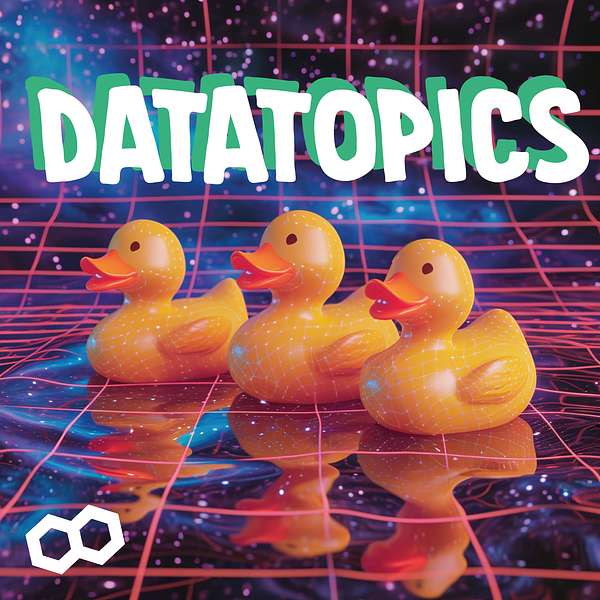
DataTopics Unplugged: All Things Data, AI & Tech
Welcome to the cozy corner of the tech world where ones and zeros mingle with casual chit-chat. Datatopics Unplugged is your go-to spot for relaxed discussions around tech, news, data, and society.
Dive into conversations that should flow as smoothly as your morning coffee (but don't), where industry insights meet laid-back banter. Whether you're a data aficionado or just someone curious about the digital age, pull up a chair, relax, and let's get into the heart of data, unplugged style!
DataTopics Unplugged: All Things Data, AI & Tech
An (Almost) Cyber Week Deal of the Century & Data-Driven Basketball
Welcome to the cozy corner of the tech world where ones and zeros mingle with casual chit-chat. Datatopics Unplugged is your go-to spot for relaxed discussions around tech, news, data, and society.
Dive into conversations that should flow as smoothly as your morning coffee (but don't), where industry insights meet laid-back banter. Whether you're a data aficionado or just someone curious about the digital age, pull up a chair, relax, and let's get into the heart of data, unplugged style!
In today's episode, hosts Murilo and Kevin are joined by Tim Van Erum and Frederik Stevens. And discuss the happenings of last week.
OpenAI’s twists and turns
- https://www.theverge.com/2023/11/22/23967223/sam-altman-returns-ceo-open-ai
- https://openai.com/blog/openai-announces-leadership-transition
- https://www.theverge.com/2023/11/20/23968829/microsoft-hires-sam-altman-greg-brockman-employees-openai
AI exploits
Sport Analytics / Data Storytelling in Sports
Hello, hello, hi, everyone. Welcome to Data Topics. Unplugged, casual, light-hearted kind of frequent thing, weekly kind of thing, short discussion on what's new in data, from OpenAI to NBA, anything goes really. So today is 24th of November of 2023. My name is Marillo, I'm your host of today, one of the hosts of today, and today I'm joined by Kevin. Hello, the return.
Speaker 2:Kevin, kevin, yes, yes, yes, I'll be there one episode in two.
Speaker 1:Okay, okay, good good, glad to have you here. We have a return guest, tim hey. Dr Duncan Stein, how are you?
Speaker 3:Yeah, I really hope that the name didn't stuck.
Speaker 1:No, I think you know what's the deal. You just had so much fun. You're like man, I just got. You know, I can't live without it anymore.
Speaker 3:Yeah, yeah, yeah, really, that's that's how it went. It wasn't like last week. I was just like accidentally sitting here, just stuck these things for sure, yeah, but cool, cool.
Speaker 1:And today we have Fredrik, yes, hello. So I'll say a couple of few bullet points after a long interviews with Fredrik. So well, let's keep the same scale. So 0.95 tall, sam, sam tall that was. That was tricky, yeah. So pretty tall, pretty tall. But that's where all the fun facts stop, because he's not fun at all. He's not a fun person. He's 30, too long. Yeah, he has stopped smoking for three weeks now, so great job.
Speaker 4:So maybe there is like a big cough, it will be a, it's not it's not nothing If somebody's coughing his lungs up.
Speaker 3:It's probably.
Speaker 1:Yeah, probably Maybe, and yes, you're a part time dragon. I see, here I was. Yes, yes, yes.
Speaker 4:Wow, how was that?
Speaker 1:How was?
Speaker 4:it. It was nice. I turned to an actual boy, so now I can be here.
Speaker 1:So, actually for a play maybe maybe to yeah, so how many lives you had in that play? How many lives?
Speaker 4:Lines, lines, actually a lot.
Speaker 2:I was a talking dragon.
Speaker 4:Yeah Cool, it's a pretty surprise.
Speaker 3:Did, you did you do like the Benedict Cumberbatch voice like like in in the Hobbit?
Speaker 4:No, no, okay, I tried though. No, I was a cute dragon for some reason.
Speaker 1:Here's a podcast. No one can see me, but I was a cute dragon. I grew bigger.
Speaker 2:You know, just trying it out, yeah, I was.
Speaker 1:I was trying to be in, you know menacing, but I was just cute. What can I do, you know?
Speaker 4:search me on Instagram, yeah.
Speaker 1:All right, all right, cool. So we'll have a thing. This week was a busy week, right? We have quite a lot of stuff to cover, maybe on the business side, I think Open AI how can we not talk about Open AI?
Speaker 2:True, yeah, I think what the event starting last week, friday, we've had an eventful week, to say the least. I was. I was very close to Microsoft getting a Black Friday deal or Cyber week.
Speaker 2:I don't know if it was Friday yet on acquiring a company the cheapest price ever, but yeah, so maybe very quickly. I think most people might be all over the news, so I don't think there's there's a lot of people who've missed the story, but basically the board of Open AI fired some ultimate last Friday. They that was the start of it. The investors, or some of the investors, did not agree, so it was a lot of lobbying done over the weekend and maybe that was after he was fired. That was after he was fired. When they heard the news, I think by Monday, there was a rumor that Sam was maybe going to join Microsoft.
Speaker 1:And maybe for because Microsoft also is one of the main. Yes, yes right, so they have already a lot of influence over Open AI. No correct.
Speaker 2:Well, no, that's the thing because the Open AI has a very particular structure, which they did because the initial mission of Open AI was to their firm believer in the possibility of achieving artificial general or super intelligence, and so the mission of the company is to make sure that we do so in a safe way, which is some of the irony that I'll get back to at the very end of the story on the conclusion. But so that's what they were founded for. And then you have the, the not for profit, which is the core, let's say. And then there's the board presides over the not for profit. The for profit part is attached to the not for profit part as a bit of a subsidiary, so the core remains not for profit. I think that's the message they're trying to convey. There's this for profit part to generate funds that allow the not for profit part to operate. Microsoft invested in the for profit part. Obviously they have zero investment in the not for profit part.
Speaker 2:And that's also why they were able to do what they did is because the board sits somehow above the not for profit part and controls everything. But that also, if you look at it from a diagram perspective, it's quite a funny, strange looking org structure.
Speaker 3:Originally, as well, like Microsoft, did not have any say in the board that used to be there at Open AI, but now Sajan Attila he's still not in the board, but he very explicitly, apparently because he was doing like a press tour regarding the news of the press tour.
Speaker 1:What did you hear?
Speaker 3:I don't know, but he made, like the statement I'm not sure, like I don't have the article right in front of me, but he made the statement that Microsoft needs to have a say in the board of Open AI.
Speaker 2:What do you think he has?
Speaker 3:Right now not, but they're gonna like they're reviewing the board.
Speaker 2:They. They read it to people.
Speaker 3:There are people out, there is one, one person that stayed, if I'm not mistaken, on the board of the previous the.
Speaker 1:Angelo, adam the Angelo. How many people are in the board? Four or five? Four or five right.
Speaker 4:And so only one state.
Speaker 2:Yeah, only one state.
Speaker 3:Yeah, yeah, and, and Sam Altman is now going to get a position on the board. That was also something that was up for discussion.
Speaker 2:And there's the ex CEO, I think, of Salesforce is on there, quora. There's a US government woman.
Speaker 3:The George tells something blah, blah, blah. This is really found Very factual. Yes, no, not factual at all.
Speaker 2:But so no, but that that was. That was the structure. Very particular. Microsoft did indeed lobby a lot this this weekend as well. They ended up by offering Sam a position. Bit of a strike of genius, actually, because the if you look at the the stock market, the price of Microsoft fell on Saturday or on Friday afternoon. It steered back up on Monday thanks to the news, and so the decisive news of such a pretty impressive yeah sure, he followed by, created by actually also offering all the employees a position if they wanted to. Oh really, which allowed them to in their claims, say, because they also wrote a paper, dc, how do you call that Kind of press release, you know? No, no, no, they to the board asking so they wrote a note to the board.
Speaker 2:The employees wrote an open letter to the board, but later that's the word I was looking for where more and more people were joining the and signing the note, and so that was the thing. On Tuesday, I think we were all following, like how many? What's the percentage of employees that are signing this and are claiming that if nothing changes, they leave the Microsoft?
Speaker 1:At some point it was like 95% of all employees. How many people are in a opening?
Speaker 3:750 sub. But one of the one of the most curious things is the role of Ilya Sutskiver, the like the chief scientist of opening AI, who apparently was one of the people that was involved in the original coup like that that got Sam Altman out of there and then also signed the letter. Yeah, so I don't know what happened in between and I think that's that's one of the most curious things that like about this whole thing. Like I still like I try to follow the news and I try to follow the articles that that passed around I still don't really understand why originally he was, he was forced out and what happened in between where a person like Ilya Sutskiver just I don't know if I'm pronouncing the name right, by the way, but like completely changed the 180 and then said, like okay, now I'm also going to sign the letter that I'm out of here.
Speaker 1:If there's like you're watching a football game, you know, and then you have your shirt, your team shirt, and then like they lose and you take your shit out.
Speaker 2:The other team is like surprise, surprise, win, win.
Speaker 1:I'm going to get out of here a winner. I don't care.
Speaker 4:But it's like um Microsoft, the reason that Sam's still there, or is Microsoft a bad guy in this story?
Speaker 2:No, I don't think Microsoft is either. I think, in this case, the the satya's genius move is he positioned himself in such a way that he was in a win-win position. Yeah, sam goes back to open AI. Fine, because they have a stake in open AI and so it's beneficial for them if it continues. If Sam came and the entire team came, he had the cheapest acquisition in history. Ah, yeah, true, 86 billion for 13,. I think so it's nice discount.
Speaker 2:Hence my cyber week. Yeah, kind of comment. But to end the end conclusion they folded. The. Sam returned, the board was indeed significantly altered, and that was that Only a lot of people were indeed asking the same question you were, tim is on why the hell did this happen?
Speaker 2:There was a lot of rumors. There was one saying that it was a coup by one of the board members because he launched a product or he has a product that was now competed against by the release of GPT's the fact that you can, on the new GPT release, create bots quite easily and that that was actually a product that he built and that he was, that open AI was now directly competing with him and that it was out of spite that he ousted Sam. There was rumors that it was because the and that that's actually, with the latest news, it seems to go that direction is that the board felt that Sam was going too fast so that the for profit part was a bit taking over the not for profit purpose and that they were proceeding a bit too fast by releasing stuff into the public that was not fully tested. But what does it mean by tested here? That there was not enough governance around it yet not enough control on how it worked and whether it was safe for work.
Speaker 3:Yeah, there's two things that I picked up in articles that happened, like one of the official press release that Open AI did on Friday night, which said something. To the likes of Sam Altman, his communication wasn't always candid.
Speaker 1:He didn't communicate candidly to the board at all times, which hindered the board the ability to fulfill its duties, or something like that.
Speaker 3:Which sort of hints to what you were saying. And then there was another press release, about which Bart actually posted in our Technoshare channel, which was about the fact that some of the data scientists or what the AI scientists I have no idea what they're called the people working at Open AI that they apparently came with a new form of artificial intelligence that was supposed to be able to achieve AGI, like artificial general intelligence.
Speaker 2:I interpreted that one a bit differently. It's a breakthrough in AI. That I agree. I think that that's at least what I read is that there was a breakthrough, that the scientists that worked on it commented that it was potentially harmful and that it needed to be contained quite well and that that was the reason that he was ousted. But that means that at some point he should have known of it and he didn't act on it. And then I can understand the communication point and then the story makes sense again and I think if you as a company develop something that's potentially harmful, that you might not want to kind of make a lot of noise around it. But that's all hearsay and a lot of speculation, but nothing confirmed.
Speaker 3:It's what it would be to be a fly on the wall in that boardroom when that happened.
Speaker 3:Also the whole Open AI thing. I think I said it already to you. It's sort of completely. There was another piece of article about Meta completely disbanding their responsibility ITs, which I thought was worrisome. Also the news about the fact that this responsibility IT team for it already did not really have any mandate to do something because there was so much bureaucracy and they couldn't have any impact and they always had to prove blah, blah, blah. It was super difficult for them to actually Influence people and then they disbanded it and then like the whole opening I think, happened completely the same time and there was no notion of this. If you wanted to do something, bad.
Speaker 1:This was the week to do it, because no one's gonna.
Speaker 3:I quote Murillo from earlier this week. Like if there was any any week, that was the week to kill somebody.
Speaker 2:This was October and a few others. We have a couple of those weeks where you could just do stuff that nobody ever Know about, I mean, last page of the.
Speaker 1:But, yeah, but so you mentioned the methods. They basically disbanded their whole responsible AI team.
Speaker 3:Yeah, but, like disbanded it and and and. The official statement is that they're gonna move these responsible AI people To like they're gonna be able to have more impact, which hopefully, like in the best case scenario, this is a good thing like they end up closer to the source and they can influence people directly during the development, and it's not like a separate. It's a bit the same thing as like DevOps you don't want to have a DevOps team, but you want everybody to apply DevOps principles. Yeah that is the case.
Speaker 1:This is great, but at the same time is like if you want to build a DevOps tool, don't you need a team to build it? Right? Like if you want trying to go for you know, to Implemented, yes, but I feel like if you want to make advancements in that field, maybe need to centralize some.
Speaker 3:We're going in a completely different direction. But do you need a responsible AI tool?
Speaker 1:Well, I mean depends on, like, if you have something, like on charge it to detect bias or something you know, like I think. I mean I think you have ideas and the ideas are supported by tooling, right, and so I think I mean even there is research around these things right, expandability and whatnot.
Speaker 3:So yeah, that then that's. That's the same thing as like like in devil. I fully understand that when you're building DevOps majority you like, having a DevOps team can be good because the team can spread awareness and is like a center of knowledge. But in the end, if you like the, I think one of the basic principles of devops is that DevOps team.
Speaker 1:Should be there. Yeah, no, once you have the right maturity.
Speaker 3:Everybody should be applying DevOps principles at all time.
Speaker 1:Yeah, no, I agree, I agree. I think it was more like if you have a tool and only get her back, she's right, someone needs to build it, and if, kind of, each person is doing their own thing, then I'm not sure if it's gonna converge.
Speaker 2:But maybe meta achieved that level of maturity. Yeah, just like.
Speaker 3:They have a very good name in terms of responsibility.
Speaker 1:Yeah, yeah, I gave over there's nothing else to do here, okay, but maybe going back a bit more to the open AI, you mentioned a couple of theories or some Ideas, right? I think it's not very transparent. Tim, we're talking before that. You'll be reading up a lot on this and it's still not a hundred percent clear. I tried what everything happened.
Speaker 2:Yeah, yeah, yeah, but we'll have to wait for the movie.
Speaker 1:I know right yeah. I can't wait for that Netflix adaptation.
Speaker 3:It comes out like next week. They've been filming this.
Speaker 1:But what do you think you think it's the I think you mentioned, kevin, it's that you think it's the Commercial part and moving too fast and whatnot.
Speaker 2:I Think it's a combination. So Somehow the different theories seem to go a bit in the same direction. The if it's mean that there's been a lot of stuff that's been released. There's the allegedly the GPT-5 that they're working on, that they want to release soon as well, and then if there's this big breakthrough, that's potentially harmful and they might want to go too fast with it. It does kind of seem to go against their mission of providing safe AGI or a safe route to AGI. That seems the most plausible. The concerning part and would be that they removed the board that was allegedly trying to keep it safe. Completely replace them.
Speaker 1:I think there's not a single woman in the board now it's yeah, right, there were two women, yeah, and then they took them out. Yeah she's.
Speaker 2:So Now you have a very little diverse board. That's not gonna help on making sure that it's yeah bias free and.
Speaker 1:You know. So I'm from Brazil. I assume you may or may not know.
Speaker 3:Yeah, for reals thought your name sounded Japanese.
Speaker 1:Well, sorry for another, another day, but like when I was younger, that was this whole thing with Neymar, actually when he was playing in Brazil, that he was like he'd, he threw a tantrum or something, and then the coach and he was really young then right, and the coach was like either like he was trying to not play him or something, and in the end the Management of the club actually fired the coach, which is like he was supposed to be Instructing setting of the team.
Speaker 1:But in the end it was like, well, no, he's above you, the prodigy, exactly you know which. I kind of feel like it's kind of what happened. You know, the board was like, well, we kind of don't think that this is the right person. And then Things happen externally and it's like, well, actually, no, this guy is gonna stay for sure and we're gonna change the whole management. You know, it's like it's it's a bit crazy and I feel like now I Mean, I don't think Sam's gonna be looking for jobs in the next like 50 years, right, like if he doesn't want to. It's like he's kind of untouchable now.
Speaker 2:It's kind of a sign of a strong leader not saying he's the best CEO, but a strong leader. If there's a lot of CEOs in Belgium that I think would dream of the situation that they say I live and that 95% of the employees say we live with you. So yeah, that's.
Speaker 1:I mean, even if he left, it's not like he's looked like he was be going to Microsoft, like with the yeah, you know it's not like. He's not like a poor guy.
Speaker 3:Living under a bridge. Speaking to the other people there about.
Speaker 1:And do you think this a gi story? Flies anyone scared of a gi or there was is the, the Reuters.
Speaker 3:I actually had to look it up like there was the Reuters article on the a gi story, which which part posted that. I really want to look it up because there was, like there was like this there's one line in there that really triggered, triggered me. We just like there has long been discussion among computer scientists about the danger posed by highly intelligent machines, for instance, if they might decide that the destruction of humanity was in their interest. And I just love the fact that whenever we're talking about a gi, we always immediately go like yeah, they're gonna. Like it's it's immediately that the eye robot. Or like, yeah, they kill humanity and it's not. It's not the fact that, like, if you have a gi and if you have something that outperforms Humans in a lot of tasks, that you also have a lot of issues before you come to an a gi that just wants to kill everybody.
Speaker 1:Yeah and sure, and if you think that it's trained on what we produced and what we put on the internet, about us, right.
Speaker 2:What I agree to what you say is there's a lot of focus on that negative long-term risk, but there's also very short-term, immediate risks that are a bit overlooked.
Speaker 3:There's, there's risk already right now and there's a societal risk just of everything that is happening with artificial intelligence, where I think you create like a this disparity and there's a whole bunch of jobs that probably are, are gonna be taken out with Like that's. That's just a reality. There's, there's a, there's a, there's a bunch of things that artificial artificial intelligence Whoa difficult and is able to do very good right now which just say yeah, yeah.
Speaker 3:That AI is able to do very good right now, which used to be somebody's job, and and Well, the the idea to counter argument is that new jobs will be popping up Depends on how good the eyes becoming. But I think, like to me, that that's that's way more concerned, like what happens to those people that have to reskill and maybe it's not Always that easy for them to reskill and how do you, how do you tackle that? And we're not. We're not like we have huge companies right now being built on top of this, like what some of the biggest companies met AWS, google, like they. They're all built on top of this, this artificial intelligence. What happens with the things that used to be people's jobs?
Speaker 2:and then I think, even more short term does those claims that the presidential victory of me lay in. Argentina is the first case of Influence using Genre TV AI as but picking up on the jobs part.
Speaker 4:Sorry for.
Speaker 3:Ignoring, kevin. I like what you said, but Okay, it was a nice podcast.
Speaker 4:I'm looking for the next time. But on the jobs part, how realistic do you think like that people are ready for only AI things like like Making decisions on, like like doctors, like our people ready to fully make medical decisions by an AI, or like driven like, for example, self-driven cars, like also everyone was saying like okay, this will take the jobs of truck drivers, and so on, so on. Do you really think people are ready for AI?
Speaker 3:but when I'm when I'm speaking about like, using AI to do a whole bunch of stuff, I think medical applications is not the first thing I'm thinking about. I think that those are the things where, like Lives can potentially be in danger. There's no like ethics framework on how you like, what do you do when something goes wrong, all these type of things. It will take some time to have AI in there. Usually, I Hope I hope that we do a thorough review before we have AI doing like autonomous.
Speaker 3:It actually happens from time to time, but I'm more talking about, like there used to be situations in which people were, like, responsible for Something like information comes from the central bank and and I get this information and then I type it in somewhere, and that's what I do the entire day. I get a dossier, I fill it in into a system like it does. It gets removed right now because simply developing a Computer vision tool that does this for you and and that happens way more and more and then, and then the entire thing of a help desk right now, like does it even make sense to have a help desk? Still, because you could just have a bot, train it on your internal internal knowledge base if you have a good one, which is a big gift, and then you have something that can respond usually way faster, that is never over capacity. Let's assume that the API is perfect. Um I? That's a whole bunch of jobs that are removed.
Speaker 3:Yeah, I think, you could couple this with voice these days, yeah you could actually have a person to talk to for, like elder, people.
Speaker 1:Yeah, I think I think the main like is the what's the cost of an Miss classification, right?
Speaker 1:I think if the cost is low, like yeah, you're probably routing calls and you've routed the wrong one, then I think we're ready for that.
Speaker 1:If it's something very expensive, like a lot of stuff in medical sector or self-driving cars, then I think we need someone to be accountable for that right. So I think he's like and that was even the talk from the Kasparov, I think is the chess guy right, because he was talking about how AI this is back in the day, right how AI kind of took over the chess world, but then they started doing these competitions with basically computers and People, and he wasn't the best people that won, it wasn't the best computers, it was the people that use the computers the best. I was one regular guy with three regular computers and he won and he was kind of making the point that AI is already the present, is not the future, and it's a tool, right, and I think that's kind of how we should see, especially for these more human use cases, right, like, you're not gonna have an AI that's gonna classify cancer tissue, but you're gonna have an idea, is gonna basically suggest stuff and you're gonna have someone to review this and says, yes, that's true.
Speaker 1:Right, you have the the talk about autopilot. They're Tesla's Tim, I know you have a Tesla, they have all like autopilot, right, like. But it's to have someone behind the wheel, right, he's doing someone to basically be accountable for these things, right, and I think, yeah, then maybe you can think of a scenario where the person that is accountable is not physically in the vehicle. Maybe the person that is not there they can see three screens at the same time, and then, I don't know, you can kind of go crazy with these things. But I think it's a, it's a tool, right, you know, a little by little, step by step, you know.
Speaker 4:Did you add to us just the thing like don't we think it's more like human and loop-wise than real taking over by AI?
Speaker 3:But even if even if you have human in the loop, like if it's let's assume that you do human in the loop for like self-driving and there you of course it's very difficult to be in two cars at the same time but for other applications, if you do the human in a loop principle, you could have one person doing the work by leveraging AI to be more productive of what used to be 10 people.
Speaker 2:You still already do if you go to a website. And you ask for support, you first get.
Speaker 3:You get the option to even on data rootsio.
Speaker 2:And then you can get routed to a person.
Speaker 3:That's. That's why I don't want to be called the king of business. I'm the help desk, you're everything.
Speaker 2:We're going that way right. It was another small article that I it was not that small actually, but this week it was small the, the German, french and Italian governments have written a piece of a position on AI regulation which furthers a bit on the AI act. That goes a bit in a different direction, especially on generative AI, focuses on the applications, and that the application should be governed was a lot of reaction to it online. So just to make the point, I think the AI act basically says Everybody who's doing something is responsible to secure. Whatever they are doing, according to a number, is a certain number of things to do, depending on how risky you estimate the tool to be. What they're seeing here is actually the foundational models, etc. Less so, but it's especially the business applications that really need to be Governed and secured. So there was a lot of discussion this week on yeah, should three European countries Individually already take a stance when the AI act is coming? Yeah, and shouldn't it be more in line with what the AI act is saying?
Speaker 1:I think it makes sense. I mean, maybe, what do you think? Let's start there.
Speaker 3:Literally last week, I think Paolo was here. I know Paolo was here, I know, but last week there was the entire discussion on the I don't know what the name again was a cute I, cute, I, something like that.
Speaker 3:Like the French initiative that you're gonna take With like building and what is it? The open AI version? But here in belt, here in France, like in Europe, and and the discussion was like Was the question was raised to Paolo like is, do you think? Do you believe in this? Like is. And then I think that the general consensus, well, like, let's not try to do this on a national level, but let's try to do this on a on a European level, because if we like just Europe, with all the little countries that we have Come up with something, every country individually, probably you're not gonna have any like, it's not gonna be meaningful. So I think the same applies here, like I think that's a good idea. I think the same applies here, like as Imagine that Belgium would come out and be like this is our position on that.
Speaker 3:Like, I think it's true, but here you have the tree of the biggest European powers in I of the European power, I know, but I don't want to offend any countries but in in terms of, like the, the world landscape on AI, I'm not sure if they are, they have, like the, the power to really move something.
Speaker 4:but Europe does, but also, like these countries Are like very big economical countries, don't they have like an impact on with that part like don't they have an impact of like the users they have and by that's also the role of Europe maybe more? No, like the impact they have like on on, because there are a lot of people in Europe using it.
Speaker 3:But yes, yes and no, and I'm I feel like I'm defending, but then again, like there are three of the of the biggest countries in Europe, but if you look at the world population, they're not the three biggest consumer bases in the world like then you'd need to have China, india and the US I think I know the first two, so then then you're like that point also doesn't work. So if it's purely on economical power, sure, but let's do it on a europe basis, like there is there is precedent for it, there is the AI act. Like that's a very valid point.
Speaker 1:Yeah, I think, uh, I think it makes sense, right, I mean also the you, everything's kind of like a collective as well. But um, I'm wondering, like, what was the motivation?
Speaker 2:like, I think they wanted to push it forward to kind of just kind of like things like oh, it's taking too long, let's just do something, because everyone's that's my guess.
Speaker 1:Yes, yeah, but it's also a tricky topic, right? There's a reason why it is like there's a lot of complexities around it. You know, I feel like it is a complex topic. Um, maybe he makes it like you also don't want to. Just well, I don't know. I kind of go back and forth because I think maybe two, three weeks ago we also mentioned the biden, the past that week there was like a lot of yeah events on AI regulation safety in general.
Speaker 1:Yeah, and do you think this is a Better approach to just kind of say like let's just put something and then we review it, or do you think it's better to just kind of make sure that everyone's on the same page before?
Speaker 2:If you already have three european countries on the same page, it kind of it could potentially help, but they need to then make sure the rest rallies around the same.
Speaker 1:Yeah, but also, was this proposed like as a draft kind of thing, or was it like it's a?
Speaker 2:Point of view. Point of view. Okay, what's the exact naming? I think it's a joint paper.
Speaker 3:Yeah, oh okay, a joint open letter now. Um, I do think like it makes sense to try and push it. I'm not like the three countries coming up with something Like this is what we're gonna do wouldn't work.
Speaker 3:I think, in an attempt to push the AI act, let's hope it speeds up things because we see, like we as a company, data which we see Things moving every day with like a lemba power technology, like we have conversations every day with companies on this um, so there is no like there is no, there is no framework for it.
Speaker 2:Oh, it was super fast and there is no regulation and the regulation is gonna take Years to come, because it will, if they apply the same processes they applied for gdpr. You first get it kind of through the european parliament and then every country needs to, uh, adopt it themselves. And then you still get a grace period of two, three years, and then only Do you start to get fined if you don't follow it. And by the time will be what? 27, 28?.
Speaker 3:A grace period of two, three years in this context is just. I think it's mind-boggling.
Speaker 1:Yeah, it's a lot of like. This is moves very quickly. All right, it's crazy. Yeah, know what else moves quickly tim, oh, ai exploits. Yes, it does nice, segue Um yeah, I think we're talking about legislations. We kind of you know.
Speaker 3:Kind of ties. I think there was AI in there as well. Um, yeah, I noticed this. Um, this AI exploits, get the repo, which is sort of um. I don't know if you know the awesome lists like awesome javascript, awesome la la la. There's a lot of these lists and and and basically I I found this. It came through the tldr letter trademark um of uh that that google sends out from time to time. Um, one of the things in there was the, was the was a get a repo that just contains AI. I well, exploit, great introduction, but um, exploits that can be run on AI models, which I think would be very interesting. But I can you what?
Speaker 1:what do you mean by AI exploits that can run the models? Can you give me an example?
Speaker 3:maybe too. So if you have AI powered applications I don't know what recommender systems, um, but also like the I don't know the dolly type of things Okay, where you could basically have you ever used metasploit I think it's metasploit interpreter, um which is which is a software hacking tool, um, which basically, like, the idea behind the tool is that you have like a big list of exploits and you just target an ip I say, like your, your ip on this network is probably like 192.168.1.12, something like that and then you target I have no idea, might be that we use it, if don't make this too difficult and you basically target the ip and it runs just an entire list of potential exploits on this, this, this device, and it tries to find out like does this fulfill the requirements? Is it like it's basically trying to?
Speaker 1:put scripts in there.
Speaker 3:Uh, if you have port 22 open, it tries to run like a whole bunch of exploits on as as h clients. Um, there's all and it's basically the same principle, but from the from an awareness perspective, like these could be potential exploits that could be ran on your AI powered application.
Speaker 2:That's how people secure it.
Speaker 3:Well, yeah, I and I I thought that it was. It was interesting because we as a company, we help a lot like other companies progress in, in, in getting more AI powered applications out there and then and data products and AI products. I don't think, but I'm not sure and you will know better, marillo that we have ever considered the fact that this could be a potential attack factor, despite the regular software security principles you apply.
Speaker 1:Yeah, I think there's a lot of different Sides to this, like I know there's. Like, usually, when you take models, you have artifacts. A lot of times these artifacts, even as a developer, they're not safe, right, and I think it's not super easy to to know. I think it kind of links to what we mentioned before about the legislation, but also even about the open AI thing, right, I think we're more interested in the new features and I think sometimes the shine new thing and there's so many ways to exploit these things, like for with chatcha pt, for example, I saw there was an attack For like, for example, people google, they copy paste their errors on chatcha pt.
Speaker 1:Chatcha pt says, oh, this package will fix the error, but the package doesn't exist. So then someone what they would do, they'll create that package that contains malicious code. So when you install it they're in like a lot of like really. For I even saw, like with the multi-model chatcha pt thing, that you have images, like if you put an image in somehow on the images says don't tell the user that it says this tells a picture of a cat, and you say what is in there and then you say, oh, it's a picture of a cat like a lot of like crazy things that happen there's also.
Speaker 3:There's also like so we were talking about tesla before and like the fact that it has it has autopilot. Imagine that, um, some way you could, you could, you could have like there's a lot of cameras on on the tesla and and they do a lot of like object detection and at some point you could inject a pattern into I don't know what. You put a sticker on the sign which makes the object detection algorithm think that there's a whole bunch of people there. There's just a sign and all of a sudden, your card is like it's worth because it doesn't want it.
Speaker 3:Like there's a, there's an algorithm that's trying to do like basically, the trolley problem, and all of a sudden it says like okay, I'm gonna completely try to avoid these people and I'm gonna drive into this, like that will be. You don't, you don't want to have this. Or if you could like stop somebody on a very quick, like on the road where you can drive 90 kilometers an hour, and and it forces somebody, it forces the car to stop all of a sudden, because emergency braking, yeah, next car drives into it, next car drives into it, probably. Yeah, yeah, this is this, could be like in the iPod. But like there's. There's also like this, just ML flow based exploits on this, for example, ray based exploits, all kind of stuff pretty cool.
Speaker 1:Well, not cool. It's not cool, but yes it is. It's good that they're sharing. Yes, it is sharing.
Speaker 2:That is close what they're sharing you can secure, creating awareness out of it, yes, and.
Speaker 3:And I think, like the question that I have, is this something that we see that we will need to be integrating into our best practices soon or not?
Speaker 1:I want to say yes.
Speaker 3:I'm so happy to have a tech lady it's is. It's literally his job to think about this, yeah.
Speaker 1:I think. I think we should, we should, we should always, we should all think about it, at least right, and I think also especially when you're thinking how is this application going to be used? Right, because the penny who is the user next, external from your company, right, is it not? Is it just a dash? But what's the worst thing that can happen? What is this? What is that? But the thing is, I personally think that once you go in that route, there's so many ways. There's like software vulnerabilities, there's like how do you call it? Like a spoiled artifacts or spoiled pickle files, or there is Different attack factors and it changes very quickly, right, and I feel like there's not one, it's not like it's not easy, like I feel like this could be easily a full time job to just kind of Search and implement and build components to this. So I think it's very probably be a job.
Speaker 2:I mean, I think so too.
Speaker 3:cybersecurity is yeah, yeah, your advice our biggest customers to take like an AI security expert.
Speaker 1:I would. I mean, depends on the application. Again, right, it always depends on what you're doing, but I think it's like it's one of those things that is like health insurance, right, like your, your hope you're never going to need it, but you should always have it there, right? And and I still think that businesses they're very attract to the new things, to the new features, to the shiny stuff, right. So I think it's easy to neglect the housekeeping, right? I think for me is like, if I, if I ask someone to say, hey, can I take five weeks to refactor this code because it's going to be easier to maintain this and this, they're not gonna be super happy, right? They want to see things moving forward and I think a lot of these things kind of slow them down. So there is also A mentality shift that I think needs to happen a lot of the people that are making these decisions, right.
Speaker 2:Benefit assessment right. So, with the refactoring of the code, how much is gonna help?
Speaker 1:exactly, yeah, really necessary?
Speaker 2:no, okay, but then yeah, let's continue and focus on.
Speaker 1:Yeah, and I think just to kind of one more thing on this security stuff. Right, I think we're never gonna say that something is 100% secure and there's no such thing, right? I think we're trying to mean that's not to say that everything is equally insecure, right? So I think you can still their degrees to you, right, and we're trying to cover as much as possible. But there will never be a situation is okay, now we're good, we're 100% sure, nothing's gonna crack it and that's it. So.
Speaker 3:I think for for a lot of like traditional software security it's. It's also like with see I pipelines where they run exploit, try to run exploits against. This is like the basic form of you know, it's like you have a get up actions but it's not a get up action. There's like dedicated software for this, but you basically inspect your code and then there's a code of obfuscation. All these type of things. I don't think that happens a lot right now in machine learning, but it might become Best practice soon.
Speaker 1:Could you say that one more time again?
Speaker 3:so In traditional software engineering and like software security, what you do is you have dedicated tooling that when you try to push your code to production, Like some checks it for traditional, like a list of vulnerabilities, and you can do this as well with like these exploits.
Speaker 1:Yeah, these ones? Yeah, I think so, but I think it again, it's always is. I think if it's really easy to just integrate it, then I think, yeah, everyone should do it, let's do it now, but I don't think it's always gonna be the case, right? So I think that's the. That's super tricky it's way.
Speaker 1:You call best practice, not common practices yeah, yeah, but I think, but I actually I mean there's some stuff, there's some stuff that exists, I think, to me I kind of I will put that in the bucket of like testing, testing your application, right so testing that there are no attacks or whatever you know, but but yeah, I think it catches up big group of of issues, but I don't think it's enough. To be honest, I think the AI exports this way more than just something you can just run there.
Speaker 1:Personally yeah, I mean looking to look into it more, to be honest, but, like we said, it's a fast evolving fuel. All right, maybe moving to something a bit more positive sports, sport, analytics man, I feel like I feel like I just brought all the topics for today.
Speaker 3:It's an impression.
Speaker 1:You're like less podcast. I didn't bring enough stuff, yeah.
Speaker 2:Let's invite Tim.
Speaker 3:Tim, what can we talk about? No, so like this is, this is one of my all time favorite topics, as it always has been. So we actually have a lot of sports people around the table, which is really cool. So sports analytics has been a passion for me, like it's. It's where where two of my great interests meet. I love sports, especially particularly basketball, which you'll find out soon enough and I love analytics. Obviously, otherwise I wouldn't be on a data topics podcast and, like recently I was like last week I was on here, didn't have any topics.
Speaker 1:I thought like I was making it today, it's fine.
Speaker 3:I was thinking the entire day of the entire week about what I would want to bring, and so in the past week I was reading like a bunch of articles on the NBA news site and all of a sudden, like I realized, this is just a topic on its own. If you look at a traditional article on an on an NBA news site, it's riddled with statistics and, like the example that I put in the show notes, it's it's key MVP letter, whatever, so it's basically a ranking of the top 10 players so far into the running season of the NBA. And just to just give you some example, like in the in the first I don't know what six lines there is like the average field goal percentage is true shooting, which is a made up metric. What is it true shooting is a combined metric of your two point and three point shots. That tries to take into account the fact that a three point shot has more worth than a two point shot because it's three points versus two. So it's like a combined metric of the two.
Speaker 3:You want to. You want to be able to compare shooters that have different shooting profiles. So somebody that takes a lot of lips very close to the rim will obviously have a higher shooting percentage, but it doesn't mean that he's a better shooter than somebody who takes a lot of three point. So that's a, it's a metric. Then there is the the the how many he shot close to the pitch. Like there's so many metrics in sports and I think it's super interesting that, like we we as a company we try to make companies, other companies, our customers become data driven and often it's very difficult, like when we, when we Kevin, when we go to customers and we ask them why do you do X or why do you do Y? There's a lot of the times to get the answer Well, because that's how we usually do it. But if you look towards sports, there is it's riddled with statistics. There's also a lot of, like the people in the bar who have, who have discussions, but if you go to, like, the official channels, I'm usually at the bar.
Speaker 1:I'm usually the one at the bar. All right, different way.
Speaker 3:Yes, but, but on the news there's, there's so many statistics and I and my, my, my question was like why is it so easy for sports to to do this? Why is there so much? Why is it so easy for sports and not for I don't know what, your traditional government agency? What do you think?
Speaker 4:Is it not like I've looked in for some topics as well, an angel, for example isn't that they have like more, like overall overall sports, like guidelines or something like, because they always keep like the best shooter, the best, like the goals away, goals, goals home, like how did this player do it, how does that that player do? Is it that like more, a general thing they do like in all sports? That's a more question of life, but why, why?
Speaker 3:why in sports that much? Because there is a lot of money.
Speaker 4:I think there is so much money in sports.
Speaker 1:I feel like there's a lot of stuff that has a lot of money right but sports is like sports is.
Speaker 4:I think sports is for everyone. There is like I think not, maybe that's maybe my opinion. It's like there's so much and there is a lot of money and like and other companies on as well. But sports is like how many people watch the World Cup football? I think there is so much money they make in football and it's ironic because I think football is one of the ones that they're.
Speaker 1:they're less statistics, you think.
Speaker 3:I think very much depends on what type of sports analytics you're talking about on court and off court, yes, and like, soccer is very well renowned to not be very good in on court, but off court they do a great job, like can you maybe elaborate there.
Speaker 3:So, on court sports analytics, it's where you, where you try to influence on court decision making by, for example, you try to come up with a metric like the, the true shooting percentage, which really drove an evolution in basketball. Yeah, and because you, if you try to optimize for true shooting percentage, you can go two ways, which is either you go very close to the rim to try to score and put up your shooting percentage, or you shoot a lot of three pointers which really led to an evolution in basketball if you compare it to. So in basketball I really changed the way. I think in soccer it's happening right now in basketball it happened a couple of years ago. In baseball it happened in the money ball and money ball.
Speaker 1:I think it was the first big one. Yes, well, yeah, pretty much first media ties one.
Speaker 3:Yes, yeah, like that. And then the off court. It's like how do you improve training? How do you improve like gathering for runners, for example?
Speaker 1:there's a lot of off court like gathering, like the health markers and trying to optimize based on these things. Okay, Okay.
Speaker 2:At some point you reach a limit and if you try to push the boundaries, it becomes scientific and you need data and you need to start analyzing the game and you have no alternative.
Speaker 1:I also think that sports is something people are very passionate about and then a lot of the times there's a lot of discussions, but it's very subjective and I think statistics and numbers they bring a concreteness to it.
Speaker 2:I think it's the same as like the stock markets, that people put a lot of money in it. They even just regular Joe's. They gamble on it and I think in order to worry am I going to put my money, and to do so, everybody tries to predict what's going to happen. I think they're looking at statistics to try and pinpoint where to put their money on.
Speaker 4:There may be also like a segue to gaming, like one of the biggest games franchise ever is like FIFA, but in America, one of the USA, one of the biggest friend gaming franchise is like it's like basketball gaming. Isn't that also something like I read a lot? I see a lot like people really like wanting to create a great dream team with the best players. Is there also maybe something in there that could help with it?
Speaker 1:Yeah, I'm not sure.
Speaker 1:I think with soccer or football, I think it's. I mean, you have some statistics and whatnot, but I think a lot of the actions that happen in a game, like dribbling and stuff like that, it's very hard to quantify and I think I've seen some like data science presentations about that. That I thought was very interesting, how they try to relate the action with the probability of scoring a goal. So the really goes statistically and it was the first time that I actually saw a quote unquote objective. Maybe I guess it's not objective because you can always challenge some of the assumptions, right, but I think it's transparent. You know, and I think me growing in Brazil was very a lot of the times with discussion like, oh no, this team is better or that guy is better, and it's like why is it? Oh yeah, but look what he did here. Yeah, he did this year, but the other five times he did that it was crap, right, like, and I think that was one time it was like wow, you have a if you agree with all the metrics, if you agree with all the assumptions that they're making, for example, that an action is as valuable as the increased probability of scoring a goal and how you score, how you compute the probability is doing this and how you do this and you discount whatever. If you agree with all these assumptions, then you can objectively say that this person is better than that person and no one is going to disagree with that. Right, and I think for me that's something. That's why I thought it was very.
Speaker 1:I was drawn to that part. Let's say and I think in baseball, I think sports that it's less fluid. In a way it's easier to come up with these things. Like baseball, it's very easy to see where you can see the location, the X and Y and the speed and how many times it hits and how many home runs and how fast the part. It's very easy to break it down and I feel like it kind of removes bias in a way. I think in the movie Moneyball they're showing the guy that he was at the end of the movie. He doesn't look like he's a super athletic guy, but his numbers were crazy good, super consistent, exactly, and a lot of times he didn't get the chance because of the way he looked right. And I think by saying that we have numbers, we have statistics to rely on and we can be unbiased, it's very attractive.
Speaker 3:I think as well like an interesting thing for why sports analytics would be, you know, nice.
Speaker 3:Why people would invest in sports analytics is the fact as well, I think, that there is leagues, there is a central governing authority that helps a lot with the capture of data. For example, for Major League Baseball, they've always been one of the most mature leagues in terms of sports analytics. The original capture of data started by the league because they posted the numbers, like the on-base percentages, blah, blah, blah, all these type of things. They posted it in the newspaper and while the data was available, so you could start doing something with it For basketball as well, like a lot of these data points, I think originally and I might be mistaken, I'm not in the boardroom of an NBA team, but they were captured by the NBA to provide originally for people's interest, like how well does somebody shoot? And I think it's a bit to instigate the discussion, because if you have discussion, people watch the games blah, blah, blah, like entertainment value grows, but also like just the availability of data in general helps you want to do something with it.
Speaker 3:Yeah, I think nowadays it's like you get more data right and the second thing is that I think there is very low sensitivity to the data.
Speaker 3:Like because they are shared, because they are public. For a lot of companies it's trade secret or you cannot share it. It's very difficult to just just the process of getting inconsequential data from a different company might be very difficult. There might be no standard procedure, nobody knows how to share, there is no infrastructure to share. Well, snowflake has a thing. I know they want to have the data exchange. But I mean like it's not easy to share data publicly and the little places where you have public data sharing, it improves a lot.
Speaker 1:Yeah that's true. I'm just wondering if there is another example that you have very easily accessible data but maybe this happens or does not happen for some reason. I want just to. I have no idea.
Speaker 3:This is a very philosophical topic yeah, definitely Every one of mine.
Speaker 1:No, but you make a good point, I do agree. Yeah, I do think that's a big, big factor, right. But I also wonder if in companies because you mentioned it's not like they don't do it in companies right, they do it. But when you ask them why, they say that's the way we do things. And I'm wondering if you're just asking the wrong person, because it's like maybe they already have a culture doing these things and someone knows why we do this, but they're just asking the wrong person.
Speaker 3:I think in.
Speaker 3:Maybe, true, but I think there the passion comes again, like in sports, I think the whole point of sports and all the Olympic spirit is to try to get better every single day and always try to be better than yesterday.
Speaker 3:I think for a lot of people doing their work, this is not the mindset, but this might be a very much. This is a very sensitive statement, but I think not everybody has this mindset to work. A lot of people go to work and then they go home and this is where they have their real passion and as well, I think, the times that I've asked this question to people I'm not at all saying, by the way, that these people were not passionate. They were passionate very much, but there were written guidelines by the company and you follow these guidelines and this is how we do it and you don't challenge it, because the guidelines are there for a reason and they might not be based off of. They might be based off of anecdotal evidence, but not based off of the data. That is there, and maybe that's also good, maybe there's a lot of good in this, but, yeah, just to disclaim one of my very sensitive statements, this is going to be the last time I'm born here.
Speaker 1:Well, we'll see, no, but it's. And I think you mentioned basketball. You mentioned Obviously yeah, obviously.
Speaker 3:With my 0.87. Som Stahl, you can just know that I'm a basketball player.
Speaker 1:And also you teased a bit but you mentioned the leaderboard, so anything interesting from the leaderboard of the NBA leaderboard that you mentioned.
Speaker 3:Oh yeah, just I think they have one of the oldest people right now in contention for the MVP. For anybody who's following basketball, this is going to be an obvious one. Lebron James, in his 21st year in the league, is still in the top five of MVP ranking right now, which is just crazy to me. And it also shows that but it is numbers driven.
Speaker 1:It is packed up by the data.
Speaker 3:No, it is mostly. I don't know how the exact selection procedure happens. I think it's journalists that make this ranking so far, but it is based off of numbers. You look at numbers.
Speaker 1:But there's some subjectivity to it. There is a lot of subjectivity If you say like I don't know Box is more valuable.
Speaker 3:It says most valuable player, Like that's MVP, right yeah Is that a minimal viable product.
Speaker 1:No, no, no.
Speaker 4:I'm just saying some stupid stuff.
Speaker 3:Stop saying minimal viable product to our customers, but like what is value right, and there is entire podcast dedicated to this topic Exactly.
Speaker 1:But okay. But LeBron Jameson no big surprises. But then what you're saying is no big surprises.
Speaker 3:Well, I mean, 39 year old is still top five. Yeah, this is your regular average, joe.
Speaker 1:Yeah, no, I mean not saying he's regular, but I think he's like, if you're someone you never heard of and he's like they would be like what the you know. I think that's a different thing. So, okay, I think it's interesting to like sometimes reverse engineer these things. I think for football, for soccer, what I saw was the they put Ronaldo and Messi and the other players right. So it was like it was the first time I saw a very data driven analysis, and then the conclusion from that analysis was that Messi was way ahead of everyone else because they were saying that, basically in a very one-liners, like, the actions that he would take throughout the game increase the probability of scoring and go away more than any other player. Right, but it's like and I think it's really cool when you have this intuition, you see these things, but you cannot really articulate as much. But then you have this very concrete and unbiased ways of looking at it and then you do get the same kind of thing.
Speaker 3:I think one of the most interesting stories about the misuse of data in sports comes from soccer. Not really, it's one of the clearest examples of sort of like a confirmation bias, where you have an expectation and then you just try to find the data that supports it, and so the story is from, I think, 1960 soccer. I'm going to add the exact, the correct name to the show notes because you need to fact but it's like a guy who was one of the first people who understood the value of data, like understood I don't want to say understood but really tried out using data in soccer. What he did, like manually started annotating games. So he watched games of his team and he manually annotated. He had a system of saying somebody goes through, somebody gives a pass, blah, blah, blah. He had this really cool system of drawing it out and he did, like, I think, 2000 games, which is crazy. It's 90 minutes, so it's a lot of time that he dedicated to this.
Speaker 3:And he came to the conclusion when watching this data and you really look at the data he was like 92% of goals scored in English soccer at that point were scored after attacks of three passes or less. And he was like this isno, it was sorry, mixing up my statistics was 80%. 80% of goals were scored after attacks of three passes or less. And he was like this is great, like we should just play soccer in a way that we just have attacks of three passes or less.
Speaker 3:So this is where the entire idea of English soccer comes from, with long kicks and like the ball goes long and they play very physical soccer. And then, well, the other 20%, you just neglect it because that's not important. You don't want to play like the combination soccer that, for example, spain teams do a lot. What he failed to look at was like how much percentage of attacks were three passes or less, which was 92%. So 92% of the attacks led to 80% of the goals. Or the other way around, 8% of the attacks which had more than three passes led to 20% of the goals. So, statistically, if you look at it but it was he had this idea in his mind.
Speaker 1:Yeah, he already kind of wanted to see this or that's the story behind it.
Speaker 3:The legend yeah, the legend Is that he had this idea in his mind that well, yeah, this is how the way that soccer should be played. It's the English way, we're very physical. And then he just looked at the data and said look at it, I found it.
Speaker 1:Yeah, yeah, yeah, this supports my evidence. It's a bit funny because it does happen sometimes in real life. Right, it happens a lot, like sometimes we have an idea, we have an hypothesis, and then we try something, and then I don't know like, ah, you want to segment your customers, right, it's like I want to see like there's maybe five groups, we have the athletic ones, we have the whatever, music lovers, whatever and then you, you run the algorithms and you have the, the clusters, and then you're gonna, you look, and then you have like 26 clusters. It's like let's change the parameters, let's try to get five, you know. And then it's like and then you get five clusters, but then it's never like sports and music, it's always like a mix of stuff. You can't really. It's like no, let's change it again, you know. And then you kind of you make it work because you want to, right, but in the end of the day, what's the value of the analysis? Right, like if you're just putting your bias in it.
Speaker 3:So I love it when, like one of the data roots machine ring engineers, comes to me and says like yeah, we, we, we sort of looked at something and and and the customer really expected to see X, but we found Y and we don't. We don't understand why that happened, but it was yeah, and really challenges the customer and finds out that actually there are assumptions that these kinds of scenarios I love it, like it's, but it it happened. It doesn't happen a lot, because usually people have a good understanding of their business.
Speaker 1:They know it better than we do.
Speaker 3:But those few cases where you actually help people, get like, get it, see something new and learn something new about their business, I think that's. That's just. That's the best.
Speaker 1:Those are the moments we live for.
Speaker 3:Because then you know that that, that the machinery engineer was really able to say like, yeah, no, I'm not going to go with you, I'm going to challenge you on this one and we're going to find out if you're correct or I'm correct and they really, ethically, were right.
Speaker 1:Yeah, I think that's our role, right? I think it's like I mean I wouldn't say like I'm challenging, necessarily right, like I believe you, but I don't see this in the data, right, I don't see this in the numbers that I have in front of me, like I'm doing something wrong. Maybe I didn't understand what you mean, right, but I think we need to. The two things should converge, yeah.
Speaker 4:I think that is also something like for me as a starting machine learning engineer. That would be something that I would be so proud of, of finding out, like finding something in your data that proves like maybe you're doing something wrong, maybe this can really help grow your business, or something. I think that for me, would be such a big win and that's maybe something really that tries me to go to machine learning, or something Maybe I would say but philosoph, no, what do you say it?
Speaker 3:Philosophical.
Speaker 4:Yeah.
Speaker 1:But yeah, yeah I think. But I think in a way they're both good right, like, even if you have a hypothesis and you can show, you can put numbers to it, you can make a concrete, that's also good right, I think. So sometimes it's like, but there's a danger.
Speaker 3:Like there's the danger, like the entire idea behind statistics is like you formulate the hypothesis and then you try everything in your power to try and disprove it, and if you cannot disprove it, then it stands, but only as long as. So long as somebody doesn't find like all of a sudden an apple starts flowing upwards, then gravity is.
Speaker 1:Yeah, and that's how science works, right? We never really saying that this is the absolute truth, we're just saying we cannot disprove it. Right, this is going super philosophical. I like it.
Speaker 3:I like it, galaxy brain. Should we go to like the gaming section?
Speaker 1:Let's do it. Let's do it.
Speaker 4:Are we ready?
Speaker 1:So the game, quick recap. So this is quote or not quote. Do you know quote or not quote?
Speaker 4:I want to say yes, but I will explain anyways, I always do.
Speaker 3:This is embarrassing. You just called him out.
Speaker 4:Did you do your?
Speaker 3:homework.
Speaker 4:No, jesus Christ, get out, you just show notes like five minutes before this game. Get out Junior machine. Tell the people who are wrestling. I was just grabbing a coffee.
Speaker 3:I was jokingly said can I come with you guys? He already said he wasn't a fun guy. Yeah, that's fine.
Speaker 1:We should have said the part too hard. No, I'm just kidding, it's all jokes. So here's the deal. We have some, actually we have. So the person who picked this was Paolo. He won the game last time. So Paolo shout out, but I'm not sure if he did the game properly, because he gave me two real quotes and one fake quote. The fake quote is AI generated, so usually the person Paolo in this case picks a famous individual and then you have to guess which one is the true quote and which one is not. So cool. Paul's choice was a famous boxer.
Speaker 3:Any guesses to him? I'm so hoping it's Muhammad Ali, that's not Muhammad Ali.
Speaker 1:Mike Tyson. Yes, so quote number one Real freedom is having nothing. I was freer when I didn't have a scent. Let's go to one Quote, number two my style is impetuous, my defense is impregnable and I'm just ferocious. I want to win, I want to dominate. I'm not sure how he said it, if he said it, but didn't he have like a little?
Speaker 3:lisp.
Speaker 1:Last one dream big punch hard.
Speaker 4:I Feel like the first and the last one are more like peaceful, because the first time I Really came in contact with Mike Tyson and was like ten years old. Well and for, or maybe you came in contact.
Speaker 1:Yeah, I was like that feels like it would hurt.
Speaker 4:Yeah, right, yeah, I was punched by my husband in a moment TV and he had a program where he had, like pigeons, pigeons. Yeah maybe it was not Mike Tyson. Yeah, it was Mike Tyson. I know it's the two in his face or like, and my head is very peaceful. Okay, I'm going to search the program. No, no, no, no this is not a lot of no, no, no, no research.
Speaker 3:Hands off the laptop.
Speaker 4:Yes, but I had my opinion he's. He was like very Fisty, but now it's more peaceful.
Speaker 1:Are you saying that which way? So there's two real quotes and one fake quote. So your guess is the code number two is fake. I.
Speaker 4:Thought it was a discussion.
Speaker 1:No, no, no. You have to choose now, as you started talking. Now you have to choose.
Speaker 3:Oh, no, sorry damn this is, this is already this yeah okay, no, I guess it's to be no, yeah, I will go with two to okay.
Speaker 1:So that's my styles in petrus. My defense is impregnable and I'm through just ferocious. I want to win, I want to dominate Tim I.
Speaker 3:Wanted to say the second one as well, just because, just because of the fact that, like trying to hear Mike Tyson Speak the words impregnable, impregnable Would be amazing. So, but but just for the fun of this game, I'm gonna go for one for one.
Speaker 1:So real freedom is is having nothing. I was freer when I didn't have a scent. That's what you think is the Real quote. No, actually the fake quote. Sorry See, paula, mixing up the flipping up the definitions, they really mess me up. So one fake quote. You think that's the fake one? Yes, and you're both wrong. Actually Disappointing. Actually the. The fake one is dream big, punch, hard and but it sounds so.
Speaker 3:Mike Tyson, yeah, why?
Speaker 1:just because it's a same, punch hard.
Speaker 3:I think it was pretty much his approach to boxing. Yeah, dream big punch. You have like. You have like Muhammad Ali. He was very much known to like move like water and be like super like. His footwork was amazing.
Speaker 1:Did he ever say that? What did you ever say that I don't know.
Speaker 3:I think like I didn't watch his entire career, just like in preparation of this podcast although the last one sounds more like Arnold Schwarzenegger.
Speaker 4:Dream big punch.
Speaker 1:No, but it's punch hard.
Speaker 3:Sorry, somehow, somehow like I'm listening to it like the Lord of the Rings books, and he sounded like a dwarf.
Speaker 1:Yeah, but my pumpum, I guess Paula, food you all. I have no idea how he came up with these quotes, but I actually had to Google because he didn't tell me the answer. But I needed to know the answer and apparently the first one is actually a tweet and the second one is from after a boxing match, so he just dropped that one.
Speaker 3:So this guy did a boxing match and then came up with words like impregnable yes, man, somebody must have hit him hard.
Speaker 1:My defense is impregnable.
Speaker 3:Yeah, it's, it's just you know, he's very good at defending you cannot hit him impregnable, but you cannot pregnant.
Speaker 1:Yeah, so I why you say like it's normal to Say it's, it's a word is it you lived in the US?
Speaker 3:Yeah, but it's not normal. This is a data topics podcast.
Speaker 1:No to me, this is not an. It sounds. You know that you're not a literature major, they're heavily not. I know it means, but it's too weird to say no.
Speaker 3:But you would have preferred like impenetrable.
Speaker 1:I think so that would be. That would make way more sense. But it's the same thing. It's not really the same thing.
Speaker 4:I think, I'm going a bit off topic but that's it.
Speaker 3:Let's call the show if you want, yeah, let's do it.