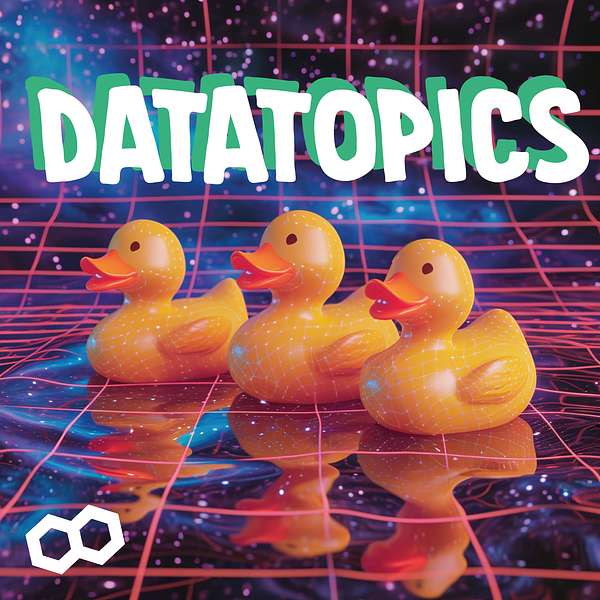
DataTopics Unplugged: All Things Data, AI & Tech
Welcome to the cozy corner of the tech world where ones and zeros mingle with casual chit-chat. Datatopics Unplugged is your go-to spot for relaxed discussions around tech, news, data, and society.
Dive into conversations that should flow as smoothly as your morning coffee (but don't), where industry insights meet laid-back banter. Whether you're a data aficionado or just someone curious about the digital age, pull up a chair, relax, and let's get into the heart of data, unplugged style!
DataTopics Unplugged: All Things Data, AI & Tech
Data Quality, Contracts and 100 Year Old Hares
Welcome to another engaging episode of Datatopics Unplugged, the podcast where tech and relaxation intersect. Today, we're excited to host two special guests, Paolo and Tim, who bring their unique perspectives to our cozy corner.
Guests of Today
- Paolo: An enthusiast of fantasy and sci-fi reading, Paolo is on a personal mission to reduce his coffee consumption. He has a unique way of measuring his height, at 0.89 Sams tall. With over two and a half years of experience as a data engineer at dataroots, Paolo contributes a rich professional perspective. His hobbies extend to playing field hockey and a preference for the warmer summer season.
- Tim: Occasionally known as Dr. Dunkenstein, Tim brings a mix of humor and insight. He measures his height at 0.87 Sams tall. As the Head of Bizdev, he prefers to steer clear of grand titles, revealing his views on hierarchical structures and monarchies.
Topics
Biz Corner:
- Kyutai: We delve into France's answer to OpenAI with Paolo Leonard, exploring the implications and future of Kyutai: https://techcrunch.com/2023/11/17/kyutai-is-an-french-ai-research-lab-with-a-330-million-budget-that-will-make-everything-open-source/
- GPT-NL: A discussion led by Bart Smeets on the Netherlands' own open language model and its potential impact: https://www.computerweekly.com/news/366558412/Netherlands-starts-building-its-own-AI-language-model
Tech Corner:
- Data Quality Insights: A blog post by Paolo on data quality vs. data validation. We'll explore when and why data quality is essential, and evaluate tools like dbt, soda, deequ, and great_expectations: https://dataroots.io/blog/state-of-data-quality-october-2023
- Soda Data Contracts: An overview of the newly released OSS Data Contract Engine by Soda. https://docs.soda.io/soda/data-contracts.html
Food for Thought Corner:
- Hare - A 100-Year Programming Language: Bart starts a discussion on the ambition of Hare to remain relevant for a century: https://harelang.org/blog/2023-11-08-100-year-language/.
Join us for this mix of expert insights and light-hearted moments. Whether you're deeply embedded in the tech world or just dipping your toes in, this episode promises to be both informative and entertaining!
And, yes. There is a voucher, go to dataroots.io and navigate to the shop (top right) and use voucher code murilos_bargain_blast for a 25EUR discount!
Yeah, and what part the coach play?
Tim:We're talking about the great couch in the show.
Murilo:Yeah, yeah, yeah, yeah, let's do it ready. Yep, Yellow, yellow. So welcome to the topics unplugged. So this is a casual I hearted, kinda bi-weekly I guess I'm not sure if I can say that anymore short discussion on what's new and data, from data quality to Programming languages, old programming languages, anything goes really. So today is the 17th of November, my name is Marilo and I'm joined with part hi. And who else we have here today?
Bart:Bart, we have two other people here. Introduce the first. All right, paolo here, hi Paolo, hey, hey, hello, hello. As data engineer at day trouts, he has difficulty stopping coffee. It's too much coffee.
Murilo:He's an addict. Basically, that's what you're saying. He's a druggy trying to recover. Come on man, be better.
Bart:Yeah, be for a summer of winter.
Murilo:Hmm, I can relate. I can relate, but a round of applause to Paolo.
Bart:Really? Oh, no, no one more. One more, we had someone Hi, yeah, last time. Yes, random facto it was. They was very tall, right.
Murilo:Yeah, I mean, people don't know my height because it's a podcast, but for me he was like average height, I guess.
Bart:Paolo is Roughly point eight, nine, some stall, just so you know oh, thanks for that information Welcome.
Paolo:Paolo, thanks, thanks for having me guys.
Murilo:And who else do we have here? We have Tim. Oh, hi, tim, hi there. So Tim is 0.87 Sam tall, just to keep the same scale. So everyone is on the same page here. What else can I say about Tim? Well, tim is a beloved, beloved date with colleague. He is so beloved, in fact, that Legend is that he's always sits on Bart's desk as a form of a mug, I guess, or picture, I'm not sure. I hope it's a mug, actually an actual picture.
Bart:It's a picture frame to picture.
Murilo:Wow, is it a frame kid? What is it? When is my picture coming, bart? Well, the next week will. Sometimes, tim is actually called, done, dr Duncan Stein. Wow, wow, that was difficult one.
Murilo:Yeah well, but I guess that's a basketball reference. I'm a. He's a very good basketball player, yes, and foot, foot, so player as well. Yeah, I'm dead. I know for first hand. Yeah, I'm very good foot so player. He is actually the, the head of business at the age of roots. So I mean, he was formerly known as king of business, but apparently he also doesn't like monarchy. So there you go. He was downgraded to to head of business. So round of applause, maybe All right. So what do we have today? Maybe we should start at the biz dev or not biz dev. Sorry, tim, the team of Tim effect the business corner. Maybe Qtai, am I saying this right, paul?
Paolo:Yeah, I think so. Never heard about the name. Hey, oh, anybody's saying it, so I'm assuming Qtai is correct. Yeah, qtai is actually France initiative to have Some kind of concurrent to open AI and they are Sorry, heavily investing into having a hub inside France in Paris I believe where they can then develop an open, open AI similar Company. Okay, which won't be for profit, which I think it's important in this case.
Murilo:Why, why? Why do you say that? Because open AI originally was not for profit, right?
Paolo:I don't think so.
Murilo:I think it went through the Y Q beta incubator, which is maybe I'm making it up.
Paolo:But I think they're very much for profit.
Murilo:Maybe they didn't make much profit in the beginning because I thought I saw something about Elon Musk on Twitter saying that when he created Open AI was supposed to be open and this and this and this, but then it was bought by Microsoft. So today he was kind of bashing like how, oh, this goes against why I Originally intended for open AI, but I'm not sure. But why? So you're saying nonprofit, I get, I mean, I think that they're obvious reasons, but could you just spill them out?
Paolo:Yeah, sure. So for me, why nonprofit is important is that you are actually trying to Get to a point where the result are more important than what your biggest customer is asking. So, for example, for Microsoft, they might want to get faster answers for your chatbot, for example, but at the cost of having Less accurate answers, and in that sense, I think having a nonprofit opens up more research possibilities, more Information. Let's say that can get into this. Yeah, in the things they are trying to build, rather than trying to get something that works fast and that works, yeah, maybe at the expense of Accuracy, like I said, yeah, I think also the nonprofit, I think.
Murilo:I Think it makes it more ethics friendly, right. That's true. I think that's also a big thing. But also, you mentioned, there's open AI, and I think this is very relevant. I think we are located in Europe, right, but I also think that Most of the the big LLM's and stuff, I think happens more in the US, which has different regulations, right. So I also think this is a big deal.
Paolo:I think too, it's a too young deal.
Bart:Interesting to know that you couldn't be involved as an advisor.
Murilo:That's really cool. That's really really cool. Maybe the big question is what's in the name? Right like, how do you sound in some French?
Paolo:No, but I think it's like cute must mean something in Japanese or Chinese, and then AI, which is cute, ai.
Murilo:Yeah, like cute AI yeah.
Bart:Maybe interesting, very similar, but at a much Smaller scale, because we're talking about was a budget for 300 million which is very significant, which is very significant.
Bart:The Dutch government is doing something similar. They are building a GPT and L I think they're calling it which the and their focus is also much more, much more specific on building a Dutch LLM being hosted by the government's also a bit of a Tactics to avoid any legal risk around copyright and all of these things that there's. That there's where there is currently a lot of weakness around, also the Risk of having no control on things from the moment that the civil servant is working on Documents internally that are not yet meant to do the for the public. Is it, then, a US entity that you want to process these things or these type of things? Go into consideration and They've budget if I remember correctly, 13 million and where they want to build an LLM from scratch For the source available and data available.
Bart:Oh this is interesting to see and their roadmap is roughly to have Something that is chat GPT 3.5 comparable in performance a year from now. That's interesting to see that we have different European countries, yeah, working on this.
Murilo:Maybe do you think this is the way to go, like each country kind of builds their own thing.
Paolo:No, I don't think it's the way to go. You need to see, I think I'm very pro Europe and I think you need to See those kind of initiatives on the European scale Rather than country by country, because then the budget would be yeah, that's way bigger, I just think.
Murilo:I just want to point out to the Because you are French speaking, yeah, and Bart is Dutch. So actually, when he was talking about the GPT, nl is like GPT modeling, right, like for Bart Dutch man. Moving on now, just kidding.
Tim:In the past when, when we had like the birds there, they have been Attempts made as well to create like a Dutch version of like birth. It's really good at these type of things.
Murilo:Actually that was a Robert. There was a Roberta. Yeah, actually was a Study with this guy, I had a project with him and he presented at one of the meetups in in hands About a year ago.
Tim:Yeah, and I remember, like I remember thinking like what is the relevance of all? Like how, how good can you train stuff like that? Because from what I am not an am the expert on this, but like GPT is, is training on a lot of like as many come, as much content as you can possibly gather, of which obviously, most likely a lot of it is coming from the internet, and I think the most common language on the internet right now still is English. Yeah, by far. I think there's not a lot of Dutch on the in the internet. Where do you get the content? Just to, just to, Listen to an interview.
Bart:That's the guy that's coordinating the GPT NL project and I think it was also realistic. Like their, their objective is not to make a super generic, super large-scale LM that can do anything, which is a bit what the Chet CPT opening is is trying to build. But they want to build something that is performant enough to do specific tasks and their Estimation of feasibility is that that that is possible?
Murilo:Okay, yeah, I'm not sure also how much, how far we can go with just fine tuning. I mean, I guess we can get into an interesting discussion on, because I guess pre-training is modeling language and I guess if you make the argument that Dutch and English, they share similar structure, they may be fine tuning makes sense.
Paolo:But I think, I don't know, maybe it's a too philosophical thing, but it's a very linguistic discussion and something we're getting into, I think and if you go like you add a layer and yet deep help, for example, which can do like okay, translation, translation, translation.
Murilo:You go from you, you ask your question in Dutch, it's translated in English, and then Ask your model in true, right like, just like a common, like a the C API for programming languages, right Like it's a common language that everyone speaks and then we just kind of know how to interact, traffic, yeah, but I guess. Yeah, I See what you're saying, but I also feel like every time you translate like if I were to speak in English, translating things from Portuguese there's a lot of stuff that gets lost, right like in the translation. So I'm wondering how, how, how this would work, right?
Paolo:Yeah, true, sometimes you lose a bit the context, which is important.
Murilo:Yeah, also things like there are things that if I say in Portuguese, it makes a lot of sense, yeah, but if I say in English or something else, it doesn't make a lot of sense, right. So I'm wondering how much you get lost in translation by that approach. But I see what you're saying. I mean, yeah, I'm wondering if that would be more effective than just training something from scratch. Another thought, because you mentioned that open data and Open source, available source, available source, yeah, I want to go into that again.
Murilo:I think we talked about maybe I don't know if it was last week or the week before that there were a few models that they had. They didn't, they weren't trained on, they were only trained on, not not proprietary data, I guess. So it's like. But there are some things, there are some references that you do need the proprietary things, right, like if you talk about a movie or if you talk about a character, if you only use Like I'm not sure, I'm not even sure if it's copyrighted, but think the example that we gave is like if you say, generate image about off a Mario Bros, right, if doesn't know what Mario Bros is, then Well, not sure what's it.
Bart:The point is that you're trying to maybe indeed.
Murilo:Yeah, I guess it's like they're still like I understand the intent, but there are still some limitations.
Bart:Well, like now you can do anything, but you need to be realistic. What you want to build, right like. If your intention is to build something, I can do anything a super generic LM like or gen AI model like, that's not, yeah, realistic, right like. Then, indeed, you need to scrape everything and say, and but they could took up a right.
Murilo:Yeah, yeah, but like is this? Can you like, should we be able to do this, I think also opening and maybe yeah, I think they got away with a lot of stuff because they were the first ones and everyone's kind of like oh, what's happening? You know, think every time, you know. So now, if you like, if someone tries to do that again, I think people are gonna be more aware, right? But I do feel like you. You lose a bit in a way.
Bart:Yeah, it puts them because it's doubtful to say that they will ever need to start from scratch again. Open AI so they, or they, or like they have a trade model with so much data, like they scraped everything. Yeah, it's crazy a bit of a In fair competitive advantage. Yeah, if new competitors in the future can't do it anymore, true, the other thing when we talk about Jenny I not necessarily alums is that you also have players that have a lot of data themselves who can do this like and I think Adobe is a very good example Adobe firefly true, they have their own, very performant.
Bart:Yeah, they are model purely based on the wrong data, know, and no copyright issues, I really know. And they, well, they have Adobe stock photos and stuff like. So they have a lot of a lot of input data. And there you have, actually that's the Jenny I model of firefly that it doesn't know super Mario Brothers, for example. So if you ask it to generate super Mario Brothers, it will still generate something, but like it's not super Mario, it'll be like a Mexican dude named Mario, his brother.
Murilo:But sorry, go ahead.
Tim:Rises to me this is. It raises an interesting question, do you feel like? Because a lot of these companies they like. The regulation on the AI and on data, I think, was less severe a couple of years ago and they gathered this huge amounts of data and now they're training.
Bart:I don't think the regulation was any different. I think if they would actually look at sorry, they would have thought about is it okay to scrape here the whole of shuttle stock without any license? Everybody would have said no, I like this, just did it.
Tim:The awareness on the regulation was less strict at that point is there.
Bart:Willingness to be aware, yeah, by open AI.
Tim:But since since now the awareness has just increased. I'm just doing it and and there's that, while these create like barriers to entry, we're playing.
Murilo:We're planning Tim to Train a little lemon real quick scripted.
Tim:Yeah, they killed my business ID starting here. No, but like you, you just create, like an Oli Kapoli, like the, just a situation which only these companies and it becomes impossible for any other company not a monarchy.
Bart:But even without this whole Data aspect, like it's still like you need so much resources to be able to even train something like this, like there, they are not ten entities out there that can do that.
Paolo:Huh that if the knowledge and the resource to do that yeah, yeah, but at the same time, I feel like there is a new LLM coming out every other week which is not really pre-trained by open AI. That just fitted some parameters.
Bart:But the major ones that we all think this is either. It's either Open AI, it's Facebook, it's on topic with shit, so loads of funding behind it. It's like it's yeah, but you also have like mistral.
Paolo:Now you have Falcon. You have like the one that Vitalia presented, the last.
Murilo:Lama, but that's this Facebook. All right, that's.
Paolo:Facebook. So it's already like yeah, five, six.
Murilo:LLMs yeah, but well, I'm not as in the LM game, but I still, from my standpoint, charge PT still best. Yeah, right, I could still Consider by far, yeah, exactly by far. So it's like, yeah, people are catching up, but there's still a considerable gap, right, and I think is open. I mean, either we're gonna saturate and then everyone's gonna catch up, or or open is gonna drop the ball and they're gonna catch up, or they're never gonna catch up.
Bart:And and the best is, it's very subjective, because if you look at all these benchmarks with where they try to Define performance of an LLM in numbers, yeah, it's not that easy to interpret like is this better or is this worse, or. But I think as an end user, like Interacting in a chat type of way, my experience with chat GPT is by far the best versus other.
Murilo:Yeah, I think it's a bit subjective, right? So even we say like how are you gonna test LLM outputs? It's different how you gonna do this, how you're gonna check data quality for LLMs. That was smooth, huh See, that's why I'm the host. I have some moments of you know. You know you can call him real, it's fine.
Bart:Okay, morillo, how could we talking data quality now? You bring this up so naturally. I know right what is data quality? Can we get to a definition?
Paolo:Well, that's a great question. I can define Please do no for me data quality it's the not metric, because it's not exactly metric, it's a. It's a way to I have notes to Start reading no, but it's really a way to see measure. Yeah, it can be measured how well your data can serve its purpose. So if you have big data set, big databases, and the data you have in those databases cannot be used, then your data quality is bad and you can actually measure this. We talk a lot about this. Data quality dimensions. Yeah, either you go on IBM, you have seven data quality dimensions or you go on colibrius 6, but those dimensions you can use them to actually see the health of your data, your data quality.
Murilo:Could you give me some hypothetical examples to illustrate that?
Paolo:Yeah, sure, so we can discuss about the dimension. Actually, that one big one is Validity of your data, basically. Okay, could you elaborate a bit more? Yeah, sure, so that that's the simplest one. For example, you have in your database an entry that says more illo is born on the first of April 1993. Not true, but then you expect. Issue Wrong, but then you expect, but that's actually linked to another issue in another dimension, but let's focus on that one. Okay, you expect them that the format of your, your birth date, is to one of fifth Force what means fifth?
Murilo:That's not my birthday, yes.
Paolo:The first of like, oh, one of four, 1993, for example. That's the formatting you don't expect to have 1993 first of April, for example in the language.
Murilo:So that's kind of the one of the issue you can encounter and maybe so like tools like pydentic Address that so by dentic is a Python tool. It's kind of popular, but if you don't know, it's like a Python tool that will validate JSON. But I think it's kind of the same thing you're saying, correct.
Paolo:Yes, yeah, actually when we, when you put by dentic, it's actually checking some part of data quality, but not Everything you can use by denting. I think it's a. It's a great tool. I looked as well.
Murilo:And the pandera, which is specific to pandas, right, yeah, so pandera is basically by dentic for tabular data. So it's basically a way you can check the columns, make sure that they have the correct types and if they don't have the right types, you can enforce that.
Paolo:Yeah but then this can be related to certain dimensions of validity, accuracy as well. So accuracy is how it relates to the actual data. So you are not born on the first of April 9th in a tree, then there is there are way to find out you so we can look at the maybe a side note.
Murilo:I was born on the 1st of September. So when he said the first off, I was like he knows, like His homework, like oh wow, it's a 94 and I was born 95. I was like that was pretty close. And so you're 95. Yeah, I'm 95. Well, why look, all I was, I'm dead. It's the eight the years. In Brazil they really ate.
Bart:Yeah, I'm sorry you got a beard when you say to result.
Murilo:Yeah.
Tim:It lived through some shit. I Imagine like eight-year-old Mojila right now like sitting on the porch, like with like a cigar.
Bart:They take quality and trying to Like. So so you have, sir. You have data, you data, you have a data set in whatever shape or form. You have certain expectations of how that data looks like or how accurate it is, or, and Data quality or data quality issues are that that's expectations are not matter.
Paolo:Yeah, that's a good definition. It can also be related to when your data is delivered Mm-hmm, or if the data is still how timely it is, exactly expectations on timeliness, which may not be. Exactly.
Tim:I think I think it's funny because, like in the con, in conversations that I have in a day-to-day with like, with like customers, like data quality comes up very often, but it's always about like the validity. It's always about like we have. We have no values, we have missing values, all that type of things.
Paolo:It's not validity.
Tim:It's, it's completeness, but like it's it's. It's where where Timeliness? People don't think of timeliness as being something that is true. I feel like if you don't think about it, if you don't have problems, I'm talking about like, like, like the conversations that I have with, like the people that I speak to on a day-to-day basis.
Bart:Data engineers, not machine engineers. But when do data quality issues pop up when you try to what?
Tim:is the cause of data quality when you yeah, when you try what people?
Paolo:because when you humans see them.
Bart:Because the cause is. Well, let's start with. When do we see them?
Paolo:Basically, when you're building dashboards or when you're trying to, you have to do a poor bi Dashboard to show like the result of your company and you see that you're missing a hundred K somewhere and you think I wait, should be higher, right you should miss more, or what do you mean?
Murilo:I don't know. We have so much money, yeah.
Paolo:And that's the type of issue you can have a look at and see first time. Okay, this is an issue, but then, in the same way, a Data analyst which is using a data set that was built for by another team, for example, they might see a lot of null values during their exploration, and even before they can do a dashboard or can do some analysis that they can present to someone else, they are encountering those data quality issues another example that I've encountered is the timestamps poof.
Murilo:Timestamps are there's time zones, there's daylight saving. Sometimes different databases have different Unix timestamps. One is milliseconds of this and then I don't know where we're like oh yeah, this, this customer is from 1972.
Bart:It's like you know, I think that's, and it's always like yeah, and then every time I see this Like because, usually, I found it is the danger is, of course, that you do not notice this right and that you have data and you make decisions based on this data yeah, manual, automated ones based on wrong data yeah, and you make the wrong decisions, and I think it's also tricky because it's it's hard to prove that it's a hundred percent correct, but it's very easy to prove that is wrong.
Murilo:I Right, like if you don't see issues, that doesn't mean it's correct. Yeah right, but if you see an issue, then you know for sure it's wrong. So every time you can say I think it's correct, but you can never say I know it's correct for sure. Is this a fair statement? Maybe with data quality tools you can do it.
Paolo:Yeah well, data quality you can do as much as a data quality engineer, let's say, in a company that you know and nothing about. So you can know, for example, that the formatting of certain things should be like this. But then the business knowledge that certain people who have been working at this company that, for example, might know that this record is correct or incorrect for sure, this is the knowledge that is needed to have correct data quality.
Bart:Really the main knowledge.
Paolo:To understand.
Bart:what are the expectations to this and where do these issues come from?
Paolo:That's yeah, they come from everything. Let's say, you have some forms that you need filling and you didn't think that. You thought, okay, everybody would fill male, female and then you didn't think of X.
Bart:Controversial.
Paolo:Yeah, you didn't think of any other gender that was possible. And people start filling those gender because this is a free from text, for example. It can also be like just an issue in the code. So you're processing data that you received and you thought, ah well, during my analysis I saw that this data would be between 10 and 15, and you're taking this assumption with you during your programming the new pipelines, for example, and then this issue once you have like a value over 15, then the issue will replicate.
Tim:Replicates.
Paolo:Replicates, then to the next step.
Murilo:Or for the timestamps thing, the issue that I had was because it was different databases and they had different epoch timestamps, I think, because I think it's like so it's the developer's fault. So it's the person right.
Bart:I think time zones is a very good example. When I build something and time zones are involved, then I'm like, oh shit, okay, but it runs here, let's just deploy it, see if issues problem down the line. Yeah.
Murilo:And the data quality issues come from. Yeah, and they like saving too. It's tricky, yeah. Yeah, they like saving, it's tricky, yeah.
Tim:Big corporates that try to roll out like solutions across the world really love data engineers like you have Just deploy.
Murilo:It's working on my machine. It works here.
Bart:Yeah, but when it's for a customer, I'm always very intelligent.
Paolo:I tested everything.
Bart:Nice, Actually not that easy to test. Huh, Time zone implications are not that easy to test because you test on a local machine. Just take a plane.
Murilo:What if you're international waters?
Tim:What if I crossed the timeline?
Murilo:Yeah, yeah, At least a couple of places Maybe. So you mentioned the dimensions. You said six or seven.
Paolo:I think, yeah, between six I four to six to seven.
Murilo:It depends really on who's saying so, you mentioned validity, completeness, timeliness, timeliness accuracy, accuracy.
Paolo:You have consistency. What is consistency? Which is a I don't know. Consistency is how consistent you are inside a data set or in between two data sets. So, for example easy example you say US in every record, but at some point you say USA, usa, yeah, okay. And in between, also in between data sets, or in one data set, you are known as Murillo, who is born on the 1st of September 1995. Correct, yeah, so that's consistency. I see, I see, I see.
Tim:But it's also validity, because the value in the second one is not correct.
Murilo:Yeah, yeah, so you have validity or accuracy, accuracy, sorry.
Tim:I was paying attention. I was trying.
Paolo:No, but in the but. Consistency is between data set, Not looking at the ground truth, the ground truth, and then you have the accuracy, which is corrected to the ground truth.
Tim:Should we explain ground truth? Go for it. No, I'm not going. You're the machinery engineer.
Murilo:I guess ground truth is the actual yeah, the actual value, the factual truth.
Paolo:Yeah, right, then we're talking about birth date. You can have like verify this if you look at like birth certificate and then for you have numerical values. The values can be between five and 10 and zero. That's your ground truth. Okay.
Tim:Are there like data quality in situations where ground truth is explicit and known? I think that's the easier type of situation.
Murilo:Yeah.
Tim:What do you do when there is no ground truth?
Bart:Do you have an example? Can you make it a bit more?
Tim:We were talking about LLMs before right, and I think in some of the situations it's possible that you don't have a ground truth for a specific answer If you ask an LLM to come up with a hypothetical and you want sort of to have a metric of the quality of the answer that you get. I'm not sure if I'm staying in the realm of data quality here.
Bart:This is philosophy, but okay.
Tim:I think because you said consistency, which can still remain valid in case there is no ground truth.
Murilo:I think there are some others where but I think it's like in general, if you have a machine learning use case or AI use case, that you make a prediction and it's a data point, but you don't know if it's correct. That's kind of the thing, just right, yeah, customer segmentation.
Tim:Clustering, there's usually no ground truth.
Paolo:Oh, like this.
Bart:It's maybe something different, but then you need domain knowledge, right, yeah, someone that makes a conclusion about a cluster. From that moment on, that becomes your own.
Tim:Yeah, that's true. Okay, so then we assume All right.
Murilo:Well, but I think from what Paul was saying, there is also different dimensions of this. Right, like you can still check for timeliness, this is still there when I expected it to be there. Do we have a lot of nulls, maybe? Yeah, you expect the clusters to be somewhat distributed, but everything goes into one cluster. I think there's still some checks you can do, right.
Bart:Maybe to out to Timber saying I think from the moment, the definition of what is correct here is a bit vague and you need a lot of domain knowledge. Out on top of that's a process where 10 entities down the line are doing something with this data. Like there is a high risk that you create a data quality issue somewhere down the line, right?
Paolo:Like. Most likely indeed, but then that's when you need this business knowledge to counteract a bit on this prediction.
Bart:Business knowledge, but we also have tools. Actually, Paul released a blog post on the state of data quality as of November 2023. Can we get an applause there too? You are?
Tim:so confident.
Paolo:I was going to start again.
Murilo:Indeed. Indeed, You're going to plug that in in the show notes for sure.
Bart:We are going to plug that in the show notes, but I'm very curious to see what the state of data quality is.
Paolo:Well, it's not state of data logory per se, it's state of the data quality tools. So in this article and I worked with a few colleagues in data routes, so we compared the four open source data quality tools that were production ready we compared them on the infrastructure compatibility. So how well can you orchestrate them, how well can you use them in your current data stack? We also compared them on the feature they had and how easy is it to use them, how well the connection to the modern data warehouse also is possible. And then lastly that's a bit on top of everything, because how well the reporting and dashboarding is present, because a lot of times the third point might feel like a feature that is not necessary when you think about data quality tools. But then if you cannot have access to the result, or if the result are presented in a way that you don't want to have to look at them, then it's an issue. Basically because you can have as much data quality as you want, but if you don't use it, it's never going to help you.
Murilo:And do you have any? If you mentioned, you compared some tools. What are the tools that you can? Maybe don't need to go through all of them, but any highlights or something that like. Are there a lot of players in the space? Are there a few players in the space? What would you personally recommend?
Paolo:Yeah, so we there were two big tools, python based, great expectation and so on.
Murilo:You mentioned Python based. Why is this? Oh, wasn't expecting that you mentioned Python based. So they're written in Python, yeah, but why is this relevant? Why did you have to mention this? For example?
Paolo:Yeah, because if it's written in Python, for me it's way easier for people to adopt those tools and then add new features to it. So since those are open source tools, it's important that they are written in a language that can be used by a lot of people and extended then.
Murilo:Yeah, and the little. The data language today, the fact of standard is Python. Yeah, Right, like it or not.
Paolo:Yeah, indeed. And then, for example, with great expectation, we had to write some custom, some custom check, and then that was possible because it was in Python, not that we couldn't do it in another language, but then the speed development is increased, of course.
Murilo:Yeah, Sorry, I mean you were saying so. You mentioned soda, great expectations.
Paolo:We also tested dbt testing. Dbt test the feature that is available in dbt. And then the last one was YLUX. Ylux was to be fair, it wasn't completely up to standards compared to the others. Okay, but why? I think it was a problem of a casting problem, let's say Casting problem. Yeah, please elaborate. Yeah, sure, I mean they offer data quality services, let's say, but that's not their main selling point Okay.
Paolo:So I think they are rather big on the image segmentation or image data quality testing, which is something else. Yeah, so then you could do stuff. Okay, by the way, yesterday we talked about DQ with Mozilla. They have this constraint constraint how do you say constraint notion to build data quality rules? That?
Paolo:is for me a bit outdated because you need to program to have this, to have your data quality rules. And if you compare this to great expectation of Soda, you have like a YAML file, a JSON file that you can just write your rules in there, connect it to the database and then you can just go up and running. And this way you have like a business user data analyst who are not really strong with Python but know enough of SQL, or like the Czech language of Soda or the language of great expectation to start building data quality rules.
Tim:Okay.
Murilo:Cool. So, from what I hear, soda and great expectations are.
Paolo:Yeah, it was a bit favor in Soda not a bit, but a great expectation. I think it's a great tool. It helped us a lot, but I think with Soda it's going forward in terms of ease of use and connection to existing data warehouse. And also, if you look at the integration with data catalogs or reporting tools, it's way easier to do that with Soda than with great expectation.
Murilo:Okay and Soda. If I understand well, he runs queries on your engine. If you have a database, it would take. You don't need another cluster or something like that, it just runs on top of it. Pretty cool, maybe.
Bart:So I think we talked a lot about data quality, but yeah, so you're just trying to make a segue to something else, huh?
Murilo:No, no, no.
Bart:Just a sub question. So let's say you're using one of these. Let's take Soda, right, you're using this. You're using this as a developer, you're a data engineer, so you're probably making defining expectations. So it's called in Soda. No checks, Checks sorry, Great expectations, Checks. You're making checks in Soda. Who are other users of the system? How does it work? Like from the moment that there is an quote-unquote issue, there's a data quality issue? How does it avoid that there are data quality issues?
Paolo:I'm very happy you asked the question, because I saw Moreno switching to another topic and I was like wait, wait, wait.
Tim:There is one.
Paolo:This is tipping those we are keeping him in check.
Bart:Oh yeah, Sorry.
Paolo:Do-do-ch.
Murilo:Good job First time. We use it right, if you ask. Less confidence we need tags on the buttons. Yeah, really.
Bart:I have like with the buttons, but there's no names on them. So on my screen there are the names within the order of the buttons. So I have to first look at my screen. Oh yeah, there it is.
Murilo:And by the time you find it it's too late. It's like we want another topic. We really change the topic anyways.
Bart:But how does an organization actually use these tools to make sure that there are no questions?
Paolo:Basically implementing the tool for me is really the easy part, like it's not complex, you can do it in a let's not say a week, but like a month or something.
Paolo:Yeah, you have the tool and you know how to integrate this with the data stack that they have.
Paolo:But then you have to have data governance. You don't need to have like big data governance with data catalogs, with data owner, cdo and chief data officer, for example, just having someone that is responsible for the tool so technically, how to integrate the tool into our team and let's call it a data steward, who is just checking the incident that the tool is generating, the data issues that we're having and that can handle them. And then next to that you can also have the data steward can also be the person who has the domain knowledge to build actually those checks. And that's really interesting. That, referring back to the constrained example I had with DQ and YLOX, is that these people don't really know how to program, be it in Python, scala or anything. So having a JSON or a ML file, that's really easy for them. They can just go and say okay, I know that to check for null value, I need to write check for null value equals 0. So that's really simple for them.
Tim:You still need to do YAML definitions.
Murilo:I get the syntax right.
Tim:It's not that simple.
Bart:True, that iterative process of defining checks or expectations, getting notified, incident reports, understanding where the incident comes from, fixing that becomes a very iterative and probably, in a complex system, a continuous process on making sure that you reach some level of quality.
Paolo:To me when you talk about data quality, you are talking about measuring how your data is going and how well it is. But then when you start talking about data quality framework, then this is encompassing everything Like the data governance, then the data quality, the data health of your data, of course. And once you have this data quality framework, you can pull out some results. But if you just focus on the tooling or if you just focus on the data governance, then you won't have the result you expect.
Tim:There's a bit of a parallel with developing something and then operating a typical software lifecycle developing something, operating something like developing something is just you and the code and it needs to work, and then operating something, there's usually a lot more responsibilities and the governance around it. For the development, there's obviously the entire movement of DevOps and whatever comes in making sure that during the development you can also operate it For data, these types of solutions. So that great expectations I think great expectations integrating into a development workflow of a data engineer is pretty clear. How does that work for us? So I don't know. As a data engineer, when you're developing a data pipeline, data products, how do you, from the development, already integrate this into your?
Murilo:solution, or maybe even should it be integrated in the development phase or is it after? Should it be the same people that are building the pipeline to build these checks, or should it be someone that has domain knowledge, or should it be both?
Paolo:I think, in the end, it's the person who has the domain knowledge that asked to build the pipeline, so it comes back to them to define what they expect of this. In the end, it's the developer. Sometimes they have this list of okay, you're reading sources and then you're doing some joins and then you're doing this, but it doesn't go beyond this. It's just like, okay, I'm building this, I'm making it as fast as I can, and then that's it, I'm putting it in production. Right, in the end, that's the business owner. That business owner, the person who has the domain knowledge.
Paolo:Yeah, you can call it a Stuart.
Bart:But I would argue that maybe if the developer that creates something is not also making checks, that if you have a culture around data quality or data governance, that the developer that is building something does use good hygiene around this, that you use schema validation, something like by dente type checking whatever right that you think about this also when you're developing something.
Murilo:I think, yeah, data quality after this discussion, is like broad right. I think the validity part is something that developers should be thinking, because otherwise this stuff you're building will break a lot. Or, like you know, it's much easier to validate it from the beginning, so you know exactly what the inputs are and everything. But I think when you talk about accuracy or consistency, it's a bit harder, right, and I think if we didn't say data quality, if we were breaking it down to the different dimensions, I think it would be easier to say, yeah, this is probably something that developers should care. This is not, I mean, maybe even consistency right, and all your inputs need to have three categories. You know, and you want that always to be the case, so you know, like, but correctness, accuracy, it's a bit you know. Should they do it, can they do it? Is this something that would change over time as well? I think it intertwines a bit.
Paolo:Yeah, I think you should be careful about having by dente next to something like soda, because in the end you duplicate the places where you store your data quality rules. And I mean it's good that the developer thinks about okay, this can only be like this and then that's fine, you add this pain and check, let's say. But then there's a bit of duplication with, like, what the data stores would come up as rules for the data set he managed or designed.
Tim:So I think, it's.
Paolo:There's a bit of a pros and cons situation here.
Murilo:There may be a question how often do we see organizations, companies, teams really investing in the data quality that is not validation, let's say Like because of the stuff that will be outside of the developers reach or the developers responsibilities, more the correctness, the consistency, all these things?
Bart:I am, I'd argue to say, if it has an impact on the bottom line, on, let's say, a service that they're building, putting in a product, is extremely dependent on their data quality. Or in a regulated industry, like, let's say, in the finance industry, where there are a lot of regulations that you need to comply to, where correct data, where things like validity if you make decisions based on data are are regulated and you are forced to pay attention to this.
Bart:Yeah, if it doesn't have an impact, like you're not going to care, right, like with so many things in life.
Murilo:Yeah, I think it's like usually there is an impact, but like because I hear a lot about data quality but then when I actually look to see what people are doing, I don't see that as much as I hear, at least. But it could be my personal experience, but that's what I was asking, so it's like, I think, for me it depends a bit.
Paolo:I think for bigger organizations it's way more important than for some organizations, I would say.
Murilo:And that's kind of what I wanted to maybe get to is like with points. Which point do we say we really need to invest? Double down on this. It's like, I guess, when we start noticing a lot of issues, is it when we start acquiring and we have different sources, or something Like I think there's a proportionality, like.
Tim:I think what I see is that this isn't it's a topic that you see both with small companies and big companies, at least from the moment that your data team putting like the airports right now, the moment that your data team becomes, you know, too big, so that it's not just like you're not fully aware of what everyone is doing, like you have at least a little bit of a data team, at that point the question already arises. But the solutions that you discussed I think Soda and Great Expectations it's. There's a learning curve involved, there's an investment that you have to make to do these type of things and for really small companies, it just doesn't make sense to have this type of scale of data quality solution or need as well.
Bart:Right, like if you look at like let's make an analogy you have your one two person developer team. You have a minimal application running somewhere. You have an issue. You're just going to look at the log of that direct application. You're going to see what the issue is from the moment that you have a team with hundreds of services running. You need something like Datalog to manage that. That's true.
Tim:And there as well, like there's a proportionality in the solution. Like I think DBT tests, for example, like for even a lot of small companies are using DBT right now. So DBT tests, it's pretty much there, right, it's a minimal implementation effort. So there, we see that a lot and it's one of the best practices they actually preach a lot as well.
Bart:Yeah, we use it internally as well, dbt test. Well, maybe a last question and then take your time.
Murilo:I like how he's asking me for the ref. I'm empowered, you are. Yeah, it's like, yes, carry on.
Bart:If you would purely answer from your own personal experience preference and I give you two scenarios and you would say it's this data quality tool that I would use. Let's say I'm a tech startup building a product, a small mobile application, and data quality is essential for me. What type of which of the four you mentioned four in your in your blog? Great expectation. So the DBT. Why are you actually mentioned DQ just now? The other scenario I'm a big corporate bank with a few million of customers. Which one would you go for?
Paolo:Depends on the startup tech. Let's say, if you're using DBT, I think it makes complete sense to just go with DBT test, because it's already there. Dbt is there, you can just go with DBT test and then, if you need more, then you can expand with elementary or red data, for example. If you're a big company, I think you have all the needs, all the needs like, for example, that soda can fill with their cloud platform. Let's say, where you can have reporting and logs as well. So I would go, rather than with soda, that can offer you full support if something goes wrong, rather than hoping that DBT test filled your field of need.
Tim:Cool. Do they play well together? Dbt test soda or DBT test great expectations, like because are they friends.
Paolo:Are they friends? I can't say for this expectation DBT, but I know that DBT and soda soda has integration with DBT, but the pity is is that it doesn't play on the strength of soda. So basically, you would use DBT, test that would build artifact for you, and then you load those artifacts with soda, which is then an extra step rather than just reading from DBT test.
Murilo:But on the other hand too, soda has plus that it plugs into different tools right. So if you say now, soda is the data quality to form organization. But I have a team that are like but we're doing everything on DBT we want to do, want to change, and it's like, yeah, okay, but just plug it in so we have the central governance.
Tim:Interesting.
Paolo:So that's yeah. That's really nice that they have this in just tool directly from DBT.
Murilo:Cool. Maybe one last thing on data quality data quality for AI, so you linked up something here.
Paolo:Yeah, yeah, since we were discussing data quality, I thought we were always talking about data quality for datasets, you know, databases, but then I think AI is also. With the regulation that we have coming in, I think with you discussed this last week as well, with Mr Kevin Maybe but then there is the AI regulation that are coming in, you are coming to a point where data quality for AI needs to be developed as well, in the sense that, with your LLM, for example, you need to see to check whether your LLM will throughout resource flows, for example, okay, and then with, for example, the tool I linked, so this card, you can check those kind of things so you can see if your LLM will will hallucinate if your chatbot will not stop answering the question you fine tune him for.
Bart:So it's really like a unit testing framework for our labs. It's an interesting development.
Murilo:Yeah, I think it kind of links back to what we were discussing before. Right, like if LLM produces something, how can we objectively measure that right? Like we don't have ground truth. And it's like yeah, bart, you can tell me charge it is better, but I can say well, I don't think so, and maybe we're both right. You know, maybe we're using the tool differently as well, so it's more subjective.
Tim:So maybe I don't know which one of you more LLM is going to be able to answer this the best, but how often in practice do you see like data contracts is something that's coming up a lot right? Soda has something for it. Recently read AI model contracts. Does does like machine learning model contracts? Does that exist? Does it?
Paolo:happen a lot. What I was going to ask is it time for having like data governance, like we have with data set for AI?
Murilo:models. Could you elaborate on what you mean by data?
Bart:model. How will this look like?
Tim:I'm actually very like it's it's. It's an open question, but I have no idea what the industry standard is on this. But maybe like a data, I think a data contract.
Murilo:Yes, could you explain what it is?
Tim:Data contract yes, for people that I'm going to look towards the data quality.
Murilo:All right, he brings it up and he's like Pablo.
Tim:No, no, no, like I'm, I'm all right, I'll take it up. I'm going to look towards the data quality expert.
Paolo:But I don't want to take up Bart's topic, so you know, go ahead with it.
Bart:It's a very. It's a relevant topic because Soda announced it yesterday.
Paolo:What to say yeah, day before yesterday, I think so.
Bart:What is the data contract?
Paolo:Data contract is basically an agreement between two stakeholders who, one producer and one what to call it user, consumer, indeed, of what should be in this data set. So it can. Then how Soda is building this is that they have a schema. So basically, you have the schema of what column you expect, the type. You can have some SLA, what's in the data contract varies, of course.
Murilo:What do you want to say SLA? What do you mean? Service level agreement?
Paolo:But it's like the data will be updated every X days, exactly, okay, so to check on the timeliness of the data quality, for example. So you have different SLA, so called a bronze, depends on the criticality of the data, and then you also have like the owner and who is using it as well.
Murilo:So basically, it's like an agreement on who's producing the data, who's consuming the data and what would be like. The data is a table in this case, and what would the table look like?
Paolo:So, columns types is there something else? You can have basically everything. You can have data quality as well, so that the consumer knows okay, the person is checking this, this and this and this. So if I have something like this, then the agreement is broken.
Murilo:Okay, cool, and the producer, consumer is also listed in the contract, does?
Tim:it.
Tim:I know that dbt also has a notion of data contracts that you have in there and it's like it's like, basically, there's some like it's with dbt test and you can have some metrics.
Tim:But you can also do the versioning of your, of your contract, where where, for example, if you have a consumer and I'm going out of my depth here, if some would be here it would be but, like you can, you can version your data contract so that, for example, you have a pipeline running and somebody is consuming this, you can basically say, okay, this is, this is going to be deprecated.
Tim:From that point on, like there's a new version, you should update to this new version of the data pipeline and you give people like a grace period in which they, like the consumer, has some time to make the changes to the product, like whatever is consuming the data. And then you know, with the new version, like the new version, the old version will not be here anymore by then. By then you need to make the change and if you haven't done that, well, your pipeline is mostly going to break, and so stuff like that is is what I think is super interesting, especially in like a very big corporation where you have highly decentralized teams, you cannot expect everybody to like one guy updates his pipeline and all of a sudden everybody has to jump in the company because the one guy changes by like yeah, and these type of things. I don't know if soda has it like the version.
Bart:It's very new. I like announced it.
Paolo:I'm assuming like they have some kind of version maybe not at the point of dbt, but guessing as long as you have a file and you host them on the top like how Tim is.
Murilo:Like it's been two days probably. You still don't know, like I didn't even know myself.
Bart:The contracts, whether it is data or an API or whatever. Like we know it much more in the API world, like things like the open API.
Bart:So this is a sort of like this is a contract with basically states like what I was saying like the data that is being produced should look like this and when you're consuming it it should look like this.
Bart:So it's, it's it's, I think, if I understand correctly, rather one of very slightly today this spec first, so you write the specifications more or less manually and you you push the responsibility to implement that to both the producer and the consumer. And it's interesting to to see the evolution in that because it's typically outstarts it. So I open API also started, but, for example, with open API, you now see that if you use something like fast API in Python, you just add some annotations to your Python functions and it generates a spec for you. So then the spec becomes it's not spec first, it's code first. You write the code and you basically generate the spec. So it'll be interesting to see if we also see this evolution in data contracts, indeed, because then the definition can become very close to the producer and you're not the. It's always difficult if you do spec first or you write it out, you still need to have the hygiene yourself to also actually correctly implemented from the moment that you have an implementation and the spec automatically, more or less automatically, comes out of that.
Murilo:You don't have that risk, yeah but I also think for tools like dbt, like you are kind of specifying the schema as well, right, like you do have, like you do have like demo files you can spec, because I remember even I was trying to, I tried to play a bit with it to create macros or something to make sure that I'm casting the types to what I wanted, but I didn't want to specify the types on my SQL and on my YAML. So I wanted to see if I can just kind of do it in one place, kind of the same spirit of fast API or a like. But but the developer, like in dbt, you are also writing the YAML file, right. So I think you could, like I see what you're saying, but I think also, like writing the API, the spec sometimes does fall in the developer, in this case, no, and the producer. I think that's the better terminology. Yeah, I think it's. Uh, it's cool, it's cool.
Paolo:Apologies for the interruption we're seeing like, like with fast tp. Are we moving away from spec first and data contract to code first, data contract?
Bart:well, not sure if we're moving away. We were creating the opportunity at least, because I think moving away from uh, spec first and going code first is very difficult in a system where you have a lot of different producers and a lot of different consumers, because If you then go code first and you have all these different artifacts spec artifacts coming from these different solutions that you need to bring together, which is a whole complex challenge on its own.
Murilo:True, but I think, I mean, I think these are all cool developers, I think.
Paolo:Yeah, I think it's Really cool to actually put a name on that. I don't think it's really new as a concept to have some kind of schema validation and Some people agreeing on when the data should come, but at least it's nice to have the name as a notion so that people can refer to it and say yeah. Let's do. Let's just do a data contract, and then you have all these type of things. You can plug it in, yeah, and start Discussing it.
Murilo:I agree, and maybe your. Your question team before is uh model contracts.
Tim:Yeah, like machine learning model contracts. Do you have you From? From the response you gave me, I don't think you did. Have you ever seen this in practice and do you think it's it's? Do you think it's something that we might move towards?
Murilo:I'm trying to still think how this would fit here, but I think one thing you could like version.
Tim:Let me give maybe I'll give you a more like super concrete example.
Murilo:Yes, I work better with concrete.
Tim:So you have, you have, you have an organization, um, that is super mature in data. They have, they have, like, uh, a lot like every, every, every unit, every team basically knows how to Use it's, it's hypothetical, knows how to use data and knows how to create machinery models as well. So you have just an awful lot of machinery models that they, that they produce, um, in such a case that that that some teams produce machinery models which others are using the results from, as sort of you know that they put it in dashboards and stuff like that. And At this point you're like you really need to have a way to govern all these models. Yeah, it's not just simply like in most companies, I think, right now, where you have a team that is doing all the machinery development. At that point, do you do, you like, do you introduce a data contract, the machinery model?
Murilo:I think it's like you do have model governance, right, and I think For me it makes more sense to to write contracts on the predictions, I guess, right. So it's like I mean again, I think we're restricting ourselves to tabular data here, right, because machinery models can be Images as well, which doesn't fit very nicely with the tabular data thing. But, for example, one thing that a practice that I like, a pattern that I like, is you have a table and then you're gonna have an id, user id, whatever the prediction id, and then you're gonna have a prediction that's gonna be on a table, there's gonna have a column, there's gonna be a type and you're gonna document what that should, what that should mean, and another, it was like the metadata of the model. So what's the model version? What's the thing? So if you update that and you keep making predictions, you should see a difference, even though we should mean the same thing, because the schema description is still the same.
Murilo:So, for example, let's imagine churn, right, so I have, okay, this customer will churn, and this was model one, version one. And this model version one was like you can put maybe githash or some vertex, whatever, so you can really go back to the whole. You know what model generated this. On the other hand, that id should be linked to a model governance Dashboard or something right that you can go back to. What is the model, what is the data that trained that model, so you can really make the ideas to make everything reproducible. But then if you release a v2 Of the churn model, then on the table at some point the metadata will change. So that's kind of how I've seen. That's a pattern that I like, but I'm not sure if that falls into the model contract. Maybe the model contract is in some way a bit of a.
Bart:Super set of that where you say we are a large organization and we are mature and that's some entity within the organization requires Some prediction to be done. There's not a another entity that develops a model and that within a model contract you have a basically a spec on how the model Should be built using this methodology. We expect it to be built, to be trained using that data set. We expect it to be able to have to allow these inputs. We expect the performance to be at least this level so that you have this type of Of basically a formal contract between the producer and the consumer. Yeah, I think I mean.
Murilo:At that point, like these, these type of things can also start serving for like Automatically retraining and stuff like that.
Tim:Or you if, if the model does not adhere anymore to the contract, you just trigger.
Murilo:Yeah, yeah, yeah. I'm just thinking like if the the consumer right, how much of the consumer have a say in? Like this is the data you should train the model in. You know definitely.
Bart:The consumer. Yeah, let's say, we're making a prediction about your credit worthiness. Right, say that again. I'm making a prediction about your credit worthiness, you're you're, I'm a bank you're knocking on the door, say I want a mortgage.
Bart:Yeah, the we're using a model for this. Let's say the Producing entity yeah, it's a development and machine learning team within the bank. Yes, very little domain knowledge. The Consumer is an entity that is actually Sitting around the table with you and pressing a button. I want to have a prediction on Marilla, so they know very well. Like. What type of data About Marilla should this model be? Yeah, great on.
Murilo:Yeah, I see what you're saying, but I think what I would expect or maybe I don't know Is that the data scientist should have domain knowledge at least enough to Select those things because you can talk about all the data that you're talking about. Select those things because you can talk about, like GDPR and all these things, and indeed they are constraints, right, but is this the producer that would say this? And I think, sometimes, what if you have many producers?
Bart:but we're talking a bit about, I think, the Perfect world yeah, we're building machine learning models becomes very simple, right, yeah, for the moment, it is as simple as creating a data set and then out of ML kicks in. Today, the concern is how do we create a highly reproducible model? How can we completely reproduce? How can we trust this, like today, that's where the priority goes, right, yeah, from the moment that is, quote unquote.
Paolo:Figured out.
Bart:Yeah, like then you start this, having these discussions on how do you have?
Murilo:contracts, I agree, but I think it's also because the well, yeah, I think, as you make it easier, lower entry barrier, right, I think if someone just can just walk in and strain on model, then I think you do need some more guidelines and you need to set the governance right. But I Think I don't know if I have a good answer yet. I mean, I kind of voiced my my thoughts here unstructurally, but I think I think we're walking towards something right. I think ML Ops kind of tackles the model governance story a bit and I think we're walking in the direction slowly.
Tim:Slow, I would argue even we're moving there quite fast, I think. Uh, yeah, a little anecdote on our part here is that a week ago I asked Bart, can we have this? And Within about four, like I asked him, can we, can we have, could we have an automatic prioritization of a couple of messages coming in For me? And within about four hours, bart had just completely no, no, I did, I agree I think Bart is of course, very good programmer To me.
Bart:I can pack stuff together very well.
Murilo:It's very good, I put it the first 20 percent. Well, but actually like well, maybe it's a different discussion. I don't know if you want to go too much into that, but I do think that AI is getting easier and easier to do, right? I think it's like, especially with gbt and auto ml and all these things, it's like, yeah, it is getting easier getting something working.
Bart:It's very easy, exactly. And now since a few days, demo's been asking me yeah, maybe you can change this me again. They're kind of ignoring that.
Paolo:But there is something working. I'm busy.
Murilo:No, but I mean I think that indeed we're going super fast. I think it's making it's getting so easy to to just build a model, right. I think what I mean it's been more like so slower. Is the the governance part, like you asked? Like is there a model contract? Like, yeah, like these questions should the producers have a say in the model that is trained? And maybe they should, but, like today, maybe that's not the case. Or how much follow-up do we?
Paolo:have data governance Is always the last thing to be implemented, right? I mean, when you see some companies that have like 100 of gigabyte and not 100, but like trillions of gigabyte of data and they don't have data quality, that's. Yeah you know in in the end, okay, they can work without it, but at some point they still have to look at it.
Murilo:Yeah, operational stuff If it is the like. If you don't have, if you have two models in production, maybe you don't need a full-blown monitoring system, right, you can just have a guy that just goes there every week, he just looks at it, right. But at the point you have Dozens, hundreds of models, then you probably need something more. I mean, yeah, I fully, I fully agree with that, but I think, indeed, as AI gets easier and easier to do, we have this tendency to have more and more stuff, and then this, this issue, becomes more relevant, like these questions become irrelevant 100.
Paolo:I think, with the regulation that are coming in, a need for governance is starting to build up.
Bart:Fair point yeah.
Murilo:Um, are we okay to keep the ball rolling Apparently?
Paolo:have kicked the ball, do it.
Murilo:No Shoot the ball team shoot the ball.
Tim:Yeah, that's why it's way nicer.
Murilo:We talk a lot about AI and data, but everything is supported by programming languages, right and uh. Apparently, there's a program language that aims to become a hundred year Program language. Do you know something about that, bart?
Bart:Um, it a little bit across my feet, thought it was an interesting, uh, an interesting viewpoint.
Murilo:So the hair programming language hair is H-a-r-e for uh, it's not hair, like is in there the top of your head? Yeah, is there a different pronunciation? I don't think so, but that's why I wanted to spell it out for the.
Bart:I did not. The hair programming language looks a little bit Uh hope I'm not gonna get canceled, for it looks a little bit like go.
Murilo:Oh, just browse through.
Bart:Hope I don't create any drama, yeah, but uh, um, they aim to become a hundred year programming language and the the identified a number of points and we'll we'll link them in the show. Note the article about uh, I'll just list them here conservatism in the language design, the importance of the standard, necessity of feature freeze To define a long-term api stability goals and to foster a culture that values stability. And I was wondering Don't you set yourself up for failure if this becomes your goal?
Murilo:because, right, because you're just like saying we're not gonna do anything, so we're gonna stay here, we're good.
Bart:I mean what? But you're gonna, you're gonna go to a like. I think then your goal is to go to move to a certain maturity, to a certain performance, or whatever you want to call, to search a certain feature set and then stabilize there. Yeah, it's weird too.
Paolo:That's a programming language. Wants to stabilize in an environment that is always evolving.
Bart:Well, yeah, that's what I'm wondering is like. Is it is something like this Is In this day and age where this goes so quick?
Murilo:maybe another question before that, like what is a hundred year programming language? Is it just like, because when there's a program language dies, like I mean, even if no one uses it, like do they mean they want people to use it? Well, I think that is the objective. Yeah but it's like how many people? Because if it's the guy that wrote this article that is using it, and then maybe his son. Yeah, like yeah you can have a program language that just does hello world.
Bart:So they actually have those very short definitions. I know all the Explain it. So they want to build a language such that you can write a program on the day that's here 1.0 or so least, and that you can still build that program a hundred years from now.
Murilo:I see.
Bart:Which is an interesting premise, right? So that means that you are, from that definition at least, fully compatible with how the language looked like a hundred years ago.
Tim:Do they mention something on on which machine you need? To be able to build it because, uh, they're like a sub player. I don't, I don't know, but like if I, if I were to, were to try to build something with uh I don't know how to say this in the In English actually like the, the punch cards.
Tim:Was also a form of programming way way back. I think I can still build the program I could. I could create a machine which I couldn't. Somebody could build the machine that still builds the programming language. Does that mean? I think it's really.
Murilo:I mean, but that's kind of what I was thinking as well.
Murilo:I was like, if there is like a handful of people using it and there's no new features, but yeah, but there's only a handful of people and that's that, that can be a easy success, right, like uh, I feel like to me is like the issue is when you want people to adopt this more, right, because people are gonna want more features, I mean, maybe I know you, I know what you're gonna think part, but like rust, they also, because they also say that right, like they're, they seem to have a commitment to never go into a two point whatever, and like I heard that they make changes and they like what's a breaking change?
Murilo:I think it depends a lot on the program, right, and they run on the cargo, all the, all the packages. They have to see what breaks and see this, but they always try to make it backwards compatible, right, so I see their definition, but I think it really hinges on how many people are actually going to be using this right, because if it's, Well, but that's a bit what I mean, right, like, are you setting yourself up for success or failure with this type of approach?
Bart:If this is your, if you're still creating a programming language from scratch and this is your premise to start from, does that also mean that it will always stay small?
Murilo:Indeed, I mean maybe, maybe, but I think it's well. I see the challenge, right, Like if you want to go 100 years and you want to be able to build the stuff from 100 years ago and you still want to have a live community, I think it is a challenge, but and I can see the reason why right, it's like they also advertise this in Rust. It's like, yeah, there will never be a two. So it's like, don't worry, whatever you wrote today will be running forever. Blah, blah, blah, but uh.
Tim:Do you think the designers of the language went and had a look at like C, because they specifically referenced C, huh, and do you think they went and had a look at C and be like okay, what are the elements that make C still so relevant today?
Paolo:How old is C?
Tim:by the way, that's a good question Is anybody walking in Cyclopedia right now?
Paolo:No, we don't have that Okay 60.
Bart:In the early 1970s, 50 plus. Yeah, which is crazy old in this day and age.
Tim:Do you think they had a look at the language and be like? Well, conservatism in language design is what makes C and C and that's why everybody's here, or, but I would probably.
Bart:Also there's like Now I'm saying stuff that might not hold, but if I have a piece of C code that I developed in the 1970s and try to build that on a C compiler today, it would probably not work.
Paolo:Yeah, yeah, because.
Bart:Question, mario, not, so I would be surprised. If it does, I would be very surprised yeah, let's try it out.
Tim:Yeah, but I think the Well yeah.
Bart:Interesting thought experiment.
Murilo:I think so. I see the challenge. I think, uh, I'm curious to see how it plays out, but I do think it's To be alive. To me, there used to be a more clarity on what do they mean, like, how many people are using this Right? Let's see they can prove it's wrong, huh. You need to make the goal 100 years 50 years from now.
Bart:There are three organizations, Maybe four Google, Facebook and Hair Inc.
Tim:Cool, cool, cool, cool, cool. And then this article is going to be like the Bible Everybody's ready.
Bart:Like rust, move to toodal-do.
Paolo:Everything broke. Murillo found dead. Yeah, yeah, yeah, no, but what's the aim of this programming language, like with Python, specifically, not specifically, but like people can pick it up and start building stuff? With Rust, you're trying to find some stability in what you're building, but with hair, what's the plus side of using hair instead of colon, for example? It's a good question.
Bart:And I don't know the answer, but I think from here I'd really like the. If your concern is I want to build something that I can Rust, that will always run and build, even 10 years from now, 15 years from now. I'm having a hard time coming up with a use case, but that is a bit of the premise that I'm making.
Murilo:Yeah, I think it's interesting. Like I kind of say, hair is designed to be a boring language, Like boring by design.
Tim:And another quote is hair as a language is not actually particularly interesting or innovative. That's sad.
Paolo:They have a way to sell it.
Tim:This is not built by a business developer.
Murilo:Shut up and take my money.
Tim:This will keep all the Rust fanboys away.
Murilo:I already turned it. I already closed the tab.
Tim:So they specifically mentioned here. Our goal is to make hair the tool of choice when writing a program which needs to be operational and maintainable for a long time, such that all of the design choices still make sense within a decade or two of hindsight. That's their business case. I don't know.
Murilo:What a real-world scenario would look like, but I think maybe they need to know more about the language to see.
Tim:Should we do an adventoof code in hair? Do it.
Murilo:No, I'm not going to stop you.
Tim:That sounds like fun.
Bart:Yeah, what's this? A promise? Do an adventoof code.
Tim:It's not a promise.
Murilo:January 1st we'll have Tim on the podcast. Share his experience.
Tim:Or to completely shame me.
Paolo:This is just like a session of like shame.
Tim:How far did you get First day, day three, tim quits data I try to install it.
Murilo:All right, all right, all right, and yeah. So I guess now, if no one else has anything to say, say now or forever, hold your, I forgot the piece. Now we can go to the game part of the show. Are you familiar with the game? Part of the show?
Tim:I thought you said gang, part of the show, the gang. Yeah, now we're going to take the show. This is where we're going to start. No, I don't know, yeah.
Murilo:Yeah, okay, but this is quote or not quote. Do we have a sound? Yeah, so if you're not, you guys you know what I'm talking about. No, no idea. No, it's the Ye or Gen. Ye, yeah, but I was trying to. You should have said yes we listen every time, so I know what. We know what it is about.
Bart:I know, but I was going to explain it anyway. It was for the listeners right, yeah, okay, good one.
Paolo:Probably so.
Murilo:Yeah, he thinks that he's a.
Bart:He's a podcaster.
Murilo:Yeah, shit. So the idea is we have a famous person, someone that everyone on the table should know, and then we have a real quote or something that the person said or produced, and fake quotes generated by LLM, copyright, whatever, and then the winner. So basically you have to guess which one is the real quote, and then we go around and then the winner actually is the one to set up the game For the next iteration.
Tim:Yes, what if you generate accidentally a quote that the person said Wow, that's an edge case Are you often questioned on your why did? You say president.
Paolo:Do you have?
Tim:inside information.
Paolo:Did I say president? I don't know.
Murilo:But I have actually two individuals, two characters today and I mixed the game, the rules, a bit up. So I think last time Kevin brought three people and two quotes for each person and I have two people but three quotes, three quotes, so two fake and one real. And I don't want to brag, I don't want to, you know, call my chickens before they hatch, but I think I did a pretty darn good job just saying so.
Murilo:The first person is a famous rapper, dog Snoop Dogg. So are you guys ready? Are you guys paying attention? Do I have your full, undivided attention? This is going to be an explicit quote.
Paolo:No, did you see the news about this, about Snoop Dogg DOG.
Bart:Yeah, no, it's stopping weed. No, oh, really yeah.
Paolo:This is my first time I've been to the game.
Bart:I've been to the game before, oh really.
Paolo:This is fake news.
Bart:That's the fake quote.
Murilo:Actually I should have put that there, If there was something like that. No, believe it.
Paolo:Yeah, there's no way, Really really.
Murilo:He issued a really nice photo of him. I think like this, like praying kind, there's no drugs. I know, paolo, giving your background, I don't need drugs. On the quotes to you know, play with your feelings. I hope there is no coffee. No, no coffee, no, nothing. But it is about the future. So first quote is and again there are three when I hang up the mic, I want to be the CEO of Snoop's Paws Claws, a doggy spa that's off the leash. Can you maybe wrap it?
Bart:I can see if it.
Murilo:I'm sorry, I cannot In the style of Snoop Dogg.
Tim:Yeah, yeah, no.
Murilo:So that's the first quote. Second quote is when I'm no longer, oh, sorry. When I'm not longer, no, no longer sorry. When I'm no longer rapping, I want to open up an ice cream parlor and call myself Scoop Dogg. That could be after a week, maybe. And then the last one is picture this Snoop Dogg, the gourmet chef, who's the chef of the fish in the culinary game.
Bart:I did right. He has a cooking book.
Murilo:Yeah.
Paolo:But did he say that?
Murilo:I received it. I believe the name is Snoop from cook to cook. Yes, I'm assuming.
Tim:I'm guessing. I feel like this was one of Data Roots' Christmas gifts if everybody received it.
Murilo:And Bart was the one that chose it.
Paolo:For sure.
Murilo:Yeah, exactly. So who wants to go first? What do you think is the real quote? So there's two fake one real, Maybe Bart has. He's making intense eye contact at the time for the listeners, so I'm going to guess Bart has. I'm going to go for the second one as fake.
Bart:There's one for there's two fake.
Murilo:And the first two, First two are fake, so the last one is real. So Bart says picture this Snoop Dogg the gourmet chef, the culinary game.
Paolo:That's what you're guessing, Paolo, I'm going to say the second one is real.
Murilo:So Paolo says the second one is real.
Tim:This is going to work out real nice but I'm going to say the first one, just because I want to believe. Snoop Dogg at some point, and this way there is.
Murilo:There is only one winner right. Oh, there's another round so we have another. So you think that when I hang up the mic, I want to be the CEO of Snoop's Paws and Claws a dog spa that's off the leash? Yes, that sounds like fun. So that's what you think. Paolo thinks that when I'm no longer rapping, I want to open up an ice cream parlor and call myself Scoop Dogg.
Tim:That's actually Rupert Grint, right.
Paolo:Ron Weasley. Yeah, he opened up.
Tim:It's just like driving around with an ice cream truck.
Paolo:It was nice 10 years ago. Now it's creepy.
Murilo:And the last one. I think Bart thinks that it's pictured this Snoop Dogg, the Gurmechev, serving up the dankest dishes in the culinary game. So, bart, I must tell you that you are wrong.
Paolo:That is not.
Murilo:Sorry, good try, nice try, but I did do a good job petting myself in the back. When I'm no longer rapping, I want to open up an ice cream parlor and call myself Scoop Dogg. Paolo, you thought that was the one Correct Applause. To be honest, I saw this on social media and I tried to verify that it's true. I found some references online and I was like okay, I found enough references that I think it's true, but I couldn't really find the source, which means that I did a quite issue rise.
Paolo:It's me.
Tim:I'm the problem.
Murilo:Which means that, tim, you are not right either. I'm sorry, tim. Alright, round two, round two. This one, I do know it is. I have checked the source, so this is a tweet, actually from Elon.
Paolo:It's commercial these days, I know.
Murilo:And that's the show. So here it goes. First quote that's one. Second quote is that's quote two.
Tim:I feel like Elon's soul of the charts these last couple of months.
Murilo:Can I say anything? I'm not sure if I picked it, so maybe let's do reverse order now.
Tim:So, tim, this is to be very clear. This is not a trick question in all three years.
Murilo:That would be good. I'll keep on mine.
Tim:I feel like the first one is actually. He did do this, like he did create Flankterrow. He created and I know there's a money bag. I'm going to go for the first one again.
Murilo:Okay.
Paolo:And Bart Bart did just peer pressure.
Murilo:Last time it was like no, no, but I don't know if he tweeted it.
Tim:It doesn't speak anymore.
Paolo:I remember something like this, but it might have been in a YouTube video or something. But all three of you are Correct.
Tim:He did Good job.
Murilo:I think we're going to have a good one. I think we're going to have a good one, good job. I think from, to be honest, I didn't think I did a bad job, but I think the fact that you guys kind of knew about something like this is what gave it away.
Tim:So I'm not I think my strategy sounded. I feel there was a high potential for creating a trick question when he sort of said something like that in a video and then you sort of just yeah, yeah indeed, but I can tell you my prompty strategy.
Paolo:Is that interesting, this feedback?
Murilo:Yes, yes, yes, thanks. Do my valuation please? No, I also. My strategy was first I asked some, I asked strategy PT for information about the person. So they come up with like a little bio and I just say, okay, you're this person. Now it's because then it's easier to Actually.
Murilo:But it's funny because for Elon Musk I had to tweak my prompt a couple times because it's like I'm not that guy, because I say like, oh, you're that person and we're friends, right, we make jokes and blah, blah, blah. And it's like, oh, I can, I'm definitely not Elon Musk, but I can pretend that I am for you, blah, blah, blah. But like I didn't like that right, so I had to tweak it a bit, like pretend you're a person like that guy or whatever. Then it accepted and then I say, hey, for Snoop Dogg, I think. I ask what would you do in the future? And for Elon Musk I ask what would you do on a zombie apocalypse? And then he and I actually ask for 10 quotes and I just picked the ones I thought was most realistic or most fun. Did you validate that?
Tim:these tweets don't exist. I did not. Also, I feel like just like unleashing this into the ether is just explaining to people how they can set up like fishing schemes. Maybe, yeah, but did you see the ad on?
Paolo:YouTube with Elon Musk talking at the conference and saying, hey, I'm Elon Musk, but it doesn't really sound like him. No, it's the real, all right. So I'm going to go ahead and say it again no it's the real.
Murilo:All right, but then. So I guess all three of you got it right for the second one, but Paulo was the only one that got it right for the, so I'll be here next week replacing Moorillo.
Tim:That's why he's the quality I know right.
Murilo:It's scary. Fair enough, I think you can. Definitely. You can send me, or you can send Bart. No, you can send Bart. No, you send me. Send it to me. I'll let you send me a famous person in quotes and then we'll honor you.
Tim:How's that? I thought you were going to ask him to send in the candidacy for hosting this podcast.
Murilo:I mean you can send it.
Tim:That's a huge conflict of interest, See I know it's interesting, just made it awkward.
Murilo:I mean, you can send it, but I'm not going to look at it. You need 25 years of experience. Sorry, all right, but that's it. Is that it? Can we call it a show?
Bart:Last thing we have With Datrude we have a shop. If you go to our website, datrudeio, on the upper right top Same Upper right corner Shopping icon you can go there. You can purchase whatever you want for 25 euros, because we are releasing a voucher that can be used by three people, which you can use with the discount vote. Murilos underscore, bargain underscore blast. Murilos, bargain blast. We'll link it in the show notes and you can go wild Bananas. I am giving you an interesting tidbit for all the Murilo fans here and a host of our show, the almost OG Muck, very prominently features the one and only Murilo.
Bart:Well, but I just wanted to put the last one in, we, sure it's Murilo. I think this is a discussion for another time, right Tim.
Paolo:I'll get into that?
Murilo:Thanks, bart. I guess that's it, thank you all Thanks.