we do the most possible good right now so that people in the future can have the best possible life right now. And I think my main problem with that movement is that it completely ignores all the injustice that is being done today, like we didn't touch upon it. But part of these big models now is also like unseen labor yeah, like workers making sense to label data sets, very back practices, like very boring work, very soul crossing work. Yeah, it's hard to square off the future benefit when you're harming people right now.
Speaker 2:That's difficult when I think about this. I agree with the stance that we need some regulation. Like you see, the EU acts around AI coming up. I think we need some checks and balances when it comes to these things. The difficult thing to me is that on a global scale, we don't have this right. No, we don't In countries at continent levels that there is regulation. But what is the Like if we don't have a global stance on this? Don't you have?
Speaker 4:Yeah, you run the risk of. That's already in the message that's being put out there. Europe is lagging behind, exactly.
Speaker 2:So maybe you will. If you don't have global regulation, you will always have what's called effective accelerationism in other.
Speaker 4:To me that's also a bit defeatist, like it's like, oh, let's give up our morals, our ethics, because, yeah, I mean, people are making decisions for us and yeah, yeah, okay, powerful countries, powerful continents indeed have other priorities. I don't think it's a good quality for us to give up our morals.
Speaker 2:No, no, I fully agree with that, but it's a difficult yeah of course.
Speaker 4:I mean, I'm young, I'm very naive, maybe in this as well, but I fully agree with what Europe is doing and I think we're on the right side of history, at least.
Speaker 2:Also fully agree on that, and I think the difficult thing there is also, like specifically for this, is that it's super hard to have regulation in place that is up to date because it is moving at such an extremely rapid pace. I don't think we've seen that in this Space, you know.
Speaker 1:I think I'm saying, I think moving fast for us, imagine, for people that are not exactly, yes, I hear not xiv, then now they need to catch up with so much time, you know, and I think, honestly, I think that's a whole a podcast episode I would have liked to heal exactly so sure, thank you.
Speaker 4:Maybe give a little bit more notice than I can prepare. I want the true experience, truth You're a rock, you.
Speaker 1:We should definitely meet up again, dive deeper even. I think there's a lot to unpack there, so but thanks, thanks.
Speaker 4:Love you. Thanks for inviting me. It's very fun.
Speaker 1:Thanks, hi, hey again. Hi, hi, still me. Hey, we have here. Yes, we had Matthew. I feel like I'm butchering it, but after a long day, excuse me, julien the good very well pronunciation. Thanks, welcome, both self patterned back you know. So welcome, welcome. It's still roots comf. Now maybe I'll let you guys first introduce yourselves.
Speaker 5:Yes, so I'm Matthew Sanci, so I'm a part of the data strategy unit. I joined data roots one and a half year ago, yeah, and my background is mostly project management, always around data related topics.
Speaker 3:Cool, and I'm Julien. I am joint in the roots Academy in March, so almost one year at the company, also in the data strategy unit and background in Economics and in data as well cool, cool, cool, and you were sharing your wisdom earlier with with all of us.
Speaker 1:Would you like to give a quick recap?
Speaker 5:at the LDR. Yes, so we presented. So the topic was modern bi lesson from the past, expectation for the future. So the idea came that so modern bi is one of the strategic teams that were was identified as one of the focus that we want to To work on in our units and we want to try to see how we can offer modern bi solutions to our customer. And so in that exercise, first we needed to define what modern bi is for the tarot, because there are a lot of different Definitions, but but we want to learn on something that we can all agree, at least within the tarot, of what modern bi is. And then the next phase is how do we market that to to our customer?
Speaker 2:Okay, maybe what is modern?
Speaker 1:Yes, I was like here.
Speaker 5:What is it? I can give you the the nice definition that we came up with, so modern bi. So it's a data driven decision-making culture powered by self-service bi tools that's enabled data democratization and that provides more flexibility To adapt to the needs of the business that are always evolving.
Speaker 1:Okay, so does that? Does that mean Having bi tools that are accessible to everyone and then everyone kind of knows how to go around To get the data that they want? Is that what this means, or dice Part?
Speaker 2:of it. Yes, maybe to better understand it. How does it? How does it compare to not modern bi?
Speaker 3:How does it compare to old-school bi and old-school bi, there was like a Boundary between the data, the IT team and the users of the of the bi itself, so that the more business people, the users, so the consumer, really consumed the, the, the information on the, on the, from the insights and in a modern, in the modern bi, we would like to have those business people participate in the, in the way the insights are created. So, more Working, working closer together with the. Of course you need the more technical people to set up everything, but they have to Work closer together and have more involvement and sooner in the process of creating those insights.
Speaker 1:Okay, is this like a response to siloed teams Kind of thing? Yes, also. No, it's actually something that you kind of see popping up right like you have this Instead of having one central data team, let's say, I mean there's not binary, right, but then you have kind of one data person with one business person with like little pods, right, I think.
Speaker 3:But the data mesh, yeah, yeah, true the ultimate goal of the modern bi or well, data matches, which is much more than business, than the NBA itself. But we also thought about calling it modern analytics instead of modern bi, because modern bi puts it in a box of bi.
Speaker 3:But yeah so much more can be done with that culture. So the image actually is On the things we propose. So the value we can propose to decline data mesh would be the ultimate goal. If you put every lever to the top interesting, then we companies could reach data mesh and.
Speaker 2:If we compared to old-school bi, where you indeed like, typically had like an IT or a bi department that's built everything for you you are, as a user, just had to. We could have specific requirements. This is what I want to see in your dashboard and everything is delivered. You're saying, with modern bi or modern analytics, like this Boundary becomes less strict, like and use it gets more like the self-service part, like they can do more themselves. How do you make sure that Stuff is still Quality-wise? Okay, that there is not a big like. Everybody does the wrong thing and like, because that is the bit the danger of going completely in different direction.
Speaker 5:So so, in the definition of modern bi, so you have those two elements of self service, data democratization and flexibility, but we also define some drivers that allow you to go for more self service more flexibility, more data democratization.
Speaker 5:One of those is governance. So, indeed, the goal is to Shift the responsibility of building, building insights to your business users, but it's not a free-for-all. You don't want everyone doing their own thing. Self service does not mean I'm doing my, I'm building my insights In my corner and I don't see what what else is happening. So you need to To provide that freedom and that flexibility. You need to put some standards, some framework, so that your user have some freedom within that framework and not do what they want. So governance is a very, very strong for the business user.
Speaker 3:It should feel like they can do everything, but the governance puts in place of everything that is required to Maintain cat quality standardization, for everything that's necessary to Do to to make it qualitative.
Speaker 2:So sweet, right tools. You've the right skills that the users but I'll you also have a set of standards on how do we, as an organization, think that we should use this together? Right, yeah.
Speaker 1:No, maybe if you squint your eyes Really hard and you think of ML Ops, it's kind of the same thing, right. Like, at least in my head, is like you want a framework that allows people that are building models to do anything they want, but within the framework, so they still the output is still qualitative and reliable, right, I think it's like a different flavor of it, you know, it's like Interesting yeah, in the slides that we were the slides we used to for research and for preparing Our presentation.
Speaker 3:one slide also talked about the BI up something as something B I ups, but we didn't use it because I think it's sexy you should just take start.
Speaker 1:They saw, because there's little, the LLM ops Get tops. I don't know if there's BI ops. We can point that to you.
Speaker 2:I think you can these days can. You can suffix everything with ops, exactly, it's cool.
Speaker 1:Yeah, like data ops. Yeah, exactly Ops ops. Maybe just like just met ops Miril ups. Oh, that's the money, it's in the money right there. But yeah, sorry, I completely do other cars.
Speaker 2:Mutil ups yeah, I love to check with Maria. We can make that happen.
Speaker 1:It's fine, we'll make it happen, we'll make it happen. But, yes, so it's like, maybe Going back a bit to the why. Right, like you're saying, modern, I think modern is good, but how? A company or organization that is adopted this modern BI ops, maybe. What are the benefits that you see? I guess like what are? Why are you pushing for this change? Because it is a big change, right, there's a governance change, expectations change, then maybe a changing technologies, right, changing training, changing roles and responsibilities. So, if we're pitching all this, how can I convince someone that is in charge quote unquote to go for this?
Speaker 5:I think the starting point is that the business realize the value that lies in data and with all the new emerging technologies, all the new advanced analytics that we see popping up on the market, there is a willingness for business to do much more things than they were doing before with the static reports and the very simple dashboard. And so if you stay in that configuration of having one central team controlling everything, you create a huge bottleneck that prevents you to experiment and try new things. So I think that, for me, is the starting point of the story is that we need to unlock the value of data by putting the capabilities not away from the IT teams, but to offer those capabilities to the business user, and then the whole story of the governance that you need to put in place because you cannot allow business to do anything they want comes into place.
Speaker 3:And also one of the initial goals of business intelligence was to create a competitive advantage towards companies not using it or using it differently, and I think today a lot of companies use the traditional way of business intelligence, of dashboarding reports. So for companies that want to evolve and want to use all the new technologies that they are ready for it, then it's the next competitive advantage will be using more modern business intelligence as a standard.
Speaker 2:Maybe out of my own experience, the bottleneck that Mace mentioned is one that is also. That's one that creates a pain and that pain makes that people also understand like we need to change something. And I think it's also a logical bottleneck in the sense that if you look at 10, 15 years ago, a company has one ERP system and, let's say, 20 dashboards come out or they're being used by a finance department to do monthly reporting. But over time, more and more tools that all generated data, much more touch points with customers generating data, a lot of questions coming to the central data department and at some point you logically have a bottleneck like a central, traditional BI department can't handle that anymore and like that bottleneck, that pain from end users is typically a reason also to say people say like we need to invest in something, like we can't continue like this, because indeed we need to have a competitive edge, we need to understand our data.
Speaker 1:So, and I think on the other side, like the argument against making that decentralization move and I don't know how theoretical it is right but would be that by decentralizing you lose a bit the expertise right, Like if you need to be focused in a group, focused for this, to really be on top of everything that is new and to really dive deeper in all these things. Of course there are ways in practice that you can mitigate that, but I'm not sure if you touched upon this on your presentation as well.
Speaker 5:So we touched upon the need for new capabilities, and that's where the new role of the analytics engineer comes into place. Is that it's someone who has now the technical knowledge to do some engineering stuff, some working on infrastructure, but also on the analytical side, and having domain and industry knowledge. You bring actually those central teams and the business team together with that role that act as a bridge, because they can talk to business, they can understand clearly what are the needs and what they want to unlock with the data, while making sure that what they are building follows the guidance and the standards that are defined by the strategy team that still exist.
Speaker 1:I see, and maybe this analytic engineer is like, would you say it's a pivotal role or not? Maybe not pivotal, but it's a pillar role on the modern BI.
Speaker 5:We see it as such. Yeah, cool yeah.
Speaker 3:If you would have seen our presentation, then the analytics engineer is kind of the Superman of the modern BI.
Speaker 1:Yeah, I think it's pretty sexy job these days, I feel, but I actually think that there is a. I do think it's a game changer as well. I also and maybe this is my personal view I also think that with AutoML tools, actually I think an analytics engineer. So maybe I'll just state explicitly what I think when I say analytics engineer is someone that knows SQL, someone that knows how to query data, someone that can create pipelines, like maybe pipelines. Here, I mean, you have a sequence of queries, a sequence of data transformations that you can stitch together in order, and I think from by being able to do that, you can create reports. You can do quite a lot of stuff.
Speaker 2:And I would add to that that the person is also very knowledgeable on the business domain. Very true, very true.
Speaker 1:They're very close to the data, the business, but they have this and actually, I think, by this stack, I think you can do quite a lot of stuff. And now, with that definition being said, that role is actually very close to doing AI as well, in my opinion. With AutoML tools and whatnot right Like all the tabular data, I do think there is an opportunity for these people to build models, deploy models. Now, in the end of the day, a model prediction is a function that takes some things and outputs some things, just like any other function, not any other UDF. So I actually I haven't seen I have seen a bit of that, to be honest, like very little, but I do think that it's something that's still on the table, like an opportunity still on the table. I think someone is going to find a way to do it nicely in the future as well. I mean, there's actually, arguably there are some already technologies that are investing this like snowflake. You know they are doing the ML stuff. There is red shit for ML, big query, ml.
Speaker 2:I agree. I think the machine learning stuff is going away from the research part, of going coming much closer to the database. We see it like you said. There are some examples there already and I think they will become simple tools to some extent. Of course, we will always have the 20 percent, which will be very complex, but the 80 percent will be coming, I think, in the coming five years very simple to use and where the analytics engineering will probably play a big role.
Speaker 4:Indeed.
Speaker 2:And maybe taking that segue a bit more on the tooling side, did you touch upon in your presentation on what is the tech stack of a modern BI setup?
Speaker 3:Not yet. We did talk about the importance of the data lake house, but in tools we were actually we're doing some. We're doing two initiatives in the data strategy unit on tooling.
Speaker 1:Maybe quickly, before you go a bit further, the lake house, could you elaborate what it is for people that never heard it before?
Speaker 3:So the lake house actually combines the strengths of the warehouse and the data lake. So it allows to be structured and well, well-governed, standardized, like the advantages of the warehouse, but also allows all kinds of data to be used, unstructured, semi-structured, from all kind of sources, which was not possible in the warehouse, which was possible in the data lake. So the lake house actually combines the strengths of both and also plays a critical part, I think, in the modern BI story.
Speaker 4:Yeah.
Speaker 1:And also I mean for me. I hear a lot of the lake house with data bricks stuff and I know that also there's the whole separation of compute and storage that data bricks has me. Snowflake also has this right. That also makes it efficient. So you only pay for the storage you use. You only pay for the compute you use and storage is usually much cheaper than compute. So also financially is an interesting alternative. A lot of the times I'm not sure if this is within the lake house definition per se, but I also know that when you talk about the modern data warehouse kind of set up, a lot of the times it has this deal there. Sorry and I didn't mean to catch you, but I just wanted to make sure we're all together here on the discussion.
Speaker 3:Yeah, no, I'm sure that the data warehouse is seen as quite expensive and everything modern. Well, it's going to use the cloud and so, as you said, it's going to use what it needs. We're going to pay on the go, yeah, so that's also an important part.
Speaker 1:So the modern BI text. Likewise, you said lake house is the central part. You also mentioned cloud.
Speaker 2:What else I think what is also interesting to see is, if we talk about self-service, is that there are a lot of tools popping up, like the established one, like Power BI, that is providing this, but, like you have, like, for example, hex, we use internal.
Speaker 3:That's one of the initiatives where actually Matthew is working on it, comparing tools like hex with tools like Power BI.
Speaker 5:It's to understand what's the difference, in terms of features, of the different tools. What are the most relevant use case in terms of profiles that are using the tools, but also in terms of actually the use case of the analytics that you want to get out of it?
Speaker 2:Cool.
Speaker 5:Maybe one of the other would be more relevant.
Speaker 1:Maybe hex? Is it SQL based or is it, like Python, sql based, both? Yeah, I was going to say because I think it's also. I'm thinking about it kind of now, but you also see a bit this mixed more between SQL and Python, right, like snowflake also is doing this. I think it's also because Python is data language right.
Speaker 2:So it's interesting to see these evolution is cool that you're still exploring these things as well. I mean looking forward to the results.
Speaker 1:That's true, that's true.
Speaker 2:That's very true, Because it's going to be an important part when we talk about self-service BI, I think and not that easy to define like what is best. In a sense. That I also think like takes off, for example, hex as a dashboarding tool, like for someone that does not have a technical background. It is still very complex. For someone that has a technical barrier, background is very simple and, for example, Power BI is much more accessible there. And I think when we talk about becoming more data driven, being more self-serve like, we should not underestimate the skills part that comes with it. It's really it's not a given that everybody in the organization can adopt like a certain level of skills in this, though to be able to do that with whatever tools out there. I think that is an important part of this.
Speaker 1:Maybe a question for you, bart what's your opinion on? So? You mentioned, like Python, hex, sql. Now we have Python for Excel as well, right? So the more user centered things are getting a bit more developer tools, I guess, like the pythons and all these things. On the other hand, too, we see, with generative AI, the developer things may be accessible to users, because now I can just ask chpt to write some Python stuff and we'll test it with all this stuff for us. Do you think that we're getting to a middle ground here? Do you feel like the developer tools for users put a lot of air quotes here? Do you think this is something that will the trend that will continue, or do you think it's just something that, like it will kind of plateau?
Speaker 2:It's a big question, milo. I think a few things to unpack there. Like I think the trend where we see like we expect much more and more technical knowledge from the average business user I'm not sure if it's a, I think it's a logical one Like I think people from their education towards in their career, like they are more exposed to these type of things At the same time, if you're looking at a global, more corporate level, like you cannot expect this from everybody tomorrow, so it's a very gradual thing. I don't think it's a good idea to push everyone like from now on, everybody needs to be proficient in Python. It's a nice objective. I'm not sure if it's going to be, if you're going to reach your goal with what you want to do. So you need to be realistic there as well.
Speaker 2:And I think there, like the things coming up around JetGPT, it's going very quickly and I think maybe within now and five years where we're saying now a business user slash towards other things. Engineer needs no sequel, needs a new, maybe a bit of Python, maybe it's not needed anymore in five years. And I think that is good because in a sense that you write Python it doesn't add anything to the value right. It doesn't make the insights or how you can use the data to actually create value. It doesn't add anything to the value, just something that needs to be done If you can scrap that let's grab it.
Speaker 1:Yeah, I just thought it was a bit. I mean maybe just in the moment, but like we have, we're saying like the BI will be more technical, but then we also see, like the generative AI, we're saying, yeah, are we going to have developer? I think the guy, the one of the CEO, the founder of stability, that's people with this ability AI, I think stability AI he was saying that there will be no developers in five years or there will be no programmers in five years, which also thinks a big statement maybe is trying to maybe fix it.
Speaker 2:But I think it's like, but I think every program programmers or every developers workflow will completely change that I fully agree.
Speaker 1:That I fully agree. But then it's like I see these two things. That to me is like. It seems like they're running towards each other. They're going in opposite directions. So yeah, that's true. I guess time will tell.
Speaker 2:Interesting discussion. Time will tell. See where modern BI will lead us True.
Speaker 1:Any last thoughts? Questions? No, maybe not questions. I'll make that one as the questions. Any other thoughts? Comments? Questions for Bart, maybe.
Speaker 5:No, probably a few blog posts we'd follow, that's cool.
Speaker 1:We need to also do we want to communicate on it. So chat GPT generated. I hope not. I hope that's what you supported. Okay, yes, yes, All right, cool. Thanks a lot. Looking forward to getting those reading those blog posts.
Speaker 3:Yeah, we're working on it.
Speaker 1:All right, thanks, guys. Thanks, thank you. Thank you Transcribed my name is.