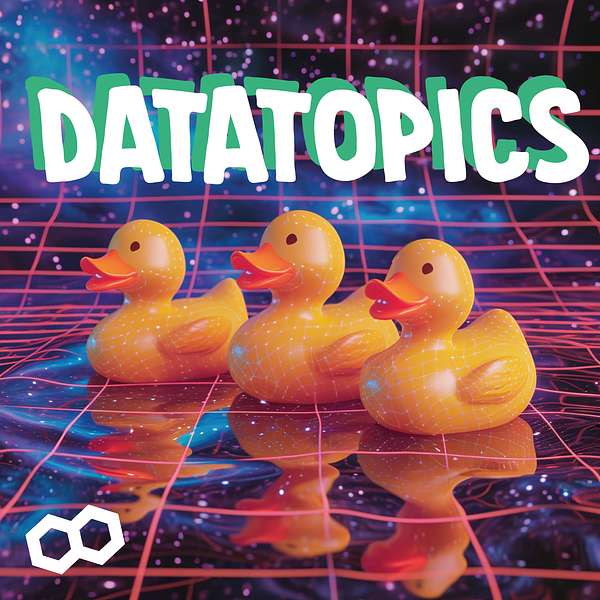
DataTopics Unplugged: All Things Data, AI & Tech
Welcome to the cozy corner of the tech world where ones and zeros mingle with casual chit-chat. Datatopics Unplugged is your go-to spot for relaxed discussions around tech, news, data, and society.
Dive into conversations that should flow as smoothly as your morning coffee (but don't), where industry insights meet laid-back banter. Whether you're a data aficionado or just someone curious about the digital age, pull up a chair, relax, and let's get into the heart of data, unplugged style!
DataTopics Unplugged: All Things Data, AI & Tech
DataTopics: Data Roles & MistralAI
Welcome to the cozy corner of the tech world where ones and zeros mingle with casual chit-chat. Datatopics Unplugged is your go-to spot for relaxed discussions around tech, news, data, and society.
Dive into conversations that should flow as smoothly as your morning coffee (but don’t), where industry insights meet laid-back banter. Whether you’re a data aficionado or just someone curious about the digital age, pull up a chair, relax, and let’s get into the heart of data, unplugged style!
In this episode, we’re joined by Maryam, an Analytics Engineer with a passion for challenges and a knack for curiosity. From sewing to yoga, Maryam brings a unique perspective to our tech-centric discussions.
- Analytics Engineer Insights: Maryam discusses her role, the rise of Analytics Engineers, and their essential tools. Read more about Analytics Engineering.
- The Emerging Role of AI Translator: Exploring the link between Analytics Engineers and AI Translators, and the skills required in these evolving fields. Learn about AI Translator.
- Mistral AI’s New Developments: Analyzing Mistral AI’s latest model and its implications for the industry. Discover Mistral AI’s update.
- ChatGPT – A Double-Edged Sword: Discussing the impacts of ChatGPT on the AI landscape and the pace of innovation. Reflect on ChatGPT’s impact.
- ChatGPT & Job Applications: A fresh take on how ChatGPT is influencing job applications and hiring processes.
- Engineering Management Insights: Exploring whether becoming an Engineering Manager is a path worth considering.
Intro music courtesy of fesliyanstudios.com.
We streamed live!
We are live Hello.
Speaker 2:I mean hello, hello, welcome to the topics. Unplugged, casual, light-hearted, kind of weekly Well, I guess we'll try weekly Weekly tentative short discussion on what's new in data, from analytics engineering to AI translation, to LLA models, to a lot of stuff basically, but anything goes. Today we're also on YouTube first-time live streaming actually. So if you cannot hear if you are on YouTube, I know we had a few people waiting If you cannot hear us, please let us know in the comments. But yeah, let's see, let's see what gives us and also if you see us as well. You see, we have some Christmas theme decorations New Year's, I guess, for Bart. I think he's a bit ahead, but we'll get there.
Speaker 2:Today is the 22nd of December. My name is Marillo. I'm your host for today. I'm joined with my partner in crime, bart Hi. I'm joined with my other partner in crime that sometimes doesn't show up, but still I love him, hi, and I'm joined by Mariam. So I'll say a few words and then I'll give you the floor to you. My name is working for three years as analytics engineer. She likes to read, she likes to take up a good challenge. She's always curious. She has a few hobbies, like so enjoying traveling and yoga. She enjoys going to restaurants, talking to friends and having a drink, even though she refused today's drink. So cheers, thanks. So maybe, mariam, would you like to share a few words.
Speaker 4:So it's my first time at the data topics. I'm very excited, also a bit stressed, because it's also the first time that we're live streaming. So if there's something to mention, keep the comments nice. I would say, yeah, I'm working with Kevin, so that's also here to see Kevin. It gives me a bit of ease, oh okay, nothing to worry about.
Speaker 2:Yeah, I think it's funny because, like, we're live streaming, but before we weren't live streaming, but we never edited it either. It's true.
Speaker 1:It gives a different feeling, it gives a different feeling.
Speaker 2:I know it feels like you're in a simulation airplane and now you're really flying. It's kind of like it's kind of the same thing, but not really. But I digress, I digress, I digress.
Speaker 1:Let's try not to mess up too much. Let's try not to get canceled. Let's take that as a threshold. That's a big step.
Speaker 2:New Year's resolution. It's like 2024. You just don't want to be canceled, right? So, mariam, you mentioned you are analytics engineer. Yeah, maybe very curious term Like what is an analytics engineer?
Speaker 4:So maybe starting with my. So I'm working for three years, but I won't say that I started out as analytics engineer. I started out as a data analyst who could work with Python, let's say, or who knew what SQL was, but it's not that. I initially said, okay, you know what, that's my goal or my role to play. So what is an analytics engineer? But I grew eventually into that role and let me explain what I would do in my job. So what I'm doing at the moment is so I'm working together with the business team, who are having a bit of a data problem, at least, to say, over flooded by CSVs and stuff. So this is like a typical project that we step into and I think our goal within the team is to, or within that team is to, make it a bit more efficient. So make them a bit more data literate, at least to say and to say okay, csvs, and Excel is not a database. That's a start, yeah.
Speaker 2:Yeah, I know it's some people may, but yeah, I know Excel sheets and everything is everywhere. Maybe you mentioned also data analyst.
Speaker 4:Yeah.
Speaker 2:So people that are not familiar with the term, how would you describe a data analyst and analytics engineer and maybe contrast it to? Maybe?
Speaker 4:Yeah, so say, a data analyst would be, for instance, someone who is mainly focusing on the business and saying, okay, this is the insights that I want to get to from my data.
Speaker 4:In that case I wouldn't say that data analysts would be okay, there's a familiar on how to use the data and everything, but it's not that they have all the up to date knowledge on data modeling, all the best practices that are being produced or put in production by data engineers.
Speaker 4:So their goal as a data analyst would be okay, how can I get my insights from data the most easiest possible way? While the analytics engineer, their goal is to provide those visualizations, those insights as well, but more on a way that they also respect the things that data engineers are putting in place, for instance, the best practices, the documentation or the versioning on get and stuff. So it's like a bit managing what data engineers did, keep maintaining it throughout the data lifecycle and still trying to provide an analytics ready data that data analysts could pick up and immediately start with their visualizations, because that's their goal and this way they won't have to be worrying about. Okay, am I using the right data or am I doing everything that I should do when I'm using data, because I think analytics engineer would take care of that.
Speaker 2:Yeah, maybe when I think of analytics engineering, it's hard for me not to think of DBT engineer. Is it the same thing? Is it the same thing today? Because DBT monopolizes the space kind of Well, they popularize the term. They popularize the term. Yeah, that's true.
Speaker 4:I'm happy that you said popularize, because it's not the first time that they use the analytics engineer term. Apparently, there was an article in 2019. I don't know the author, but he mentioned the term analytics engineer. So although everyone it's nice to have some of the brains or colleagues. He sent me the article. It's like we always reference both.
Speaker 1:We link it to DBT yeah.
Speaker 4:But apparently that they and they popularized it but didn't create it. So that's nice.
Speaker 3:Because I think it does make a lot of sense. It's kind of the natural evolution. If first there was chaos and then you started to have central data teams who were bringing a lot of data together to try and provide business with reports, and then they were still kind of pushing out those reports, so they were doing everything, and then you saw kind of a movement towards more self-service. But the self-service flavor we got then was basically you could slice and dice an existing report and you could do a bit more with it, but business was still limited to kind of slicing and dicing and existing report. And then the next evolution was how can we decentralize even more? Because business wanted more and wanted faster.
Speaker 3:And as a central data team you have the challenge.
Speaker 3:You have marketing asking you for something and finance asking you for something, and they're all pulling your sleeve and who do you prioritize?
Speaker 3:So giving them the reins allows them to get there faster and so that you can each can kind of every unit can prioritize for themselves. But then the risk arises if they can do more than just slicing and dicing a report or even building a report, how do you make sure that if they start modeling data or if they start to kind of maybe even pull in data into that environment, that they do so in a way that does not pollute your entire environment. And I think that that's where kind of this evolution came, from data analysts to analytics engineer over the past few years, which makes a lot of sense, and indeed it was popularized by dbt, because that modeling part is something that that that they support and that they support very well that business could do now, but according to kind of the best practices that the data engineering teams have put in place over over the years. I don't know if that's the way you see it as well.
Speaker 4:Yeah, I think David there really helped. So all the features that they provide. It really supports what analytics engineer is trying to bring out to the data. So they provide documentation, which is a very good. Also the data lineage parts. It is very handful and, I think, also easy to read, easy to explain for analytics and engineering to if they want to explain, okay, how you see your data influence. They have, like, all the features that were required to have. Also like to have prediction environment that you can easily say, okay, I'm deploying it now to prediction that it's easy to track. So that's why David made it like very popular, so it's nice.
Speaker 2:Yeah, I think well again, maybe I am still with the dbt engineer as the analytics engineer.
Speaker 2:So I'm just going to go with that. If my assumptions are wrong, please correct me. But I think what really attracts me like I think it's the really where the money is is that we had, like the analysts that were writing SQL queries. You know there's a lot of data SQL can handle really well. Now, dbt, you can kind of like stitch queries together and now you have pipelines, and now you have all these things.
Speaker 2:It's like I've seen data engineering projects that were basically just a scheduler, like Airflow or something, but in the end we're just using BigQuery, right, because you don't have to worry about the computer skills and everything. And it feel like I mean at the time it was like, okay, this is normal. But looking back now is like, okay, you're going to have Airflow, which is like another beast on its own, and now you need to manage this just to run queries in order, right. Like you can tell someone, hey, run this, then run this, then run this, and dbt kind of did that right. Like it's it kind of stitched everything together and by doing that there's so much you can do, there's so much value you can bring. Is one person, yeah, right.
Speaker 3:That's the whole point. But then that's where I channel. I do want to challenge your statement of saying it's a dbt engineer, because if today you need to have one person in a business team that can kind of help that business team get insights, I would rather go for an X engineer than a data analyst, because that an X engineer can also. It goes. He goes beyond just modeling the data. It's modeling up until the visualization of the data and then hopefully business teams are gradually becoming more and more data literate that they can work with those repos themselves. You don't need the analyst to kind of pre analyze and give you the insights upfront anymore. So you can. You can basically go from model to report and business consumes the repos themselves and it's not an analyst anymore and it's kind of crunch the numbers and get insights out of it. But the younger generation is now kind of filling a lot of those, those business units as well and they can do more data savvy. So they can, they can read data much better.
Speaker 1:Interesting trend to have thought about it like that. So you're saying like more and more people are becoming data savvy and maybe there is less need in a specific data analyst role.
Speaker 3:Yes, but then analytics engineer will cover more because you will also cover the visualizing part.
Speaker 1:So that's why I'm saying it's not just a dbt engineer it goes to meet, to meet also much more than just the the tech stack Like. It's to me like if you have a, like a spectrum like the data analyst and the data engineer. The data analyst is extremely close to the business and the sense of business is very well, is data savvy in a sense, very good with Excel, these type of things, all the services spectrum. Like the data engineer, much farther removed from the business, he doesn't want to know about the business and but there's a lot of our technicals. How should we model stuff from a technical point of view? What are best practices? We keep this maintainable. And the analytics engineer is a bit in between and, like, still very close to the business, also knows these, these best practices. And indeed, like in a changing world where people are becoming more tech savvy, where maybe you need less, I think a lot of depends on the tooling as well.
Speaker 4:It goes a bit hand in hand, if you can say because dbt.
Speaker 4:Indeed, that made analytics engineer more popular, because there was a tool now that everything that analytics one engineer could do can do easier. I think, for instance, becoming more tech savvy, that there are more, less visualizations that an analyst is doing, that the people who are using the visualizations creating by itself is again Microsoft co-pilot A lot of those things that you can just ask. I think those are the tools that are going to help them to get their insights. So I think tooling with the roles evaluation, that goes actually hand in hand.
Speaker 2:Maybe a question then. I mean I understand from from what I hear is like analytics engineer does more than just create dbt pipelines or whatever. There's also the visualization is the whole business understanding. But can you have an analytics engineer that does not know dbt or do you feel like this is a is there.
Speaker 1:Like a lot of people, that's very hard. What would be the cool bump into dbt at every corner.
Speaker 4:Yeah, yeah, but I think well, that's my personal meaning I think you can be an analytics engineer without having to use dbt, because it's about putting in things into practice, like the versioning part, like the putting the best practices, like the documentation.
Speaker 2:So it's like you advocate for these principles, and dbt is a nice box that kind of carries everything, so it makes your job easier. But if you don't want to, you could and is today the the fact to standard box.
Speaker 1:Yeah, these things Exactly and it's huge and, like communities, huge they announced today on LinkedIn that they reached their slack community as 100,000 members. Really, 100,000 is crazy.
Speaker 2:Almost the same as our listeners.
Speaker 1:Yeah, they've had time to go.
Speaker 2:We'll get there. We'll get there. That is interesting Indeed, maybe talking about that.
Speaker 1:But I also find interesting about dbt as a tool, technical tool, that there is it's such big community so much hype, so much like a bit of a paradigm change on how we, how we approach this and it is so super simple as a tool. Yeah, and it's literally just. I have a tool that says please execute these SQL files in this order and populates them. That's the tool, right?
Speaker 2:Yeah, like it's, it's, it's very interesting to see that something so with some templating right like take this X and I'll put a number of whatever. Yeah.
Speaker 4:Funny thing that I read on an article was someone mentioned that people often say, yeah, but what dbt do is I could do it also in my Python scripts.
Speaker 1:Yes, everyone can do it with their Python scripts.
Speaker 4:But dbt create product ties it.
Speaker 2:Yeah, exactly Also. They may look nice, you know, like they came up, like they put a lot of stuff, but that's I think. That's why what appeals me, you know, is like such a simple idea, but it's just genius because no one thought about it. It's not like no one could do it, but it's like it was no one thought to put it in a product.
Speaker 2:Yeah, but like do it like I mean, yeah, before I learned about TBT I did something like that. I had a Python script that will go and iterate and change this and change that. Actually I was using Snowflakes or scheduling tasks and all these things. But because the challenge I had, I had a data analyst. That was really good, he really needed the data, he knew SQL really well and really the only thing I wanted is to say, okay, run this, then run this, then run this, then run this, and then I wrote a script and blah, blah, blah. But even then at the end it wasn't as good as TBT. I feel like TBT comes with a big community. It has a lot of it's like very battle tested and yeah, I think to me that's what's the appeal. It's like there are some things that some people invent and I'm like, wow, this is crazy. No wonder I didn't think of that. But then you look at TBT, it's like I could have thought of that. But I think that these things for me makes it even more genius.
Speaker 3:I don't think a good idea needs to be rocket science. I think if it's sometimes kind of having the perspective to solve something very practically and that can be a very simple solution, can be a huge invention or a huge addition to the.
Speaker 2:I completely agree, but then that's why, for me, is the perception, having that different point of view and seeing that, that to me I think it's like wow. That impresses me more than something that is super complex, that I would never thought, because I don't have the Everyone had the same information, that they did, but they just had a moment. There is like oh yeah, let's do this. So I think it's really cool. One thing you mentioned, bart, is the data engineers and the business or data analysts.
Speaker 3:So there's a spectrum.
Speaker 2:A question for Marty a few, bart, so we can relax. I mean, you can't. He was waiting for this. If you ever heard, it's just the first time you hear this sound it probably is. Bart thought it would be good to include this in our buttons. It's a fun sound, right? I was just challenging him in before. He's like why do we need this? We should put something else. He's like oh it will come handy.
Speaker 4:And that's the first one that he uses.
Speaker 2:Yeah, it's the first one, he was there, his finger was twitching.
Speaker 4:He was like. I saw him looking at the button. I thought it's going to come now.
Speaker 2:But my question for you, Marty, is how does analytics engineers support data engineers, Like do they relieve the pressure of data engineers? How is this?
Speaker 4:I think a part of it I mentioned before. So data engineers I think they do quite a lot of hard work into creating the pipelines, making the data clean, putting, hopefully, some data quality checks and stuff, but in the end it's not maintained. So they do transformations but when they go through to the business or a data list or a data scientist, they are like, yeah, but it's not done how I need it, so it's not done correctly. So they started to redoing stuff and in that process I think they might use melt practices. To say Just a small example, for instance, data engineer put their time to create tables with everything in lowercase and putting an underscore in the name, but when data analysts or data scientists are working on their data, they created tables that they wanted to have, but with uppercase, lowercase, all mixed up. So it's like the things that data engineer implemented they are not maintained somehow.
Speaker 4:So I think how analytics engineer would support them in this process is to take those press practices, to take the work that they did and maintaining it, saying okay, now we are using deployment pipelines, we are keep going to use deployment pipelines. If you want to create a view that gives an overview of this tables, we are going to keep using that view. We're going to test this on development or create it on development. We're going to put it to UAT and then put it into production and not just create a view in the production. So it's a bit of like they set up something that it's called. It's used throughout the data lifecycle. That's what I can explain.
Speaker 2:Yeah, yeah, that's true. I think the breadth. Let's say that analytics engineers they can put different hats.
Speaker 3:I think they understand.
Speaker 2:I think it's also good.
Speaker 3:You mentioned a bit earlier, data engineer doesn't always care about the content of the data that they're working with and analytics engineer does so. From that perspective, if you need to model data, you know what the fields mean, you know what.
Speaker 3:Okay, it might be the right format, it might be a string and you expect a string Great, but it's the content that you expect it to be, and that's something it's very hard to judge if you're more on the technical side and you don't see how business is using it, what it means to them, and how it's combined with other elements to actually drive decisions, support, insights and so forth. And so there, I think they complement each other, where, on the one hand side, you want to make sure that the data feeds into your data platform in a clean way, but then making that data meaningful requires understanding the content of it, and if you try to do that for an entire organization, yeah, good luck. If you think an analytics engineer will typically more I work more for specific business units or be assigned to specific business units, and in that sense it's much more manageable because you're much closer to it and you have a better understanding of how the people on the other side of that so it's like data set, use it.
Speaker 2:It also bridged a bit the silos as well.
Speaker 3:Yeah, otherwise you iterate a lot. It's like it's stitched together this way. It's like nah, this isn't how we're using it.
Speaker 2:And if you have analytics engineers more embedded on the different units. Are we also supporting a data mesh like?
Speaker 1:you said it Wow.
Speaker 2:It was on the notes, but thanks, but yeah, maybe what's a data mesh for people that never heard this before.
Speaker 4:Okay, I'm going to take my part. So I think the best way to explain data mesh would be that in I'm just analytics engineer or a data analyst, so that we have data literate teams. So in that case, instead of that we are that there is data requests are just coming. So with the data request, I mean inside request or visualizations request or analytics request that are coming within the data team. We're going to work the other way around, meaning that the data team is going to provide the data as a product and then the teams are going to consume data and create their own insights and stuff. So this way, analytics engineer, for instance, would be closely working within a data team and it will be making sure that the request that within the business team sorry, so he will make sure that the insights that are asked by those teams are delivered by the data team. So delivering data as a product by data team and consuming it and saying, okay, what can we do or what insights do we need from this?
Speaker 2:How much is this adopted? These days, you feel More and more.
Speaker 2:Yeah, I think I have a feeling that as data becomes more, everyone understands the value that everyone's like okay, I want this day.
Speaker 2:And then I think what I observe people see the value and they start asking a lot the data team that was centralized. And then they start hitting limits and they realize as well that maybe there are some things they could do and they know the business domain for their unit better. And then they say, oh, maybe I can do this. But then it's like okay, but if you do this, I also make sure you update this. I want to make sure there's documentation if the definition changes. And then you start saying, okay, then I'll own this, and then this kind of starts bubbling up in different places and then you kind of get more to a data mesh kind of. But it's kind of like natural. But I think the first time I heard about data mesh it felt like it was something you need to be very intentional about. But I have the impression now that it's almost like a natural progression of things. You know, like people are kind of self-organizing more in these ways.
Speaker 3:Yeah, I think what I've seen at many organizations is the journey I described a few moments ago, where you go from chaos to kind of a centralized data team and at some point they become victim to their own success. They are serving business, they have a lot of demands, but at some point they just can't follow anymore and everybody gets frustrated. They get frustrated because they can't follow Business, gets frustrated because they need to wait too long for what they want. And that's a bit of context in which I was also supporting an organization four and something years ago when I first heard about data mesh and when I heard the concept. And again, it's a bit like what we said with DBT. It's not rocket science. I mean, what Zammak defined was kind of a logical evolution. Federated operating models were something that people were already talking about for quite a while. She just coined it and kind of described it as the logical next evolution At the right moment when it became relevant for a lot of organizations. But so, yes, then the solution somehow is let's then, as a central data team, just focus on the infrastructure, the governance, making sure that everybody works in a consistent way and that we can kind of plug stitched stuff together If it's a data product that finance develops and then marketing makes one and operations makes one. And how do we make sure that?
Speaker 3:At some point you might want to see what's the cost of this and who worked on it.
Speaker 3:And that means you need to combine data from HR together with data from finance together with data from operations. But you can do so because the standards are the same and in order to have those standards, you need good governance. And that's why it's not a big bang. It's not like tomorrow I'm going to implement data mesh, but it's more like that's the vision we want to evolve towards. So that means we're going to gradually train the business teams or make sure that they hire the right profiles to be able to do so. We're going to put in place the right governance to have those standards, have those things that make it interoperable further down the road, because otherwise we're kind of going down a one-way street and evolve into that direction. I think that's where a lot of organizations are today. I think nobody is like full-blown data mesh, best practice, everything, blah, blah, blah, and probably two organizations will have a different interpretation of data mesh, which doesn't really matter. It's the idea that counts, but that idea is stuck, so that's the interesting thing.
Speaker 2:I think it's more like, indeed like the intention, right, like you want to decentralize, you want to do this. I think most times in practice, you have an idea, you have a name, but there's wiggle room right. There are different flavors of the same thing. Actually, maybe a side note tangent Not so long ago, I heard that Scrum is actually a very strict definition and on the definition of Scrum, they say that anything that is not exactly what you're listing there, it's not Scrum. Did you know that? So, like, if you don't have daily stand-ups every day, it's not Scrum. You're not doing Scrum, you're doing something else.
Speaker 2:Like, but they are very strict and it's like then who's doing Scrum? Like who is you know what I'm saying? Like, but to me I feel like this I mean, I don't know who wrote that and why they wrote that, but to me these very absolute statements are very counterproductive.
Speaker 3:Yeah, they're some ideal world.
Speaker 2:Yeah, it's like who lives in this, you know rainbow Same with Scale Edge Island.
Speaker 3:Yeah, spotify model, and if it's not a Spotify model, it's not Scale Edge Island.
Speaker 2:But yeah, but it's like who cares right, Like, why are you so nippy about this?
Speaker 4:You know, it's like I recently heard a funny saying say. Someone said we doesn't work in an agile way, but we work in an agile spirit.
Speaker 1:Yeah, yeah, yeah, I think that's politically correct.
Speaker 2:You're not going to offend anyone by saying that In a previous live.
Speaker 3:I heard people, a lot of people, speaking about what Gile. It was like we're working a bit in between waterfile and agile.
Speaker 2:But that just sounds weird, Like no. Thank you for your contribution. All right, maybe a question for you, Mariam. Well, is there something like similar to analytics engineers, to AI engineers as well?
Speaker 4:Yeah, that's some brain food that I put there, and I think we have a term that pops up here and there is AI translators and in my real thinking, I think those would be the person.
Speaker 4:I don't know if it's similar to analytics engineer, but I would say that those would be the people.
Speaker 4:I think we needed them more and more in today's everything that's happening now, that makes understand, that makes business understand the use and just general concepts of AI and the tooling.
Speaker 4:So I think that is someone like analytics engineer, would be someone closing, standing close to business and saying you know what's a judge APT or generative AI. You can use this in this way, saying, for instance, I think a lot of concerns that people have at the moment or the companies have is judge APT safe? That would be a concern that analytics engineer, ai translator can take up and say, okay, maybe the normal judge APT, the online version, is not safe for your company data, but there are other things that you can implement for implementing via Azure or something else that would make sure that your data is safe. So this person would make it help them with their day-to-day business questions that the or insecurities that they have regarding security costs are also to see do you really need it, do you not need it? That would be someone closer standing and making it sure that what's AI things are implemented. It's not a black box. It's more visual because it's happening so fast.
Speaker 4:I don't know if they can take it up.
Speaker 1:It reminds me very much about a term that McKinsey coined, I want to say four years ago analytics translator.
Speaker 4:Yeah, I came through their articles as well that they also implemented really a training that put people into analytics through AI concepts, but also from the business concept. But I think that's a good idea.
Speaker 2:Yeah, I have a different opinion Well, not different necessarily On what On well analytics engineers for AI. So my opinion is I think, like I said, you can quote a lot of it.
Speaker 1:No, wait we also got confusing terms, not analytics engineers. Mckinsey coined a term analytics translator. Yes, yes, yes.
Speaker 2:No, but what I'm saying. So the question originally was is there something like analytics engineers for AI? Okay, okay, right, so it's not really. I'm not against what you're saying. It's like a bit of a side parallel track. Okay, good, but what I think? So there's a lot you can do with SQL. In fact, a lot of SQL engines they have like an ML like Redshift has Redshift ML, bigquery has BigQuery ML.
Speaker 2:Snowflake has this and a lot of like, I still think, well, a lot of AI cases that you need to build models are still from tabular data. Yeah, sure, right, tabular data. Think of SQL. A lot of the times, there's a lot of AutoML tooling that does a lot of the work for me, right, like you can do some feature engineering in SQL, maybe, like usually the AutoML tooling is just for you to select the types you know.
Speaker 2:Maybe it's a categorical feature, so meaning it's like there are different categories, but maybe an SQL is expressed as a string, but there's only five different strings, right? So usually you have a UI that you do that and then you say, okay, train a model for me, and then you can actually export this model as a UDF, a user defined function, which basically is a function as a transformation SQL. If you do that, once you have the UDF, you can actually stitch it back and then that just becomes a transformation step in your dbt pipeline. So what I'm saying is like, if you know SQL well which arguably analytics engineers should- you can do a lot of feature engineering.
Speaker 2:You can prepare the data. You can also like. There are some data science things like is there data leakage? What are the precision recall? How can you detect these things? Right, there's a bit of there, but I don't think it's too much for people to pick up In my opinion.
Speaker 2:I think it's still manageable. And then you can have someone like an analytics engineer. They can also build machine learning models. Maybe they're not going to be the best ones, but they can put a lot of models. They can do quite a lot of stuff, they can schedule stuff, they can have the pipelines Right and I already have a name for this. Go for it Machine Learning Analytics Engineer. It's a bit long, so maybe we'll just put that yes. Or you can put the money too, you know, since you're a coin and stuff, there we go. And if you're wondering how Barton came so good at pressing buttons, all of a sudden, he actually he put some labels there.
Speaker 2:So I have to curtain behind you know it's like, uh yeah, that's why we're late for the live stream. So I apologize but reiterate what is the title going to be? Machine Learning Analytics Engineer? It's a bit longer. It's a bit longer. M-l-a-e for short. Patent pending.
Speaker 1:Yeah, but what you're saying is Machine.
Speaker 3:Learning Engineer was already taken, and then Machine. Learning.
Speaker 1:Engineer was already taken Exactly.
Speaker 2:So you just, you just put it together, you know just like. But you're actually not challenging the. No, I'm not challenging that. The AI. Yeah, yeah, uh, but I think it's like it's something else. It's something else, it's something different.
Speaker 1:That's what I think, If you're saying that the analytics engineer role will will evolve to also encompass that part. Yeah, but I think More easily.
Speaker 2:But I think you could also encompass what you're saying. Right, like, if it's someone that has domain of the data of the business, right, they're already close to business, they know how it works, and then they understand a bit of ML, they can explain a bit how these things work, right, okay, like they can, like they can coexist.
Speaker 1:But I think You're saying, because this technology become easier and easier and easier, that this might be a natural evolution of the world. I think so.
Speaker 2:I mean, I think there is an opportunity there. There is stuff on the table you know, Like if most of the use cases you have are tabular, Like you can do quite a lot with SQL, and I think it's like the.
Speaker 1:And do you even need SQL to be as from now?
Speaker 2:Well, that's another question right, you do the natural language. You could do natural language, indeed, I don't think it but it's too early. We're going to get to the GPTs. Okay, sorry, sorry.
Speaker 3:Do we really need a separate rules too For that? I say, somehow you have, if you take the ML engineer, you kind of make sure that the that, especially the more complex ones, are built really robustly. It's a time and you kind of then in your comparison you compare that to a data engineer, I guess, because that's you are saying like, what does that mean for AI? Didn't the kind of the role of a data scientist, wasn't that kind of the point to kind of make?
Speaker 2:Yes, you're just saying, I think.
Speaker 4:Because I think that's because data scientists were like I think we're completing a lot of transformations and stuff, so they were spending a lot of time cleaning the data, creating the models, and that's what you said that now that they're easier to lane, now they can do it more easily and if there's better data, maybe they can spend less time on creating the models, but more also explaining it, like what is the use for it? How do you can? But I think again, yeah, data scientists, but then there would be like more into the business of they would have to incorporate more and more into the business as well.
Speaker 3:Yeah, for me the scientist had to be a bit close of the business by default.
Speaker 1:Yeah, I think that's what. I think. The bit depends a bit like also on the scale Like if you have a huge corporation like, you tend to focus on a specific role. If you're a smaller team, you tend to take a bit more diverse roles a bit of business, a bit of tech, a bit of.
Speaker 2:Yeah, I just think that again, the feature engineering part, a lot of the struggles I see are in the compute right, because there's a lot of data but SQL you don't hear there's much of SQL, right. So if you say, okay, I'm going to do build and features SQL, in the end a model is just a function, it's a transformation right. Like how you get there. It's a bit more complicated, but after you have a model training, it's just that right and we know how to do this in SQL, like you just put there.
Speaker 1:Yeah, but still I think, like for everything, like you need to build up a bit of expertise and something to focus on it. If you're like in a, if you want to make it your, yeah, if you want to be very good at that, like I think at some point like there are trade-offs to say like let's create dedicated roles for something specific.
Speaker 2:Yeah.
Speaker 1:Versus.
Speaker 2:Let's make a role that is very versatile, like there are, yeah maybe the analytics engineer will just start doing this and we'll still call analytics engineer, right? I would still call the machine learning analytics engineer, even though it's long. But that's just me, right? But I do think it's. I don't know, I just feel like it's a natural thing. I mean, you can still have a world where you have analytics engineers that create this like SQLs and whatever in the pipelines, and the machine learning engineers or the data scientists they create the UDF, right, and you can just plug it in. But I do think there is a I saw this is also a pattern that we kind of see sometimes. Maybe I digress, maybe I digress, but now you mentioned GPT, natural language, all these things. I only mentioned natural language. No, my bad, what do you mean by natural language, bart?
Speaker 4:What do you think that's? The first thing coming to your mind.
Speaker 2:No, but last week, unfortunately we weren't able to record. We had the Roots Conf. We also released the part one. Part two coming soon, right, bart?
Speaker 1:Yes, we actually recorded a. Roots Conf. Yes, yes, yes.
Speaker 2:But last week as well, there was also something that came out that was pretty cool. La Plata Forme. You like that?
Speaker 1:We need to ask. Kevin is perfectly bilingual, well frilingual, I would say. Can you say this? How should we pronounce this in French? La Plata Forme Sounds sexy, yeah.
Speaker 2:Sound cool. We got warm here. Yeah, you know I'm a bilingual. It's like I have more than one, but I forget all the languages sometimes. But yeah, what is this? So? Mistroai? It's a French startup that actually raised also the news.
Speaker 3:Paris, right yeah, Paris based.
Speaker 2:So EU based kind of competing with OpenAI right and they released a very JPD-like API right. That's what's the La Plata.
Speaker 3:Forme.
Speaker 2:I gotta stop saying this, otherwise.
Speaker 2:Oh my God stop. So yeah, I mean it's basically if you don't like JPD or whatever, if you like, support EU companies. I guess this is an option. Bart, I know for a fact that you've played with it. I did. What is your feeling on this Before you go in there? I know that when they released this as well, they advertised that this is better than JPD 3.5. Yeah, again, we already discussed here before that benchmarking JPD models a bit tricky right, a lot of times very subjective. They seem that there's a lot of potential, but maybe, bart, what was your experience with this?
Speaker 1:So maybe just to answer that so the platform was released and the platform is simply managed models. So they host the models for you. You get an endpoint and you can send an API request and then you get a chat completion that's what I play with or an embedding back. That's what Lapla, that's what it is Laplatform, that's Laplatform.
Speaker 2:I needed to put that in a button too.
Speaker 1:That's what that is. But also they also released a new model. I don't know exactly when I want to say last week 7000 parameters, something. Very good one, and that's the one that you're actually talking about. That was better than.
Speaker 2:But the model is the same as the endpoint, or no?
Speaker 1:Well, that's a good question and I'm not 100% sure. The documentation is a little bit vague. So, when you use the endpoint, you have Mistral Tiny, you have Mistral Medium. No, you have Mistral Tiny, you have Mistral Small, you have Mistral Medium. Mistral Small is said to be using the model that was released via Torrenlink Quite interesting and the Mistral Medium is the highest quality endpoint, but it's a bit vague on what it is exactly. But probably the information is out and didn't surge enough, but I use that one, which is apparently the highest quality one out there today.
Speaker 3:So interesting. So they use the same approach as Gemini, having a tiny version and something that can run on the phone. Basically, I suppose, yeah, I think so, something like that. And then bigger and bigger versions.
Speaker 1:So I played around with it and I think it's fair to say it's better than Chats GPT 3.5. This is just purely based on playing around. To me it's a bit like Chats GPT 4 on Shrooms it hallucinates a lot. The code generation is actually quite nice, but when you ask them to summarize factual stuff that happened in the past, it's half of the time wrong.
Speaker 2:Interesting. But then and that's my experience when you say summarize, do you give the information or do you just ask the web?
Speaker 1:No, it doesn't access the web, so I just ask it. To who, for example, I can ask where's Marilo? You have a long name. Can you tell me your name?
Speaker 2:Marilo, look when you ask that queen of it.
Speaker 4:This is after Kevin, who is this Marilo.
Speaker 1:But I can ask who is this Marilo that works at Daydreams and it will give me a very nice summary and 50% of that will be correct and 50% will be like you will think oh wow, yeah, yeah, you're trying to motivate me. No, no, but like it's going to be a bit overkill, but you didn't pass my like. You didn't look online, no this is just purely based on the weight, on exactly the data that I've seen.
Speaker 1:It knows about me, then it knows about you, yeah, but it's more impressed about it to you than it should be, wow that's consensus.
Speaker 3:Yeah, right, where's the button?
Speaker 2:Where's the? There we go, All right, Moving on. I guess no, no, no.
Speaker 1:But so we said that was an interesting experience, but I do at the same time, I think it's impressive what they already have.
Speaker 2:Yeah, but they actually have been raising quite a lot of money.
Speaker 1:They raised a shit ton of money.
Speaker 3:But actually no. I mean, yes, they did, but this is kind of it's an interesting thing because so far Europe has been on the forefront of regulation but not really on the model side yet. So we were regulating companies that were somewhere else, but Mistral is the first high-profile one, because they openly fight open AI, claiming that they at least are open. I want to challenge that.
Speaker 3:I will hold the egg on this oh okay, and they raised I think 133 million a few months ago and now raised money again I think 450ish. But there's a German company called Aleph Alpha which also raised 460 million a few months ago. And then the difference is that Aleph Alpha is closed. They target government and private organizations and have a different business model, which is fine. Currently, there are only 61 people working at Aleph Alpha, which, if you compare the open AI 1,200, it's like what? So it's really not the only European AI, llm Gen, ai company spinning up. They've all developed their models. It's the open source one. Yeah, exactly.
Speaker 1:The open source one part, and I think, because it's the open source one, it's a bit like tutored as Europe's open savior on LLMs. And I wonder if their strategy on open source is fully for the greater good, because if you look at the people that let, if you look at funding rounds 450 millionish none of them are NGOs right.
Speaker 4:They're all commercial investors.
Speaker 1:That's why they want to make money. So if you look at the license, that is Zander, but you do. It is not hard at some point to say a new version we will not publish. They will say like, maybe if it's based on the older version, it will still relate to older version. It's not hard to change that right. I hope they never do it. They will always stay open source. But at the same time, because it's open source today, they're a bit tutored as a savior. It allows them to grow very quickly. Everybody knows about.
Speaker 3:Mistal.
Speaker 1:Much less people know about Alpha, for example. At the same time, their product is not really up to par. If you look at the platform, it's just starting out. It's literally just an endpoint. It's not as far as open AI with its assistants and stuff like that, but they have a huge community because they open source. They get a lot of benefits from the open source part. If you look at the Apache license, it's very interesting to see that because they publish it like this on the models that they open source, not on the platform. On the models they have zero liability and they don't have to provide any warranties because they publish it like this. An interesting thing to think about as well.
Speaker 2:Even when Sam was here, we talked about the different open source models. Yeah, but there was something like a law that if you're open, source stuff.
Speaker 1:That is something in the making that would change this potentially in the future, but that is today's not the case.
Speaker 2:So your hot take is that they're not open source, they're just good at marketing. In other words, open source equals market.
Speaker 3:They're going to start with something that's open and as soon as it's successful, they're going to make a paying version of it. Why do all these people put money in this company Is at some point you get money out, and if you're forever open source, there's not going to be any return on that investment.
Speaker 4:But also the regulations part. That's what you're also mentioning, that they're trying to avoid that they have to put in documentation or follow all the regulations by being open source.
Speaker 1:At this stage. We'll see what the AI Act does. I think the AI Act will change that.
Speaker 3:That was an article that came out two or three days ago reporting that the French government has lobbied a lot at the European Union for the moment to weaken the AI Act Because now they have a company that's playing in that field and suddenly they have an interest. So, it's like maybe we want to tune it down. The Germans are the same Because they have Aleph Alfa, but they also have Helsing, which is even lesser known because it's in the defense industry. So it's Gen AI, but for defense.
Speaker 1:What I'm wondering about if we ignore a bit the AI Act, if you take something like this from a legal point of view so they create something, they publish it as open source in this case, apache 2, where there is no liability, there is no warranty, and then you create a hosted service. I'm thinking, but I'm not sure about this, but I'm thinking that it will be liable as a laser of that host of service. Can it respond quickly enough, can it take the load, does it function as the API specs work, but that they're not liable on anything. That's the output of the actual model, because the model they're hosting is an open source asset.
Speaker 1:I'm wondering about this. I'm not sure on the legality of it. It's an interesting discussion.
Speaker 3:But it's going to limit because the AI Act doesn't come into play and I think if any company wants to build something on top of it, they will need the one building something will need to require Mistral to comply with a certain number of provide evidence that they comply with a certain number of things. And then if nobody builds something on top of it because Mistral says, no, it's open source and so we're not going to provide anything, nobody's going to build anything on top of it and in that case it's going to collapse again.
Speaker 1:So they will have to yeah, but definitely I agree with that I was just trying to bring back.
Speaker 2:The open source equals marketing. And then it's not a new take.
Speaker 1:I know that we said this a while ago, but it was a bit too tricky for people. I don't think open source is marketing definitely not, but I think you can position it well. I think it is strong in your marketing. Yeah, I think it's still open source for a lot of different reasons. As a greater good aspect to it, it informs people about your doing, how you're doing stuff, what is the state of the art, and don't put it behind a payroll, yeah, but just because something is for the good does it mean you sacrifice?
Speaker 2:yourself, but we can't ignore that it's an important market for people. I mean there are benefits to you as well. Right, like you said, the liability stuff, there's the community, there's brand awareness, there's all these things. It doesn't need to be. If you're doing something for good, does it mean you always need to hurt yourself? I agree. You also mentioned that the way they released the model, the big model, I think 87 gigabytes or something, not sure. They actually just released a tour link on Twitter Torrent, not Tor, sorry, torrent Thanks.
Speaker 3:Torrent that's been a while.
Speaker 4:Yeah, I was thinking. Torrent, it was to download movies.
Speaker 2:Whoa For police, just right here.
Speaker 1:I never downloaded a movie. Yes, it's just a piece of paper. Why are you?
Speaker 3:crossing your fingers by me.
Speaker 2:Maybe the question is Okay, the models open source, cool, so what does it change a lot? Like, okay, I can download the models. Well, first of all, can I Do I have all the space? Do I have the hardware? And if I do, can I run the inference? Am I really like? Is this now like, okay, anyone can do this now, kind of deal, or is it still limited to the people that have the infrastructure resources that can afford for this? I think inference everybody can do it. Yeah, you think it's fine to like if you download it.
Speaker 1:I need some resource to do it, but I think for the inference is manageable.
Speaker 2:Is it financially sustainable If I download the models and I just rent the GPU on the cloud and just run it? Is this like match kind of what you would pay if you have the platform or platform? You're getting better at it.
Speaker 4:Yeah.
Speaker 3:Keep practicing the beer.
Speaker 1:This is a difficult question and it's a bit like do you want to buy it or build it yourself?
Speaker 1:Well, like you get here, basically you get like a major component to build it yourself, but you're still building the tooling around it yourself. If you want to use it day to day, yeah. And nor like if we don't look at it from a point of view like I'm in my notebook and I'm experimenting better, yeah. But if you really, if you really want to automate some process with it for example, like I would use Kevin, what would they use the platform? Yes, yes In this case, but at this point, yeah, I guess it's just like if there are privacy concerns you know like, and then, oh, let's host something.
Speaker 2:But I also heard that in the passing. Well, if you want to host something yourself, it's going to cost way too much. So it's too cheaper to just go with ChagPT or OpenAI or whatever right.
Speaker 1:But that depends a bit on like, what are you building? Right? Like, if you, if you're what is, if you want to build like a small web shop, to build your to sell your Christmas hats, and you want to use this to automate some translations OpenAI or Kevin, what the platform? That's probably fine, right? Like there are no real data privacy issues or like intellectual property issues that you should be concerned about. Yeah, but it's the moment that you're the, the National Police Corp and you're you're doing this stuff. You want to translate stuff that is related to investigations and you probably want to be on-prem in a very, very secure environment and you want to do everything yourself.
Speaker 2:And you don't think that, you don't think that the costs are much different in the two.
Speaker 1:I think it depends on like the use case should give an answer on. Is this cost realistic or valid for use case?
Speaker 2:No, but I'm really just thinking like compared with Chesh PT. You have Chesh PT, anyone has the same thing on the platform. Sorry, it was a bit sloppy. Are the costs about the same Should you worry about? Is this something it takes into account or I don't like, I'm just the thing is that the way I use it, it is so minimal.
Speaker 1:I'm not processing thousands of PDFs every day. I think. Then this at that level, you need to start thinking about the cost from the API endpoints point of view. I see, I see. I know that when Lab platform came out, they defined and maybe I'm a little bit bullshitting here, but I think it was two euros or dollars per one million tokens, something like that. And that announcement came out and a number of other platforms immediately said are we going to lower it?
Speaker 2:Oh really.
Speaker 1:So there is really like a price competition at this point going on.
Speaker 2:Yeah, but ChedGPT still feels like it's ahead. Right, I think it is.
Speaker 1:Actually I heard very subjectively, just from my own experimentations.
Speaker 2:I actually heard that. Sam, what's your first name? Sam? The guy from OpenAI Ultimate. Yes, he said in an interview that no one can catch up with OpenAI. They're too far ahead. And he said this and he's like well, it's your job to try. I guess you need to try to beat us, but you're never going to do it. It's hopeless, he said.
Speaker 3:Everybody's comparing to GPT-4 for the moment and rumors say that they have 4.5 ready to come out now-ish, any moment. So that means if everybody's comparing themselves to their old version, yes, then they're miles ahead. I mean and the same with images, because the Dalai Tri was nice, okay, stability now of E6 was a major new E6, sorry that released this week somewhere Yesterday Yesterday was. I mean, the images are amazing. So on that front maybe a bit less, but on text it's.
Speaker 4:Yeah, If you look at the popularity of GPT, I think everyone. It's also how much data you put in or people that you're using it. They're already training the model. They already started quite a long time ago, so everyone is using it. Everyone starts with GPT. Okay, then they might go to other platforms, but they started there, so it's already doing a lot.
Speaker 3:They're on Microsoft. Yeah, it's already popular. It has a lot of attention.
Speaker 4:It has a lot of money. I think a lot of it's in their advantage.
Speaker 2:Yeah, I agree. I agree with that, and you mentioned also image generation.
Speaker 3:Yeah, mitjerni was a Peter de Bözer, an old colleague and friend of mine. He made this small video on LinkedIn, I think not a video, but like clickable pictures.
Speaker 4:I don't know how Carousel yeah also French word.
Speaker 3:But just saying the first version I think was he went through the versions of Mitjerni and you could kind of click and every time he had the same render with the same prompt, but in the newer version, and the differences are in a year and a half it's insane.
Speaker 2:Yeah, but we have even on the tools, so the previous version of data topics. I'd say there are some image generated, ai generated images and like the difference is crazy.
Speaker 1:And so for me personally, I used Mitjerni v5 up to v5.2.
Speaker 1:I think it was the latest version A lot until Dalit Riekenmaier came out and the major difference was that Mitjerni v5 and everything before that it was very much descriptor driven.
Speaker 1:So you had to be very specific on it's not just this Brazilian guy wearing a Christmas hat standing in the snow, but you also need to be, you need to have the scriptals exactly how you wanted to look, to basically think a bit about what was this data trained on? I wanted to be HDR 4K inspired by ArtStation, to really have this style. So of course, you use words, but it's not really natural language, and Dalit Riekenmaier changed this by really having natural language. I want to have a picture of a Brazilian guy wearing a Christmas hat standing in the snow, and then you get a first version and then you iterate on that by adding a small description and, while not exactly the same because you don't have this chat functionality, the v6 now also is very much a natural language. You don't have to really think about the scripts, just in natural words express what you want to see, and it's really way, way better than it was.
Speaker 1:What I also notice is that text generation is way better and, I think, on par with Dalit Riekenmaier. Text generation in images. You mean Text generation in images. What I mean is that I want to see a guy holding a logo that says the city is over there, and if you used to do this with 3 version, go and look there are letters, but it's horrible.
Speaker 1:And it's still a bit horrible, like with Dalit Riekenmaier and with v6, like you still need to do a few retries, like you get typically four versions, like in one of them, and it's okay, but you can get there and it's like to me also. That is a bit on par now with Dalit Riekenmaier. So that's interesting to see and I personally like the style of mid-journey much more. Like it's more of an artistic style Interesting.
Speaker 2:We talked about text. I'm going to move a bit on, I don't know if anyone will. We talked a bit about text. We talked a bit about images as well, but all about audio generated. Audio generated yes, so right now we're in a Christmas Video.
Speaker 3:Is that next Because?
Speaker 2:Video as well, I guess. But if you say, like videos, like sequences of images. Even though there is a component right, you need to sync it through.
Speaker 3:Yeah, but we had a colleague of ours posted some stuff today, when it's always short clips like two, three seconds, whatever, and two out of three were actually quite interesting. It was one with the train. It was a bit strange because the was going forward. It was a bit strange. Technically shouldn't happen if there should be a lot of wind for the train to go forward.
Speaker 2:But that becomes better and better being better quick as well.
Speaker 3:Yes, that's for me that's going to be the thing of 24.
Speaker 2:True, I think it's there.
Speaker 4:I think it's up there, I guess isn't it that Ciam and I also had integrated in it that you can? That either they could recognize the video of what was happening in the video. You could upload the video in Gemna and then recognize what's happening and you can see okay, is some, if you put an exercise or something, okay, is it executed well or not?
Speaker 3:But was it the demo or not? Because, gemna, I don't know the controversy.
Speaker 4:Yeah, yeah, it was not in their official demo.
Speaker 3:The official demo was apparently completely mocked up A bit.
Speaker 2:It was completely manufactured, but audio generation.
Speaker 1:Yes, you sent me a few things.
Speaker 2:I did send you. I'm not promising it's good.
Speaker 1:You sent me some AI generated music.
Speaker 2:Music is maybe a strong.
Speaker 4:It's a side career.
Speaker 2:Maybe music is a very strong word for it. There are some sounds AI generated. This is also a festivity. You know a?
Speaker 1:Christmas time. We made it a bit of a competition, right.
Speaker 2:Oh, did we. I knew it was a competition I probably would have. It's always.
Speaker 3:Yeah.
Speaker 2:I know right.
Speaker 1:We each have three short clips right.
Speaker 2:I sent you three. I sent you maybe more, I don't know, but it's not, oh, you have four you have three, you have more chances. Yeah, but I don't like my chances.
Speaker 1:And we used a Hucking FaceSpace by Facebook MusicGen. It's a Facebook-based model.
Speaker 2:Right, you used the same right, yes, meta, sorry. Did you pay for the extra, the more expensive stuff, Because I thought you could pay no you bought it.
Speaker 1:No, no, no, no. We have a Hucking Face on.
Speaker 2:Purely prompting skills. We start with yours.
Speaker 1:Sure, let's do it. And what was the assignment?
Speaker 2:So the assignment was to generate Christmas-related songs using this Christmas atmosphere. Christmas atmosphere yeah, and I tried different things. I'll talk a bit about it afterwards.
Speaker 1:Yeah, I'm wondering what. Let's see. If I'm not sure if we can play this line, let me try it.
Speaker 2:That's the wrong one. It's the wrong one.
Speaker 3:Yeah, it's the very Christmas-y one, yeah very Christmas-y one, then maybe let me just Tell me what you want to start with this one.
Speaker 1:This one this one, this one, this one, this one, this one, this one.
Speaker 2:This one, the other ones I have no idea actually.
Speaker 1:The other one was a bit yeah, 50 Centish.
Speaker 4:Yeah, I could, it was extremely 50 Centish.
Speaker 2:That's actually what I was trying to input. I just Because I wanted to Well what I was trying to do. I thought it would be funny to take some song like 3D, you know, like rap or something like this, and then make it Christmas-y. So I tried to put it in, but it did not work. Okay. So that's why I think maybe this is one of the downed original.
Speaker 1:Oh wow, this looks like a.
Speaker 3:Dark Christmas.
Speaker 4:Yeah, it's a bit spooky.
Speaker 3:Yeah, but you see, but that's the thing.
Speaker 2:So, but this one I didn't put like. So there were two options you can pass in an audio file and then say in that a prompt, or you can just kind of request something. So this one I just requested, okay, but actually I think all the other ones.
Speaker 4:It's like building up to a suspense.
Speaker 3:Yeah, yeah, yeah so.
Speaker 1:I think it's one I have for you, but this is like a bunch of guys wearing bananas in, like a low rider in a Cadillac, like with hydraulic.
Speaker 2:The car that jumps. Yeah, it's how it's made Christmas in Brazil. That's listening to Christmas songs.
Speaker 3:No, I think you can even put that as kind of an intro to a Bruce Pinkstein song. Really, I think that could somehow work, yeah.
Speaker 2:Really yeah, but I think we can just regard the other ones.
Speaker 3:Okay, really.
Speaker 2:I think the other ones are actually the.
Speaker 3:That was the best one you should have built up, man.
Speaker 2:I'm actually wondering what I did, because I thought I downloaded a couple, but I think everything else is just the original songs. Actually An ancient song messed up. So yeah, apologize for that, okay.
Speaker 1:But this was a nice one, right. Yeah, it was okay, but like it was a bit.
Speaker 2:I will say Aggressive. I tried 50 Cent, I tried Snoop Dogg, I tried DMX, I tried Ritten.
Speaker 3:I feel, like all the stuff kind of sounded.
Speaker 2:No, I didn't try Metallica, but I tried to put there and I said make it more Christmasy. And then I was like okay, this didn't work. And then I actually went to Ched's PT and I say describe Christmas songs and it says, oh, it usually has a lot of bells, a lot of brass instruments and this and the festive. And I copy pasted the finish and I put it there. Still nothing. But I still feel like you have to be a bit descriptive.
Speaker 3:Yeah, because here you had bells and you had bass and I think that was kind of the main.
Speaker 2:Yeah but, I, think what else you need to for a Christmas song.
Speaker 3:But that's yours Okay.
Speaker 4:It's super happy, yeah, right.
Speaker 1:It's nice right.
Speaker 4:It's not bad. I can see like Reynolds with Sansa and his yeah, yeah, yeah she's not bad. It gives you the vibe.
Speaker 1:Give you another one Do it.
Speaker 2:This sounds more Christmasy if you.
Speaker 4:Yeah, I see Snoop, yeah, oh huh, not in Belgium, just raining.
Speaker 2:I have a last one.
Speaker 1:We have to go 10 degrees. Final one.
Speaker 4:That's a romantic.
Speaker 3:Say Kevin, say it for Kevin Go platform.
Speaker 2:All right, this is different shows. All right, what was your favorite?
Speaker 3:The first one, Pombard series.
Speaker 2:Really the organ. Yeah, what about you?
Speaker 4:I think the second one was good as well, but yours was in a different context.
Speaker 1:I think we just have to go further with that, I'll try, I think if I'm like, on Christmas day, go to wake up very early and drink like three or three espresso's, go to the gym, work out very hard.
Speaker 2:That's the second episode. Okay, so if it was at the gym just a full? Album afterwards All right, cool. Well, yeah, actually I think the audio. Actually I think audio is probably the trickiest one.
Speaker 2:I think generating audio is probably a very hard task, I think also music is mathematical True but I remember, for the AI song contest, the DeRuids, I think I remember looking into so many things like if you want to put lyrics and if you want to like the rhythm and the tone, and how do you represent these things. It's not sure I feel like it's more challenging, I guess. I think I'll have a harder time generating something with music than images or even video or text, I think.
Speaker 3:Question because we participated in the AI song contest for a few years in a row. Pre-gen AI True Would it not be a lot easier now.
Speaker 1:I think so Well, I would guess yes, you have tons of managed things that are way better than we just showed them. This is a free, open source. I think it would be easier. Youtube also announced that they're going all in on Gen AI Together. It's interesting to partner up with some specific artists that give their consent to use their art.
Speaker 4:I think they would do it very good because they have a lot of data.
Speaker 1:We do videos as well.
Speaker 4:I think they have a good scope.
Speaker 2:It's going to be interesting to see. We should maybe have a story and prepare something for music.
Speaker 4:This year maybe did we win no seriously. Yeah every year. Definitely go for it now, yeah.
Speaker 2:I don't know. It's going to be cool to have a look, but not everything is all rosy, I think. Job applications Do you have any thoughts on that part? Yeah, I see that side. It's been through stuff I've been through stuff.
Speaker 1:I'm going to be very honest In my position. It's been a while that I read a lot of motivation letters. We just recently opened a vacancy for a marketing intern.
Speaker 4:Oh.
Speaker 1:Very excited to have someone on board. If you're interested, check out careers of date Risaleo. But do not use chat GPT for your motivation letter. Everybody does it.
Speaker 3:Everybody does it, but then it has no value anymore.
Speaker 1:It has no value anymore, because you can have it. Everything looks the same. Everything looks the same. Everyone now has a striking personality. You have to constantly read these types of words. Immediately, it triggers. You didn't read your own, you didn't write yourself, yeah, but it's crazy.
Speaker 2:If you read a lot of motivation letters, it's very easy to tell. But maybe if someone is writing once and they're like, oh yeah, this is good enough, I don't think they'll tell.
Speaker 1:I have, from the point of view that the person that I'm writing is really good, because they do it once.
Speaker 2:They probably submit it a lot of places. But they're like oh yeah, this looks like a person did it.
Speaker 1:And they will think oh, striking personality. I guess I do.
Speaker 3:I think we'll also just need to evolve and stop asking motivation letters and ask something else they really do need to apply themselves.
Speaker 2:But even then it's a bit hard right. What if it's the alternative?
Speaker 3:I don't have the answer yet. We can ask that it's the same with take education, where a lot of the professors and stuff are complaining but everybody is using it to write their papers. But then maybe ask them to do something different, yeah, for a point. And criticize stuff that has been written, which is then maybe a different approach, and here maybe we just need to do the same and just take it as a given People will use it. But what can we do differently?
Speaker 4:to kind of Using it is not a problem. I think making it more personal because it's that you can use Chatchapiti to write your text better that's a good way to use it. But if someone is not start taking the time to make it something more personal and then submitting it, do we?
Speaker 3:want to spend the. It's very difficult to pick out. I mean, I've also read quite a few the last months and they just look all the same.
Speaker 4:But doesn't that mean that they're just that that person didn't spend the time enough to make it more personal?
Speaker 2:Yeah, but I'm even wondering, like you have the technical coding interviews, right? Oh, do a linked list, chatchapiti. What's the link links? Okay, that's it. But it really makes you wonder, right, like, what kind of exercise can you give?
Speaker 1:Well, yeah, and I think you can give an exercise, like, for example, from marketing I thought about this From a marketing interview. You can give an exercise. No wait, I'm going to say first of all, I think they're there. Why the motivation letter is there? Because we in the system of use is optional. You can select it whether you want the motivation letter or not. We did for the marketing intern and to me a marketing intern, like we do not have marketing team, I'm not sure what I should focus on. Interns are typically people that are still in school.
Speaker 1:So, they have very few resumes that truly jump out in terms of experiences. So you're also interested, like, realistically say, this person has very limited professional experience. You also think interested like what is the motivation? What is this person about? That's why I think motivation letter is interesting, if it's not said to generate. But what is the alternative to me is like that you ask like really an assignment, like create a visual of this, create a visual of that.
Speaker 3:Yeah, or we are looking for. Even in the motivational letter is read to our website or socials.
Speaker 4:True, exactly, and come with something your insights on our brand and what we could do differently.
Speaker 1:And that to me immediately is that I'm a bit hesitant about because it requires, like an investment from the person.
Speaker 3:Even from before, a letter also required an investment.
Speaker 2:And now it's just a prompt and you get it, and arguably it's less right Because you like you, could reuse most of the motivation Like if you write text.
Speaker 1:I have a bio right.
Speaker 2:I write it once. I can submit it different places.
Speaker 3:So I think it's like the level of investment is a bit but the job, because a lot of jobs require you to submit a portfolio or things like that, which also require you to apply yourself.
Speaker 4:But asking a motivation letter? I think you don't. If that person creates it via Jej-B-T, then you automatically don't want it because it's going to be, because marketing person is going to create your posts on LinkedIn. So if he's not or she's not taking her or his time, then you really it's a personality thing.
Speaker 2:Yeah, I think it's funny because it's like in the motivation letter the person shows how unmotivated they are.
Speaker 4:They just copy base it from Jej-B-T, because that's going to happen with your LinkedIn posts. Yeah.
Speaker 3:Plus, we want them to watch, to look at our website before they kind of commit to joining us. Or look at our socials to kind of have a better feel. Or am I going to feel?
Speaker 2:But indeed I think Mariam struck a good point that it's not like you don't want them to use it necessarily, right? Because if in the reality, in the job, someone asks, hey, can you write something? You could like, yeah, jej-b-t. I'm thinking also from the coding perspective, right, if you ask what's the link list, can you do this with the link list, whatever. And I use Jej-B-T and you say, oh, no, but that's cheating. I was like, oh, if I was on the job wouldn't the same thing. That's true. So it's like, maybe even the kind of skills that we were. The question we were asking is like, maybe outdated, maybe even right, exactly, and it's not cheating, it's fair games. That's what you would do on the job. And maybe the issue is not that you're using Jej-B-T, but the issue is that if you use Jej-B-T the way you're using for motivation letters and you do the same thing for LinkedIn posts or whatever posts, like as a marketing person, that's really bad, right, like there's no DNA, there's no uniqueness, right, you're just like a, you're a chatbot now.
Speaker 4:I think you are not looking for someone who can write very well. You're looking for someone who can see insights, who can work with those insights, create something that is like oh okay, that person looked at it and he knows or she knows. So you're not looking like he can write a good post.
Speaker 2:Yeah, indeed so, but I can imagine it's very frustrating.
Speaker 4:Yeah.
Speaker 2:Because it's like you open, like you're, oh, like 15 people applied, great, and then you started as a Jej-B-T.
Speaker 1:Do you have a formula and do you use Jej-B-T for in your communication?
Speaker 2:In my communication. Very rarely, I think, what I think I use more well. For example, one time I was preparing some slides and I needed to give a little blob about the technologies why I'm using, why I'm motivating the use of these things. So I just say, hey, Jej-B-T, generate 40 bullet points why you should use this, and then I pick like five and I rewrite it a bit Okay. So that's that, that's interesting.
Speaker 4:For me it's like the other way around. So what I do when I use Jej-B-T is like okay, I want to have to write about this and my thoughts are this, this, this, and I want to write about this concept as well. Can you write it into a text? So I provide with like, okay, this is what I want to write. Can you write it, the text for me? So I tried to give the context when I asked Jej-B-T.
Speaker 2:Yeah, I think, indeed I think, that's also. There are different ways you can use it. I think that's also a good one, but I think for me it doesn't replace me, in the sense that I kind of know what I'm looking for, right, like I know that if this is clear hallucinations, forget it, you know. Like I'm not using this as a like, as a place of myself, in a way.
Speaker 1:Yeah, yeah.
Speaker 2:What I'm trying to say is that it replaceable. Wow, ouch People hurt. Look at the time. Whoa, it's late.
Speaker 1:I saw, and sometimes they use it actually my communication. You're using your communication. Yeah, it was more, mostly when I'm Especially with you.
Speaker 2:Yeah, I was going to say it explains a lot. I was wondering why you keep saying you have a striking personality.
Speaker 1:No, it's not in direct messages, it's more like formal communication and in a combination formal communication and limited in time, because it really, really speeds up stuff. Yeah, and what I used to do is that I have that I say I want to write. So all the content typically comes from. But I say I want to write this and this and this and make some bullet points, make like a formal communication and distorted voice. You get a first output. Then you typically say like the next step is that I say make it a bit more level headed. No extraordinary words, no striking no accelerating.
Speaker 1:Yeah, get the next version. That's what I used to do, and then I rewrite a bit here and there. Now I have a chatbot you can create like your own chatbot. You can have like a pre-context. Yeah, and I actually injected some larger things that I wrote before, and then I say use this as a tone of voice to generate the input for the next thing, and that actually really helps.
Speaker 3:That really helps, that's crazy how easy it is to make your personal system.
Speaker 4:We can clone the art now.
Speaker 1:I enjoy writing a little bit, sometimes a bit as a hobby, and what I do there. I think it's a bit cheating.
Speaker 1:What I do now actually did in the last two weeks a bit is that you post in your text and you say Art comments to this text and you give an example how you got the comments. I do double dashes and then and then you art comments to the text on how you can improve the text. And that's actually very interesting because you don't get just a blob of text that is a rewritten version. That's very hard to work in, but you get like an outside opinion on your text. That to me, was very valid. It was like a peer writer kind of thing Interesting.
Speaker 2:Yeah, it's crazy, there's a me. Is there an app for this? I feel like there could be, like you write stuff and it just kind of gives you real time, almost like the word.
Speaker 1:What I used to use is Grammarly. Grammarly does this right?
Speaker 2:Yeah, I did, but I guess this is a Grammarly on steroids. It could do way more than that Serious Right.
Speaker 1:Like elephants level Starlight, exactly yeah.
Speaker 2:Yeah, but I think ChagPPT has a lot of good stuff. A lot of bad stuff Changed a bit the flavor, let's say Came across into this article from LinkedIn saying that ChagPPT is the best and worst thing to ever happen to AI. I thought it was interesting take. But his argument is that people say unprecedented pace of AI progress, but usually what they're saying is the level of applications in this realization and I think ever since this article was posted there were a few different research developments. But his argument is that the R&D, like the problems that we've had since the transformer architecture, which is what ChagPPT is hasn't actually improved that much. Like it kind of because the industrialization and the popularity and just having bigger models instead of trying to find better architectures and really truly break through in the research area. So on one hand, chagppt popularized a lot AI and GPT models but on the other hand, it kind of stopped true research or what they call true research.
Speaker 3:And I don't want to challenge that because it was. What was it two weeks ago, when somebody wrote an article also on kind of the next architecture?
Speaker 2:for models.
Speaker 3:So that I don't necessarily think it stops progress per se. It just amplified the adoption, that for sure I think we won't be talking about AI. I mean, every application now has an AI component to it, where even just a year ago half of them did not, so it's just become a lot more. Now everybody's joined the race. Nobody can afford not to.
Speaker 2:Yeah.
Speaker 1:But he's talking from an R&D point of view and that's more of a foundational research like that that has stopped and that the focus is not on the next architecture but more on how can we make it bigger and better and distributed.
Speaker 2:I think on one hand, so ChagPPT popularized AI. I think that's a pretty safe statement, but I think by popularizing it are we saying that more people are curious about AI and they're trying to improve it to the next level, like new architectures and really completely different things. So that's one path, happy path. Everyone's so bought in the idea that this is so popular that we're trying different things. And on the other hand, we can say, oh, this is where the money is. So all the research papers now are just trying to build a better model to beat a benchmark on. Maybe. Oh, now we have a new Dutch transformer model on this benchmark, Now we have one in Portuguese. You know, like I think you can go a bit both ways and sometimes at the same time, we've never seen this amount of money.
Speaker 1:That's true. And like isn't it also a bit like the question on ROI? From the moment that we see bigger models do not bring extra value? Like, the focus will probably be more on what is the next architecture, and there is a lot of money to do this now.
Speaker 2:Maybe we should have someone that is more like into research to discuss this, because sometimes I have the impression that ROI for research is publishing papers. And the easiest way to publish papers to say, oh, we have a new state of the art being this benchmark. Well, there's a whole nother. Yeah, it's a big statement on the state of research in AI, right, but sometimes like maybe another deep dive, yeah, yeah.
Speaker 2:But in Brazil, for example, I do hear that there's a lot of. They reproduce a lot of the stuff. They see some other places because it's kind of easy right, Like you see, oh, they have this transformers.
Speaker 1:You need four papers to get to PhD, right, I guess? Sorry, don't get smart.
Speaker 2:I said we're not getting canceled this year.
Speaker 4:We say these things. It's just one more podcast.
Speaker 2:We survived that Like the very end. We almost made it, but yeah, but I think I did think this article was published a while ago as well. Let me check the date three months ago. So indeed, I have seen the past month some new things here and there, and I don't know if it's also our perception, because we see a lot of the GPT stuff and maybe if we were more in the research maybe we would see more different things.
Speaker 4:But differences between AI product and AI research. I think the focus is a lot on AI products now and while the AI research is getting them, I think less funding and that's causing a problem. But in the end, if there are more products than someone has to do research as well, so maybe it will evaluate in a sense. That's okay, someone spent their time on that part as well.
Speaker 3:And we still see new things coming out on the current architecture. So, yeah, we haven't reached the limits of what we have now and I do suppose that under the radar people are still kind of developing the next architecture for when we do reach that limit, Because also all those commercial companies that are now vested in it they will need that next frontier. So our NDP teams are also looking into how can we push the limits six months from now when we do reach the max of what the current architecture could support.
Speaker 4:I think it doesn't make sense to stop the money to the AI research, because I think everything that is developing so quickly accelerates everything Like if you're not quick enough, maybe your algorithm is not going to. But maybe that's also the problem that you're not quick enough and that does bother other people, some people.
Speaker 2:Yeah, I think there's still a lot to be done, right? I mean, there's always more to be done. So I think, to be seen, I'm really curious. What's going to be the next really indispensable architecture, right? The transform architecture which is what we see everywhere, like the GPTs. It's not that new. It's like it's pre-open AI, jgpt boom, right. So I'm curious.
Speaker 4:There are a lot of companies who are spending lots of money with new rallying, for instance. They're also spending a lot on AI and making sure that we get to the human part as well. So I think there's a lot of things that are going on, maybe on a bigger scale, maybe smaller companies that we're working on got a bit side-drilled by them.
Speaker 2:Yeah, and I do think there's also research that is not, like you said, to productionalize, right. Like you mentioned as well the MISTROL they have different sizes of models, right. So I think now we have these huge things and we see how capable it is, but also can we make it more accessible for people? There's also a new research, right.
Speaker 3:And maybe it's gotten to a point where it's become so business-critical at some point that the next research is not going to come from research, it's not going to come from universities.
Speaker 2:True.
Speaker 3:It's not going to come from the R&D labs of big companies who are creating the next architecture or the next new disruption. But keep it commercial.
Speaker 2:Yeah.
Speaker 4:True, you might have to look at the profile of that person.
Speaker 2:Yeah, maybe, Maybe he's not there. I just saw that I was like well, that's an interesting thought.
Speaker 4:Maybe I should have discussed that Maybe it's the market or the sector that you're coming from.
Speaker 2:Could be. Could be All right changing gears a bit, Are we all okay if I take a more light topic?
Speaker 1:Go for it.
Speaker 2:So one thing. Another article I came across I thought it was interesting is why should you or anyone become an engineer manager?
Speaker 1:Yeah, Is it a light topic? I'm not sure.
Speaker 2:It's less, maybe not maybe a light, but it is what it is.
Speaker 3:Can you repeat? So why would you become an engineering manager? Why?
Speaker 2:should you or anyone become an engineer manager? So I think it's like a lot of the times for engineers they see the next step as becoming more of a manager right, which is less technical, is less like contributing to visually. But then you also see a lot of stories Maybe you don't see right, but that you read people that they were engineers, they were programmers and then they moved to a more managerial role and then they kind of regret it because they want to be technical and they don't want to this If you say engineering manager, you mean manager of a day that you could be.
Speaker 2:Yeah, you know you could be a software team, right? Yeah, I think the article goes on to say why is it important? You know the engineer manager, how a good engineer manager will improve the productivity and whatnot. But they also list some other reasons and I think for me I'm not an engineer manager necessarily, but I do my professional progression. I do see a lot of these benefits that they've mentioned here. So one blanket statement is that they say that being a good engineer manager will make you better at life and relationships.
Speaker 1:There's a lot of thoughts.
Speaker 2:I think you can say that about any profession, right Well, but I think you're self and anything but I think it's like, because they mentioned engineer manager, so I think this article is target towards software engineers and I think they're saying like well, if you're an engineer manager, you have to deal with people, and by dealing with people, you can better.
Speaker 1:That is the premise that you have to quote unquote manage people.
Speaker 2:I think, yeah, that's kind of like they didn't mention this explicitly, but I think that's the undertone right, like you're a manager, you're dealing with people, so, for example, so they drill down a bit right. So they say self regulation, right, like getting frustrated and like, regularly, yourself being aware of these things, being aware that you're being impatient, being an engineer manager put you in a position that you have to improve this. Okay, maybe do you agree with these things, do you think? Maybe just go one by one and I'll just ask how.
Speaker 1:Okay, let's go.
Speaker 2:Yeah, which one? Self-regulation. You think managing people makes you better at regulating yourself?
Speaker 1:Versus sitting behind your laptop all day coding. I think, yes, I think, say if you sit behind your laptop all day coding and you have your tickets assigned and that is the only thing that you focus on and it's very easy to say, to be non-constructive and not have any impact on you, okay, you can say fuck this, fuck that I don't understand why this is not working. I don't agree with this person. You can say all the same, it doesn't impact your related day job. From the moment that you are in a position where you quote unquote lead a team or manage a team or, together with a few people, needs to achieve a goal, you need to be conscious of these things, that you have an impact on other people.
Speaker 2:Yeah.
Speaker 1:And then you need to have with your team, you need to make sure that you go in a certain direction, and I think that makes it as a completely different perspective that you need to have on self-regulation, for example.
Speaker 2:And you felt that you feel like this is also something you relate to personally, or just like theoretically, like I can understand it personally.
Speaker 2:I think the second one is like self-awareness, which I think is very much linked right Like you gotta realize that you're patient in this and this, so I think this I'll just skip this one. They mentioned understanding other people, which I think to me, I think it's more like I understand that people are different and, for example, Bart, you're someone that is very direct. Maybe someone is not so direct, right? So the way that I'm gonna relate to you, I think that's I can relate to understand the other people, in this sense that people have different profiles and I don't wanna come across too strong to you, Maybe, Kevin, if you're not as direct as Bart, but some people can just be very direct and they still get the message and they won't be offended at all.
Speaker 3:Is this something that I and I think with him goes for the previous points. I agree and I think it's quite logical in a sense, but not by default. It's not the fact that you become a manager that by default you're gonna be attentive to. This person has a different communication style than I do, and so how do I adapt my communication to be more effective, in kind of hurting the group in a certain direction?
Speaker 3:It's, a good manager will gain those lessons and will kind of see those things. But it's not a given because you're a manager that suddenly you see those things. You need to be open-minded and willing to discover and willing to see those things, because you can just as well like you said, bart, when you said, yeah, as a coder, and then you're grumpy and you're making comments about stuff, you can very well just become a manager and keep doing the same and you're just gonna spiral down the motivation of the team because now you do have that influence and so it's not by definition becoming a manager that will give you those benefits If you're stepping into a manager hole with the right mindset kind of being in there and like I wanna learn how this works and I'm gonna do it to the fullest.
Speaker 3:then absolutely, I think you can learn.
Speaker 1:It's like maybe also because you are in a position that you're a manager. You're also in an environment that allows you to really grow in this and to see, like I grow in it and immediately see the effects of that as well.
Speaker 2:You're put in a position in which you can learn these things Like you're put through the experiences that can make you better, if you're willing. Yes.
Speaker 4:Now okay, just my so, because now we are saying okay, you can put into the situation where you can learn those things, but do we really think like a data engineer or a software engineer are not putting on those?
Speaker 1:No, no, it's not as extreme as that. Yeah, so, because it's like. But I think like if you look at date routes people that work as a data engineer they are consultants. Yeah, they're in different position. If you're within a company, if you're one of the thousands of data engineers in Facebook, then you're part of a very small, probably team where you have very clear assignment, like this is what you work on. Then you can be less conscious of these things and not having impact you.
Speaker 4:But then if you're, in that position, then the step that you take to become a manager is quite high, quite big, and I think it's becoming a manager. The step is never that high. You are learning it step by step and then at some point, okay, you are like okay, now I can be that person.
Speaker 3:But they're gonna give you that confidence if you already demonstrated in other ways. So during the stand-ups you're already kind of listening more to people and you have that attitude or kind of showing interest in more that side of the job as well. If you clearly demonstrate that you're not interested in it, then likely you're not gonna be granted a chance to.
Speaker 4:So it's not that if you were an engineer and suddenly now you became a manager, you have all the tests of it. Either you build it up and then you became, you developed over how you say it, you became a manager.
Speaker 2:But I still feel like, once you're putting that role, like there's a big, there's a change, like you can feel it more, like it becomes I think is what you're saying, or you said, kevin that this becomes a central part of your role to do a good job. This is your center around these things, whereas, like if you're an engineer that takes the lead on this, I feel like your job doesn't really depend on this. Like you're, it's more of a added, like an extra thing. Right, people are paying you to do programming, to program whatever, right, but I feel like as soon as you become a manager, then your main bread and butter becomes on how you relate to people, how you can understand them better, et cetera, et cetera. So I think there is a shift. There still Maybe another setting good boundaries, and that one's maybe a more debatable one. Do you feel like managers, they're better setting boundaries than it's happening in approval.
Speaker 2:I think so too right, but do you feel like, do you think you're exposed?
Speaker 1:more, You're probably exposed to more people asking questions.
Speaker 2:Yeah and you have to say like no, not gonna answer this now, it's best my time, or something. You feel like this happens more as a manager than a I think you're simply more exposed to these questions.
Speaker 1:I mean, maybe because of that, be more aware of it.
Speaker 3:But what you see a lot with young managers is they try to kind of imagine you're working on something and, as an individual developer, you're working on your part of the task and it might be a bit behind schedule. A lot of managers feel it as their personal responsibility to make sure that the total gets done, and so they will compliment for where some of the people fall back.
Speaker 3:And but if you do that left, right, right, left, left, right then suddenly where the team kind of goes through cycles, you just keep a flat line at the top of that cycle because you're complimenting for the drops in that cycle for everybody else, and so it's difficult at some point to say now my thing goes down, my contribution goes down, and then the risk is that the product or the release or the thing that my team is responsible for won't be there.
Speaker 1:I think that's the you feel personally liable for yeah, good point, yeah, good point, yeah, the result.
Speaker 2:Yeah, I agree Sensitivity, power dynamics. I think that what you're mentioning here is like because you're in a leadership role, you're sensitive that asking someone to do something, they may see this as not a kind request but something that they need to do.
Speaker 1:Okay.
Speaker 3:Yeah, I saw it more as being exposed also to politics.
Speaker 2:No, I think that's what they meant. I need to have a reread article in the past days, but it's like if you are leading the team and you say, hey, what do you think of this, they may feel pressured to say, oh, I like it because you're the boss. Do you feel like this is?
Speaker 4:That depends on your team. It depends on who you're in your Mariam, for example.
Speaker 3:She's going to say Kevin, no.
Speaker 1:No, but I think it depends also. Let's say, marilo becomes a team lead tomorrow, the colleagues from a team that you already know it's like cool, marilo got a promotion the day after someone new joins the team. To that person that you that didn't know Marilo before, marilo will be his boss. Yeah, that's true.
Speaker 4:And it also depends on your manager whether he or she has an open ear. Like, if you know okay he's not going to listen or he is just asking to ask yeah then I won't know when we'll manage it, but if you know, okay, he does something with your contribution. It's, I think it's a bit of two-way role. It depends on your manager, but also on your employees.
Speaker 2:Yeah, I agree. I think the last two here are hard conversations and the art of being on the same side, which I think it's kind of not necessarily related. I think hard conversations, I think it's easy to see why. But I also see I think this is also a good example from my personal experience as well how having to do this on my job because I do know this is part of the job, right, and I think it made it easier gave some experience to deal with in personal life as well.
Speaker 2:I like your notes and things need to be addressed, et cetera, et cetera. The art of being on the same side. It's more on, if you disagree with someone. I think it's to know how you can navigate this conversation in a way that you can make it clear that you're on the same side, right, Like you're on the same team, Like we disagree on how to do it, but we all want the same thing, which is also useful in personal relationships as well. Like, a lot of the times, it's not like you're 180 against each other, it's more like you have different ways of looking at it and it's fine and you're looking for common ground.
Speaker 1:Exactly right. To me, a lot of these things come down a bit to empathy, like trying to understand and be open to why people want to do something, what drives them, what motivates people and why don't they agree, like try to put yourself on someone else's shoes.
Speaker 2:I agree. These people right yeah, so I think that's kind of it. Maybe one thing that they mentioned, I think, say work is kind of the ideal sandbox for practicing life skills, because social contract is more explicit, and I thought it was also an interesting. I mean, I kind of agree, it was also an interesting way to put it. But it's just kind of like your work, it's work right, like I'm not.
Speaker 2:If I'm telling you this that you're not doing a good job, it's not because I'm a jerk, it's just because, as a company, we have certain standards, you know, and it's not me personally saying this to you, it's more like it's work. You shouldn't take it personally, right. And what they're trying to say here is that work creates this environment where you don't, you don't have to say things personally. You don't have, and you expect the person to take things personally as well. And that's what they mean by the social contract being more explicit. Do you agree with this? Do you think work is? Do you see a difference? Maybe Like, if you're a work, you have an easier time having hard conversations than in personal life, or setting boundaries or giving feedback, or something like this, or Because they did mention as well, like you can be your professional self at work. So it's almost like it's not Marillo.
Speaker 2:It's Marillo the tech lead, so maybe he's a jerk, but that's fine, it's not Marillo, you know.
Speaker 3:I agree and I don't agree. I think there's. Has my professional communication taught me things that I can apply at home? Absolutely Without a doubt? But the label or the mask you can put on at home, you cannot always put on in private situations. So you don't always have the same kind of like you say so the contract part, as between two people at work there's usually kind of a you do this and you do that, and so there's a role distribution and so this is what is expected of that role. If you're among friends, you don't necessarily have that kind of label. So if you need to have a difficult conversation with somebody, it's not out of title that you can have that conversation. You need to have it genuinely. And it's still a very different approach to having that difficult conversation than it would be under the umbrella of a specific title or a role that you take up at that point.
Speaker 2:Yeah, it's hard to say this not personal. Yeah, your personal life, exactly.
Speaker 4:But I really like what Kevin said, because in the end, it's like you are still working with people, right? You can't ignore that part. You can't be like, okay, you know what, I'm gonna be very professional and say I don't like you, but because you're still working with people and you have to take them into account and also we have to see how much, like we work eight hours per day, that's a big third part of your day.
Speaker 4:So you can't say I had to work as work, because work is not just work. I see more, sometimes more people on my job than I see my friends, I see my family. So you can't just say work is work.
Speaker 3:Yeah, I feel just like For me.
Speaker 4:I think for me, like when I chose Joe's a job, for me people is like the most important thing that I look for a job. If I can't match with people, I don't want to work there. So I don't know.
Speaker 2:I agree, I agree. I think this is a. It's not a hard line. Manio, I think you are put in different positions, right, like I think work kind of forces you to put in certain circumstances that maybe you wouldn't have to in your personal life. But that's it. I think we don't have any more time. I think some people have a hard stop at six, but maybe we have seven minutes. Do you think we have time to play a quote or no quote? Go for it, hit it. So I was the big winner last time.
Speaker 2:So this morning I wrapped up. This is, I hope we're all familiar with this.
Speaker 1:Maybe explain it.
Speaker 2:So, yeah, maybe it's a good idea. Thank you, bart, for keeping me honest. Do you know? Quote no, quote no, but I'm gonna explain it anyways.
Speaker 1:This is like a question, like did you listen to the previous episode?
Speaker 2:So here's the scenario we have GPT related model. We asked it to generate two fake quotes and then we have one real quote and then we try to guess. So it's almost like a Turing test. Is it Turing test, right?
Speaker 3:Yeah, it's true.
Speaker 2:We're trying to. It's almost like that, but with NLP, GPD or whatever. Mistro, Mistro, yeah. Generator quotes. Who I chose for this week is actually Rick from Rick and Morty, oh yeah so we're all familiar with Rick and Morty. No, okay, so it's a difficult one. It's a cartoon. I guess it's fine. You can still play with that.
Speaker 3:Well, maybe it won't be Anyway, I suppose.
Speaker 2:So here's the first quote. I don't like your unemployed genes, unemployed genes in my grandchildren, jerry. But life is made of little concessions Could be real. That's the first quote. Second quote Morty. In the great scheme of things we're just specks of dust arguing on a cosmic scale.
Speaker 1:That's number two. I think that is real.
Speaker 2:Number three weddings are basically funerals with cake. That's number three. So we have one real quote and we have two fake quotes.
Speaker 4:I've heard the third one before.
Speaker 2:You've heard the third one before, but you didn't know who Rick was. How did you?
Speaker 4:I know.
Speaker 2:She's like I just don't stop.
Speaker 4:I think I've heard the third one and I already liked it.
Speaker 2:Then you get the first pick. What do you think? What quote you think is the real quote?
Speaker 4:I think the third. Oh sorry, the fake quote there are two fakes no.
Speaker 3:No no there's two real ones. Two reals, one fake.
Speaker 4:Okay, I would say, wait the second one. It was hard to read for you.
Speaker 2:I'll read it again yeah Two fake one real. Number one I don't like your unemployed genes in my grandchildren, jerry, but life is made of little concessions. Second one Morty. In the great scheme of things, we're just specks of dust arguing on a cosmic scale. Number three weddings are basically funerals with cake.
Speaker 4:I want to say well, the first and the third are the real.
Speaker 2:So you're picking two as the fake one.
Speaker 4:No, so the second is the fake one.
Speaker 2:Second is the fake one. All right, bart seems a bit troubled, so I'll go for. Kevin.
Speaker 3:I'll say the first is the fake one. Just a, You're playing the spread.
Speaker 1:Yeah, I think the third. The second one is too politically correct you think the third one is too politically correct.
Speaker 2:Bart, you are incorrect. You have that. Sorry, mariam, you chose Morty. In the great scheme of things, we're just specks of dust arguing on a cosmic scale, and you are correct. Oh right, there you go, which makes you Kevin incorrect as well. Sorry about that. Oh.
Speaker 4:Yeah.
Speaker 2:I'm not sure it's still how it fits, but that means, mariam, you're the big winner of quote or not quote, so maybe.
Speaker 4:First time at podcast and first time.
Speaker 2:There we go. Yeah, so that means the next time you can bring the quote.
Speaker 4:That's a good idea.
Speaker 2:Yes, yes, yes, so you have to pick a person. Two real quotes, one fake quote. I think it's a bit. If you want to mix it up, I think that's also fine, but and I think that's it. We've got to call it a pot. Anyone has any other thoughts?
Speaker 1:We're going to do the last. I lost Kevin Aha.
Speaker 2:La platform, la platform, oh my God.
Speaker 1:Make it a bit lower and a bit slower, la platform. Wow, it knows that note. Have a merry Christmas everybody.
Speaker 2:Yes, that's true. I'll see you on 2024, in 2024. Actually, but we'll have an episode as part two of the Rootscom. Yes, next week, but we'll pre-record yes, but we'll see each other live in 2024. Cool Thanks y'all. Thank you for being here. Yes, thanks for your cooperation on the live stream. Still Vaxx.